- 1Xiyuan Hospital, China Academy of Chinese Medical Sciences, Beijing, China
- 2College of First Clinical Medicine, Shandong University of Traditional Chinese Medicine, Jinan, China
- 3Wangjing Hospital, China Academy of Chinese Medical Sciences, Beijing, China
Background: The Yizhiqinxin formula (YZQX) has been used to treat Alzheimer’s disease (AD) or major depression disorder (MDD). However, its specific underlying mechanisms and therapeutic targets remain unclear.
Methods: The ingredients and putative targets of YZQX were screened using the TCMSP and Drugbank databases. Next, the GEO database was used to retrieve relevant differentially expressed genes (DEGs) in AD or MDD and normal tissues. The PPI network was established, merged, and further screened to identify the main ingredients and core targets of YZQX against AD and MDD comorbidities. We performed enrichment analysis of core targets to identify biological processes and pathways. Finally, AutoDock software was used to validate the binding affinity between the crucial targets of direct action and their corresponding ingredients.
Results: A total of 43 ingredients were identified from YZQX, of which 43 were screened to yield 504 targets. By establishing the PPI network, 92 targets were regarded as targets of YZQX against AD and MDD comorbidities in the core network. Promising targets (HSP90AA1, ESR1, AKT1, VCAM1, EGFR, CDK1, MAPK1, CDK2, MYC, HSPB1, and HSPA5) and signaling pathways (PI3K-Akt signaling pathway, ubiquitin-mediated proteolysis, MAPK signaling pathway, etc.) were filtered and refined to elucidate the underlying mechanism of YZQX against AD and MDD comorbidities. Molecular docking confirmed the ingredients of YZQX (quercetin and kaempferol) could bind well to multiple crucial targets.
Conclusion: The ingredients of YZQX, such as quercetin and kaempferol, might treat AD and MDD comorbidities by acting on multiple targets and pathways.
Introduction
As a complex, multifactorial, progressive neurodegenerative disorder, Alzheimer’s disease (AD) is a major cause of dementia, resulting in the dysregulation of cognitive function, daily behavior, memory, and so on (Alexiou et al., 2019; Fiorini et al., 2020; Li et al., 2020). According to data from the Alzheimer’s Association Report, approximately 50 million people worldwide suffered from AD in 2018, and the number of AD patients is estimated to increase to 152 million by 2050 (2021). The gradually increasing morbidity of AD leads to an enormous economic and social burden, posing a significant challenge for both individuals and society (Uzun et al., 2011; Fransquet et al., 2018). Currently, therapeutic methods for AD included not only drug therapy but also non-pharmacological treatments of immunotherapy, natural products, and so on (Alexiou AT et al., 2019). It is well known that major depression disorder (MDD) is associated with an increased risk for onset of several neurological conditions, such as AD (Hayley, 2021). MDD is the most common psychiatric comorbidity in dementia, affecting approximately 50% of patients with AD (Chi et al., 2014; Romano et al., 2014). MDD is a heterogeneous disorder and accompanied by the primary symptoms of low mood, anhedonia, and loss of energy (Chi et al., 2014; Romano et al., 2014), as well as psychological signs and symptoms (American Psychiatric Association, 2013). An increasing body of evidence has shown that MDD could trigger, accompany, or aggravate the clinical hallmarks of dementia while accelerate cognitive decline, decreasing quality of life, and increasing mortality in patients with AD (Bennett and Thomas, 2014). Given these known associations and similar mechanisms of pathogenesis between depression and AD, it is necessary to reveal the biological mechanisms and develop effective treatment.
In recent years, increasing interest has been devoted to the use of traditional Chinese medicine (TCM) to prevent or treat chronic diseases because of their lower toxicity and more significant curative effects (Chao et al., 2017). YZQX formula, also known as Fuzheng Quxie Decoction, is a traditional Chinese herbal formula composed of three therapeutic herbs, a radix of Panax ginseng (Chinese name, Renshen), the rhizome of Coptis Chinensis (Chinese name, Huanglian), and Chuanxiong Rhizoma (Chinese name, Chuanxiong). Data from previous studies revealed that the YZQX formula could enhance learning and memory ability in SAMP8 mice and reduce tau hyperphosphorylation (Yang et al., 2017). This formula has also been shown to improve cognitive impairment and alleviate Aβ deposition by activating autophagy and the mTOR signaling pathway in vivo and in vitro (Yang et al., 2019). It is worth mentioning that ginseng treatment induces a variety of effects, including anti-depressive effects, in addition to suppressing or delaying the neurodegenerative process (Ong et al., 2015; Hou et al., 2020). In addition, Lee et al. studied the active ingredients of the YZQX formula, such as berberine, and found that they also exhibited antidepressant activities (Lee et al., 2012). However, at present, TCM plays a role mainly by acting on multiple ingredients, targets, and pathways. The specific mechanisms by which the YZQX formula prevents the co-occurrence of AD and MDD are still unclear.
Network pharmacology is a novel and powerful tool that could clarify the potential mechanisms of multiple ingredients, targets, and pathways of TCM (Berger and Iyengar, 2009). Molecular docking is a computer-aided drug design method that is frequently used to predict interactions and binding affinity between proteins and ligands (Kitchen et al., 2004). In recent years, molecular docking has grown into a popular method to explore and research TCM and their targets. The previous study has adopted molecular docking to explore the interaction between donepezil and human transferrin, which contributed to aid in having a better understanding of the activity and mechanism of protein and drug binding (Shamsi A et al., 2020). Therefore, in the present study, we adopted an integrated method of network pharmacology and molecular docking combined with high-throughput sequencing data to search for common targets and pathways for YZQX in the treatment of AD and MDD comorbidities and to mine the related biological mechanisms and signaling pathways. A flowchart of our study is presented in Figure 1.
Methods
Active Ingredients Identified by Yizhiqinxin Screening
Oral bioavailability (OB) and drug-likeness (DL) are the two main parameters of the absorption, distribution, metabolism, and excretion (ADME) model (Barton et al., 2006) that affect drug absorption throughout the gastrointestinal tract. Hence, by using “Renshen,” “Huanglian,” and “Chuanxiong,” as keywords, we identified the active ingredients of the YZQX formula, screening for compounds with oral bioavailability (OB) ≥30% and drug-likeness (DL) ≥0.18 in the Traditional Chinese Medicine Systems Pharmacology (https://tcmspw.com/tcmsp.php) (Ru et al., 2014).
Collection of Putative Targets
After screening the active ingredients of the YZQX formula, the next significant process was to identify their corresponding targets. The putative targets of the active ingredients in the YZQX formula were identified and collected using the DrugBank database (https://www.drugbank.ca/) (Law et al., 2014). Further, all of these obtained targets were limited to the species “Homo sapiens” in the Uniprot database (https://www.uniprot.org/) (2017). In addition, we used the Cytoscape 3.7.2 software to construct and present a Herb-ingredient-target network of the YZQX formula. In this network, nodes represent formulas, herbs, ingredients, and targets, while the edges represent their interaction relationships.
Search, Identification, and Analysis of Differentially Expressed Genes in Alzheimer’s Disease and Major Depression Disorder
The microarray data of GSE63061 and GSE98793 were downloaded from the GEO database (http://www.ncbi.nlm.nih.gov/geo/) using their microarray platforms, GPL10558 (Illumina HumanHT-12 V4.0 expression bead chip) and GPL570 (Affymetrix Human Gene Expression Array), respectively. Among them, the GSE63061 dataset contained 139 AD samples and 134 normal samples, and the GSE98793 dataset contained 128 MDD samples and 64 normal samples. Thereafter, the probes were converted into gene symbols according to the annotation information of the corresponding platform. The DEGs between disease (AD or MDD) groups and normal groups were identified and screened as implemented in the R software (Version 3.6.0) limma package with a threshold value of p < 0.05. DEGs with p < 0.05, and |log2 fold change (FC)| > 0.1 were then presented visually using volcano plots. Venn diagrams were used to further obtain the overlapping genes from the AD and MDD microarray datasets.
