- 1Animal and Bioscience Research Department, Animal and Grassland Research and Innovation Centre, Teagasc, Athenry, Ireland
- 2Genetics and Biotechnology Laboratory, Plant and AgriBiosciences Research Centre (PABC), Ryan Institute, National University of Ireland Galway, Galway, Ireland
Rumen microbiome composition and functionality is linked to animal feed efficiency, particularly for bovine ruminants. To investigate this in sheep, we compared rumen bacterial and archaeal populations (and predicted metabolic processes) of sheep divergent for the feed efficiency trait feed conversion ratio (FCR). In our study 50 Texel cross Scottish Blackface (TXSB) ram lambs were selected from an original cohort of 200 lambs. From these, 26 were further selected for experimentation based on their extreme FCR (High Feed Efficiency, HFE = 13; Low Feed Efficiency, LFE = 13). Animals were fed a 95% concentrate diet ad libitum over 36 days. 16S rRNA amplicon sequencing was used to investigate the rumen bacterial and archaeal communities in the liquid and solid rumen fractions of sheep divergent for FCR. Weighted UniFrac distances separated HFE and LFE archaea communities from the liquid rumen fraction (Permanova, P < 0.05), with greater variation observed for the LFE cohort (Permdisp, P < 0.05). LFE animals exhibited greater Shannon and Simpson diversity indices, which was significant for the liquid rumen fraction (P < 0.05). Methanobrevibacter olleyae (in liquid and solid fractions) and Methanobrevibacter millerae (liquid fraction) were differentially abundant, and increased in the LFE cohort (P.adj < 0.05), while Methanobrevibacter wolinii (liquid fraction) was increased in the HFE cohort (P.adj < 0.05). This suggests that methanogenic archaea may be responsible for a potential loss of energy for the LFE cohort. Bacterial community composition (Permanova, P > 0.1) and diversity (P > 0.1) was not affected by the FCR phenotype. Only the genus Prevotella 1 was differentially abundant between HFE and LFE cohorts. Although no major compositional shifts of bacterial populations were identified amongst the feed efficient cohorts (FDR > 0.05), correlation analysis identified putative drivers of feed efficiency with Ruminococcaceae UCG-014 (liquid, rho = −0.53; solid, rho = −0.56) and Olsenella (solid, rho = −0.40) exhibiting significant negative association with FCR (P < 0.05). Bifidobacterium and Megasphaera showed significant positive correlations with ADG. Major cellulolytic bacteria Fibrobacter (liquid, rho = 0.43) and Ruminococcus 1 (liquid, rho = 0.41; solid, rho = 41) correlated positively with FCR (P < 0.05). Our study provides evidence that feed efficiency in sheep is likely influenced by compositional changes to the archaeal community, and abundance changes of specific bacteria, rather than major overall shifts within the rumen microbiome.
Introduction
The world’s population is expected to increase by 2 billion persons in the next 30 years, from 7.7 billion currently to 9.7 billion in 2050 (UN, 2019). In addition, rising gross domestic product (GDP) in developing countries and urbanization is driving dietary shifts toward animal-based protein products (Thornton, 2010; Henchion et al., 2017). There is increasing demand on livestock production systems to support the dietary requirements and demand of a rapidly growing population (Hunter et al., 2017). Feed is the largest economic factor influencing profitability in livestock enterprises, accounting for up to 70% of total direct costs (Kenny et al., 2018). Due to the cost of feed as an external input, improving profitability of livestock systems has significantly focused on the identification of animals capable of maximizing the utilization of feed (McGovern et al., 2018). Research to date provides evidence that highly feed efficient animals consume less feed, while at the same time maintaining the same level of production as less efficient animals (Carberry et al., 2012; Shabat et al., 2016; Claffey et al., 2018). Additionally, highly efficient animals produce less methane and less manure due to reduced consumption of feed (Kenny et al., 2018). Therefore, improving feed efficiency has the potential to simultaneously increase profitability within the livestock sector while reducing the environmental impact of livestock production.
Feed Conversion Ratio (FCR) and Residual Feed Intake (RFI) are two widely used measures of feed efficiency (Bhatt et al., 2013; Zhang et al., 2017; Claffey et al., 2018; McGovern et al., 2018). FCR is calculated as the kilogram ratio of dry matter intake (DMI) to average daily gain (ADG), while RFI measures the residual difference between observed and predicted feed intake against bodyweight maintenance and animal performance (Berry and Crowley, 2013). FCR and RFI have an inverse relationship with feed efficiency, with superior FCR and RFI measures corresponding to poorer animal production, and vice versa (Cannas et al., 2019). Both measures are related as they both require feed intake as a variable. However, a major limitation of FCR is that it is dependent on ADG, which can result in the selection of larger and faster growing animals that require more maintenance. In contrast, RFI is independent of growth rate and considered a more robust measurement of feed efficiency (Santana et al., 2012).
Ruminants depend on the microbes (composed mainly of bacteria, archaea, fungi, and protozoa) residing in the rumen to ferment and transform their feed into volatile fatty acids (VFAs), proteins and vitamins. The primary VFAs produced (acetate, propionate, and butyrate) contribute approximately 80% of the hosts metabolized energy requirements (Keogh et al., 2017; Li and Guan, 2017; Abecia et al., 2018; Zeineldin et al., 2018). Furthermore, the concentrations of different VFAs within the rumen have been associated with feed efficiency of the host (Li and Guan, 2017). The underlying biological mechanisms regulating production efficiency are dependent on a number of internal and external factors including age, sex, genotype, and diet, all of which are known to influence rumen microbial structure and function (Henderson et al., 2015; Shabat et al., 2016; Claffey et al., 2018; Thomas et al., 2019). Hence, there is a potential association between feed efficiency and the rumen microbiome. Indeed, previous research performed by our group and others has identified links between the rumen microbiome and animal variation in feed efficiency phenotypes (Carberry et al., 2012; Jewell et al., 2015; Shabat et al., 2016; Ellison et al., 2017; McGovern et al., 2018).
Understanding of microbiome composition and functioning has advanced in recent years through the application of high-throughput next-generation sequencing (NGS) technologies for metagenomic analyses (Quince et al., 2017). Popular NGS platforms such as the MISeq (Illumina) and MINion (Oxford Nanopore) coupled with metagenomic approaches that either target specific genes (16S rRNA) or the whole bacterial genome, are providing insights into complex microbial populations in the rumen, which are otherwise difficult to identify using culture-dependent approaches (Zhou et al., 2015; Kachiprath et al., 2018; Gu et al., 2019). Additionally, the development of user-friendly computational software is enabling researchers to extrapolate more information from biological data. For instance, CowPI, a functional prediction tool, can infer the functional potential of different rumen microbiome profiles using 16S rRNA data (Wilkinson et al., 2018).
There are approximately 1.2 billion sheep in the world that are primarily reared for commodities such as meat, milk and wool (Pulina et al., 2018). Sheep production remains an important agricultural enterprise internationally, which is exemplified by continual annual growth of the sheep dairy sector (Pulina et al., 2018). To date, most research investigating the relationship between feed efficiency and the rumen microbiome has been conducted in cattle. However, sheep are less expensive, require less feed, reach maturity quicker and are more manageable than cattle, making sheep a practical and economical model for ruminant research (Delano et al., 2002).
In a previous study by our group (Claffey et al., 2018), FCR was measured for a cohort of Texel cross Scottish Blackface (TXSB) lamb rams over 36 days and was found to vary across the group. While the rumen microbiome has been shown to be associated with feed efficiency in cattle (Carberry et al., 2012; Jewell et al., 2015; McGovern et al., 2018) such a relationship has not been extensively examined in sheep. Therefore, the objective of the current study was to investigate the bacterial and archaeal populations present in both solid and liquid fractions of the rumen of sheep that are divergent for the FCR phenotype, using amplicon sequencing targeting the 16S rRNA gene. In addition, the archaeal and bacterial populations identified were correlated with FCR to further identify possible microbial drivers of feed efficiency. To determine the potential functionality of the microbiome taxa that are differentially abundant due to FCR, CowPI (Wilkinson et al., 2018) was used to predict functional genes of metabolic pathways associated with feed efficiency in sheep.
Materials and Methods
All animal procedures used in this study were conducted under experimental license from Ireland’s Health Products Regulatory Authority (HPRA) in accordance with the European Union (EU) protection of animals used for scientific purposes regulations 2012 (S.I. No. 543 of 2012). This study was conducted as part of a larger study designed to examine the production efficiency of purebred Scottish Blackface and TXSB wether and ram lambs (n = 200) (Claffey et al., 2018). The current study focused on the rams of the TXSB breeds of sheep used in that study. Briefly, twenty-six lambs of the TXSB were separated into divergent feed conversion efficiency quartile cohorts [high and low feed efficiency (HFE and LFE) animals with 13 animals in each group] according to their extreme FCR values, from an original group of 50 individuals [HFE = 3.83 ± 0.40, LFE = 6.05 ± 0.92, (p < 0.05)]. The experiment was performed over a period of 36 days of intensive indoor feeding. Lambs were individually penned on expanded metal-floored feeding pens (182 cm L × 122 cm W) and allowed tactile, olfactory, and visual contact with each other through the pen partitions. Lambs were allowed a 12-days pre-experimental acclimatization period to adapt to a 95% concentrate diet. Relative to commencement of ad libitum, concentrate feeding (day 0), lambs were offered 150-g/d fresh weight of concentrate feed on days −12, −11, and −10 increasing by 100-g/d fresh weight concentrate on each day from days −9 to day −1 to minimize the risk of any digestive upsets. For the duration of the finishing period, lambs were offered 100-g/d DM of silage and had ad libitum access to concentrates; ad libitum concentrate was described as access to concentrate feed at all times over the 36-days experimental period. Concentrate and silage samples were collected weekly and dried overnight at 55°C and pooled for determination of CP, ADF, NDF, and ash. Concentrate and silage were offered daily with individual lamb refusals recorded twice weekly (Claffey et al., 2018). Lambs were transported to the slaughter facility on the morning of slaughter. Animals were slaughtered at a mean age of 292 days old. Production variables [average daily feed intake (ADI), total weight gain (TWG), FCR, and ADG] were calculated post slaughter. All production data used in the study has been previously described (Claffey et al., 2018).
Rumen Sampling, DNA Extraction and 16s rDNA Library Preparation
Liquid and solid fractions from rumen content were collected immediately after slaughter. Fractions were separated by squeezing rumen digesta through four layers of sterile cheesecloth, which were collected in 250 ml centrifuge bottles. Both fractions were frozen immediately in liquid nitrogen after separation and then stored at −80°C. Under liquid nitrogen, each sample was homogenized to a fine frozen powder using a pestle and mortar. Extraction of microbial DNA from the samples was performed using the method described by Yu and Morrison (2004). DNA purity was assessed using Nanodrop 1000 spectrophotometer. The 260/280 ratio averaged 1.83. To generate the PCR amplicons of the V4 hyper-variable region (of the 16S rDNA), 515F-806R primers were used on a template of 25 ng of rumen microbial DNA (Caporaso et al., 2011). 515F-806R primers target both bacterial and archaeal populations (Willis et al., 2019). The 515F-806R primers were designed with Nextera overhang adapters. The PCR amplification was conducted using 2X KAPA HiFi HotStart ReadyMix DNA polymerase (Roche Diagnostics, West Sussex, United Kingdom). The PCR conditions were as described in McGovern et al. (2018). Finally, the amplicons were sequenced on an Illumina MiSeq platform using the 500-cycle version 2 MiSeq reagent kit (Illumina, San Diego, CA, United States).
