- 1Guang’anmen Hospital, China Academy of Chinese Medical Sciences, Beijing, China
- 2Dongzhimen Hospital, Beijing University of Chinese Medicine, Beijing, China
- 3Beijing First Hospital of Integrated Chinese and Western Medicine, Beijing, China
- 4Affiliated Hospital of Traditional Chinese Medicine, Southwest Medical University, Luzhou, China
Background: Treatment-resistant depression (TRD) may have different physiopathological neuromechanism in different age groups. This study used the amplitude of low frequency fluctuations (ALFF) to initially compare abnormalities in local functional brain activity in younger and older patients with TRD.
Materials and methods: A total of 21 older TRD patients, 19 younger TRD, 19 older healthy controls (HCs), and 19 younger HCs underwent resting-state functional MRI scans, and the images were analyzed using the ALFF and further analyzed for correlation between abnormal brain regions and clinical symptoms in TRD patients of different age groups.
Results: Compared with the older TRD, the younger TRD group had increased ALFF in the left middle frontal gyrus and decreased ALFF in the left caudate nucleus. Compared with the matched HC group, ALFF was increased in the right middle temporal gyrus and left pallidum in the older TRD group, whereas no significant differences were found in the younger TRD group. In addition, ALFF values in the left middle frontal gyrus in the younger TRD group and in the right middle temporal gyrus in the older TRD were both positively correlated with the 17-item Hamilton Rating Scale for Depression score.
Conclusion: Different neuropathological mechanisms may exist in TRD patients of different ages, especially in the left middle frontal gyrus and left caudate nucleus. This study is beneficial in providing potential key targets for the clinical management of TRD patients of different ages.
Introduction
Major depressive disorder (MDD) is a common clinical psychiatric disorder with depressed mood, decreased cognitive function and somatic disorders as the main clinical features (Lawson et al., 2017). Epidemiological surveys show that MDD is expected to be the number one disease burden globally by 2030 (Ho et al., 2018). In addition, MDD is the leading cause of disability worldwide, with approximately 800,000 deaths by suicide each year (Fabbri et al., 2021). However, despite numerous studies, 30–40% of MDD patients still do not respond significantly antidepressants (Bergfeld et al., 2018). This type of MDD that does not respond significantly to two adequate doses and courses of antidepressant medication can be called treatment-resistant depression (TRD) (Gaynes et al., 2020). TRD is a complex subtype of MDD with lower quality of life, higher costs and more severe activity impairment than non-TRD (Qiao et al., 2017; Jaffe et al., 2019). Therefore, understanding the pathogenesis of TRD and exploring potential biomarkers are of great importance to guide clinical treatment.
Patients with TRD may have differences in clinical symptoms at different ages. Previous studies have shown that younger TRD have more severe depressive symptoms than older TRD (Conelea et al., 2017). In addition, older TRD tend to have more severe cognitive and somatic dysfunction (Mulsant and Pollock, 1998; Knöchel et al., 2015). It has also been shown that age has been shown to be a moderator of response to treatment with numerous antidepressants (Ochs-Ross et al., 2020). Therefore, these different clinical symptoms suggest that different neuropathological mechanisms may exist in TRD patients of different ages.
However, there are fewer studies on the age classification of younger and older TRD patients, and there is no uniform consensus. A study defined the age of younger TRD patients as under 60 years (<60 years), while older TRD patients were defined as over 60 years (≥60 years), suggest that the younger group was more likely to have a history of psychiatric hospitalization and higher depression severity scores (Conelea et al., 2017). Another study defined the age range of TRD in adolescents as 14–17 years (Ghaziuddin et al., 2011). In addition, some studies have defined the age range of older TRD as 55–72 years (Lijffijt et al., 2022) and 65–84 years (Gronemann et al., 2020), but these studies were single-age studies and lacked the effect of different age boundaries on the primary outcome. Therefore, the neurobiological evidence for the age boundary between younger and older TRD is unclear and further clinical studies are necessary to elucidate it.
In recent years, resting state-functional functional magnetic resonance (rs-fMRI) has been gradually applied in the field of psychiatric disorders, including MDD (Wang et al., 2020; Liu P. et al., 2021), autism (Guo et al., 2017), and bipolar disorder (Zhang et al., 2021a). Amplitude of low-frequency fluctuation (ALFF) is a commonly studied metric in rs-fMRI and is able to describe the intensity of spontaneous brain activity in the resting state from an energy perspective (Zang et al., 2007). In addition, ALFF has been recently applied to clinical studies of MDD subtypes of disease (Guo et al., 2012; Liu et al., 2015). Only one study of ALFF at different ages in MDD with a first episode and without medication, and the abnormal brain regions in both groups were concentrated in the frontal, temporal, parietal, and occipital lobes (Guo et al., 2013). Up to date, little is known about the neuroimaging differences between younger and older TRD.
However, the use of an earlier age of onset to differentiate between different age groups of MDD patients is susceptible to severe psychopathology and risk factors (Klein et al., 1999; Zisook et al., 2007). Previous studies have found that age-related changes in affective, cognitive, and reasoning functions stabilize between the ages of 20 and 60 (Hedden and Gabrieli, 2004). In addition, another clinical study with a large sample observed different clinical symptoms of MDD in early onset depression (EOD) and late onset depression (LOD), using a cut-off age of 40 years (Korten et al., 2012). Therefore, we focused on younger TRD (21–40 years) and older TRD (41–60 years) as the age division range. This study was based on ALFF and focused on the differences in local functional brain activity between younger TRD and older TRD patients. In addition, to explore whether there is a correlation between abnormal brain area alters and clinical symptoms in the TRD group. This study will provide some insight into understanding the neuropathological mechanisms of TRD at different ages.
Materials and methods
Participants
A total of 40 outpatients with TRD from Guang’anmen Hospital, China Academy of Chinese Medical Sciences, Beijing First Hospital of Integrated Chinese and Western Medicine, and Xuanwu Hospital of the Capital Medical University, were recruited for this study. All patients with TRD showed the initial diagnosis of MDD in the fifth edition of the American Diagnostic and Statistical Manual of Mental Disorders (DSM-V). The inclusion criteria were as follows: (1) age, 21–60 years; (2) 17-item Hamilton depression rating scale (HAMD-17) score > 17; (3) right-handedness; (4) no response to two or more adequate doses and courses of antidepressant therapy. Thirty-eight gender- and age-matched healthy controls (HCs) (16 men and 22 women) were included in the HC group, which reflected the following: (1) age, 21–60 years; (2) HAMD-17 score < 7; (3) right-handedness; (4) no history of any mental illness in first-degree relatives.
The exclusion criteria for patients and HCs were as follows: (1) serious mental illness and other diseases such as cardiovascular and cerebrovascular disorders; (2) history of drug and alcohol abuse; (3) any contraindications to MRI, such as presence of a heart pacemaker, metal fixed false teeth, or severe claustrophobia; (4) pregnant or lactating status; (5) bipolar disorder or suicidal ideation.