Construction of the Protein-Protein Interaction Network
To explore all the possible interactions among genes, the PPI network of YZQX-related targets and AD-MDD overlapped targets were established using the Cytoscape plugin BisoGenet (Martin et al., 2010), which integrates PPI data from the following six databases: the Biological General Repository for Interaction Datasets (BioGRID), the Biomolecular Interaction Network Database (BIND), the Database of Interacting Proteins (DIP), the Molecular INTeraction Database (MINT), the Human Protein Reference Database (HPRD), and the IntAct Molecular Interaction Database (IntAct).
Merging and Analysis of Protein-Protein Interaction Networks
Building on the previous process, two PPI networks were intersected into a merged network for further analysis using Cytoscape 3.7.2 software. Next, the CytoNCA (Tang et al., 2015) plugin of Cytoscape was used to calculate and evaluate the topological significance of each node in the network based on these parameters of degree centrality (DC), betweenness centrality (BC), closeness centrality (CC), eigenvector centrality (EC), local average connectivity-based method (LAC), and network centrality (NC). A previous study (Tang et al., 2015) specified in detail the definitions and computing methods of these parameters; in brief, a higher value represents greater importance of the node in the merged network. We calculated the degree of centrality in a merged network. It is worth mentioning that this gene is considered “a big hub” when the degree centrality of one node is more than twice the median degree centrality in a merged network. Subsequently, a sub-network was extracted based on two times the median degree centrality and used to perform the next screening. The median values of the other five parameters served as threshold values to further identify the hub genes and core network. Finally, after two screenings, the core network of YZQX treating comorbidity with AD and MDD was obtained.
In addition, we also further analyzed the attributes of network screened by CytoNCA using MCODE plugins in Cytoscape software.
Enrichment Analysis of the Core Network
ClusterProfiler (Yu et al., 2012), a new ontology-based package of R software version 3.6.0, which assigned the GO and Kyoto Encyclopaedia of Genes and Genomes (KEGG) database, was used for enrichment analysis. Of note, the parameter was set to a p-value cutoff <0.05, which was deemed to be significant. The GO term contained three categories: biological process (BP), cellular component (CC), and molecular function (MF). The results of GO, sorted from small to large according to the p-value as a parameter, and the top 10 terms of each category were selected using a bubble plot for visualization. For the top five terms, a sub-network was then established in Cytoscape software version 3.7.2 to visualize the relationships between genes and GO terms. Furthermore, based on the genes of the core network of YZQX in AD and MDD treatment, KEGG pathway analysis was performed, and a bubble plot was plotted.
Molecular Docking Verification
To explore the possible docking modes and binding affinities between macromolecules and small molecules (ligands) in-depth, we performed molecular docking based on molecular modeling techniques using AutoDock software (Yang et al., 2016). We subsequently identified the direct-acting targets in the core network and their corresponding bioactive ingredients for molecular docking. The X-ray crystal structures of key targets were obtained from the RCSB Protein Data Bank (PDB, https://www.rcsb.org/) and saved in PDB format. The MOL2 formats of the bioactive ingredients were downloaded from the ZINC database (http://zinc.docking.org/). Before molecular docking, we used AutoDock software (version 4.2.6) to remove the ligand, hydrogenate, calculate the charge, add protein type for the macromolecules, and saved it in PDBQT format. Subsequently, we ran the AutoDock to perform molecular docking between macromolecules and ligands based on the default parameters. Blinding energy of less than “−5” represented a better binding interaction between macromolecules and small molecules (Gaillard, 2018). Finally, the PyMOL software (version 2.4.1) was used to visualize the docking results.
Results
Ingredients and Their Putative Targets of Yizhiqinxin and Ingredient-Target Network
A total of 43 ingredients in YZQX were screened from the TCMSP database with the criteria of OB ≥ 30% and DL ≥ 0.18. These were defined as candidate bioactive ingredients, of which seven were from Chuanxiong, 14 from Huanglian, and 22 from Renshen. The detailed characteristics, including molecular ID, molecular name, structure, OB, and DL, of the selected bioactive ingredients are presented in Table 1. We retrieved the DrugBank database using candidate bioactive ingredients and identified 504 ingredient-related targets. From each of the Chinese herbs in YZQX, 39, 251, and 214 potential targets were obtained, respectively (Supplementary Table S1). After removing the reduplicated targets, the total number of putative targets associated with bioactive ingredients was adjusted to 193. In addition, these targets were annotated as gene symbols in the UniProt database.
Construction of Ingredient-Target Network in Yizhiqinxin
Subsequently, using Cytoscape software, we established a network indicating the relationship among Chinese herbs, ingredients, and putative targets, as shown in Figure 2. This ingredient-target network contained 231 nodes and 541 edges. Quercetin was the most prolific, with 141 potential targets, followed by kaempferol (56), and beta-sitosterol (28).
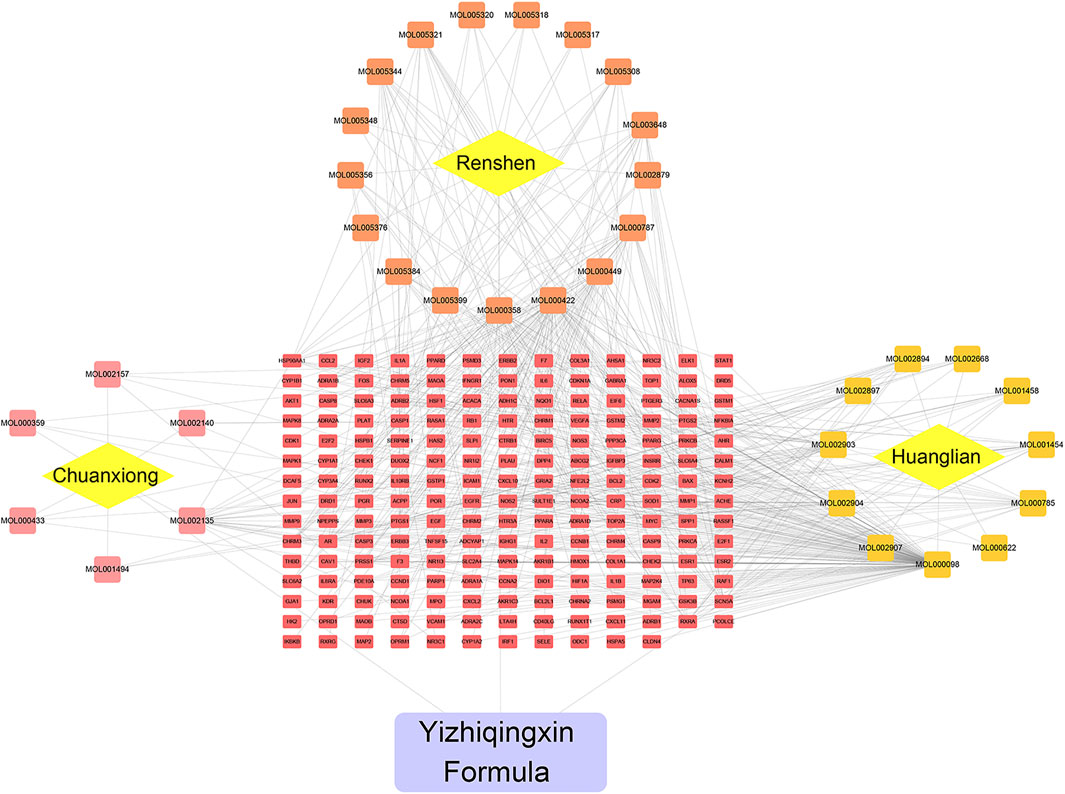
FIGURE 2. Herb-Ingredient-target network of YZQX. Yellow rhombus represents herb, the square represents ingredients, the red rectangle represents targets, the purple rectangle represents the YZQX formula.