Bioinformatic Analysis
Raw paired-end sequenced reads were quality checked with FASTQC (version 0.11.5) (Andrews, 2010). Primers and ambiguous basecalls were removed using Cutadapt (version 1.18) (Martin, 2011). Processing and analysis of amplicon reads was performed using Divisive Amplicon Denoising Algorithm 2 (DADA2), as described in Callahan et al. (2017). Read filtering, dereplication, sample inference, chimera removal, merging of paired end reads, and taxonomic classification were all performed following the DADA2 tutorial from https://benjjneb.github.io/dada2/tutorial.html (version 1.12) with minor alterations. Taxonomic classification was performed to the genus level using the SILVA classification database (sourced from https://zenodo.org/record/1172783#.XWLkpd-YW6A) (Callahan, 2018). The final output from DADA2 was an Amplicon Sequence Variant (ASV) table and a corresponding taxonomy table. A phylogenetic tree was constructed using the phangorn package (Schliep, 2011). A phyloseq object containing the ASV table, taxonomy table, phylogenetic tree and experimental metadata was built using the R/Bioconductor package Phyloseq (version 1.26) (McMurdie and Holmes, 2013) prior to downstream analysis. Finally, CowPI was used to predict the functional processes of the microbial community within each sample using the ASVs generated from the DADA2 pipeline (Wilkinson et al., 2018). Basic local alignment search tool (BLAST) against the rRNA/ITS database was used to further classify methanogens representative ASV sequences of interest (Johnson et al., 2008).
Compositional and Statistical Analysis
Compositional and statistical analyses were carried out using various libraries/packages in R studio (running R version 3.6.1). Samples were separated according to rumen phase (liquid and solid) for independent analysis and compared between the feed efficient cohorts (LFE vs. HFE). Taxa unassigned at the phylum level, with less than 5 counts and prevalent in 3 or less samples were filtered from the data. For the analysis of alpha and beta diversity counts were normalized by subsampling to the minimum sampling depth; bacteria reads (liquid = 63,924, solid = 75,873) and archaea reads (liquid = 896, solid = 1182). Principle coordinate analysis (PCoA) based on weighted and unweighted UniFrac distances was performed for ordination analysis to visualize compositional differences between the two cohorts for both rumen fractions. PERMANOVA analysis with 9999 permutations was conducted using the Adonis function from the R/Bioconductor package Vegan (version 2.5-5) (Oksanen et al., 2019). Vegans betadisper and permutest functions were used to test for homogeneity of variance. Alpha diversity indices Shannon, Simpson, and observed ASVs were obtained for each of the rumen samples and compared between cohorts using the non-parametric Wilcoxon rank sum test. Alpha and beta diversity analysis was conducted at the ASV level for both bacterial and archaeal populations.
To profile the bacterial community populations, taxa were agglomerated to higher taxonomic ranks (i.e., phylum to genus) due to poor classification at the species level and counts were transformed to relative abundances. Archaeal populations were assessed at the genus and ASV level. Differential relative abundance analysis was conducted from phylum to genus level for bacteria populations and conducted at the genus to ASV level for archaea populations. For lower taxonomic ranks (i.e., genus and ASV) analysis was only conducted on taxa had a relative abundance greater than 0.1% and were prevalent at least 30% of samples. The Wilcoxon rank sum test was implemented to test for differences in relative abundance of taxa between the cohorts, and Benjamini-Hochberg (B-H) was used to correct for multiple testing. Spearman’s correlation analysis was also performed to test for associations between relative abundance of taxa and production traits of feed efficiency (FCR and ADG).
STAMP (v.2.1.3) (Parks et al., 2014) was used to conduct principal component and statistical analysis following functional prediction using CowPI (Wilkinson et al., 2018). The relative abundance of reads mapped to each functional process was compared between cohorts using Whites non-parametric t-test with B-H correction for multiple testing.
Results
Animal Production Traits Differed Across the Divergent Feed Efficiency Cohorts
A Wilcoxon rank sum test was performed to test the null hypothesis that production traits; FCR, ADG, average daily intake (ADI), TWG, did not differ between the two cohorts. For the four production traits significant differences were found in their medians (p < 0.05), confirming that production traits were statistically different between feed efficiency cohorts (Table 1; Claffey et al., 2018).
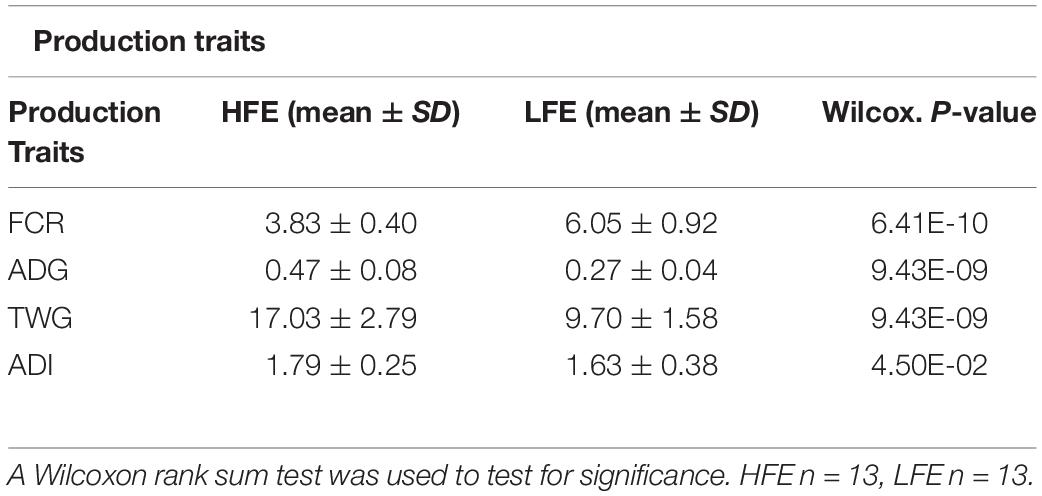
Table 1. Production traits related to feed efficiency (FCR, ADG, ADI, TWG) analyzed per feed efficiency cohort.
Over 1600 Unique ASVs Identified in Both Rumen Fractions
Following data processing, quality filtering and chimera removal, and a total of 6,326,753 amplicon reads remained for analysis (solid phase = 3,061,130, liquid phase = 3,265,623). The average number of reads per sample in the liquid rumen phase was 125,600, and 117,735 in the solid rumen phase. 1691 uniquely identified ASVs were obtained from the reads in both rumen fractions. After prevalence filtering and removal of unclassified ASVs at the phylum level 560 and 513 ASV’s mapped to the kingdom bacteria, while 12 and 11 ASV’s mapped to the kingdom archaea for liquid and solid rumen fractions, respectively. Initial exploratory analysis using PCoA ordination based on weighted UniFrac distances detected two samples from the LFE cohort as outliers (Animal ID: 10707 and 10835) (Supplementary Figure S1). Further investigation revealed that in both fractions the genus Prevotella 1 had a relative abundance of approximately 70%. The samples from both animals were considered highly biased and removed prior to downstream analysis.
Effect of Rumen Fraction and FCR on Microbial Community Composition and Diversity
Ruminal fraction (i.e., liquid and solid) had no effect on microbial profiles (P < 0.05). Similar microbial composition, diversity and relative abundances were observed between the two fractions (Supplementary Figure S2). PCoA analysis on bacteria community composition showed considerable overlap between HFE and LFE samples, based on weighted (liquid, P = 0.28, R2 = 0.05, PermDisp = 0.91; solid, P = 0.48, R2 = 0.03, PermDisp = 0.71) (Figure 1) and unweighted (liquid, P = 0.10, R2 = 0.06, PermDisp = 0.37; solid, P = 0.15, R2 = 0.06, PermDisp = 0.69) UniFrac distances (Supplementary Figure S3). Alpha diversity indicators; Shannon, Simpson, and observed ASVs were not significant between HFE and LFE cohorts for either rumen fraction (P > 0.05), although LFE cohort exhibited greater diversity (Figure 2). For archaea populations greater variation in community composition was observed in the LFE cohort and found to be significantly divergent from the HFE cohort in the liquid rumen fraction based on weighted UniFrac distances (liquid, P = 0.01, R2 = 0.18, PermDisp = 0.01; solid, P = 0.12, R2 = 0.08, PermDisp = 0.10) (Figure 1). Shannon and Simpson indices were increased in the LFE liquid fraction (P > 0.05) and observed ASV was increased in the LFE solid fraction (P > 0.05) when compared to the HFE cohort (Figure 2).
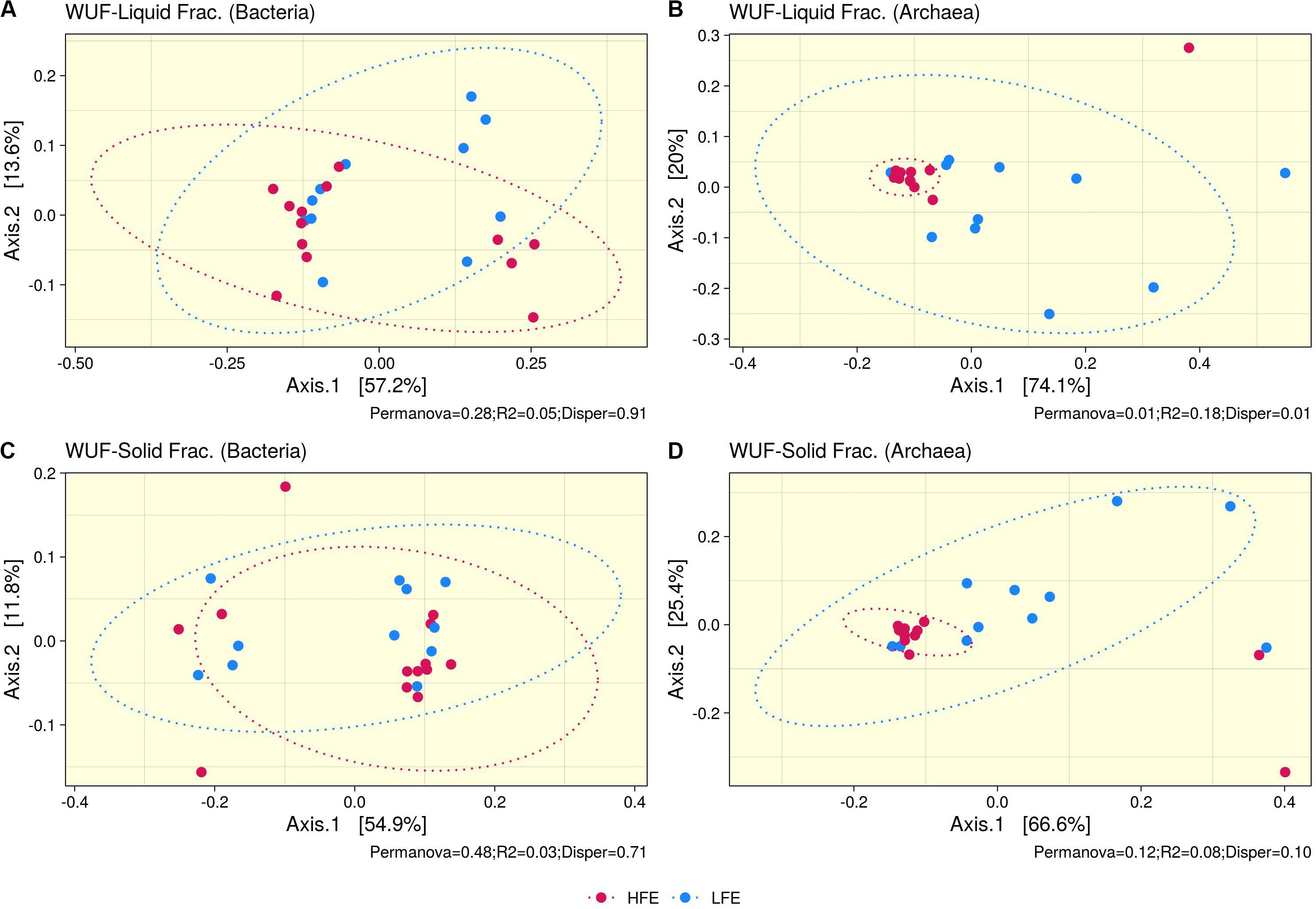
Figure 1. Beta diversity analysis. PCoA ordination plots based on weighted UniFrac distances for bacteria (A,C) and archaea (B,D) populations, for liquid (A,B) and solid (C,D) rumen fractions. Permanova P-value (Permanova), R2, and homogeneity of dispersion analysis (Disper) is provided for each analysis. Dots represent the different microbial samples and colors represent different feed efficient cohorts, HFE (Dark Pink) and LFE (Blue). HFE n = 13, LFE n = 11.