All patients were required to sign an informed consent form before enrollment. This study was approved by the ethics committee of Guang’anmen Hospital, China Academy of Chinese Medical Sciences.
Clinical materials and subgroups
In this study, we collected clinical information on all participants, including gender, age, years of education, and duration of illness. Patients in the TRD group were diagnosed by experienced psychiatrists and assessed for depression severity using the HAMD-17 scale. According to previous studies (Korten et al., 2012), all patients were divided into younger TRD group (21–40 years) and older TRD group (41–60 years). The HC group was also divided into two subgroups: younger HC group (21–40 years) and older HC group (41–60 years).
Scan acquisition
All subjects in this study underwent MRI using a Magnetom Skyra 3.0 T scanner (Siemens, Erlangen, Germany), and the scans were performed at Guang’anmen Hospital, China Academy of Chinese Medical Sciences, and the scan parameters were the same. Before the scanning procedure, the subjects were instructed to remain awake and avoid active thinking. During the scanning process, the subjects were required to wear earplugs and noise-canceling headphones, to use a hood to immobilize the head, and to lie flat on the examination bed. The scanning procedure involved a localizer scan, high-resolution three-dimensional T1-weighted imaging, and BOLD-fMRI.
The scanning parameters were as follows: for three-dimensional T1-weighted imaging, time repetition/time echo = 2500/2.98 ms, flip angle = 7°, matrix = 64 × 64, field of view = 256 mm × 256 mm, slice thickness = 1 mm, slice number = 48, slices = 192, scanning time = 6 min 3 s; for BOLD-fMRI, time repetition/time echo = 2000/30 ms, flip angle = 90°, matrix = 64 × 64, field of view = 240 mm × 240 mm, slice number = 43, slice thickness/spacing = 3.0/1.0 mm, number of obtained volumes = 200, and scanning time = 6 min 40 s.
Image processing
fMRI data preprocessing
The acquired rs-fMRI data were preprocessed using MATLAB-based DPARSF 5.1 software (DPARSF 5.11) (Chao-Gan and Yu-Feng, 2010), as follows: (1) conversion of DICOM raw data to NIFTI format; (2) removal of the first 10 time points to stabilize the data; (3) slice timing; (4) realignment of head motion (removal of patients with head movements greater than 2 mm in any direction and motor rotation greater than 2°); (5) the resulting aligned image time series for each subject were each co-registered with the corresponding 3D T1-weighted image and the Diffeomorphic Anatomical Registration Through Exponentiated Lie Algebra (DARTEL) tool was used to normalize the data for all subjects to Montreal Neurological Institute (MNI) space, which was performed using the MNI coordinate space with 3mm × 3mm × 3mm; (6) linear detrending in order to reduce the influence of MRI equipment; (7) regression of covariates, including brain white matter signal, cerebrospinal fluid signal, and head movement parameters; (8) smoothening (a 6-mm full-width at half-maximum Gaussian kernel).
Amplitude of low frequency fluctuations analysis
Data were spatially normalized and smoothed, and a fast Fourier transform was performed to switch the time series to the frequency domain to obtain the power spectrum. The square root of the power spectrum at each frequency was calculated to obtain the average square root of the ALFF measurement for each voxel in the range of 0.01–0.08 Hz. Finally, time bandpass filtering (0.01–0.08 Hz) was performed. To reduce the inter-individual variability, ALFF was transformed to zALFF using Fisher’s z transformation before statistical analysis.
Statistical analyses
Clinical data analysis
Clinical data were analyzed using the SPSS 23.0 statistical software (IBM Corporation, Somers, NY, United States). One-way analysis of variance was used to compare age and educational level among the four groups, and the chi-square test was used to compare gender differences. A two-sample t-test was used to compare the duration of disease and HAMD-17 scores between the two patient groups, with a threshold of P < 0.05 (two-tailed) set as statistically significant.
fMRI data analysis
Within-group patterns
Imaging data were analyzed using the DPARSF toolbox, and a voxel-based one-way analysis of variance was performed to compare the whole-brain ALFF map among the four groups. Gender, age, years of education, and framewise displacement (a metric derived from Jenkinson’s formula < 0.2) were used as covariates, and brain areas with ALFF differences among the four groups were corrected for Gaussian random fields (GRF). The corrected cluster level was set at P < 0.05 (two-tailed), and threshold voxel levels of P < 0.005 were defined as statistically different. The threshold was set to clusters > 20 voxels.
Between-group differences
We extracted the mean ALFF values of abnormal brain regions in each of the four groups and performed post hoc between-group 2-sample t-test analysis in SPSS 23.0 software to show the difference between each two groups (younger TRD group vs. older TRD group, younger TRD group vs. younger HC group, older TRD group vs. older HC group, younger HC group vs. older HC group). And using Bonferroni correction, the threshold was statistically significant at P < 0.0125 (0.05/4).
Correlations with symptoms
To verify the relationship between clinical symptoms and abnormal brain areas in the younger TRD group and the older TRD group, we performed Pearson correlation analysis between ALFF values and HAMD-17 scores for abnormal brain areas extracted from the two groups separately. Significance was set at a statistical threshold of P < 0.05 (two-tailed).
Results
Characteristics of research datasets
Two older TRD patients were excluded because of excessive head movement displacement. Therefore, a total of 19 younger TRD patients, 19 older TRD patients, 19 younger HCs, and 19 older HCs met the inclusion criteria. There were no significant differences between the younger TRD group and the older TRD group in terms of gender, years of education, duration of illness, and HAMD-17 scores. There were no statistical differences between the younger TRD group and the older TRD group in terms of gender, age, and years of education when compared to matched controls in each age group (Table 1).
Abnormal amplitude of low frequency fluctuations among four groups
Age, gender, years of education, and frame displacement were used as covariates. One-way ANOVA revealed statistically significant differences in ALFF among the four groups in the left middle frontal gyrus, right middle temporal gyrus, right postcentral gyrus, left pallidum, and left caudate nucleus (Table 2 and Figure 1).
