Evaluating the Efficiency of Restricted Pseudo Likelihood Estimation in Balanced and Unbalanced Clustered Binary Data Models
DOI:
https://doi.org/10.37376/sjuob.v36i2.4299Keywords:
Hierarchical Generalized Linear Model, Restricted Pseudo Likelihood, Balanced Clustered, Unbalanced ClusteredAbstract
Clustered binary data analysis is a common task in various fields, such as social sciences and epidemiology. Restricted Pseudo Likelihood Estimation (PLE) is a widely used approach for analyzing clustered binary data, providing flexibility in handling complex dependencies within clusters. This study aims to evaluate the efficiency of Restricted Pseudo Likelihood Estimation in balanced and unbalanced clustered binary data models. Using simulated data, we compare the performance of PLE in balanced and unbalanced clustered binary data scenarios. We consider various factors such as the number of clusters, cluster sizes, and intra-cluster correlation. The preferred class of models for clustered binary data is the Hierarchical Generalized Linear Model (HGLM). This article compares the performance of a restricted pseudo-likelihood estimation method of the Hierarchical Generalized Linear Model (HGLM) with equal and unequal cluster sizes. Through comprehensive simulation experiments, we assess the accuracy and precision of PLE estimates in terms of parameter estimation, standard errors, and hypothesis testing. Our findings provide insights into the efficiency of Restricted Pseudo Likelihood Estimation (RPLE) in balanced and unbalanced clustered binary data models. The results highlight the advantages and limitations of PLE in different scenarios, aiding researchers in selecting appropriate modeling approaches for their specific data characteristics. The results can guide researchers in making informed decisions regarding the selection and application of PLE in their own studies, ultimately enhancing the validity and reliability of statistical analyses in the presence of clustered binary data.
Downloads
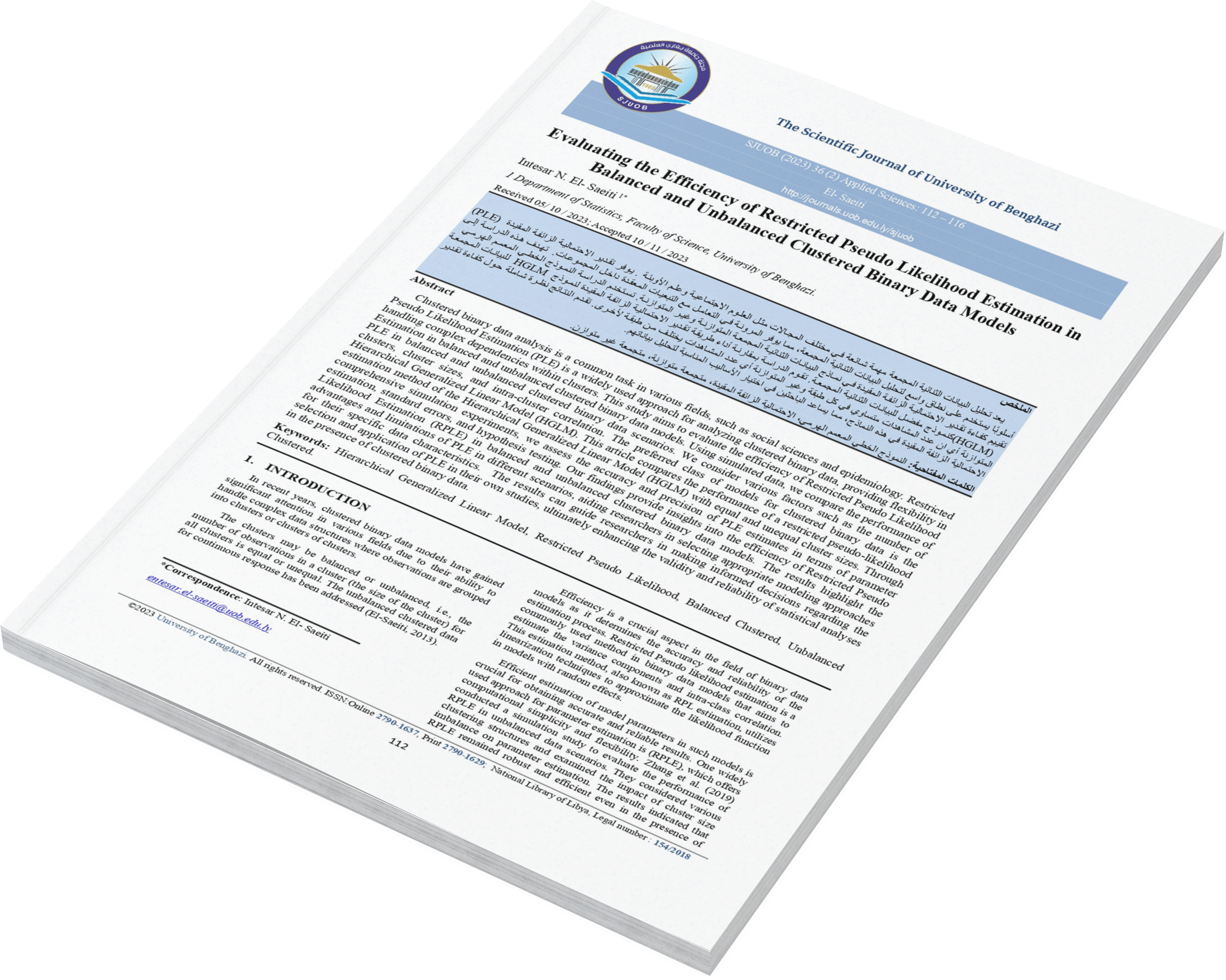
Downloads
Published
How to Cite
License
Copyright (c) 2023 The Scientific Journal of University of Benghazi

This work is licensed under a Creative Commons Attribution-NonCommercial-NoDerivatives 4.0 International License.