Identification of Alzheimer’s Disease-Related and Major Depression Disorder-Related Differentially Expressed Genes
We downloaded the AD expression datasets and selected DEGs by calculating the difference in gene expression between 134 control samples and 139 AD samples. Ultimately, 4,873 DEGs, including 2,104 downregulated and 2,769 upregulated genes, were identified from the GSE63061 dataset using the limma package in R software. Additionally, based on the cutoff criterion of p < 0.05, a total of 1,707 DEGs, containing 889 downregulated and 818 upregulated genes, were extracted and analyzed from the microarray data GSE98793. The DEGs with p < 0.05, and |log2 FC| > 0.1 in AD or MDD were visualized in a volcano plot (Figures 3A,B). And the detailed information of AD- and MDD-related DEGs was presented in Supplementary Table S2. Moreover, the overlapping genes of AD and MDD were 378, as shown in Figure 3C.
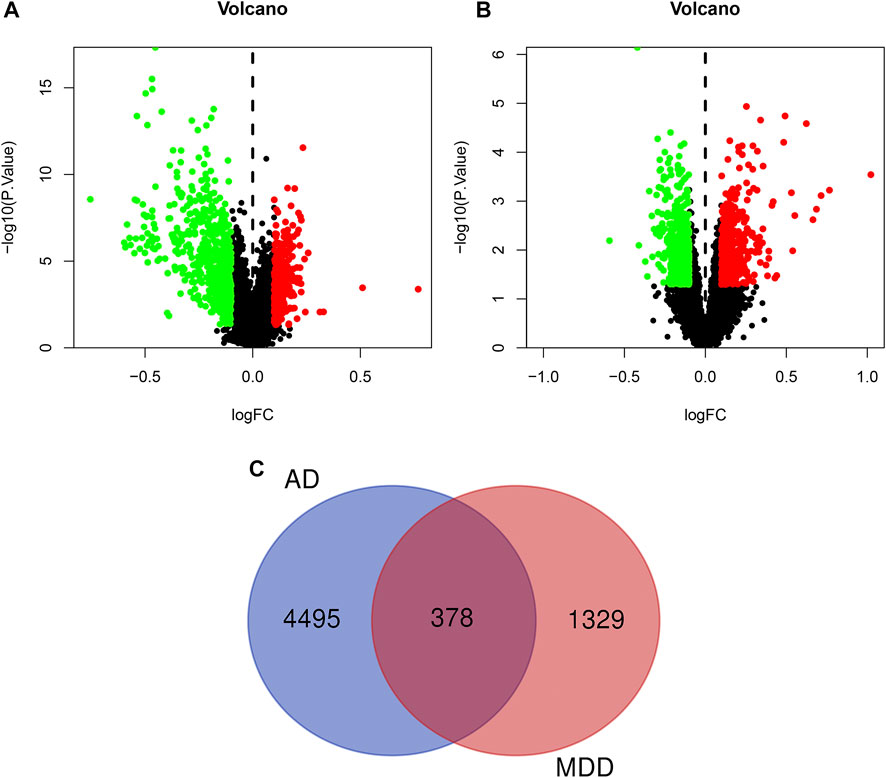
FIGURE 3. Volcano plot of differentially expressed genes in AD (A) and depression (B). The red dots represent significantly up-regulated genes, the green dots represent significantly down-regulated genes. Panel (C) refers to the overlapped genes of AD and depression.
Merging and Analysis of Protein-Protein Interaction Networks
In the present study, we used the BisoGenet plugin based on the six PPI databases to generate PPI networks of YZQX putative targets and comorbidity with AD- and MDD-related targets (Figure 4). As presented in Figure 4A, the PPI network of AD with depression-related targets consisted of 162,057 edges and 6,435 nodes. The PPI network of putative targets associated with YZQX contained 154,133 edges and 6,322 nodes (Figure 4B).
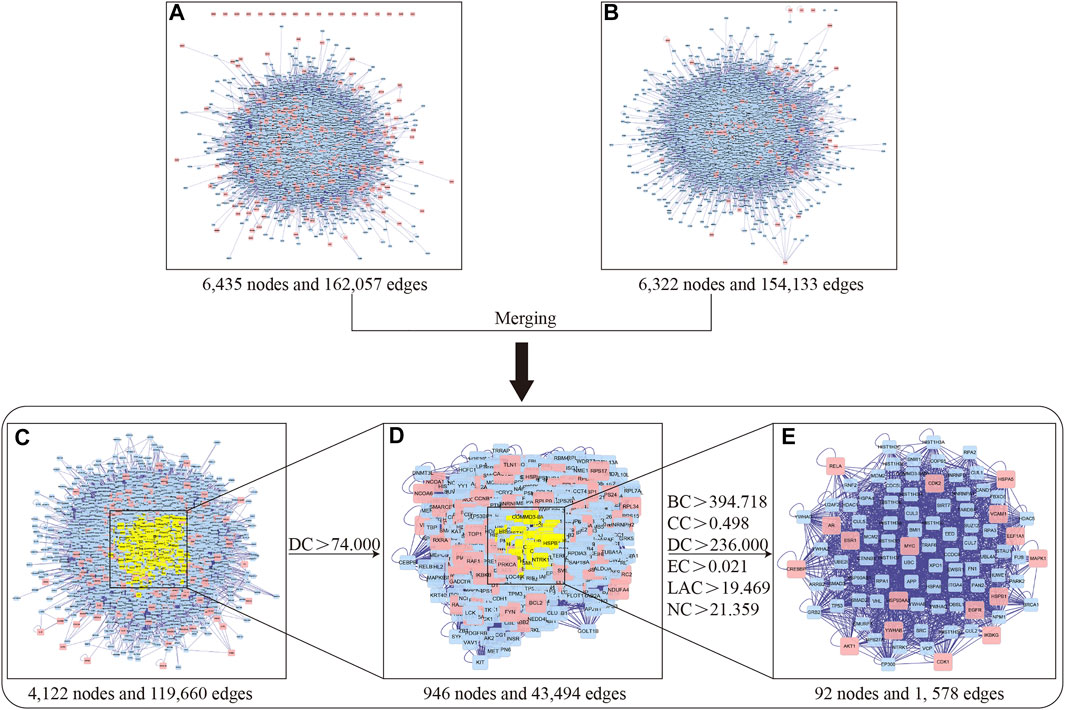
FIGURE 4. Identification of core network and targets of YZQX against AD with depression. (A) AD with depression-related targets PPI network. (B) YZQX putative targets PPI network. (C) The interactive PPI network of YZQX putative targets and AD with depression-related targets. (D) PPI network of significant targets extracted from Panel (C). (E) PPI network of candidate YZQX targets for AD with depression treatment extracted from Panel (D). Notes: AD, Alzheimer’s disease; DC, degree centrality; BC, betweenness centrality; CC, closeness centrality; EC, eigenvector centrality; LAC, local average connectivity-based method; NC, network centrality.