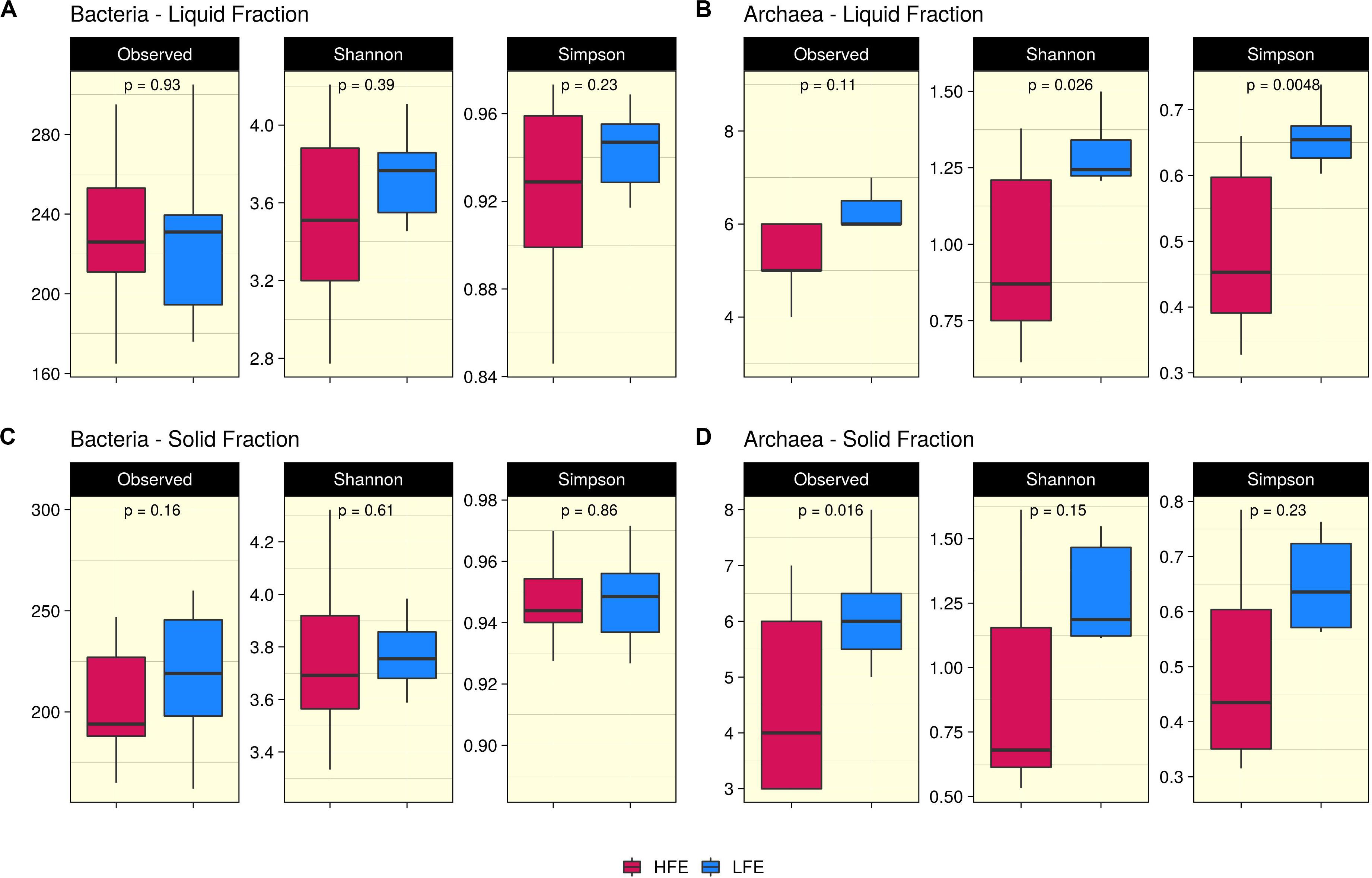
Figure 2. Alpha diversity analysis. Boxplots representing variations in alpha diversity in the rumen liquid (A,B) and solid (C,D) rumen fractions between high (Dark Pink) and low (Blue) feed efficiency cohorts. Alpha diversity metrics include Shannon, Simpson, and observed ASVs for both bacteria (A,C) and archaea (B,D) populations. HFE n = 13, LFE n = 11.
Significant Effects of FCR on Microbial Abundance
After filtering a total of 13 bacterial phyla were identified in both rumen fractions, however FCR phenotype did not affect their relative abundance. Firmicutes and Bacteroidetes constituted the most abundant phyla, respectively. Together they represented 77% and 83% of the mean relative abundance in the HFE cohort, and 82% and 83% of the mean relative abundance in the LFE cohort, for liquid and solid rumen fractions, respectively (Table 2). The Firmicutes and Bacteroidetes ratio (F:B) was not affected by FCR phenotype (P > 0.1) in either the liquid or solid rumen fractions. Proteobacteria and Actinobacteria were the next most abundant phyla, respectively. The mean relative abundance of Fibrobacter was increased in both HFE and LFE solid rumen fractions compared with liquid fractions, while also exhibiting a greater mean relative abundance in the LFE cohort compared the HFE cohort (P > 0.05) (Table 2).
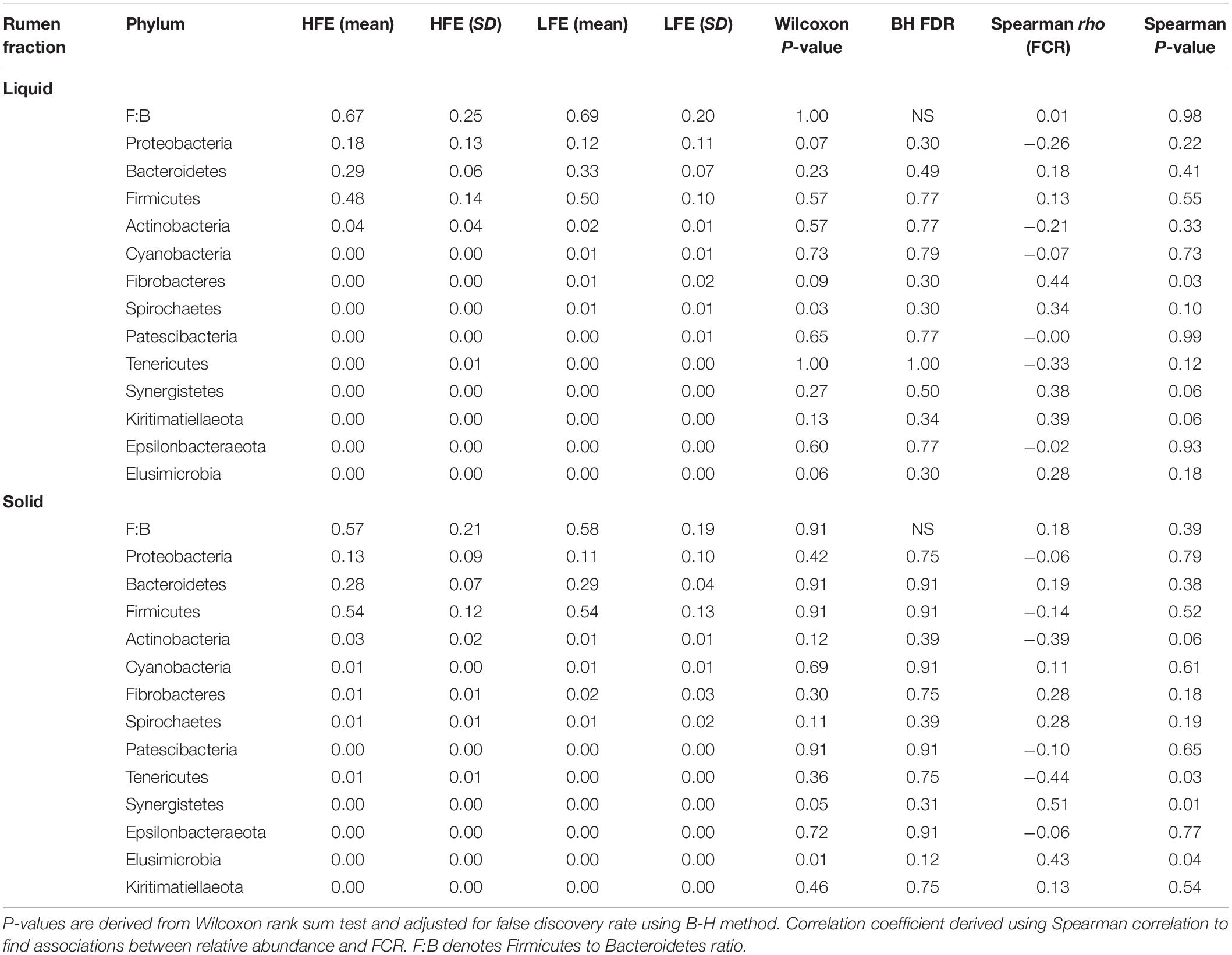
Table 2. Mean relative abundance and standard deviation of bacterial phyla in rumen liquid phase for both HFE and LFE cohorts.