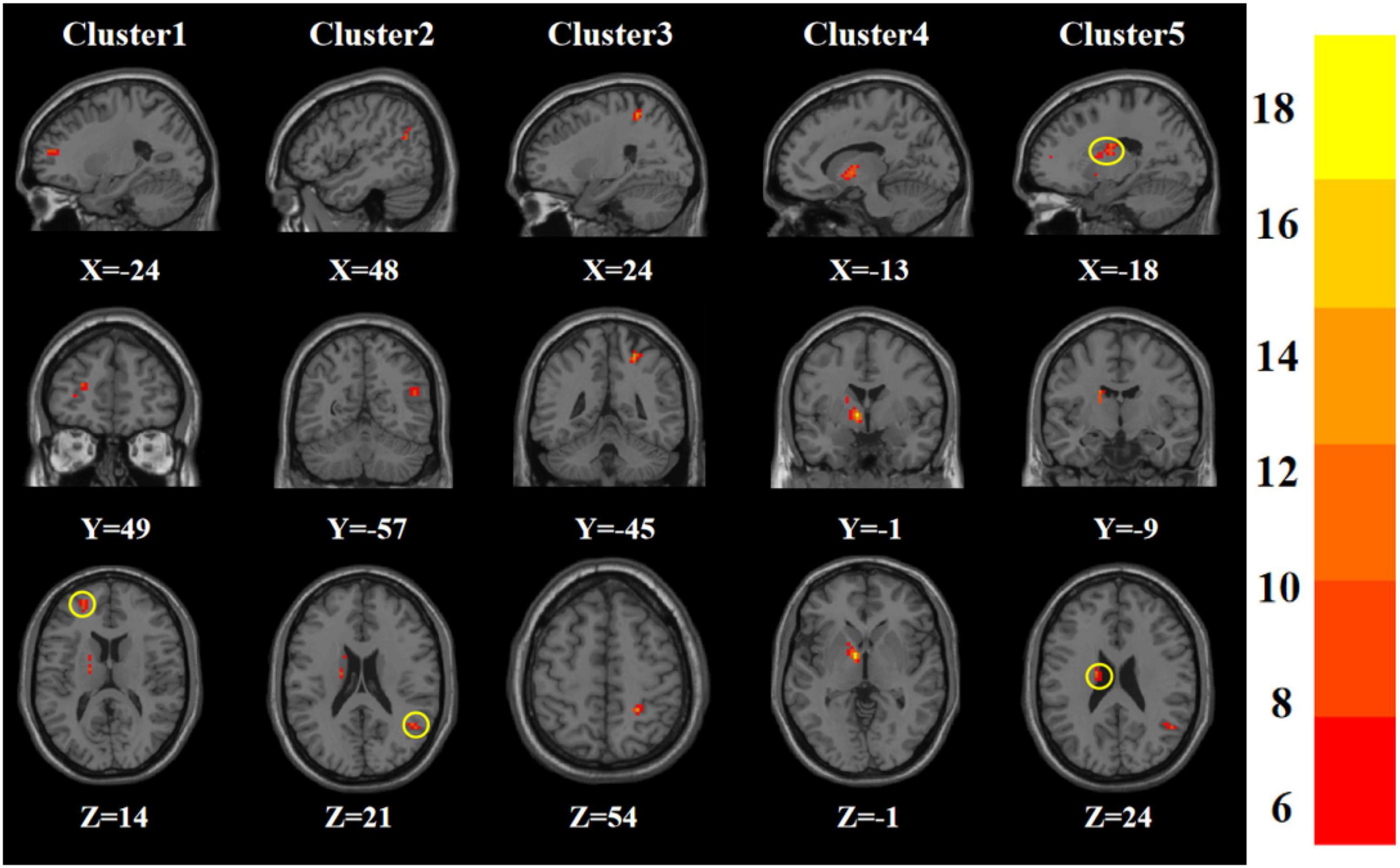
Figure 1. Statistical maps showing ANOVA result of ALFF abnormalities among patients with younger TRD, older TRD group, younger HC group, and older HC group (GRF corrected). The color bars indicate the F-value.
Abnormal amplitude of low frequency fluctuations in younger treatment-resistant depression group vs. older treatment-resistant depression group
Compared to the older TRD group, the younger TRD group had increased ALFF in the left middle frontal gyrus and decreased ALFF in the left caudate nucleus (Figure 2).
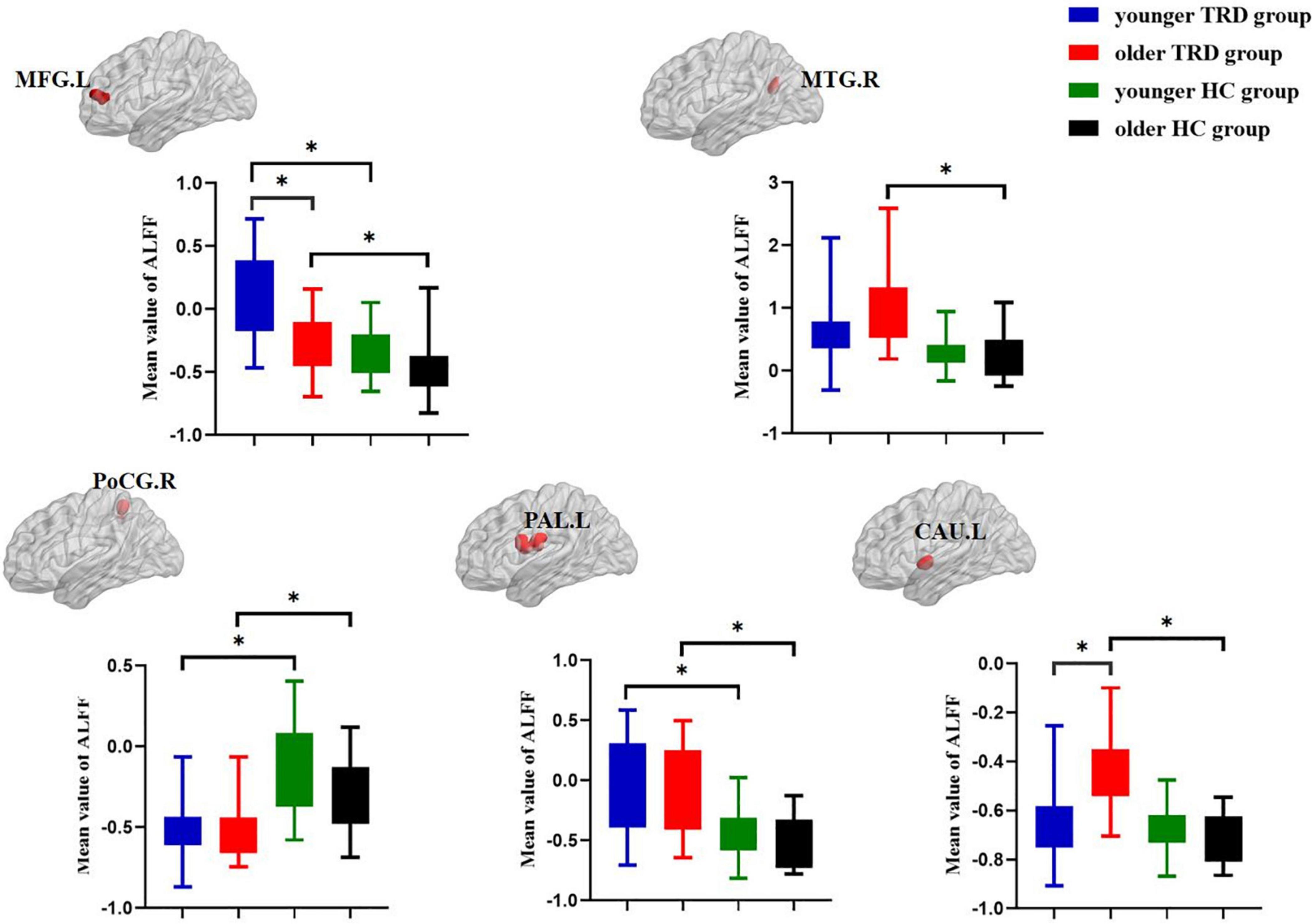
Figure 2. Post hoc two-sample t-tests (Bonferroni corrected) comparison showing ALFF values differences at peak voxel between each pair group (younger TRD group vs. older TRD group, younger TRD group vs. younger HC group, older TRD group vs. older HC group, younger HC group vs. older HC group). MFG.L, Left middle frontal gyrus; MTG.R, Right middle temporal gyrus; PoCG.R, Right postcentral gyrus; PAL.L, Left pallidum; CAU.L, Left caudate nucleus. *P < 0.0125.