To explore the pharmacological mechanisms of YZQX in treating AD and MDD comorbidities, we utilized the merge network function supplied by Cytoscape software to generate a new merged network, with 119,660 edges and 4,122 nodes, consisting of overlapping genes from the two networks mentioned above (Figure 4C). After DC calculation, 946 nodes and 43,494 edges with a DC of more than two-fold the median were selected for integration into the subnetwork (Figure 4D). Next, these genes were further screened based on topological property analysis of DC, BC, CC, EC, LAC, and NC using the CytoNCA plugin in the Cytoscape software. The median values showed BC values of 394.718, CC of 0.498, EC of 0.021, NC of 21.359, LAC of 19.469, and the two-fold median DC of 236 (Figure 4E), and finally, a core network including 92 targets together with 1,578 edges was identified for YZQX against AD and MDD comorbidities. The detailed topology parameter of the core network involving 92 targets is presented in the Supplementary Table S3.
According to the ranking of MCODE score, the largest cluster has 25 nodes and 215 edges, with a score of 17.917 points; The smallest module has three nodes and three edges, with a score of three points. And the clusters are presented in Supplementary Figure S1.
Enrichment Analysis of Core Targets
To reveal the biological mechanisms of the 92 crucial targets, the cluster profile package in R software was used to perform GO and KEGG enrichment analysis, yielding a total of 1,212 BPs, 95 CCs, 119 MFs, and 117 KEGG pathways (p < 0.05) (Supplementary Tables S4, S5). As indicated in Figure 5, the highly enriched GO terms in BP were involved in DNA-binding transcription factor activity regulation, DNA metabolic process regulation, protein deubiquitination, protein modification by small protein removal, and the DNA biosynthetic process. The main CCs of the 92 core targets were related to nuclear chromatin, focal adhesion, cell-substrate adherens junction, cell-substrate junction, and ubiquitin ligase complex. MFs focused on ubiquitin-protein ligase binding, ubiquitin-like protein ligase binding, phosphatase binding, ubiquitin-like protein transferase activity, and RNA polymerase II transcription factor binding. In addition, based on the KEGG pathway data analysis, we found that the PI3K-Akt signaling pathway, ubiquitin-mediated proteolysis, estrogen signaling pathway, MAPK signaling pathway, and TGF-beta signaling pathway concurrently occupied the process of YZQX treatment of comorbidity with AD and MDD (Figure 5E).
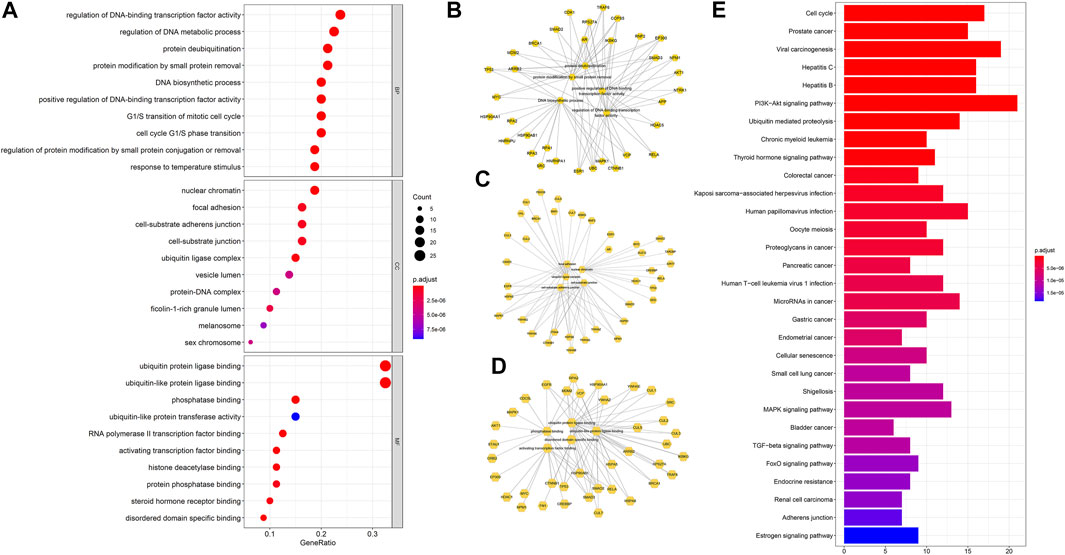
FIGURE 5. The top 10 terms of GO analysis about targets involved in the core network (A). (B) Sub-network showing the top five BP terms and related genes; (C) Sub-network showing the top 5 CC terms and related genes; (D) Sub-network showing the top five MF terms and related genes. (E)The top 30 KEGG pathway enrichment of core targets of YZQX against AD with depression. Pathways that had significant changes of p. adjust <0.05 were identified. The dot size represents a number of genes and color represents the p. adjust value.
Validation of Molecular Docking
The PPI network indicated that HSP90AA1, ESR1, AKT1, VCAM1, EGFR, CDK1, MAPK1, CDK2, MYC, HSPB1, and HSPA5 are pivotal targets in the core network mentioned above, and these nodes were also overlapping genes of AD and MDD comorbidity. In accordance with key targets, we worked backward to their corresponding bioactive ingredients serving as ligands, thus performing validation of molecular docking. In total, 2 ingredients were docked with AKT1, 2 with CDK1, 3 with CDK2, 6 with ESR1, 11 with HSP90AA1, 1 with EGFR, 1 with HSPA5, 1 with HSPB1, 1 with MAPK1, 1 with MYC, and 1 with VCAM1. The ligand-receptor binding conformation had the lowest binding energy, indicating that this conformation is the most stable, and thus the possibility of interaction is the greatest. The top three docking combinations for the target macromolecules and corresponding ingredients included Fumarine and HSP90AA1, Worenine and ESR1, berberine and HSP90AA1, with binding energies of −6.83, −6.6, and −6.26 kcal/mol, respectively. The other specific docking scores of the ingredients with targets are shown in Supplementary Table S6. If the docking score was less than −6 kcal/mol and ranked as the top 4, PyMOL was used to display the three-dimensional view of the docking mode of the ingredients and the target (Figure 6).