A total of 104 and 99 bacterial genera were identified in the liquid and solid rumen fraction, respectively. The genus Prevotella 1 was the only bacterial taxa differentially abundant, increased in the LFE liquid fraction (FDR = 0.02) (Figure 3). The most dominant genera in both fractions were Prevotella 7, Succinivibrionaceae UCG-001 and Lachnospiraceae NK3A20 group (Figure 4) and their abundance did not differ between feed efficient cohorts (P > 0.05). Genera were predominantly enriched to families Lachnospiraceae (liquid = 24.0%; solid = 24.0%), Ruminococcaceae (liquid = 16.3%; solid = 17.0%), Veillonellaceae (liquid = 8.7%; solid = 9.0%), Erysipelotrichaceae (liquid = 8.7%; solid = 9.0%), and Prevotellaceae (liquid = 8.7%; solid = 9.0%).
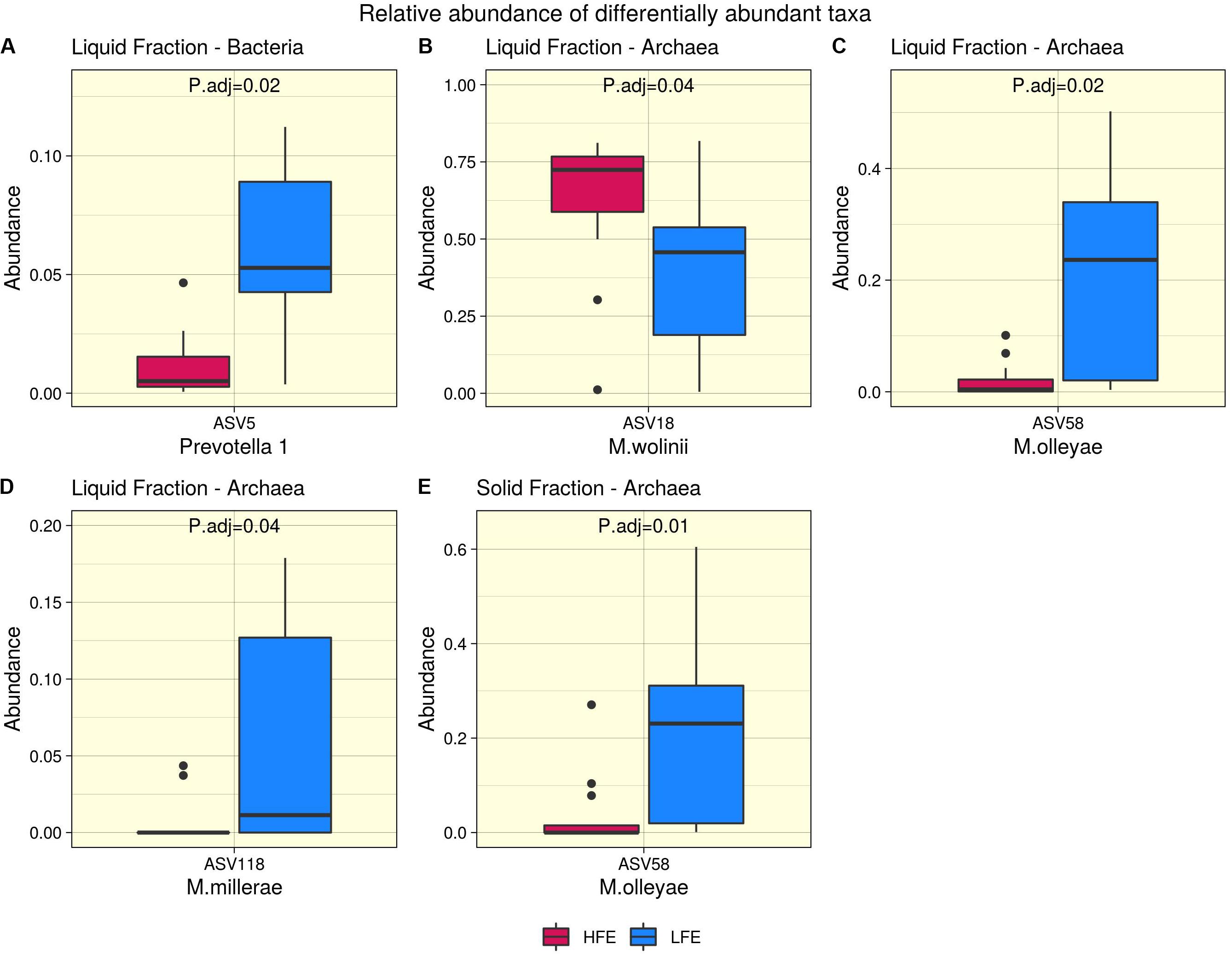
Figure 3. Differential abundance analysis. Bacteria (A) and archaea (B–E) taxa found to be differentially abundant between high (Dark Pink) and low (Blue) feed efficiency cohorts for liquid (A–D) and solid (E) rumen fractions. HFE n = 13, LFE n = 11.
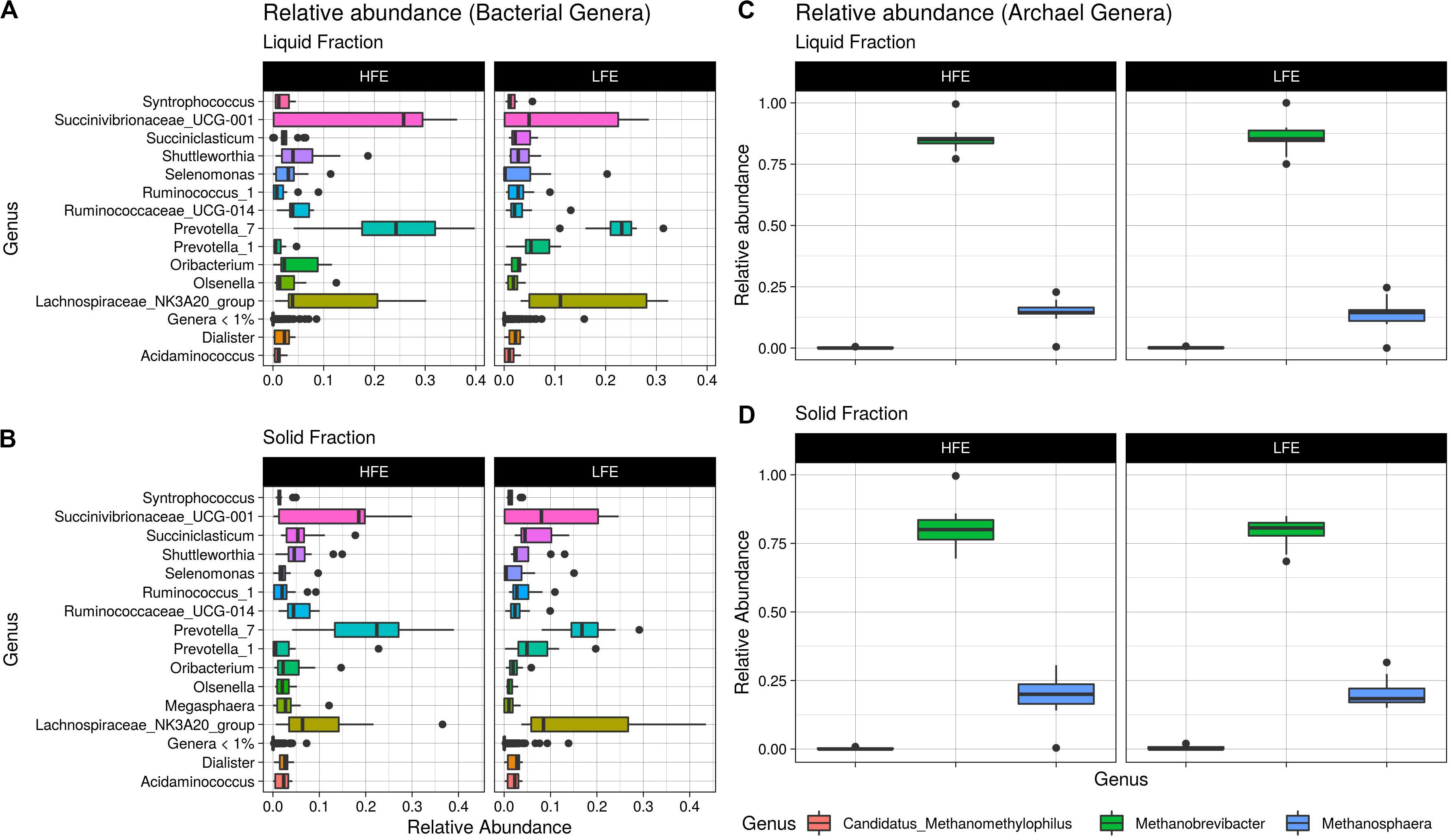
Figure 4. Relative abundance boxplots. Boxplots representing the variations in relative abundance of dominant bacterial genera (>1% relative abundance) (A,B) and archaea genera (C,D) in the rumen of HFE and LFE cohorts for liquid (A,C) and solid (B,D) rumen fractions. HFE n = 13, LFE n = 11.
After profiling of the archaeal population, three genera were identified. Methanobrevibacter was the most dominant, followed by Methanosphaera and Candidatus Methanomethylophilus, respectively, and their abundances were not affected by the FCR phenotype (P > 0.05). At the ASV level, 11 taxa were identified from the liquid rumen fraction and 10 from the solid rumen fraction. Three archaeal ASVs showed difference in relative abundance between the divergent FCR cohorts. In the liquid rumen fraction ASV58 and ASV118, identified to closely match Methanobrevibacter olleyae (98.8%) and Methanobrevibacter millerae (99.2%), respectively, were increased in the LFE cohort. Whereas ASV18, identified as Methanobrevibacter wolinii (100%) was increased in the HFE cohort. In the solid rumen fraction M. olleyae was increased in the LFE cohort (Figure 3).
Significant Associations of Different Bacterial Taxa With FCR and ADG
Spearman’s correlation analysis was performed to identify putative bacterial drivers of feed efficiency. At the genus level, relationships were only explored for genera that were prevalent in greater than 30% of samples and had minimum relative abundance of 0.1%. At the genus level, in the rumen liquid phase the relative abundance of Ruminococcaceae UCG-014 (rho = −0.51) exhibited the strongest negative correlation with FCR (P < 0.05), while Prevotella 1 (rho = 0.56), Coprococcus 1 (rho = 0.44), Ruminococcus 1 (rho = 0.41), and Fibrobacter (rho = 0.43) exhibited the strongest positive correlation with FCR (P < 0.05). Only Bifidobacterium (rho = 0.41) exhibited a significant positive association with ADG (P < 0.05), while Prevotella 1 (rho = −0.72), Pseudoramibacter (rho = −0.56), Coprococcus 1 (rho = −0.51), Ruminococcus 1 (rho = −0.50), Ruminococcus 2 (rho = −0.43), Acetitomaculum (rho = −0.45), Rikenellaceae RC9 gut group (rho = −0.42), Fibrobacter (rho = −0.61), and Treponema 2 (rho = −0.57) exhibited significant negative associations with ADG (P < 0.05) (Table 3).

Table 3. Spearman’s rank correlation showing dominant bacterial genera (>0.1%) that had a significant relationship with either FCR and/or ADG in the liquid rumen phase.