Abnormal amplitude of low frequency fluctuations in younger treatment-resistant depression group vs. younger healthy control group
Compared with the younger HC group, the younger TRD group had increased ALFF in the left middle frontal gyrus and left pallidum, and decreased ALFF in the right postcentral gyrus (Figure 2).
Abnormal amplitude of low frequency fluctuations in older treatment-resistant depression group vs. older healthy control group
Compared with the older HC group, the older TRD group had increased ALFF in the left middle frontal gyrus, right middle temporal gyrus, left pallidum, and left caudate nucleus, and decreased ALFF in the right postcentral gyrus (Figure 2).
Abnormal amplitude of low frequency fluctuations in younger healthy control group vs. older healthy control group
There was no significant difference in the comparison of ALFF between the younger HC group and the older HC group (Figure 2).
Correlation between amplitude of low frequency fluctuations and clinical symptoms
To test whether there was a correlation between clinical characteristics and abnormal brain regions ALFF in the younger TRD group and the older TRD group, we further performed a Pearson correlation analysis. We found that the left middle frontal gyrus ALFF values in the younger TRD group were positively correlated with HAMD-17 scores (r = 0.499, P = 0.029). In addition, the right middle temporal gyrus ALFF values in the older TRD group were positively correlated with HAMD-17 scores (r = 0.507, P = 0.026) (Figure 3).
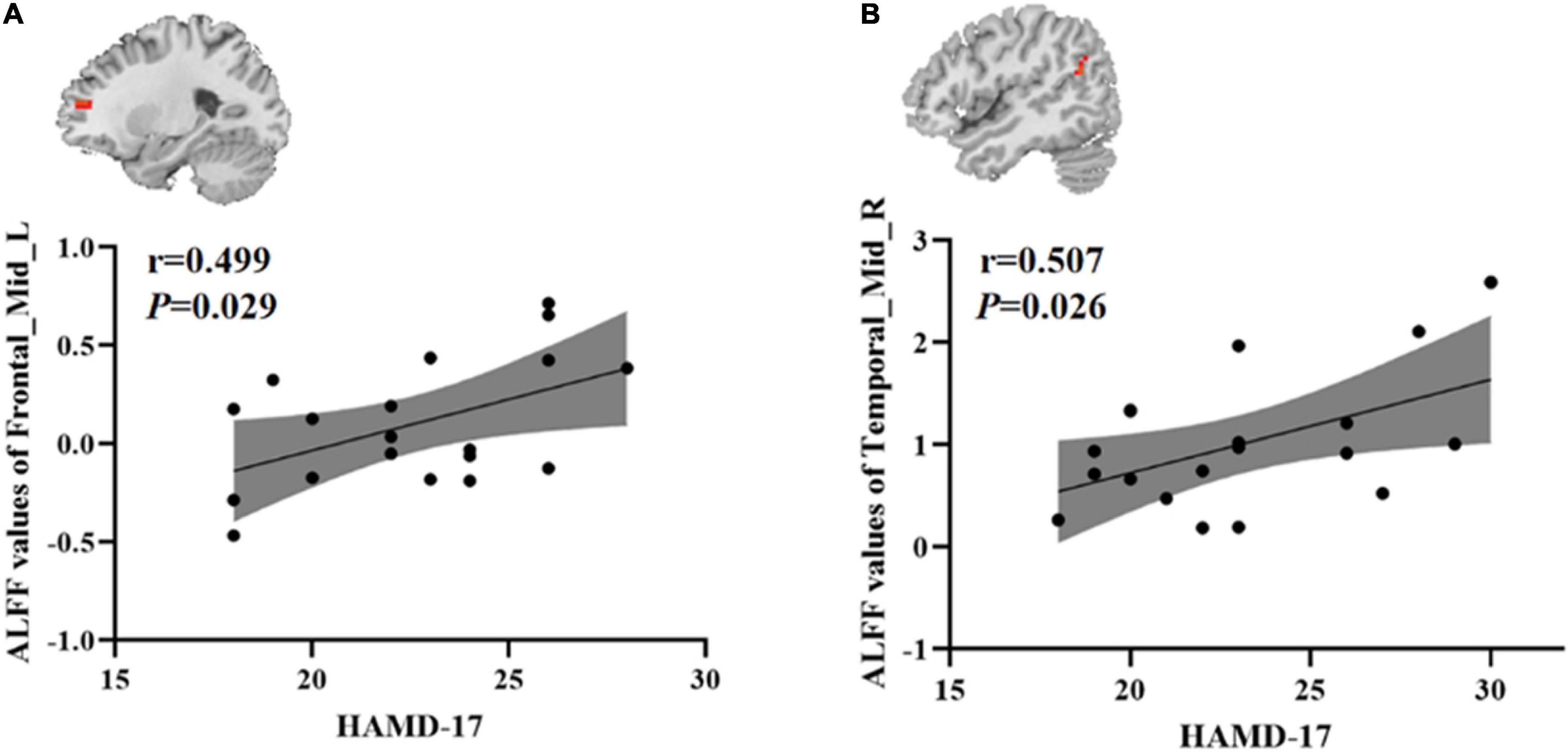
Figure 3. Positive correlation between the ALFF values of abnormal brain regions and the HAMD-17 scores: (A) ALFF values in the younger TRD group; (B) ALFF values in the older TRD group; Frontal_Mid_L, Left middle frontal gyrus; Temporal_Mid_R, Right middle temporal gyrus; ALFF, amplitude of low-frequency fluctuations; HAMD-17, 17-item Hamilton Rating Scale for Depression.