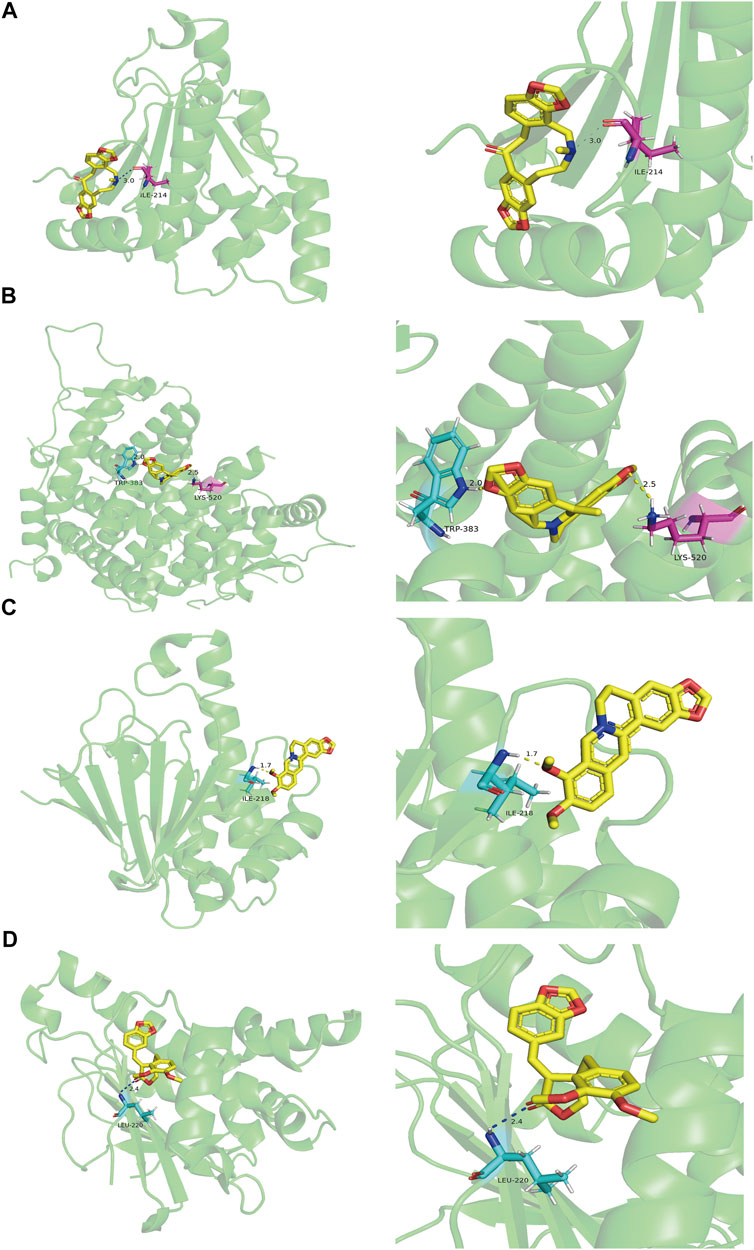
FIGURE 6. The molecular docking results of key targets and their corresponding ingredients. (A) Fumarine and HSP90AA1 (B) Worenine and ESR1 (C) berberine and HSP90AA1 (D) suchilactone and HSP90AA1.
Discussion
Depression is recognized as the most common comorbidity of AD and affects the treatment of AD patients (Chi et al., 2014; Romano et al., 2014). Hence, the development of an effective treatment for patients with AD and MDD comorbidity is extremely important. As a traditional Chinese medicine, the formula or its main ingredients in YZQX have been widely used to treat comorbid AD and MDD with remarkable clinical effects (Lee et al., 2012; Ong et al., 2015; Yang et al., 2017; Yang et al., 2019; Hou et al., 2020). In this present study, we coupled network pharmacology with GEO database analysis to explore the common mechanisms of YZQX in treating AD and MDD comorbidity. By establishing and screening a PPI network of the overlapping targets of YZQX and AD as well as MDD, 92 targets were identified and considered as core genes. Of these, HSP90AA1, ESR1, AKT1, VCAM1, EGFR, CDK1, MAPK1, CDK2, MYC, HSPB1, and HSPA5 acted directly on these diseases. Further, KEGG pathway analysis revealed that these core targets were mainly related to the PI3K-Akt signalling pathway, ubiquitin-mediated proteolysis, estrogen signalling pathway, MAPK signalling pathway, TGF-beta signalling pathway, etc. The abovementioned evidence suggests the clinically promising potential of YZQX in the treatment of AD comorbidity with depression.
In this study, we identified 43 ingredients in YZQX, and molecular docking was performed to compare these crucial targets with the corresponding ingredients. Quercetin and kaempferol could bind well to multiple crucial targets, which served as the main bioactive ingredients. Quercetin is a predominant flavonoid that has multiple beneficial properties, including antioxidative and anti-inflammatory effects (Brüll et al., 2015). Moreover, data from previous studies revealed its neuroprotective effects on AD (Rishitha and Muthuraman, 2018), which may be ascribed to its ability to cross the blood-brain barrier (BBB) and exert antioxidant and anti-inflammatory effects in the brain (Youdim et al., 2004). Likewise, quercetin also markedly promoted neurogenesis by increasing the level of doublecortin and subsequently alleviating depressive-like behavior (Mehta et al., 2017). Additionally, in previous network pharmacology studies, quercetin was also found to be an ingredient that contributed significantly in the treatment of comorbidity with AD and MDD, participating in polypharmacological and synergistic mechanisms (Qi et al., 2020; Yuan et al., 2020). Kaempferol is a natural flavonoid, which is found in numerous fruits and vegetables and can trigger multiple biological mechanisms, including anti-inflammation, antioxidant activity, neuroprotective effect, and antidepressant effects (Kim et al., 2007; Kim et al., 2008; Silva Dos Santos et al., 2020). An in vitro study on rats indicated that kaempferol improved the cognitive function in AD rat models, thereby exerting its neuroprotective effect (Zarei et al., 2019). Likewise, Gao et al. have shown that kaempferol also plays a role in antidepressant capacities mainly mediated through its anti-inflammatory and antioxidant effect which work by enhancing the AKT/β-catenin signaling pathway (Gao et al., 2019). Of note, substantive evidence is accumulating to suggest that treatments with anti-inflammatory and anti-depressant agents may also reduce or prevent dementia in patients with MDD (Hayley, 2021).
Moreover, in this study, PPI networks between the ingredient-target of YZQX and comorbidity with AD and MDD were established using Cytoscape, followed by merging and topological parameters. Eventually, 92 targets were identified and enrichment analysis was performed to further clarify the underlying mechanisms of YZQX treatment in patients with comorbid AD and MDD. KEGG analysis indicated that 92 targets were mainly related to the following pathways: PI3K-Akt signalling pathway, ubiquitin-mediated proteolysis, estrogen signalling pathway, MAPK signalling pathway, TGF-beta signalling pathway, etc. Converging reports on the PI3K-Akt signaling pathway have shown that it plays a crucial role in multiple processes of cell reproduction, differentiation, and apoptosis. Further enhancing PI3K/Akt function may prevent the development of AD by alleviating the downstream effects of Aβ (Curtis and Bandyopadhyay, 2021). Moreover, when the PI3K/Akt signaling axis in neurons is activated, it could reduce Tau hyperphosphorylation by suppressing GSK-3β activity (Koh et al., 2008; O’Neill et al., 2012; O’Neill, 2013). More importantly, this was also associated with neuroplasticity and could exert an anti-depressive effect by strengthening synapse formation and axon dendrite extension (Singh et al., 2017; Ali et al., 2018). Relevant research has also found that several neurotrophins, particularly brain-derived neurotrophic factor (BDNF), play a key role in the pathogenesis of MDD (Wiener et al., 2015; Castrén and Kojima, 2017). Additionally, BDNF activates the PI3K/Akt pathway, thereby increasing the length and complexity of dendrites and regulating neuronal survival (Jaworski et al., 2005; Kumar et al., 2005). Growing evidence now indicates that the MAPK signaling pathway plays a crucial role in a variety of biological processes and is capable of regulating inflammation and the stress response (Venigalla and Turner, 2012). It is worth mentioning that this pathway is closely associated with not only neuron apoptosis, but also β-amyloid deposition and tau hyperphosphorylation in AD (Song et al., 2014; Kheiri et al., 2018). In addition, the differential expression of miRNA profiles in rat models of depression also concentrates on the MAPK pathway and thereby affects the pathogenesis of depression (Fang et al., 2020).