In the solid rumen phase, Ruminococcaceae UCG-014 (rho = −0.55) and Olsenella (rho = −0.40) exhibited a significant negative association with FCR (P < 0.05), while Pyramidobacter (rho = 0.53), Pseudoramibacter (rho = 0.42), Ruminococcus 1 (rho = 0.43), Acetitomaculum (rho = −0.42), Prevotella 1 (rho = 0.39), and Coprococcus 1 (rho = 0.42) exhibited significant positive associations with FCR (P < 0.05). Bifidobacterium (rho = 0.44) and Megasphaera (rho = 0.43) were significantly positively associated with ADG (P < 0.05), while Prevotella 1 (rho = −0.56), Coprococcus 1 (rho = −0.41), Ruminococcus 1 (rho = −0.53), and Acetitomaculum (rho = −0.41), Roseburia (rho = −0.45), Pseudoramibacter (rho = −0.45), Fibrobacter (rho = −0.51), Pyramidobacter (rho = −0.52), and Treponema 2 (rho = −0.48) were among those showing significant negative associations with ADG (P < 0.05) (Table 3).
At the genus level, Fibrobacter (liquid, rho = 0.44), Synergistetes (liquid, rho = 0.038; solid, rho = 0.51), and Elusimicrobia (solid, rho = 0.43) exhibited a significant positive relationship with FCR (P < 0.05), whereas Tenericutes (solid, rho = −0.44) showed a significant negative relationship with FCR (P < 0.05) (Table 2).
Functional Potential and Microbial Processes Similar Between Low and High Feed Efficient Cohorts
Principal component analysis, which captured over 75% variation with the first 2 principal components, indicated no separation in functional potential between feed efficient cohorts (Supplementary Figures S4, S5). No significant differences in metabolic processes were observed between cohorts for both liquid and solid rumen fractions (P.adj > 0.1).
Discussion
The current study examined the effect of the FCR phenotype on ruminal bacteria and archaeal populations obtained from the liquid and solid rumen fractions of TxSB ram lambs offered a high concentrate diet ad libitum. Rumen liquid and solid fractions are widely used for microbiome research (De Mulder et al., 2016; Ji et al., 2017) and for animal feed efficiency analyses (McGovern et al., 2018). Both are differentiated ecological niches, which can contribute to feed efficiency in different ways. The solid rumen fraction is largely composed of plant and grain biomass and selects for various adherent cellulolytic and saccharolytic microbial species that play a significant role in the breakdown of feed within the rumen (De Mulder et al., 2016). While the liquid rumen fraction is considered to contribute less to the metabolic activity of rumen, it does provide readily available nutrients for free living microbes and facilitates their movement to newly consumed feed (De Mulder et al., 2016). Our analysis reveals a similar microbial community composition, diversity and relative abundance profiles between the liquid and solid rumen fractions (Supplementary Figure S2). A range of studies have also reported comparable findings in microbial diversity and community composition between liquid and solid rumen fractions in both cattle and sheep (Schären et al., 2017; McGovern et al., 2018; Li et al., 2020). The high degree of similarity observed between the fractions may be attributed to the method used to separate the fractions (McGovern et al., 2018). Alternatively, it may also reflect the frequent interchange of microbes between the liquid and solid fractions (De Mulder et al., 2016; Schären et al., 2017). Ultimately, due to the large degree of homogeneity observed between the two rumen fractions it is difficult to determine whether either plays a distinctive role toward feed efficiency in the current study.
In the rumen, methane production is considered beneficial to the host as it regulates the partial pressure of hydrogen facilitating microbial growth and promoting digestion within the rumen (Wallace et al., 2015; Lan and Yang, 2019). However, the production of methane is known to result in a loss of dietary energy to the host, of circa 2–12% depending on the diet (Johnson and Johnson, 1995), thereby impacting on the production performance of the animal. Several studies have linked higher methane emission to feed inefficiency in ruminants (Nkrumah et al., 2006; Zhou et al., 2010; Fitzsimons et al., 2013). The abundance of methanogenic archaea has also been correlated with higher levels of methane emissions (Wallace et al., 2015) and poorer feed efficiency (Zhou et al., 2010). As a result, numerous approaches have been developed to target rumen archaea to reduce methane emissions and improve animal production, including vaccines and small molecule enzyme inhibitors (Matthews et al., 2019). Although numerous studies in ruminants have reported no correlation between the overall abundance of methanogens and methane emissions, they have shown positive correlations between methane production and compositional changes within the archaea community (Danielsson et al., 2012; Shi et al., 2014; Tapio et al., 2017). In particular, increased abundance of taxa assigned to the Methanobrevibacter SGMT clade (i.e., Methanobrevibacter gottschalkii, M. millerae, and Methanobrevibacter smithii) is strongly correlated with methane emissions compared to those within the RO clade (i.e., Methanobrevibacter ruminantium, M. olleyae, M. wolinii) (Tapio et al., 2017). Members of the SGMT clade harbor 2 methyl coenzyme M reductase isozymes McrI and McrII, enabling them to utilize hydrogen more efficiently than those within the RO clade, which solely express McrI (Tapio et al., 2017). In line with such studies, our results show no major shifts in the relative abundance of archaea taxa at the genus level or higher taxonomic ranks between the feed efficient cohorts. However, compositional changes were observed at the ASV level. LFE animals exhibited greater variation in community composition (based on weighted UniFrac distances) (Figure 1) and increased diversity (as calculated by Shannon and Simpson and observed ASVs) (Figure 2) compared to their HFE counterparts. The relative abundance of M. millerae (SGMT clade) and M. olleyae (RO clade) was increased in the LFE liquid fraction, while M. wolinii (RO clade) was increased in the HFE liquid fraction. M. olleyae was also increased in the LFE solid fraction (Figure 3). Compositional changes within the Methanobrevibacter genus between divergent cohorts may partially explain the observed differences in feed conversion and animal production in our study.
Bacteria are the most diverse microbial domain found within the rumen and are capable of extracting energy from a wide variety of dietary substrates, including fiber, starch, sugars, and protein (Tapio et al., 2017). Due to the dependence of the host on bacterial fermentation it can be considered that the rumen bacterial population plays a critical role in the feed efficiency of the animal. Indeed, previous studies in both cattle and sheep have reported significant associations between the feed efficiency of the host and rumen bacterial populations (Jewell et al., 2015; Shabat et al., 2016; Ellison et al., 2017). In our study, no significant differences in bacterial alpha diversity between HFE and LFE lambs were observed (Figure 2). This is consistent with a number of studies in cattle (Myer et al., 2015; McGovern et al., 2018). Although, differences in alpha diversity were not significant in the present study, the HFE cohort exhibited a less diverse bacterial community than their LFE counterparts. This finding is in agreement with a larger study in cattle that reported lower bacterial diversity associated with higher feed efficiency (Shabat et al., 2016). Furthermore, we found no major shifts in community composition and relative abundance of taxa between feed efficient cohorts in either liquid or solid rumen fractions. Weighted UniFrac distances was unable to differentiate bacterial community composition between HFE and LFE cohorts with only a small percentage of the variation explained by the FCR phenotype (Figure 1). This finding was supported by our differential relative abundance analysis, which identified the genus Prevotella 1 as the only bacterial taxa differentially abundant between the two feed efficient cohorts, increased in the LFE liquid fraction (Figure 3). While we detected no major differences in the relative abundance of taxa, we have identified several taxa exhibiting significant correlations of relative abundance with FCR and/or ADG (Table 3).
Prevotella 1, Fibrobacter, Ruminococcus 1, Coprococcus, Pseudoramibacter, and Pyramidobacter all exhibited significant positive associations with FCR and negative associations with ADG (Table 3). Prevotella species are known to ferment a wide variety of substrates including starches, peptides, proteins and hemicellulose (Matsui et al., 1998; Xie et al., 2019), which contribute to the feed efficiency of the host. Indeed, different Prevotella species have been associated with both higher and lower feed efficiency in cattle and sheep (Ellison et al., 2017; Brooke et al., 2019; Delgado et al., 2019). For instance, Ellison et al. (2017) reported that the abundance of Prevotella ruminicola increased significantly for L-RFI lambs when fed a concentrate based diet and decreased in L-RFI lambs when fed a forage-based diet. The opposite was reported for Prevotella bryantii (Ellison et al., 2017). This indicates a dietary effect on the abundance of Prevotella species, which is likely attributed to the metabolic divergence observed within the genus Prevotella (Matsui et al., 2000). Matsui et al. (2000) reported differential production of polysaccharide degrading enzymes and growth rates among Prevotella species when grown on various growth substrates in vitro. A recent study identified Prevotella 1 as the most dominant genus in both the liquid and solid rumen fractions of lambs (Li et al., 2020). However, Li et al. (2020) offered lambs a higher ratio of forage to concentrate (45:55), which contrasts with the 95% concentrate diet provided to lambs in the current study. This suggests that Prevotella 1 may require fibrous tissue or substrates released following fiber degradation for optimal growth, where the association with poorer feed efficiency in the current study may be driven by differences in the quantity of dietary intake observed between the two feed efficient cohorts (Table 1).
Members of the genera Fibrobacter and Ruminococcus are predominant fiber-digesting bacteria in the rumen, specifically the species Fibrobacter succinogenes, Ruminococcus flavefaciens, and Ruminococcus albus (Koike and Kobayashi, 2001). All of these three species largely depend on cellulose for growth and energy, although R. albus can utilize more efficiently a variety of other substrates produced following breakdown of plant fibers (La Reau and Suen, 2018). In contrast to our findings (Table 3), McGovern et al. (2018) found negative associations between RFI and the relative abundance of Fibrobacter and Ruminococcus OTUs. This may have resulted from variations in the ratio of dietary concentrates to forage fed to the animals during the two studies, in addition to differences in how feed efficiency was measured (FCR compared to RFI). Indeed, the abundance of these cellulolytic bacteria in the rumen has previously been shown to diminish with reductions in the ratio of dietary forages (Carberry et al., 2012; Henderson et al., 2015; Zhang et al., 2017). This suggests that the cellulolytic activities of Fibrobacter and Ruminococcus may become redundant in high concentrate-based diets, and that their increased abundance in the LFE cohort may confer inefficiency in energy extraction from feed.
Species within the genus Coprococcus metabolize carbohydrates for growth and energy, producing predominantly butyrate and acetate as fermentation end products (Whitman, 2015). In contrast to our findings (Table 3), previous studies in cattle have shown positive associations between Coprococcus and host feed efficiency (Jewell et al., 2015; McGovern et al., 2018). This may be explained by differences in animal models or diets used in the studies. Indeed, Kim et al. (2014) profiled the fecal microbiota from steers fed three different diets (high grain, moderate grain, and silage/forage) and found three distinct OTU’s belonging to Coprococcus that differed significantly between the treatment groups (Kim et al., 2014).
Associations between Pseudoramibacter and ruminant feed efficiency (Table 3) are not well reported in the literature. Pseudoramibacter is a member of the Eubacteriaceae family and can utilize carbohydrates for energy (Deusch et al., 2017), while producing fermentation end products butyrate, acetate, formate, and hydrogen (Deusch et al., 2017; Palakawong Na Ayudthaya et al., 2018).