Discussion
To our knowledge, this is the first study using the ALFF method to analyze abnormalities in the physiopathological mechanisms of the brain between younger TRD and older TRD. The present study found no significant differences in clinical symptoms between younger and older TRD, but abnormal neuronal functional activity in some brain regions, with abnormalities associated with cognitive control networks (CCN) and reward networks. Compared to the matched HC group, TRD also exhibited abnormalities in some brain regions at different ages. The older TRD showed more extensive ALFF abnormalities than younger TRD. This study provides new insights into the differences in physiopathological mechanisms in patients with TRD at different ages.
This study found that the younger TRD group had increased ALFF in the left middle frontal gyrus than the older. The middle frontal gyrus is an important component of the dorsolateral prefrontal cortex (DLPFC) and an important component of the CCN, which is closely associated with negative emotions, top-down attention, and working memory (Fales et al., 2009; Wang et al., 2016; Egorova et al., 2018). Patients with MDD with DLPFC damage tend to show low interest in things, memory loss, and lack of motivation (Hamilton et al., 2012; Kang et al., 2012; Martin et al., 2017). Previous studies have found that EOD has increased ALFF in the superior frontal gyrus than LOD, suggesting that hyperactivity of the superior frontal gyrus in the resting state may provoke strong negative affect for the individual (Guo et al., 2012). Another study also showed that regional homogeneity (ReHo) was increased in the right inferior frontal triangular gyrus of the EOD than in the LOD, suggesting that abnormal functional activity in the prefrontal lobe helps to distinguish the EOD from the LOD (Zhang et al., 2021b). Therefore, the results of the present study suggest that the hyperactivity of the left middle frontal gyrus in the resting state in younger TRD may be related to the high level of stress caused by the life and work environment of young people. In addition, we further found that ALFF in the left middle frontal gyrus of the younger TRD group was positively correlated with HAMD-17 scores, whereas this was not found in the older TRD. This suggests that the left middle frontal gyrus may be a neuroimaging marker for young TRD and is an important brain region for distinguishing younger TRD from older TRD.
We found that ALFF was decreased in the left caudate nucleus in the younger TRD group compared to the older TRD group. The caudate nucleus is an important component of the striatum and is one of the central nodes of emotional processing (Pizzagalli et al., 2009; Stoy et al., 2012). The caudate nucleus is involved in the cortico-striato-pallidum-thalamus emotion regulation loop, which regulates the body’s response to external stimuli and maintains the balance of emotion regulation (Alexander et al., 1990; Haber and Calzavara, 2009; Peters et al., 2016). Meanwhile, the caudate nucleus is also an important component of the reward network and is involved in pleasure deficit and motivated reward processing in humans (Macpherson and Hikida, 2019; Cao et al., 2021). Previous studies found that ALFF in the right caudate nucleus was significantly increased in the MDD group than in the HC group, suggesting that abnormal spontaneous brain activity in the caudate nucleus may be associated with MDD (Liu et al., 2014; Chen et al., 2022). Another study also found that ketamine improved patients’ emotional perception through its modulatory effect on the caudate nucleus in TRD patients (Murrough et al., 2015). Therefore, the results of this study suggest that the degree of functional impairment of the left caudate nucleus is more severe in older TRD patients than in younger TRD. This further suggests that different physiopathological mechanisms may exist in patients with TRD at different ages of onset.
We found increased ALFF in the right middle temporal gyrus and left caudate nucleus in the older TRD group compared to the older HC group, which was not found in the younger TRD group compared to the younger HC group. Middle temporal gyrus is involved in emotional perception, audiovisual processing, memory and social cognitive functions, and is also an important component of the default mode network (DMN) (Raichle et al., 2001; Raichle and Snyder, 2007; Xu et al., 2019; Liu M. et al., 2021). Several previous studies have shown abnormalities in the functional activity of the DMN in patients with TRD, and the DMN varies by disease stage and age (de Kwaasteniet et al., 2015; Huang et al., 2020; Woody et al., 2021). A work showed that early-onset recurrent depression was increased ReHo in the right middle temporal gyrus than in the younger HC group, suggesting that this partially compensatory elevation of DMN may be one of the causes of abnormal brain function in early-onset recurrent depression (Sun et al., 2022). Therefore, the results of this study suggest that dysfunction and abnormalities of the right middle temporal gyrus and left caudate nucleus in older patients with TRD may be an important pathogenetic mechanism in younger patients with TRD. In addition, we found that ALFF values in the right middle temporal gyrus of the older TRD group were positively correlated with HAMD-17 scores, suggesting that this region may be an important neuroimaging marker and potential therapeutic target for older TRD patients. However, whether ALFF in this brain region associated with clinical symptoms can be a valid marker of TRD progression needs to be further elucidated.
Interestingly, compared with the two matched HC groups, the two subtype TRD groups had increased ALFF in the left middle frontal gyrus, left pallidum, and decreased ALFF in the right postcentral gyrus. The pallidum is not only a component of the striatum, but also a transmission node connecting the prefrontal cortex to the amygdala, which is closely associated with motivation and reward circuits in MDD patients (Smith et al., 2009; Knowland et al., 2017). Previous studies have found that the functional connectivity (FC) of median cingulate and paracingulate gyri and left pallidum was decreased in MDD patients compared to the HC group (Huang et al., 2021). In addition, a review also showed that vagus nerve stimulation and deep brain stimulation can reverse striatal abnormalities and thus alleviate TRD symptoms (Mohr et al., 2011). The postcentral gyrus belongs to the somatosensory-motor area, which is a higher-level center for the regulation of somatosensory and motor functions in the human body and an important part of the frontoparietal network, and is closely related to executive control and emotion management functions (Zhang et al., 2019; Liu M. et al., 2021). The postcentral gyrus plays an important role in the physiopathological mechanisms of TRD, and abnormalities of the postcentral gyrus predispose therefore TRD patients to somatic disorders (Klok et al., 2019). Previous studies have also found that electroconvulsive therapy can alleviate residual dysfunction in depression by reversing abnormal FC in the middle occipital gyrus and postcentral gyrus. Therefore, the results of this study suggest that cognitive control, reward motivation, and somatosensory-motor function were impaired in patients with TRD at different ages, independent of age of onset.
Some limitations need to be noted. First, for ethical reasons, TRD patients were not discontinued from antidepressants prior to enrollment. Therefore, we do not exclude the potential effects of antidepressants on brain function. Second, we only compared the differences in brain function between the TRD and HC groups, but the nTRD group was not included in this study. Therefore, the results of brain regions with abnormal ALFF (TRD group vs. HC group) only suggest an association with major depression and lack the specificity of TRD pathophysiology, which needs further study in the future. Third, only one clustering method was used to analyze the images in this study, and we will use different clustering methods to compare the results in future studies to improve the scientific significance of the results of this study. Finally, the small sample size of the present study limited the age classification range of the subjects. Therefore, we will further expand the sample size in future studies to improve the scientific value of this study.