Some hub targets predicted by molecular docking in this study have also been reported in previous studies (Pirskanen et al., 2005; Sulistio and Heese, 2016; Lackie et al., 2017). ESR1, located on chromosome 6q25, is an essential mediator of the hormonal response in estrogen-sensitive tissues. During the course of AD, estrogen could help in the following activities: promoting neuronal cell survival, alleviating neuronal damage, protecting the neuronal cell from neurotoxins, and strengthening synaptic transmission and neurogenesis (Pirskanen et al., 2005). When binding with estrogen, ESR1 can regulate gene expression and its corresponding function via the interactions between regulatory regions and target genes (Xu et al., 2013). A study of the relevant literature revealed that ESR1 serves as a key factor of depression, particularly in women (Ancelin et al., 2007; Osterlund, 2010; Ryan et al., 2012). Interestingly, ESR1 can control the activity of serotonin-activated neurons by regulating the quantity and function of serotonin receptors and controlling neurotransmitters (Summer and Fink, 1995; Andrade et al., 2005). HSP90AA1, a member of the heat shock family, is highly conserved in eukaryotes. Of particular interest was the finding that HSPs are also involved in regulating the stabilization and function of p-tau in AD (Sulistio and Heese, 2016; Lackie et al., 2017). Apart from this, they also play a key role in protein folding and proteosomal degradation (Lackie et al., 2017).
In the present study, the underlying mechanisms and molecular targets of YZQX for AD with depression were illustrated using integrated network pharmacology and molecular docking approach. YZQX may exert its effects via action on the PI3K-Akt signalling pathway, ubiquitin-mediated proteolysis, Estrogen signalling pathway, MAPK signalling pathway, and TGF-beta signalling pathway. This study further identified HSP90AA1, ESR1, AKT1, VCAM1, EGFR, CDK1, MAPK1, CDK2, MYC, HSPB1, and HSPA5 as the key targets of YZQX in the treatment of AD with comorbid depression. This study further confirmed that YZQX is a promising therapeutic method for the development of safe and effective multitarget for AD with depression.
Data Availability Statement
The original contributions presented in the study are included in the article/Supplementary Material, further inquiries can be directed to the corresponding authors.
Author Contributions
HL, TZ, and NL: Conceptualization, methodology, software. TZ and NL: Data curation, writing—original draft preparation. WW: Visualization, investigation. SC: Supervision, validation. HL: Writing—reviewing and editing.
Funding
This work was supported by the National Natural Science Foundation of China under Grant (81873350), the Beijing Natural Science Foundation under Grant (7202174), and the Innovation Team and Talents Cultivation Program of the National Administration of Traditional Chinese Medicine under Grant (ZYYCXTD-C-202007). In addition to the fund mentioned, our manuscript was also supported by the China Academy of Chinese Medical Sciences Innovation Fund (NO. CI2021A01401, NO. CI2021A01405) in the revision stage of the manuscript.
Conflict of Interest
The authors declare that the research was conducted in the absence of any commercial or financial relationships that could be construed as a potential conflict of interest.
The reviewer XZ declared a shared parent affiliation with the authors to the handling editor at the time of review.
Publisher’s Note
All claims expressed in this article are solely those of the authors and do not necessarily represent those of their affiliated organizations, or those of the publisher, the editors and the reviewers. Any product that may be evaluated in this article, or claim that may be made by its manufacturer, is not guaranteed or endorsed by the publisher.
Supplementary Material
The Supplementary Material for this article can be found online at: https://www.frontiersin.org/articles/10.3389/fphar.2022.853375/full#supplementary-material
References
Alexiou, A., Kamal, M. A., and Ashraf, G. M. (2019). Editorial: The Alzheimer's Disease Challenge. Front. Neurosci. 13, 768. doi:10.3389/fnins.2019.00768
Ali, T., Kim, T., Rehman, S. U., Khan, M. S., Amin, F. U., Khan, M., et al. (2018). Natural Dietary Supplementation of Anthocyanins via PI3K/Akt/Nrf2/HO-1 Pathways Mitigate Oxidative Stress, Neurodegeneration, and Memory Impairment in a Mouse Model of Alzheimer's Disease. Mol. Neurobiol. 55 (7), 6076–6093. doi:10.1007/s12035-017-0798-6
American Psychiatric Association (2013). Diagnostic and Statistical Manual of Mental Disorders. 5th Edn. Washington, DC: American Psychiatric Association.
Ancelin, M. L., Scali, J., and Ritchie, K. (2007). Hormonal Therapy and Depression: Are We Overlooking an Important Therapeutic Alternative? J. Psychosom Res. 62 (4), 473–485. doi:10.1016/j.jpsychores.2006.12.019
Andrade, T. G., Nakamuta, J. S., Avanzi, V., and Graeff, F. G. (2005). Anxiolytic Effect of Estradiol in the Median Raphe Nucleus Mediated by 5-HT1A Receptors. Behav. Brain Res. 163 (1), 18–25. doi:10.1016/j.bbr.2005.04.015
Barton, H. A., Pastoor, T. P., Baetcke, K., Chambers, J. E., Diliberto, J., Doerrer, N. G., et al. (2006). The Acquisition and Application of Absorption, Distribution, Metabolism, and Excretion (ADME) Data in Agricultural Chemical Safety Assessments. Crit. Rev. Toxicol. 36 (1), 9–35. doi:10.1080/10408440500534362
Bennett, S., and Thomas, A. J. (2014). Depression and Dementia: Cause, Consequence or Coincidence? Maturitas 79 (2), 184–190. doi:10.1016/j.maturitas.2014.05.009
Berger, S. I., and Iyengar, R. (2009). Network Analyses in Systems Pharmacology. Bioinformatics 25 (19), 2466–2472. doi:10.1093/bioinformatics/btp465
Brüll, V., Burak, C., Stoffel-Wagner, B., Wolffram, S., Nickenig, G., Müller, C., et al. (2015). Effects of a Quercetin-Rich Onion Skin Extract on 24 H Ambulatory Blood Pressure and Endothelial Function in Overweight-To-Obese Patients with (Pre-)hypertension: a Randomised Double-Blinded Placebo-Controlled Cross-Over Trial. Br. J. Nutr. 114 (8), 1263–1277. doi:10.1017/s0007114515002950
Castrén, E., and Kojima, M. (2017). Brain-derived Neurotrophic Factor in Mood Disorders and Antidepressant Treatments. Neurobiol. Dis. 97 (Pt B), 119–126. doi:10.1016/j.nbd.2016.07.010