The phylum Synergistetes was negatively correlated with feed efficiency in the solid rumen fraction (Table 2). This association was primarily driven by the genus Pyramidobacter. Members of the Pyramidobacter genus are asaccharolytic, non-motile and produce acetic acid and isovaleric acid (Downes et al., 2009). The abundance of Pyramidobacter has previously been associated with low RFI in Simmental bulls (McGovern et al., 2018) and isolated from higher methane emitting steers (Wallace et al., 2015). McGovern et al. (2018) found the relative abundance of Pyramidobacter and Fibrobacter to be positively correlated. This may indicate that Pyramidobacter relies on co-dependence with Fibrobacter for nutrient utilization following fiber degradation in the rumen.
The genera Roseburia, Treponema 2, Mogibacterium, Rikenellaceae RC9 gut group, Acetitomaculum and Ruminococcus 2 all exhibited significant negative associations with ADG in either or both rumen fractions in the current study, but showed no significant associations with FCR (Table 3). Roseburia utilizes carbohydrates for growth and its abundance is known to increase with greater ratios of dietary concentrates (McCann et al., 2014; Zhang et al., 2018). Butyrate is the primary VFA produced by Roseburia and its production is largely dependent on the availability of acetate (Duncan et al., 2002). Supporting the findings of our study, Li et al. (2019) identified a greater abundance of Roseburia in feed inefficient Kinsella composite hybrid steers fed a high-energy diet (Li et al., 2019). In contrast, Ellison et al. (2017) reported a greater abundance of Roseburia in feed efficient lambs fed a concentrate diet. Other studies in this area have not reported any association between Roseburia and feed efficiency (Carberry et al., 2012; Jewell et al., 2015; McGovern et al., 2018). Given the saccharolytic activity of Roseburia, it is unclear why the genus correlated negatively with feed efficiency in lambs fed a high concentrate diet. One suggestion is that a greater availability of acetate may be present in the rumen of lower feed efficient lambs. Roseburia has been reported to be a net utilizer of acetate during growth (Duncan et al., 2002). The correlation between the abundance of Acetitomaculum and lower feed efficiency may support this possibility. The genus Acetitomaculum is capable of utilizing hydrogen to reduce carbon dioxide for the formation of acetate in a process known as acetogenesis (Greening and Leedle, 1989; Le Van et al., 1998).
Mogibacterium has previously been identified in the rumen of both sheep (Mi et al., 2018) and cattle (Myer et al., 2015; Freetly et al., 2020). Mogibacterium belongs to the order Clostridiales from the phylum Firmicutes and is described as incapable of breaking down carbohydrates for energy (Whitman, 2015). Similar to the finding presented in the current study (Table 3), a recent study found Mogibacterium to be enriched in the jejunum of lower ADG steers fed a high-energy diet (Freetly et al., 2020). In addition, Mogibacterium has also been associated with higher methane-emitting steers (Wallace et al., 2015).
Members of the genus Treponema have been associated with pathological conditions including digital dermatitis (Wilson-Welder et al., 2015), yaws disease and syphilis (Newbrook et al., 2017), while others are part of the normal microflora in the GI tract of animals (Newbrook et al., 2017). Treponema species such as Treponema bryantii and Treponema succinifaciens ferment carbohydrates (Cwyk and Canale-Parola, 1979; Stanton, 1984), but are also known to be involved in the breakdown of fiber (Xie et al., 2018). In a recent study (Ellison et al., 2019) identified a greater abundance of the species Treponema maltophilum in the rumen of feed efficient lambs fed a forage-based diet. McGovern et al. (2018) also identified two Treponema OTU’s positively correlating with feed efficiency in Simmental bulls. This contrasts with the findings of our study, which found Treponema associating significantly with poorer ADG and tending toward feed inefficiency in lambs fed a high concentrate diet (Table 3).
Rikenellaceae RC9 gut group has previously been identified in the rumen of domesticated livestock (Petri et al., 2013; Ishaq et al., 2019; Ren et al., 2019). Petri et al. (2013) observed a reduction in the abundance of Rikenellaceae in heifers treated with a diet comprising mixed forage and concentrate to those fed forage alone (Petri et al., 2013). Additionally, the abundance of unclassified Rikenellaceae was found to decrease in goats fed with high grain diets compared to hay based diets (Liu et al., 2015). The finding from these studies may indicate a preference for forage-based diets for the Rikenellaceae RC9 gut group and could explain its correlation with reduced ADG in our study where animals were fed a concentrate diet.
Ruminococcaceae UCG-014 and Olsenella also exhibited significant negative associations with FCR and were not found to be significantly associated with ADG (Table 3). The uncultivable genus Ruminococcaceae UCG-014 (family Ruminococcaceae) showed the strongest negative associations with FCR (Table 3). Ruminococcaceae is considered a dominant family within the rumen of livestock (Creevey et al., 2014; Henderson et al., 2015) and generally more abundant in animals fed forage-based diets (Henderson et al., 2015). Within the Ruminococcaceae family certain members are known cellulolytic fermenters, such as R. albus and R. flavefaciens (Perea et al., 2017). However, other members are non-cellulolytic and actively ferment various forms of polysaccharides (Hook et al., 2011; Petri et al., 2012; La Reau and Suen, 2018). Indeed, Ellison et al. (2017) found particular Ruminococcus species to be more enriched in sheep fed a concentrate diet, compared to those fed a forage-based diet and vice versa. Additionally, in a study conducted on dairy cows the abundance of Ruminococcaceae NK4A214 was increased in a high grain diet (Pan et al., 2017). It is unclear why Ruminococcaceae UCG-014 shows significant associations with FCR in our study. One possibility is that it may indicate that Ruminococcaceae UCG-014 is associated with improved carbohydrate metabolism in the rumen.
Olsenella ferment starch and glycogen substrates and produce lactic, acetic, and formic acid (Göker et al., 2010). Members of the genus Olsenella have been identified in oral cavities and GIT of humans and animals (Kraatz et al., 2011; Ellison et al., 2017; Kubasova et al., 2018; Elolimy et al., 2020). In line with our findings (Table 3), Elolimy et al. (2020) reported a greater abundance of Olsenella in hindguts of feed efficient Holstein heifer calves (Elolimy et al., 2020). However, other studies have reported a greater abundance of Olsenella in the rumen microbiota of low feed efficient lambs when fed a concentrate diet (Ellison et al., 2017) and in the fecal microbiota of low feed efficient piglets (Kubasova et al., 2018).
The genera Megasphaera and Bifidobacteria exhibited significant positive associations with ADG but no significant associations with FCR (Table 3). Megasphaera has previously been associated with high feed efficiency in Holstein dairy cattle (Shabat et al., 2016), and also exhibited greater abundance in lower methane emitting sheep (Kamke et al., 2016). Megasphaera is known to metabolize lactate within the rumen, which it utilizes for production of important VFAs for animal growth (e.g., acetate, propionate, and butyrate) (Chen et al., 2019), indicating its association with ADG in ruminants. Removal of lactate is important mechanism in regulating pH levels within the rumen, preventing rumen lactic acidosis and maintaining rumen health and function (Hernández et al., 2014; Chen et al., 2019).
Bifidobacterium species are known to produce a broad spectrum of carbohydrate modifying enzymes, which facilitate the metabolism of a wide variety of dietary carbohydrates. This enables members of the Bifidobacterium genus to efficiently adapt, extract energy and contribute to the feed efficiency of the host when offered a high-energy diet (Pokusaeva et al., 2011). Indeed, Ellison et al. (2017) found Bifidobacterium to be significantly more abundant in the rumen of feed efficient lambs when fed a concentrate diet. Furthermore, a study conducted by Abe et al. (1995) showed that oral administration of Bifidobacterium improved daily weight gain and FCR of young calves (Abe et al., 1995). Our findings are consistent with both of these studies, suggesting that Bifidobacterium may contribute significantly in extracting energy from carbohydrate based diets.
The relationship of feed efficiency with the rumen microbiota composition and abundance has not been extensively researched in sheep. In one study, Ellison et al. (2017) examined the effect of feed efficiency, diet and breed on the rumen microbial populations from the rumen of sheep. That study differed from our study in several key aspects. Firstly, in the study by Ellison et al. (2017) RFI was used to distinguish feed efficient cohorts, which contrasts with the FCR measurement used in our study. Secondly, Ellison et al. (2017) used wether lambs spanning three different breeds of sheep – Rambouillet, Hampshire, and Suffolk, whereas TXSB ram lambs were used in our study. Thirdly, Ellison et al. (2017) fed animals with both concentrate and forage based diets. Although animals were not fed with a forage-based diet in this study, the composition of concentrates in the diets used in both studies varied. A further study carried out by Perea et al. (2017) also examined the effect of RFI on the microbial populations in the rumen, as well as multiple other sites from the digestive tract, of wether lambs fed a forage-based diet. Differences in diet, breeds, sex, measures of feed efficiency and analytical methodologies used between the studies may have contributed to the differences in the findings between the studies.
The dominance of Firmicutes and Bacteroidetes in the rumen of ruminants is widely reported throughout the literature (McGovern et al., 2018; Paz et al., 2018; Trabi et al., 2019; Liu et al., 2019). Consistent with those studies Firmicutes and Bacteroidetes were identified as the most abundant phyla in the rumen of ram lambs fed a high concentrate diet. Prevotella 7, Succinivibrionaceae UCG-001, and Lachnospiraceae NK3A20 group were found to be the three most abundant genera in our study (Figure 3). This finding is also in line with a large global study set out to characterize the core rumen microbiota in small and large ruminants (Henderson et al., 2015). Henderson et al. (2015) identified Prevotella and unclassified Lachnospiraceae among the most abundant bacterial groups in the rumen. In addition, Prevotella and unclassified Succinivibrionaceae were found to be the most abundant bacterial groups in ruminants when fed a concentrate diet (Henderson et al., 2015). The degree of similarity between the taxa of sheep and cattle indicate that sheep models may serve as a useful and robust model for rumen microbiome research, as they are less expensive and more manageable than cattle (Delano et al., 2002).
To limit global warming to below 1.5°C above pre-industrial levels by 2050, in line with the Paris Agreement (United Nations/Framework Convention on Climate Change [UNFCCC], 2015), as well as feeding a growing population, there is an urgent requirement to increase production while reducing methane emissions intensity from livestock (Islam and Lee, 2019). In 2018, the IPCC’s Special Report on Global Warming of 1.5°C detailed reduction targets for global biogenic methane to between 24 and 47% of 2010 levels by 2050 (IPCC, 2018). Reducing methane emissions from livestock production represents a promising mitigation strategy that can be achieved by sustainable intensification of livestock production and/or reduced livestock product consumption (Herrero et al., 2016). In the context of sustainable intensification of ruminant livestock production, our findings indicate that genetically selecting for feed efficient animals can be a potential route for improving production and reducing feeding costs, while achieving methane emissions reductions in ruminant production systems.