Conclusion
To summarize, we found that different neuropathological mechanisms may exist in TRD patients of different ages, especially in the left middle frontal gyrus and left caudate nucleus. This study is beneficial to provide potential key targets for clinical treatment of TRD patients in different age groups.
Data availability statement
The raw data supporting the conclusions of this article will be made available by the authors, without undue reservation.
Ethics statement
The experimental protocol was approved by the Ethics Committee of Guang’anmen Hospital, China Academy of Chinese Medical Science (NO. 2017-021-SQ), Trial registration, China Clinical Trials Registry, chiCTR1800014277. All patients signed an informed consent form before enrollment. The patients/participants provided their written informed consent to participate in this study. Written informed consent was obtained from the individual(s) for the publication of any potentially identifiable images or data included in this article.
Author contributions
JF conceived and designed the experiments and revised the manuscript. JS collected cases, analyzed data, and wrote manuscript. CG analyzed data and revised manuscript. YM, ZD, ZW, YiL, LC, DG, XL, and KX collected cases and analyzed data. YH scanned the subjects. XY and XX evaluated patients and collected cases. YoL revised the manuscript. All authors contributed to the article and approved the submitted version.
Funding
This research was supported by the National Natural Science Foundation of China (82174282 and 81774433), China Academy of Chinese Medical Sciences Innovation Fund (CI2021A03301), and National Key Research and Development Program of China (2018YFC1705802).
Conflict of interest
The authors declare that the research was conducted in the absence of any commercial or financial relationships that could be construed as a potential conflict of interest.
Publisher’s note
All claims expressed in this article are solely those of the authors and do not necessarily represent those of their affiliated organizations, or those of the publisher, the editors and the reviewers. Any product that may be evaluated in this article, or claim that may be made by its manufacturer, is not guaranteed or endorsed by the publisher.
Footnotes
References
Alexander, G. E., Crutcher, M. D., and DeLong, M. R. (1990). Basal ganglia-thalamocortical circuits: Parallel substrates for motor, oculomotor, “prefrontal” and “limbic” functions. Prog. Brain Res. 85, 119–146.
Bergfeld, I. O., Mantione, M., Figee, M., Schuurman, P. R., Lok, A., and Denys, D. (2018). Treatment-resistant depression and suicidality. J. Affect. Disord. 235, 362–367. doi: 10.1016/j.jad.2018.04.016
Cao, Z., Ottino-Gonzalez, J., Cupertino, R. B., Juliano, A., Chaarani, B., and Banaschewski, T. (2021). Characterizing reward system neural trajectories from adolescence to young adulthood. Dev. Cogn. Neurosci. 52:101042. doi: 10.1016/j.dcn.2021.101042
Chao-Gan, Y., and Yu-Feng, Z. (2010). DPARSF: A MATLAB toolbox for “pipeline” data analysis of resting-state fmri. Front. Syst. Neurosci. 4:13. doi: 10.3389/fnsys.2010.00013
Chen, Q., Bi, Y., Zhao, X., Lai, Y., Yan, W., Xie, L., et al. (2022). Regional amplitude abnormities in the major depressive disorder: A resting-state fMRI study and support vector machine analysis. J. Affect. Disord. 308, 1–9. doi: 10.1016/j.jad.2022.03.079
Conelea, C. A., Philip, N. S., Yip, A. G., Barnes, J. L., Niedzwiecki, M. J., Greenberg, B. D., et al. (2017). Transcranial magnetic stimulation for treatment-resistant depression: Naturalistic treatment outcomes for younger versus older patients. J. Affect. Disord. 217, 42–47. doi: 10.1016/j.jad.2017.03.063
de Kwaasteniet, B. P., Rive, M. M., Ruhé, H. G., Schene, A. H., Veltman, D. J., Fellinger, L., et al. (2015). Decreased Resting-State Connectivity between Neurocognitive Networks in Treatment Resistant Depression. Front. Psychiatry 6:28. doi: 10.3389/fpsyt.2015.00028
Egorova, N., Cumming, T., Shirbin, C., Veldsman, M., Werden, E., and Brodtmann, A. (2018). Lower cognitive control network connectivity in stroke participants with depressive features. Transl. Psychiatry 7:4. doi: 10.1038/s41398-017-0038-x
Fabbri, C., Pain, O., Hagenaars, S. P., Lewis, C. M., and Serretti, A. (2021). Transcriptome-wide association study of treatment-resistant depression and depression subtypes for drug repurposing. Neuropsychopharmacology 46, 1821–1829. doi: 10.1038/s41386-021-01059-6
Fales, C. L., Barch, D. M., Rundle, M. M., Mintun, M. A., Mathews, J., Snyder, A. Z., et al. (2009). Antidepressant treatment normalizes hypoactivity in dorsolateral prefrontal cortex during emotional interference processing in major depression. J. Affect. Disord. 112, 206–211. doi: 10.1016/j.jad.2008.04.027
Gaynes, B. N., Lux, L., Gartlehner, G., Asher, G., Forman-Hoffman, V., Green, J., et al. (2020). Defining treatment-resistant depression. Depress. Anxiety 37, 134–145. doi: 10.1002/da.22968
Ghaziuddin, N., Dumas, S., and Hodges, E. (2011). Use of continuation or maintenance electroconvulsive therapy in adolescents with severe treatment-resistant depression. J. ECT 27, 168–174. doi: 10.1097/YCT.0b013e3181f665e4
Gronemann, F. H., Jorgensen, M. B., Nordentoft, M., Andersen, P. K., and Osler, M. (2020). Socio-demographic and clinical risk factors of treatment-resistant depression: A Danish population-based cohort study. J. Affect. Disord. 261, 221–229. doi: 10.1016/j.jad.2019.10.005
Guo, W. B., Liu, F., Xue, Z. M., Xu, X. J., Wu, R. R., and Ma, C. Q. (2012). Alterations of the amplitude of low-frequency fluctuations in treatment-resistant and treatment-response depression: A resting-state fMRI study. Prog. Neuro Psychopharmacol. Biol. Psychiatry 37, 153–160. doi: 10.1016/j.pnpbp.2012.01.011
Guo, W. B., Liu, F., Xun, G. L., Hu, M. R., Guo, X. F., Xiao, C. Q., et al. (2013). Reversal alterations of amplitude of low-frequency fluctuations in early and late onset, first-episode, drug-naive depression. Prog. Neuro Psychopharmacol. Biol. Psychiatry 40, 153–159. doi: 10.1016/j.pnpbp.2012.08.014
Guo, X., Chen, H., Long, Z., Duan, X., Zhang, Y., and Chen, H. (2017). Atypical developmental trajectory of local spontaneous brain activity in autism spectrum disorder. Sci. Rep. 7:39822. doi: 10.1038/srep39822
Haber, S. N., and Calzavara, R. (2009). The cortico-basal ganglia integrative network: The role of the thalamus. Brain Res. Bull. 78, 69–74. doi: 10.1016/j.brainresbull.2008.09.013