Chao, J., Dai, Y., Verpoorte, R., Lam, W., Cheng, Y. C., Pao, L. H., et al. (2017). Major Achievements of Evidence-Based Traditional Chinese Medicine in Treating Major Diseases. Biochem. Pharmacol. 139, 94–104. doi:10.1016/j.bcp.2017.06.123
Chi, S., Yu, J. T., Tan, M. S., and Tan, L. (2014). Depression in Alzheimer's Disease: Epidemiology, Mechanisms, and Management. J. Alzheimers Dis. 42 (3), 739–755. doi:10.3233/jad-140324
Curtis, D., and Bandyopadhyay, S. (2021). Mini-review: Role of the PI3K/Akt Pathway and Tyrosine Phosphatases in Alzheimer's Disease Susceptibility. Ann. Hum. Genet. 85 (1), 1–6. doi:10.1111/ahg.12410
Fang, K., Xu, J. X., Chen, X. X., Gao, X. R., Huang, L. L., Du, A. Q., et al. (2020). Differential Serum Exosome microRNA Profile in a Stress-Induced Depression Rat Model. J. Affect Disord. 274, 144–158. doi:10.1016/j.jad.2020.05.017
Fiorini, R., Luzzi, S., and Vignini, A. (2020). Perspectives on Mild Cognitive Impairment as a Precursor of Alzheimer's Disease. Neural Regen. Res. 15 (11), 2039–2040. doi:10.4103/1673-5374.282256
Fransquet, P. D., Lacaze, P., Saffery, R., McNeil, J., Woods, R., and Ryan, J. (2018). Blood DNA Methylation as a Potential Biomarker of Dementia: A Systematic Review. Alzheimers Dement 14 (1), 81–103. doi:10.1016/j.jalz.2017.10.002
Gaillard, T. (2018). Evaluation of AutoDock and AutoDock Vina on the CASF-2013 Benchmark. J. Chem. Inf. Model. 58 (8), 1697–1706. doi:10.1021/acs.jcim.8b00312
Gao, W., Wang, W., Peng, Y., Deng, Z., Hayley, S., Hakim, A. M., et al. (20192021). Antidepressive Effects of Kaempferol Mediated by Reduction of Oxidative Stress, Proinflammatory Cytokines and Up-Regulation of AKT/β-catenin cascadeDepression, Dementia and Immune Dysregulation. Metab. Brain Disbrain 34144 (23), 485746–494760. doi:10.1007/s11011-019-0389-510.1093/brain/awaa405
Hayley (2021). 2021 Alzheimer's Disease Facts and Figures. Alzheimers Dement 17 (3), 327–406. doi:10.1002/alz.12328
Hou, W., Wang, Y., Zheng, P., and Cui, R. (2020). Effects of Ginseng on Neurological Disorders. Front Cel Neurosci 14, 55. doi:10.3389/fncel.2020.00055
Jaworski, J., Spangler, S., Seeburg, D. P., Hoogenraad, C. C., and Sheng, M. (2005). Control of Dendritic Arborization by the Phosphoinositide-3'-Kinase-Akt-Mammalian Target of Rapamycin Pathway. J. Neurosci. 25 (49), 11300–11312. doi:10.1523/jneurosci.2270-05.2005
Kheiri, G., Dolatshahi, M., Rahmani, F., and Rezaei, N. (2018). Role of p38/MAPKs in Alzheimer's Disease: Implications for Amyloid Beta Toxicity Targeted Therapy. Rev. Neurosci. 30 (1), 9–30. doi:10.1515/revneuro-2018-0008
Kim, H. K., Park, H. R., Lee, J. S., Chung, T. S., Chung, H. Y., and Chung, J. (2007). Down-regulation of iNOS and TNF-Alpha Expression by Kaempferol via NF-kappaB Inactivation in Aged Rat Gingival Tissues. Biogerontology 8 (4), 399–408. doi:10.1007/s10522-007-9083-9
Kim, S. K., Kim, H. J., Choi, S. E., Park, K. H., Choi, H. K., and Lee, M. W. (2008). Anti-oxidative and Inhibitory Activities on Nitric Oxide (NO) and Prostaglandin E2 (COX-2) Production of Flavonoids from Seeds of Prunus Tomentosa Thunberg. Arch. Pharm. Res. 31 (4), 424–428. doi:10.1007/s12272-001-1174-9
Kitchen, D. B., Decornez, H., Furr, J. R., and Bajorath, J. (2004). Docking and Scoring in Virtual Screening for Drug Discovery: Methods and Applications. Nat. Rev. Drug Discov. 3 (11), 935–949. doi:10.1038/nrd1549
Koh, S. H., Noh, M. Y., and Kim, S. H. (2008). Amyloid-beta-induced Neurotoxicity Is Reduced by Inhibition of Glycogen Synthase Kinase-3. Brain Res. 1188, 254–262. doi:10.1016/j.brainres.2007.10.064
Kumar, V., Zhang, M. X., Swank, M. W., Kunz, J., and Wu, G. Y. (2005). Regulation of Dendritic Morphogenesis by Ras-Pi3k-Akt-mTOR and Ras-MAPK Signaling Pathways. J. Neurosci. 25 (49), 11288–11299. doi:10.1523/jneurosci.2284-05.2005
Lackie, R. E., Maciejewski, A., Ostapchenko, V. G., Marques-Lopes, J., Choy, W. Y., Duennwald, M. L., et al. (2017). The Hsp70/Hsp90 Chaperone Machinery in Neurodegenerative Diseases. Front. Neurosci. 11, 254. doi:10.3389/fnins.2017.00254
Law, V., Knox, C., Djoumbou, Y., Jewison, T., Guo, A. C., Liu, Y., et al. (2014). DrugBank 4.0: Shedding New Light on Drug Metabolism. Nucleic Acids Res. 42, D1091–D1097. doi:10.1093/nar/gkt1068
Lee, B., Sur, B., Yeom, M., Shim, I., Lee, H., and Hahm, D. H. (2012). Effect of Berberine on Depression- and Anxiety-like Behaviors and Activation of the Noradrenergic System Induced by Development of Morphine Dependence in Rats. Korean J. Physiol. Pharmacol. 16 (6), 379–386. doi:10.4196/kjpp.2012.16.6.379
Li, M., Reisman, J., Morris-Eppolito, B., Qian, S. X., Kazis, L. E., Wolozin, B., et al. (2020). Beneficial Association of Angiotensin-Converting Enzyme Inhibitors and Statins on the Occurrence of Possible Alzheimer's Disease after Traumatic Brain Injury. Alzheimers Res. Ther. 12 (1), 33. doi:10.1186/s13195-020-00589-3
Martin, A., Ochagavia, M. E., Rabasa, L. C., Miranda, J., Fernandez-de-Cossio, J., and Bringas, R. (2010). BisoGenet: a New Tool for Gene Network Building, Visualization and Analysis. BMC Bioinformatics 11, 91. doi:10.1186/1471-2105-11-91
Mehta, V., Singh, T. R., and Udayabanu, M. (2017). Quercetin Ameliorates Chronic Unpredicted Stress-Induced Behavioral Dysfunction in Male Swiss Albino Mice by Modulating Hippocampal Insulin Signaling Pathway. Physiol. Behav. 182, 10–16. doi:10.1016/j.physbeh.2017.09.019
O' Neill, C. (2013). PI3-kinase/Akt/mTOR Signaling: Impaired On/off Switches in Aging, Cognitive Decline and Alzheimer's Disease. Exp. Gerontol. 48 (7), 647–653. doi:10.1016/j.exger.2013.02.025
O'Neill, C., Kiely, A. P., Coakley, M. F., Manning, S., and Long-Smith, C. M. (2012). Insulin and IGF-1 Signalling: Longevity, Protein Homoeostasis and Alzheimer's Disease. Biochem. Soc. Trans. 40 (4), 721–727. doi:10.1042/bst20120080
Ong, W. Y., Farooqui, T., Koh, H. L., Farooqui, A. A., and Ling, E. A. (2015). Protective Effects of Ginseng on Neurological Disorders. Front. Aging Neurosci. 7, 129. doi:10.3389/fnagi.2015.00129
Osterlund, M. K. (2010). Underlying Mechanisms Mediating the Antidepressant Effects of Estrogens. Biochim. Biophys. Acta 1800 (10), 1136–1144. doi:10.1016/j.bbagen.2009.11.001
Pirskanen, M., Hiltunen, M., Mannermaa, A., Helisalmi, S., Lehtovirta, M., Hänninen, T., et al. (2005). Estrogen Receptor Beta Gene Variants Are Associated with Increased Risk of Alzheimer's Disease in Women. Eur. J. Hum. Genet. 13 (9), 1000–1006. doi:10.1038/sj.ejhg.5201447