In summary, our study investigated the rumen bacterial and archaeal populations in the rumen of ram lambs divergent for the FCR phenotype, which were fed a concentrate diet. Although lambs were found to be significantly divergent for feed efficiency, no major shifts in the rumen bacterial composition were observed. Correlation analysis suggests that the differences in feed efficiency may be attributed to a number of specific bacterial taxa within the rumen rather than the community as a whole. On the other hand, archaea community composition, diversity and the relative abundance of a Methanobrevibacter ASV differed between HFE and LFE cohorts, which may partially explain a loss of energy in the LFE cohort. Whilst no significant difference in predicted metabolic processes was detected using CowPI, a limitation of this technique is that it infers microbial metabolic pathways based of 16S rDNA data and does not measure the microbial transcriptome or proteome (Wilkinson et al., 2018). Our study was also somewhat limited by poor classification at the species level. The aforementioned limitations can potentially be overcome by the use of both shotgun metagenomics for greater resolution of taxonomic classification (Quince et al., 2017; Brumfield et al., 2020) and metatranscriptomics uncovering the functional potential of the rumen microbiome (Shakya et al., 2019). Our current study focused exclusively on interrogating bacterial and archaeal populations from the rumen. A more comprehensive understanding of the contribution of the sheep rumen microbiota to animal feed efficiency would ideally investigate all major microbial populations within the rumen, including protozoa and fungi (Newbold et al., 2015; Tapio et al., 2017).
Data Availability Statement
The datasets presented in this study can be found in online repositories. The names of the repository/repositories and accession number(s) can be found below: https://www.ncbi.nlm.nih.gov/, PRJNA643304.
Ethics Statement
The animal study was reviewed and approved by the Ireland’s Health Products Regulatory Authority (HPRA) in accordance with the European Union (EU) protection of animals used for scientific purposes regulations 2012 (S.I. No 543 of 2012). Written informed consent was obtained from the owners for the participation of their animals in this study.
Author Contributions
MD and NC designed and conceptualized the animal model and collected rumen samples for analysis. SW, CS, and SM designed and conceptualized the microbiome study, carried out detailed interpretation of the data, and drafted the manuscript. TO’R and SW performed the DNA extraction and amplicon library generation. SW and PS coordinated the sequencing runs. SM conducted all of the bioinformatics and statistical analyses. PS provided assistance with data analysis. SM wrote the first draft of the manuscript, which was revised by SW and CS. All authors contributed to the final manuscript revision, read and approved the final draft of the manuscript.
Funding
SM was funded by a Teagasc Walsh Fellowship grant (Ref: 2015218). The animal study was funded by the DAFM (RAMLAMB – 11/SF/310). CS acknowledges funding support from the Science Foundation Ireland.
Conflict of Interest
The authors declare that the research was conducted in the absence of any commercial or financial relationships that could be construed as a potential conflict of interest.
Acknowledgments
The authors acknowledge the contribution of Gerard Burke for his feeding and excellent care of animals.
Supplementary Material
The Supplementary Material for this article can be found online at: https://www.frontiersin.org/articles/10.3389/fmicb.2020.01981/full#supplementary-material
FIGURE S1 | Beta diversity analysis. NMDS ordination plot based weighted and unweighted UniFrac distances. Dots represent the different samples. Colors represent different feed efficient cohorts, HFE (Pink) and LFE (Blue). The plots show outlying samples (10707, 10835), which were removed prior to downstream analysis. HFE n = 13, LFE n = 13. (A) Weighted Unifrac distances for liquid fraction (Bacteria). (B) Unweighted Unifrac distances for liquid fraction (Bacteria). (C) Weighted Unifrac distances for solid fraction (Bacteria). (D) Unweighted Unifrac distances for solid fraction (Bacteria).
FIGURE S2 | Analysis of ruminal fractions. PCoA ordination plots with weighted (A) and unweighted (B) UniFrac distances. Alpha diversity analysis; Shannon simpson and observed ASV for solid and liquid rumen fractions (C). Colors represent different feed efficient cohorts, HFE (Dark Pink) and LFE (Blue). Stacked bar chart representing the relative abundance of 10 most abundant genera (D). HFE n = 13, LFE n = 11.
FIGURE S3 | Beta diversity analysis. PCoA ordination plots based on weighted UniFrac distances for bacteria (A,C) and archaea (B,D) populations, for liquid (A,B) and solid (C,D) rumen fractions. Permanova P-value (Permanova), R2, and homogeneity of dispersion analysis (Disper) is provided for each analysis. Dots represent the different microbial samples and colors represent different feed efficient cohorts, HFE (Dark Pink) and LFE (Blue). HFE n = 13, LFE n = 11.
FIGURE S4 | PCA plot comparing microbiome functional profiles for each sample sampled from the liquid rumen phase. HFE n = 13, LFE n = 11.
FIGURE S5 | PCA plot comparing microbiome functional profiles for each sample sampled from the solid rumen phase. HFE n = 13, LFE n = 11.
References
Abe, F., Ishibashi, N., and Shimamura, S. (1995). Effect of administration of bifidobacteria and lactic acid bacteria to newborn calves and piglets. J. Dairy Sci. 78, 2838–2846. doi: 10.3168/jds.s0022-0302(95)76914-4
Abecia, L., Martínez-Fernandez, G., Waddams, K., Martín-García, A. I., Pinloche, E., Creevey, C. J., et al. (2018). Analysis of the rumen microbiome and metabolome to study the effect of an antimethanogenic treatment applied in early life of kid goats. Front. Microbiol. 9:2227. doi: 10.3389/fmicb.2018.02227
Andrews, S. (2010). FastQC: A Quality Control Tool for High Throughput Sequence Data. Cambridge: Babraham Institute.
Berry, D. P., and Crowley, J. J. (2013). Cell biology symposium: genetics of feed efficiency in dairy and beef cattle. J. Anim. Sci. 91, 1594–1613. doi: 10.2527/jas.2012-5862
Bhatt, R. S., Karim, S. A., Sahoo, A., and Shinde, A. K. (2013). Growth performance of lambs fed diet supplemented with rice bran oil as such or as calcium soap. Asian Aust. J. Anim. Sci. 26, 812–819. doi: 10.5713/ajas.2012.12624
Brooke, C. G., Najafi, N., Dykier, K. C., and Hess, M. (2019). Prevotella copri, a potential indicator for high feed efficiency in western steers. Anim. Sci. J. 90, 696–701. doi: 10.1111/asj.13197
Brumfield, K. D., Huq, A., Colwell, R. R., Olds, J. L., and Leddy, M. B. (2020). Microbial resolution of whole genome shotgun and 16S amplicon metagenomic sequencing using publicly available NEON data. PLoS One 15:e0228899. doi: 10.1371/journal.pone.0228899
Callahan, B. (2018). Silva Taxonomic Training Data Formatted for DADA2 (Silva Version 132). Zenodo doi: 10.5281/zenodo.1172783
Callahan, B. J., Mcmurdie, P. J., and Holmes, S. P. (2017). Exact sequence variants should replace operational taxonomic units in marker-gene data analysis. ISME J. 11, 2639–2643. doi: 10.1038/ismej.2017.119
Cannas, A., Tedeschi, L. O., Atzori, A. S., and Lunesu, M. F. (2019). How can nutrition models increase the production efficiency of sheep and goat operations? Anim. Front. 9, 33–44. doi: 10.1093/af/vfz005
Caporaso, J. G., Lauber, C. L., Walters, W. A., Berg-Lyons, D., Lozupone, C. A., Turnbaugh, P. J., et al. (2011). Global patterns of 16S rRNA diversity at a depth of millions of sequences per sample. Proc. Natl. Acad. Sci. U.S.A. 108:4516. doi: 10.1073/pnas.1000080107
Carberry, C. A., Kenny, D. A., Han, S., Mccabe, M. S., and Waters, S. M. (2012). Effect of phenotypic residual feed intake and dietary forage content on the rumen microbial community of beef cattle. Appl. Environ. Microbiol. 78, 4949–4958. doi: 10.1128/aem.07759-11
Chen, L., Shen, Y., Wang, C., Ding, L., Zhao, F., Wang, M., et al. (2019). Megasphaera elsdenii lactate degradation pattern shifts in rumen acidosis models. Front. Microbiol. 10:162. doi: 10.3389/fmicb.2019.00162
Claffey, N. A., Fahey, A. G., Gkarane, V., Moloney, A. P., Monahan, F. J., and Diskin, M. G. (2018). Effect of breed and castration on production and carcass traits of male lambs following an intensive finishing period. Transl. Anim. Sci. 2, 407–418. doi: 10.1093/tas/txy070
Creevey, C. J., Kelly, W. J., Henderson, G., and Leahy, S. C. (2014). Determining the culturability of the rumen bacterial microbiome. Microb. Biotechnol. 7, 467–479. doi: 10.1111/1751-7915.12141
Cwyk, W. M., and Canale-Parola, E. (1979). Treponema succinifaciens sp. nov., an anaerobic spirochete from the swine intestine. Arch. Microbiol. 122, 231–239. doi: 10.1007/BF00411285
Danielsson, R., Schnürer, A., Arthurson, V., and Bertilsson, J. (2012). Methanogenic population and CH<sub>4</sub> production in swedish dairy cows fed different levels of forage. Appl. Environ. Microbiol. 78:6172.
De Mulder, T., Goossens, K., Peiren, N., Vandaele, L., Haegeman, A., De Tender, C., et al. (2016). Exploring the methanogen and bacterial communities of rumen environments: solid adherent, fluid and epimural. FEMS Microbiol. Ecol. 93:fiw251. doi: 10.1093/femsec/fiw251
Delano, M. L., Mischler, S. A., and Underwood, W. J. (2002). “Chapter 14 – biology and diseases of ruminants: sheep, goats, and cattle,” in Laboratory Animal Medicine (Second Edition), eds J. G. Fox, L. C. Anderson, F. M. Loew, and F. W. Quimby (Burlington: Academic Press), 519–614. doi: 10.1016/b978-012263951-7/50017-x
Delgado, B., Bach, A., Guasch, I., González, C., Elcoso, G., Pryce, J. E., et al. (2019). Whole rumen metagenome sequencing allows classifying and predicting feed efficiency and intake levels in cattle. Sci. Rep. 9:11.
Deusch, S., Camarinha-Silva, A., Conrad, J., Beifuss, U., Rodehutscord, M., and Seifert, J. (2017). A structural and functional elucidation of the rumen microbiome influenced by various diets and microenvironments. Front. Microbiol. 8:1605. doi: 10.3389/fmicb.2017.01605
Downes, J., Vartoukian, S. R., Dewhirst, F. E., Izard, J., Chen, T., Yu, W.-H., et al. (2009). Pyramidobacter piscolens gen. nov., sp. nov., a member of the phylum ‘Synergistetes’ isolated from the human oral cavity. Int. J. Syst. Evol. Microbiol. 59, 972–980. doi: 10.1099/ijs.0.000364-0
Duncan, S. H., Barcenilla, A., Stewart, C. S., Pryde, S. E., and Flint, H. J. (2002). Acetate utilization and butyryl coenzyme A (CoA):acetate-CoA transferase in butyrate-producing bacteria from the human large intestine. Appl. Environ. Microbiol. 68, 5186–5190. doi: 10.1128/aem.68.10.5186-5190.2002
Ellison, M. J., Conant, G. C., Lamberson, W. R., Austin, K. J., Van Kirk, E., Cunningham, H. C., et al. (2019). Predicting residual feed intake status using rumen microbial profiles in ewe lambs1. J. Anim. Sci. 97, 2878–2888. doi: 10.1093/jas/skz170
Ellison, M. J., Conant, G. C., Lamberson, W. R., Cockrum, R. R., Austin, K. J., Rule, D. C., et al. (2017). Diet and feed efficiency status affect rumen microbial profiles of sheep. Small Rumin. Res. 156, 12–19. doi: 10.1016/j.smallrumres.2017.08.009
Elolimy, A., Alharthi, A., Zeineldin, M., Parys, C., and Loor, J. J. (2020). Residual feed intake divergence during the preweaning period is associated with unique hindgut microbiome and metabolome profiles in neonatal Holstein heifer calves. J. Anim. Sci. Biotechnol. 11:13.