Hamilton, J. P., Etkin, A., Furman, D. J., Lemus, M. G., Johnson, R. F., and Gotlib, I. H. (2012). Functional neuroimaging of major depressive disorder: A meta-analysis and new integration of base line activation and neural response data. Am. J. Psychiatry 169, 693–703. doi: 10.1176/appi.ajp.2012.11071105
Hedden, T., and Gabrieli, J. D. (2004). Insights into the ageing mind: A view from cognitive neuroscience. Nat. Rev. Neurosci. 5, 87–96. doi: 10.1038/nrn1323
Ho, M. C., Hsu, Y. C., Lu, M. L., Gossop, M., and Chen, V. C. (2018). ‘Cool’ and ‘Hot’ executive functions in suicide attempters with major depressive disorder. J. Affect. Disord. 235, 332–340. doi: 10.1016/j.jad.2018.04.057
Huang, H., Botao, Z., Jiang, Y., Tang, Y., Zhang, T., Tang, X., et al. (2020). Aberrant resting-state functional connectivity of salience network in first-episode schizophrenia. Brain Imaging Behav. 14, 1350–1360. doi: 10.1007/s11682-019-00040-8
Huang, Q., Xiao, M., Ai, M., Chen, J., Wang, W., Hu, L., et al. (2021). Disruption of Neural Activity and Functional Connectivity in Adolescents With Major Depressive Disorder Who Engage in Non-suicidal Self-Injury: A Resting-State fMRI Study. Front. Psychiatry 12:571532. doi: 10.3389/fpsyt.2021.571532
Jaffe, D. H., Rive, B., and Denee, T. R. (2019). The humanistic and economic burden of treatment-resistant depression in Europe: A cross-sectional study. BMC Psychiatry 19:247. doi: 10.1186/s12888-019-2222-4
Kang, H. J., Voleti, B., Hajszan, T., Rajkowska, G., Stockmeier, C. A., Licznerski, P., et al. (2012). Decreased expression of synapse-related genes and loss of synapses in major depressive disorder. Nat. Med. 18, 1413–1417. doi: 10.1038/nm.2886
Klein, D. N., Schatzberg, A. F., McCullough, J. P., Dowling, F., Goodman, D., Howland, R. H., et al. (1999). Age of onset in chronic major depression: Relation to demographic and clinical variables, family history, and treatment response. J. Affect. Disord. 55, 149–157. doi: 10.1016/s0165-0327(99)00020-8
Klok, M., van Eijndhoven, P. F., Argyelan, M., Schene, A. H., and Tendolkar, I. (2019). Structural brain characteristics in treatment-resistant depression: Review of magnetic resonance imaging studies. BJPsych Open 5:e76. doi: 10.1192/bjo.2019.58
Knöchel, C., Alves, G., Friedrichs, B., Schneider, B., Schmidt-Rechau, A., Wenzler, S., et al. (2015). Treatment-resistant Late-life Depression: Challenges and Perspectives. Curr. Neuropharmacol. 13, 577–591. doi: 10.2174/1570159x1305151013200032
Knowland, D., Lilascharoen, V., Pacia, C. P., Shin, S., Wang, E. H., and Lim, B. K. (2017). Distinct Ventral Pallidal Neural Populations Mediate Separate Symptoms of Depression. Cell 170, 284–297.e18. doi: 10.1016/j.cell.2017.06.015
Korten, N. C., Comijs, H. C., Lamers, F., and Penninx, B. W. (2012). Early and late onset depression in young and middle aged adults: Differential symptomatology, characteristics and risk factors? J. Affect. Disord. 138, 259–267. doi: 10.1016/j.jad.2012.01.042
Lawson, R. P., Nord, C. L., Seymour, B., Thomas, D. L., Dayan, P., Pilling, S., et al. (2017). Disrupted habenula function in major depression. Mol. Psychiatry 22, 202–208. doi: 10.1038/mp.2016.81
Lijffijt, M., Murphy, N., Iqbal, S., Green, C. E., Iqbal, T., Chang, L. C., et al. (2022). Identification of an optimal dose of intravenous ketamine for late-life treatment-resistant depression: A Bayesian adaptive randomization trial. Neuropsychopharmacology 47, 1088–1095. doi: 10.1038/s41386-021-01242-9
Liu, C. H., Ma, X., Song, L. P., Tang, L. R., Jing, B., Zhang, Y., et al. (2015). Alteration of spontaneous neuronal activity within the salience network in partially remitted depression. Brain Res. 1599, 93–102. doi: 10.1016/j.brainres.2014.12.040
Liu, J., Ren, L., Womer, F. Y., Wang, J., Fan, G., Jiang, W., et al. (2014). Alterations in amplitude of low frequency fluctuation in treatment-naïve major depressive disorder measured with resting-state fMRI. Hum. Brain Mapp. 35, 4979–4988. doi: 10.1002/hbm.22526
Liu, P., Tu, H., Zhang, A., Yang, C., Liu, Z., Lei, L., et al. (2021). Brain functional alterations in MDD patients with somatic symptoms: A resting-state fMRI study. J. Affect. Disord. 295, 788–796. doi: 10.1016/j.jad.2021.08.143
Liu, M., Wang, Y., Zhang, A., Yang, C., Liu, P., Wang, J., et al. (2021). Altered dynamic functional connectivity across mood states in bipolar disorder. Brain Res. 1750:147143. doi: 10.1016/j.brainres.2020.147143
Macpherson, T., and Hikida, T. (2019). Role of basal ganglia neurocircuitry in the pathology of psychiatric disorders. Psychiatry Clin. Neurosci. 73, 289–301. doi: 10.1111/pcn.12830
Martin, D. M., McClintock, S. M., Forster, J. J., Lo, T. Y., and Loo, C. K. (2017). Cognitive enhancing effects of rTMS administered to the prefrontal cortex in patients with depression: A systematic review and meta-analysis of individual task effects. Depress. Anxiety 34, 1029–1039. doi: 10.1002/da.22658
Mohr, P., Rodriguez, M., Slavíčková, A., and Hanka, J. (2011). The application of vagus nerve stimulation and deep brain stimulation in depression. Neuropsychobiology 64, 170–181. doi: 10.1159/000325225
Mulsant, B. H., and Pollock, B. G. (1998). Treatment-resistant depression in late life. J. Geriatr. Psychiatry Neurol. 11, 186–193. doi: 10.1177/089198879901100404
Murrough, J. W., Collins, K. A., Fields, J., DeWilde, K. E., Phillips, M. L., Mathew, S. J., et al. (2015). Regulation of neural responses to emotion perception by ketamine in individuals with treatment-resistant major depressive disorder. Transl. Psychiatry 5:e509. doi: 10.1038/tp.2015.10
Ochs-Ross, R., Daly, E. J., Zhang, Y., Lane, R., Lim, P., Morrison, R. L., et al. (2020). Efficacy and safety of esketamine nasal spray plus an oral antidepressant in elderly patients with treatment-resistant depression-TRANSFORM-3. Am. J. Geriatr. Psychiatry 28, 121–141. doi: 10.1016/j.jagp.2019.10.008
Peters, S. K., Dunlop, K., and Downar, J. (2016). Cortico-striatal-thalamic loop circuits of the salience network: a central pathway in psychiatric disease and treatment. Front. Syst. Neurosci. 10:104. doi: 10.3389/fnsys.2016.00104
Pizzagalli, D. A., Holmes, A. J., Dillon, D. G., Goetz, E. L., Birk, J. L., Bogdan, R., et al. (2009). Reduced caudate and nucleus accumbens response to rewards in unmedicated individuals with major depressive disorder. Am. J. Psychiatry 166, 702–710. doi: 10.1176/appi.ajp.2008.08081201
Qiao, J., Qian, L. J., Zhao, H. F., Gong, G. H., and Geng, D. Q. (2017). The relationship between quality of life and clinical phenotype in patients with treatment resistant and non-treatment resistant depression. Eur. Rev. Med. Pharmacol. Sci. 21, 2432–2436.
