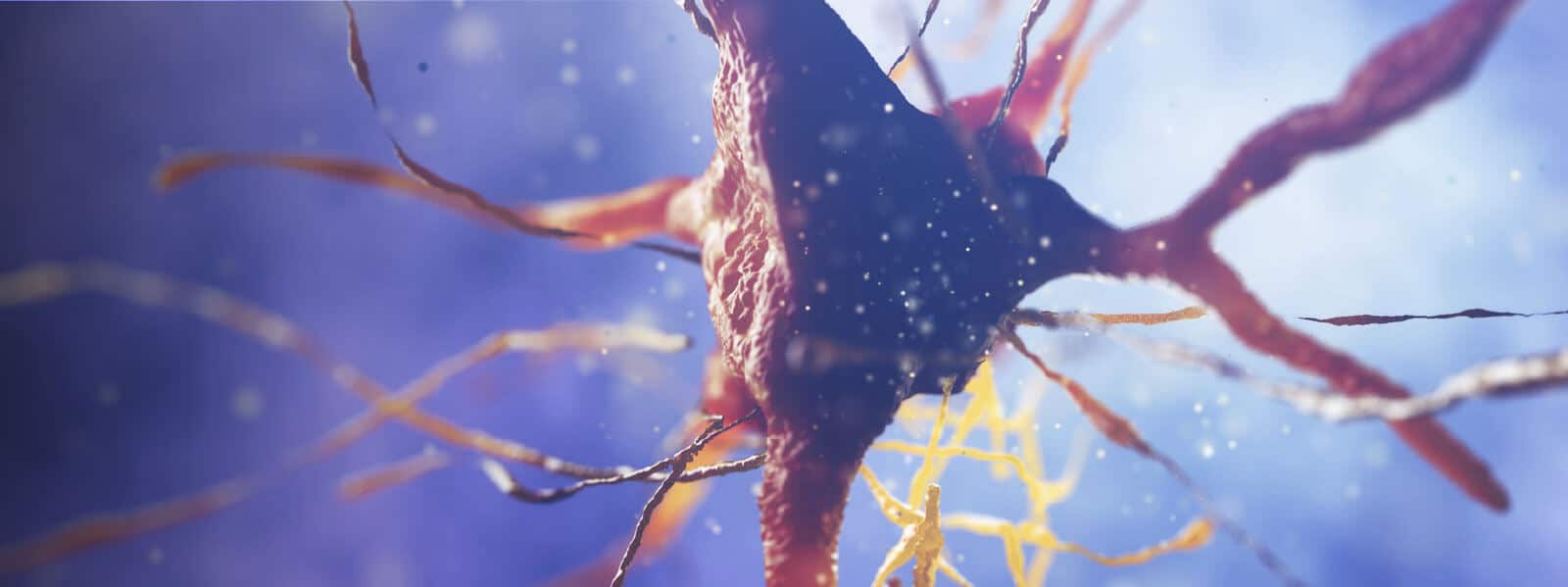
Physiology News Magazine
Is the motor system at rest while we are sleeping?
A commonly held belief is that the motor system is at rest in sleep, because we barely move – and if we do move it is often pathological. But this assumption might not be entirely true. There is evidence that elements of the motor system remain functionally coupled in sleep in ways specific for each sleep stage, perhaps to allow physiological movements in sleep and to facilitate motor learning
Features
Is the motor system at rest while we are sleeping?
A commonly held belief is that the motor system is at rest in sleep, because we barely move – and if we do move it is often pathological. But this assumption might not be entirely true. There is evidence that elements of the motor system remain functionally coupled in sleep in ways specific for each sleep stage, perhaps to allow physiological movements in sleep and to facilitate motor learning
Features
Farid Salih & Pascal Grosse
Klinik und Poliklinik für Neurologie, Charité-Universitätsmedizin Berlin, Augustenburger Platz 1, 13353 Berlin, Germany
https://doi.org/10.36866/pn.76.15
With the advent of more refined methods to study the physiology of sleep it gradually became evident that sleep is associated with highly dynamic changes in cerebral activity. A variety of different brain rhythms (i.e. oscillations) have been extensively described in simultaneous recordings from cortical and thalamic neurons in naturally sleeping animals (Steriade, 2006). These rhythms orchestrate the cyclic occurrence of non-rapid eye movement sleep (including slow-wave sleep) and rapid eye movement (REM) sleep across a night. At sleep onset three cardinal rhythms – sleep spindles (12–17 Hz), delta (1–4 Hz) and slow oscillations (0.5–1 Hz) – are generated in cortico-thalamic networks. As a common feature, all these rhythms are associated with prolonged hyperpolarisation of thalamocortical and neocortical neurons which inhibit the cortico-petal transmission of sensory signals, and thus allow the cortex to disconnect from the rest of the brain and the individual to effectively fall asleep (Steriade, 2006). In humans, the same oscillations have been identified to be highly coherent across different cortical sites during non-REM stage 2 (N2) and slow wave sleep (N3) (but not in REM), as revealed by frequency analysis of the scalp electroencephalogram (EEG) (Achermann & Borbély, 1998). In fact, the spontaneous generation of these rhythms has challenged the previously held assumption that neuronal networks are ‘inactive’ during sleep. Current investigations now address the question of whether these rhythms play a functional role for learning and cognition apart from just initiating and maintaining sleep.