Qi, P., Li, J., Gao, S., Yuan, Y., Sun, Y., Liu, N., et al. (2020). Network Pharmacology-Based and Experimental Identification of the Effects of Quercetin on Alzheimer's Disease. Front. Aging Neurosci. 12, 589588. doi:10.3389/fnagi.2020.589588
Rishitha, N., and Muthuraman, A. (2018). Therapeutic Evaluation of Solid Lipid Nanoparticle of Quercetin in Pentylenetetrazole Induced Cognitive Impairment of Zebrafish. Life Sci. 199, 80–87. doi:10.1016/j.lfs.2018.03.010
Romano, A., Pace, L., Tempesta, B., Lavecchia, A. M., Macheda, T., Bedse, G., et al. (2014). Depressive-like Behavior Is Paired to Monoaminergic Alteration in a Murine Model of Alzheimer's Disease. Int. J. Neuropsychopharmacol. 18 (4). doi:10.1093/ijnp/pyu020
Ru, J., Li, P., Wang, J., Zhou, W., Li, B., Huang, C., et al. (2014). TCMSP: a Database of Systems Pharmacology for Drug Discovery from Herbal Medicines. J. Cheminform 6, 13. doi:10.1186/1758-2946-6-13
Ryan, J., Scali, J., Carrière, I., Peres, K., Rouaud, O., Scarabin, P. Y., et al. (2012). Estrogen Receptor Alpha Gene Variants and Major Depressive Episodes. J. Affect Disord. 136 (3), 1222–1226. doi:10.1016/j.jad.2011.10.010
Silva Dos Santos, J., Gonçalves Cirino, J. P., de Oliveira Carvalho, P., and Ortega, M. M. (2020). The Pharmacological Action of Kaempferol in Central Nervous System Diseases: A Review. Front. Pharmacol. 11, 565700. doi:10.3389/fphar.2020.565700
Singh, A. K., Kashyap, M. P., Tripathi, V. K., Singh, S., Garg, G., and Rizvi, S. I. (2017). Neuroprotection through Rapamycin-Induced Activation of Autophagy and PI3K/Akt1/mTOR/CREB Signaling against Amyloid-β-Induced Oxidative Stress, Synaptic/Neurotransmission Dysfunction, and Neurodegeneration in Adult Rats. Mol. Neurobiol. 54 (8), 5815–5828. doi:10.1007/s12035-016-0129-3
Song, J., Park, K. A., Lee, W. T., and Lee, J. E. (2014). Apoptosis Signal Regulating Kinase 1 (ASK1): Potential as a Therapeutic Target for Alzheimer's Disease. Int. J. Mol. Sci. 15 (2), 2119–2129. doi:10.3390/ijms15022119
Sulistio, Y. A., and Heese, K. (2016). The Ubiquitin-Proteasome System and Molecular Chaperone Deregulation in Alzheimer's Disease. Mol. Neurobiol. 53 (2), 905–931. doi:10.1007/s12035-014-9063-4
Summer, B. E., and Fink, G. (1995). Estrogen Increases the Density of 5-hydroxytryptamine(2A) Receptors in Cerebral Cortex and Nucleus Accumbens in the Female Rat. J. Steroid Biochem. Mol. Biol. 54 (1-2), 15–20. doi:10.1016/0960-0760(95)00075-b
Tang, Y., Li, M., Wang, J., Pan, Y., and Wu, F. X. (2015). CytoNCA: a Cytoscape Plugin for Centrality Analysis and Evaluation of Protein Interaction Networks. Biosystems 127, 67–72. doi:10.1016/j.biosystems.2014.11.005
UniProt (2017). UniProt: the Universal Protein Knowledgebase. Nucleic Acids Res. 45 (D1), D158–d169. doi:10.1093/nar/gkw1099
Uzun, S., Kozumplik, O., and Folnegović-Smalc, V. (2011). Alzheimer's Dementia: Current Data Review. Coll. Antropol 35 (4), 1333–1337.
Venigalla, R. K., and Turner, M. (2012). RNA-binding Proteins as a point of Convergence of the PI3K and P38 MAPK Pathways. Front. Immunol. 3, 398. doi:10.3389/fimmu.2012.00398
Wiener, C. D., de Mello Ferreira, S., Pedrotti Moreira, F., Bittencourt, G., de Oliveira, J. F., Lopez Molina, M., et al. (2015). Serum Levels of Nerve Growth Factor (NGF) in Patients with Major Depression Disorder and Suicide Risk. J. Affect Disord. 184, 245–248. doi:10.1016/j.jad.2015.05.067
Xu, C. Y., Jiang, Z. N., Zhou, Y., Li, J. J., and Huang, L. M. (2013). Estrogen Receptor α Roles in Breast Cancer Chemoresistance. Asian Pac. J. Cancer Prev. 14 (7), 4049–4052. doi:10.7314/apjcp.2013.14.7.4049
Yang, B., Yang, Y. S., Yang, N., Li, G., and Zhu, H. L. (2016). Design, Biological Evaluation and 3D QSAR Studies of Novel Dioxin-Containing Pyrazoline Derivatives with Thiourea Skeleton as Selective HER-2 Inhibitors. Sci. Rep. 6, 27571. doi:10.1038/srep27571
Yang, Y., Jia, X., Feng, J., Wang, Z., Cao, Y., Liu, J., et al. (2017). Fuzheng Quxie Decoction Ameliorates Learning and Memory Impairment in SAMP8 Mice by Decreasing Tau Hyperphosphorylation. Evid. Based Complement. Alternat Med. 2017, 5934254. doi:10.1155/2017/5934254
Yang, Y., Wang, Z., Cao, Y., Liu, J., Li, P., Li, H., et al. (2019). Yizhiqingxin Formula Alleviates Cognitive Deficits and Enhances Autophagy via mTOR Signaling Pathway Modulation in Early Onset Alzheimer's Disease Mice. Front. Pharmacol. 10, 1041. doi:10.3389/fphar.2019.01041
Youdim, K. A., Shukitt-Hale, B., and Joseph, J. A. (2004). Flavonoids and the Brain: Interactions at the Blood-Brain Barrier and Their Physiological Effects on the central Nervous System. Free Radic. Biol. Med. 37 (11), 1683–1693. doi:10.1016/j.freeradbiomed.2004.08.002
Yu, G., Wang, L. G., Han, Y., and He, Q. Y. (2012). clusterProfiler: an R Package for Comparing Biological Themes Among Gene Clusters. Omics 16 (5), 284–287. doi:10.1089/omi.2011.0118
Yuan, N., Gong, L., Tang, K., He, L., Hao, W., Li, X., et al. (2020). An Integrated Pharmacology-Based Analysis for Antidepressant Mechanism of Chinese Herbal Formula Xiao-Yao-San. Front. Pharmacol. 11, 284. doi:10.3389/fphar.2020.00284
Keywords: Yizhiqingxin, Alzheimer’s disease, major depression disorder, network pharmacology, molecular docking
Citation: Zhang T, Wei W, Chang S, Liu N and Li H (2022) Integrated Network Pharmacology and Comprehensive Bioinformatics Identifying the Mechanisms and Molecular Targets of Yizhiqingxin Formula for Treatment of Comorbidity With Alzheimer’s Disease and Depression. Front. Pharmacol. 13:853375. doi: 10.3389/fphar.2022.853375
Received: 12 January 2022; Accepted: 04 April 2022;
Published: 25 April 2022.
Edited by:
Athanasios Alexiou, Novel Global Community Educational Foundation (NGCEF), AustraliaReviewed by:
Udhaya Kumar S., Vellore Institute of Technology, IndiaAnas Shamsi, Jamia Millia Islamia, India
Xiaomeng Zhang, China Academy of Chinese Medical Sciences, China
Copyright © 2022 Zhang, Wei, Chang, Liu and Li. This is an open-access article distributed under the terms of the Creative Commons Attribution License (CC BY). The use, distribution or reproduction in other forums is permitted, provided the original author(s) and the copyright owner(s) are credited and that the original publication in this journal is cited, in accordance with accepted academic practice. No use, distribution or reproduction is permitted which does not comply with these terms.
*Correspondence: Nanyang Liu, 1756487910@qq.com; Hao Li, xyhplihao1965@126.com