Raichle, M. E., MacLeod, A. M., Snyder, A. Z., Powers, W. J., Gusnard, D. A., and Shulman, G. L. (2001). A default mode of brain function. Proc. Natl. Acad. Sci. U.S.A. 98, 676–682. doi: 10.1073/pnas.98.2.676
Raichle, M. E., and Snyder, A. Z. (2007). A default mode of brain function: A brief history of an evolving idea. NeuroImage 37, 1083–1099. doi: 10.1016/j.neuroimage.2007.02.041
Smith, K. S., Tindell, A. J., Aldridge, J. W., and Berridge, K. C. (2009). Ventral pallidum roles in reward and motivation. Behav. Brain Res. 196, 155–167. doi: 10.1016/j.bbr.2008.09.038
Stoy, M., Schlagenhauf, F., Sterzer, P., Bermpohl, F., Hägele, C., Suchotzki, K., et al. (2012). Hyporeactivity of ventral striatum towards incentive stimuli in unmedicated depressed patients normalizes after treatment with escitalopram. J. Psychopharmacol. 26, 677–688. doi: 10.1177/0269881111416686
Sun, J. F., Chen, L. M., He, J. K., Wang, Z., Guo, C. L., Ma, Y., et al. (2022). A Comparative Study of Regional Homogeneity of Resting-State fMRI Between the Early-Onset and Late-Onset Recurrent Depression in Adults. Front. Psychology 13:849847. doi: 10.3389/fpsyg.2022.849847
Wang, M., Ju, Y., Lu, X., Sun, J., Dong, Q., Liu, J., et al. (2020). Longitudinal changes of amplitude of low-frequency fluctuations in MDD patients: A 6-month follow-up resting-state functional magnetic resonance imaging study. J. Affect. Disord. 276, 411–417. doi: 10.1016/j.jad.2020.07.067
Wang, Y. L., Yang, S. Z., Sun, W. L., Shi, Y. Z., and Duan, H. F. (2016). Altered functional interaction hub between affective network and cognitive control network in patients with major depressive disorder. Behav. Brain Res. 298, 301–309. doi: 10.1016/j.bbr.2015.10.040
Woody, M. L., Panny, B., Degutis, M., Griffo, A., and Price, R. B. (2021). Resting state functional connectivity subtypes predict discrete patterns of cognitive-affective functioning across levels of analysis among patients with treatment-resistant depression. Behav. Res. Ther. 146:103960. doi: 10.1016/j.brat.2021.103960
Xu, J., Lyu, H., Li, T., Xu, Z., Fu, X., Jia, F., et al. (2019). Delineating functional segregations of the human middle temporal gyrus with resting-state functional connectivity and coactivation patterns. Hum. Brain Mapp. 40, 5159–5171. doi: 10.1002/hbm.24763
Zang, Y. F., He, Y., Zhu, C. Z., Cao, Q. J., Sui, M. Q., Liang, M., et al. (2007). Altered baseline brain activity in children with ADHD revealed by resting-state functional MRI. Brain Dev. 29, 83–91. doi: 10.1016/j.braindev.2006.07.002
Zhang, H., Qiu, M., Ding, L., Mellor, D., Li, G., Shen, T., et al. (2019). Intrinsic gray-matter connectivity of the brain in major depressive disorder. J. Affect. Disord. 251, 78–85. doi: 10.1016/j.jad.2019.01.048
Zhang, Z., Bo, Q., Li, F., Zhao, L., Wang, Y., Liu, R., et al. (2021a). Increased ALFF and functional connectivity of the right striatum in bipolar disorder patients. Prog. Neuropsychopharmacol. Biol. Psychiatry 111:110140. doi: 10.1016/j.pnpbp.2020.110140
Zhang, Z., Chen, Y., Wei, W., Yang, X., Meng, Y., Yu, H., et al. (2021b). Changes in Regional Homogeneity of Medication-Free Major Depressive Disorder Patients With Different Onset Ages. Front. Psychiatry 12:713614. doi: 10.3389/fpsyt.2021.713614
Keywords: treatment-resistant depression (TRD), amplitude of low-frequency fluctuation, MRI, major depressive disorder (MDD), age
Citation: Sun J, Guo C, Ma Y, Du Z, Wang Z, Luo Y, Chen L, Gao D, Li X, Xu K, Hong Y, Yu X, Xiao X, Fang J and Liu Y (2022) A comparative study of amplitude of low-frequence fluctuation of resting-state fMRI between the younger and older treatment-resistant depression in adults. Front. Neurosci. 16:949698. doi: 10.3389/fnins.2022.949698
Received: 21 May 2022; Accepted: 08 August 2022;
Published: 25 August 2022.
Edited by:
Xiang Li, Massachusetts General Hospital and Harvard Medical School, United StatesReviewed by:
Caihong Wang, The First Affiliated Hospital of Zhengzhou University, ChinaEnze Shi, Northwestern Polytechnical University, China
Copyright © 2022 Sun, Guo, Ma, Du, Wang, Luo, Chen, Gao, Li, Xu, Hong, Yu, Xiao, Fang and Liu. This is an open-access article distributed under the terms of the Creative Commons Attribution License (CC BY). The use, distribution or reproduction in other forums is permitted, provided the original author(s) and the copyright owner(s) are credited and that the original publication in this journal is cited, in accordance with accepted academic practice. No use, distribution or reproduction is permitted which does not comply with these terms.
*Correspondence: Jiliang Fang, fangmgh@163.com; Yong Liu, 1909768139@qq.com
†These authors have contributed equally to this work and share first authorship