In our recent study we asked how these sleep-specific brain rhythms translate into the functional organisation of the motor system (Salih et al. 2009). As a change circumscribed functional network. A novel approach to study a functional neuronal network in human sleep is to record surface EEG along with local field potentials from depth electrodes in patients who undergo deep brain stimulation (DBS). While the electrodes are externalised it is possible to record signals from the DBS target areas. For our investigation, we studied seven patients undergoing DBS of the globus pallidus (GP), a motor element in the basal ganglia loop, to treat dystonia. With simultaneous recordings of local field potentials from globus pallidus and regular scalp EEG in N2, N3, REM and wakefulness, we used a set of methods pertaining to frequency analysis to look into the functional coupling between globus pallidus, motor and visual cortex. Frequency analysis investigates neuronal oscillations and is based on the cross-correlation between two separate signals in the time and frequency domain (Grosse et al. 2002). The most important measures applied in our study were coherence and the directed transfer function (DTF). Coherence is the principal measure of the linear dependence or correlation between two signals in the frequency domain. It is mathematically bounded between zero and one, where one indicates a perfect linear relationship and zero indicates that the two signals are not linearly related at a particular frequency. The DTF investigates any possible asymmetry in the flow of coherent activity between signals (Kaminski & Blinowska, 1991). Where the DTF of coherent activity at two recording sites is asymmetric, the ‘effective direction of coupling’ predominates in one direction. However, neither coherence nor an asymmetrical DTF necessarily implies a direct connection between the two areas in which activity is recorded. Thus, information can be transferred indirectly between recorded structures, via one or more unrecorded structures, or activity in both recorded structures may be driven by a third unrecorded structure.
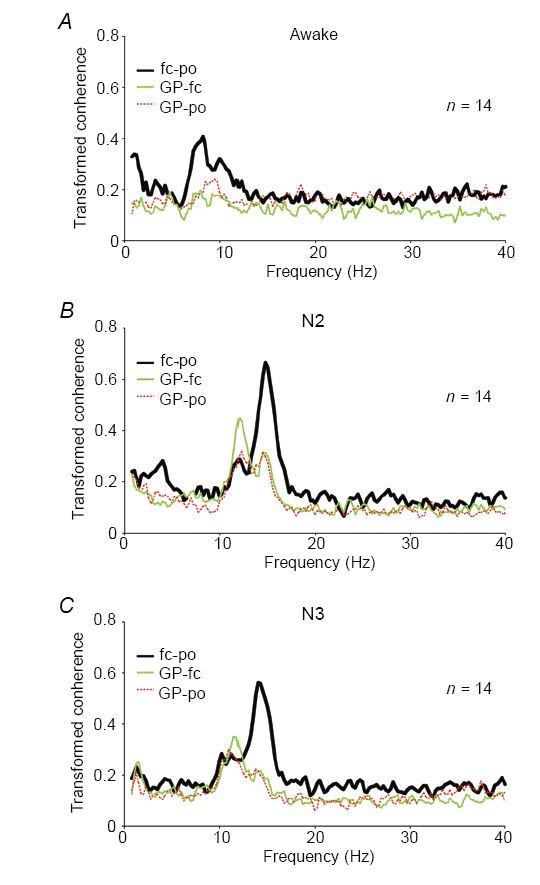
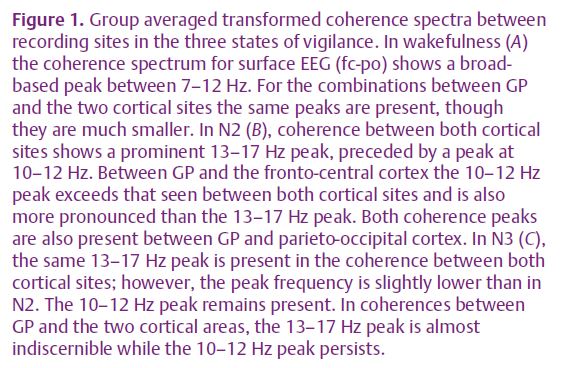
In our study, we could identify significant coherence between globus pallidus and motor cortex as well as between globus pallidus and visual cortex in frequency bands specific for sleep (i.e. 9.5–17 Hz in both N2 and N3, 0.5–4 Hz in N3, Fig. 1A–C). Importantly, there appear to be two different physiological activities represented within the broad band of significant coherence from 9.5 to 17 Hz in non-REM sleep. As expected, there was a component in the range of sleep spindles with a maximum centred at ~14 Hz. This was best seen in the coherence between motor and visual cortex as well as between globus pallidus and both cortical sites during N2, and between motor and visual cortex in N3. The DTF suggested that the spindle frequency component was preferentially driven from visual cortex (Fig. 2A–C). The second component consists of an activity in the α-band (9.5–12.5 Hz). This coherent α-rhythm was elevated especially between both components of the motor system, i.e. globus pallidus and motor cortex. The DTF further suggested that this α-rhythm consisted of a preferential drive from globus pallidus to the motor cortex in N2 and a more symmetric drive in N3.
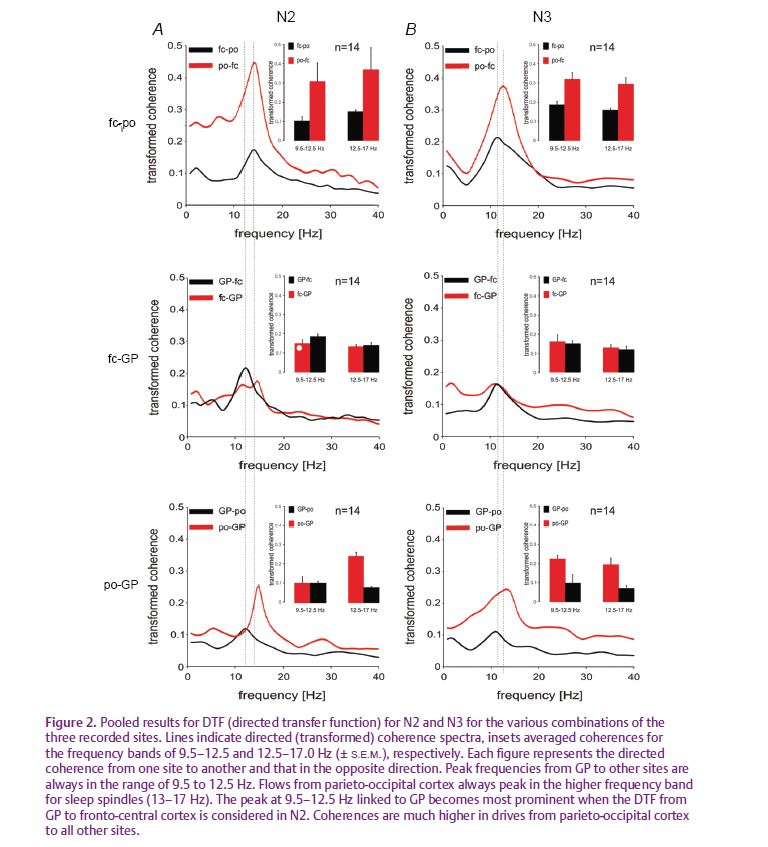
Based on the extensive evidence from intracellular thalamo-cortical recordings in naturally sleeping animals, the coherence patterns we identified between globus pallidus, motor and visual cortex in the range of sleep spindles are most probably explained by a common ‘third’ generator, namely the reticular thalamic network. In contrast, our results for coherence in the α-band are consistent with more direct functional coupling between globus pallidus and the motor cortex in non-REM sleep. It has long been established that α-rhythms can be a feature of non-REM sleep in surface EEG, particularly during slow-wave sleep. However, until recently the significance of this α-rhythm had been unclear. Our data now link the α-rhythm more specifically to the motor system as its potentially specific signature. Focussing on δ-waves and spindle rhythms, other studies have recently associated these oscillations to brain networks involved in memory consolidation (Huber, 2004). Functionally, procedural memory formation may require slow-wave sleep rhythms, and also training on a declarative task increases sleep spindle density significantly (Gais et al. 2002). From a physiological point of view, the strong coupling between motor cortex and the basal ganglia in the α-frequency band might indicate, by analogy, the reshaping of synaptic plasticity in motor circuits and the levelling of the amount of movements while motoneuron activity is largely reduced during sleep. With a clinical perspective in mind, it is also tempting to hypothesise that modifications of this α-rhythm are somehow involved in the generation of the many sleep-related movement disorders.
References
Achermann P & Borbély AA (1998). Coherence analysis of the human sleep electroencephalogram. Neuroscience 85, 1195–1208.
Gais S, Mölle M, Helms K & Born J (2002). Learning-depending increases in sleep density. J Neurosci 22, 6830–6834.
Grosse P, Cassidy M & Brown P (2002). EEG-EMG, MEG-EMG and EMG-EMG frequency analysis: physiological principles and clinical applications. Clin Neurophysiol 113, 1523–1531.
Huber R, Ghilardi MF, Massimini M & Tononi G (2004). Local sleep and learning. Nature 430, 78–81.
Kaminski MJ & Blinowska KJ (1991). A new method of the description of the information flow in the brain structures. Biol Cybern 65, 203–210.
Salih F, Sharott A, Khatami R, Trottenberg T, Schneider G, Kupsch A, Brown P & Grosse P (2009). Functional connectivity between motor cortex and globus pallidus in human non-REM sleep. J Physiol 587, 1071–1086. http://jp.physoc.org/content/587/5/1071.long
Steriade M (2006). Grouping of brain rhythms in corticothalamic systems. Neuroscience 137, 1087–1106.