Fitzsimons, C., Kenny, D. A., Deighton, M. H., Fahey, A. G., and Mcgee, M. (2013). Methane emissions, body composition, and rumen fermentation traits of beef heifers differing in residual feed intake1. J. Anim. Sci. 91, 5789–5800. doi: 10.2527/jas.2013-6956
Freetly, H. C., Dickey, A., Lindholm-Perry, A. K., Thallman, R. M., Keele, J. W., Foote, A. P., et al. (2020). Digestive tract microbiota of beef cattle that differed in feed efficiency. J. Anim. Sci. 98:skaa008.
Göker, M., Held, B., Lucas, S., Nolan, M., Yasawong, M., Glavina Del Rio, T., et al. (2010). Complete genome sequence of Olsenella uli type strain (VPI D76D-27C). Stand. Genomic Sci. 3, 76–84. doi: 10.4056/sigs.1082860
Greening, R. C., and Leedle, J. A. (1989). Enrichment and isolation of Acetitomaculum ruminis, gen. nov., sp. nov.: acetogenic bacteria from the bovine rumen. Arch. Microbiol. 151, 399–406. doi: 10.1007/bf00416597
Gu, W., Miller, S., and Chiu, C. Y. (2019). Clinical metagenomic next-generation sequencing for pathogen detection. Annu. Rev. Pathol. Mech. Dis. 14, 319–338. doi: 10.1146/annurev-pathmechdis-012418-012751
Henchion, M., Hayes, M., Mullen, A. M., Fenelon, M., and Tiwari, B. (2017). Future protein supply and demand: strategies and factors influencing a sustainable equilibrium. Foods 6:53. doi: 10.3390/foods6070053
Henderson, G., Cox, F., Ganesh, S., Jonker, A., Young, W., Global Rumen Census, C., et al. (2015). Rumen microbial community composition varies with diet and host, but a core microbiome is found across a wide geographical range. Sci. Rep. 5:14567.
Hernández, J., Benedito, J. L., Abuelo, A., and Castillo, C. (2014). Ruminal acidosis in feedlot: from aetiology to prevention. Sci. World J. 2014:702572.
Herrero, M., Henderson, B., Havlík, P., Thornton, P. K., Conant, R. T., Smith, P., et al. (2016). Greenhouse gas mitigation potentials in the livestock sector. Nat. Clim. Change 6, 452–461. doi: 10.1038/nclimate2925
Hook, S. E., Steele, M. A., Northwood, K. S., Dijkstra, J., France, J., Wright, A.-D. G., et al. (2011). Impact of subacute ruminal acidosis (SARA) adaptation and recovery on the density and diversity of bacteria in the rumen of dairy cows. FEMS Microbiol. Ecol. 78, 275–284. doi: 10.1111/j.1574-6941.2011.01154.x
Hunter, M. C., Smith, R. G., Schipanski, M. E., Atwood, L. W., and Mortensen, D. A. (2017). Agriculture in 2050: recalibrating targets for sustainable intensification. Bioscience 67, 386–391. doi: 10.1093/biosci/bix010
Ishaq, S. L., Lachman, M. M., Wenner, B. A., Baeza, A., Butler, M., Gates, E., et al. (2019). Pelleted-hay alfalfa feed increases sheep wether weight gain and rumen bacterial richness over loose-hay alfalfa feed. PLoS One 14:e0215797. doi: 10.1371/journal.pone.0215797
Islam, M., and Lee, S.-S. (2019). Advanced estimation and mitigation strategies: a cumulative approach to enteric methane abatement from ruminants. J. Anim. Sci. Technol. 61, 122–137. doi: 10.5187/jast.2019.61.3.122
Jewell, K. A., Mccormick, C. A., Odt, C. L., Weimer, P. J., and Suen, G. (2015). Ruminal bacterial community composition in dairy cows is dynamic over the course of two lactations and correlates with feed efficiency. Appl. Environ. Microbiol. 81, 4697–4710. doi: 10.1128/aem.00720-15
Ji, S., Zhang, H., Yan, H., Azarfar, A., Shi, H., Alugongo, G., et al. (2017). Comparison of rumen bacteria distribution in original rumen digesta, rumen liquid and solid fractions in lactating Holstein cows. J. Anim. Sci. Biotechnol. 8, 16–16.
Johnson, K. A., and Johnson, D. E. (1995). Methane emissions from cattle. J. Anim. Sci. 73, 2483–2492. doi: 10.2527/1995.7382483x
Johnson, M., Zaretskaya, I., Raytselis, Y., Merezhuk, Y., Mcginnis, S., and Madden, T. L. (2008). NCBI BLAST: a better web interface. Nucleic Acids Res. 36, W5–W9.
Kachiprath, B., Puthumana, J., Gopi, J., Solomon, S., Krishnan, K. P., and Philip, R. (2018). Amplicon sequencing based profiling of bacterial diversity from Krossfjorden, Arctic. Data Brief 21, 2522–2525. doi: 10.1016/j.dib.2018.11.101
Kamke, J., Kittelmann, S., Soni, P., Li, Y., Tavendale, M., Ganesh, S., et al. (2016). Rumen metagenome and metatranscriptome analyses of low methane yield sheep reveals a Sharpea-enriched microbiome characterised by lactic acid formation and utilisation. Microbiome 4, 1–16. doi: 10.1186/s40168-016-0201-2
Kenny, D. A., Fitzsimons, C., Waters, S. M., and Mcgee, M. (2018). Invited review: Improving feed efficiency of beef cattle–the current state of the art and future challenges. Animal 12, 1815–1826. doi: 10.1017/s1751731118000976
Keogh, K., Waters, S. M., Cormican, P., Kelly, A. K., O’shea, E., and Kenny, D. A. (2017). Effect of dietary restriction and subsequent re-alimentation on the transcriptional profile of bovine ruminal epithelium. PLoS One 12:e0194445. doi: 10.1371/journal.pone.0194445
Kim, M., Kim, J., Kuehn, L. A., Bono, J. L., Berry, E. D., Kalchayanand, N., et al. (2014). Investigation of bacterial diversity in the feces of cattle fed different diets1. J. Anim. Sci. 92, 683–694. doi: 10.2527/jas.2013-6841
Koike, S., and Kobayashi, Y. (2001). Development and use of competitive PCR assays for the rumen cellulolytic bacteria: fibrobacter succinogenes, Ruminococcus albus and Ruminococcus flavefaciens. FEMS Microbiol. Lett. 204, 361–366. doi: 10.1111/j.1574-6968.2001.tb10911.x
Kraatz, M., Wallace, R. J., and Svensson, L. (2011). Olsenella umbonata sp. nov., a microaerotolerant anaerobic lactic acid bacterium from the sheep rumen and pig jejunum, and emended descriptions of Olsenella, Olsenella uli and Olsenella profusa. Int. J. Syst. Evol. Microbiol. 61, 795–803. doi: 10.1099/ijs.0.022954-0
Kubasova, T., Davidova-Gerzova, L., Babak, V., Cejkova, D., Montagne, L., Le-Floc’h, N., et al. (2018). Effects of host genetics and environmental conditions on fecal microbiota composition of pigs. PLoS One 13:e0201901. doi: 10.1371/journal.pone.0201901
La Reau, A. J., and Suen, G. (2018). The Ruminococci: key symbionts of the gut ecosystem. J. Microbiol. 56, 199–208. doi: 10.1007/s12275-018-8024-4
Lan, W., and Yang, C. (2019). Ruminal methane production: associated microorganisms and the potential of applying hydrogen-utilizing bacteria for mitigation. Sci. Total Environ. 654, 1270–1283. doi: 10.1016/j.scitotenv.2018.11.180
Le Van, T. D., Robinson, J. A., Ralph, J., Greening, R. C., Smolenski, W. J., Leedle, J. A., et al. (1998). Assessment of reductive acetogenesis with indigenous ruminal bacterium populations and Acetitomaculum ruminis. Appl. Environ. Microbiol. 64, 3429–3436. doi: 10.1128/aem.64.9.3429-3436.1998
Li, F., and Guan, L. L. (2017). Metatranscriptomic profiling reveals linkages between the active rumen microbiome and feed efficiency in beef cattle. Appl. Environ. Microbiol. 83, e00061-17. doi: 10.1128/AEM.00061-17
Li, F., Hitch, T. C. A., Chen, Y., Creevey, C. J., and Guan, L. L. (2019). Comparative metagenomic and metatranscriptomic analyses reveal the breed effect on the rumen microbiome and its associations with feed efficiency in beef cattle. Microbiome 7:6.
Li, Z., Mu, C., Xu, Y., Shen, J., and Zhu, W. (2020). Changes in the solid-, liquid-, and epithelium-associated bacterial communities in the rumen of hu lambs in response to dietary urea supplementation. Front. Microbiol. 11:244. doi: 10.3389/fmicb.2020.00244
Liu, C., Wu, H., Liu, S., Chai, S., Meng, Q., and Zhou, Z. (2019). Dynamic alterations in yak rumen Bacteria community and Metabolome characteristics in response to feed type. Front. Microbiol. 10:1116. doi: 10.3389/fmicb.2019.01116
Liu, J.-H., Bian, G.-R., Zhu, W.-Y., and Mao, S.-Y. (2015). High-grain feeding causes strong shifts in ruminal epithelial bacterial community and expression of Toll-like receptor genes in goats. Front. Microbiol. 6:167. doi: 10.3389/fmicb.2015.00167
Martin, M. (2011). Cutadapt removes adapter sequences from high-throughput sequencing reads. EMBnet. J. 17, 10–12.
Matsui, H., Ogata, K., Tajima, K., Nakamura, M., Nagamine, T., Aminov, R. I., et al. (2000). Phenotypic characterization of polysaccharidases produced by four prevotella type strains. Curr. Microbiol. 41, 45–49. doi: 10.1007/s002840010089
Matsui, H., Ushida, K., Miyazaki, K., and Kojima, Y. (1998). Use of ratio of digested xylan to digested cellulose (X/C) as an index of fiber digestion in plant cell-wall material by ruminal microorganisms. Anim. Feed Sci. Technol. 71, 207–215. doi: 10.1016/s0377-8401(97)00164-8
Matthews, C., Crispie, F., Lewis, E., Reid, M., O’toole, P. W., and Cotter, P. D. (2019). The rumen microbiome: a crucial consideration when optimising milk and meat production and nitrogen utilisation efficiency. Gut Microbes 10, 115–132. doi: 10.1080/19490976.2018.1505176
McCann, J. C., Wickersham, T. A., and Loor, J. J. (2014). High-throughput methods redefine the rumen microbiome and its relationship with nutrition and metabolism. Bioinform. Biol. Insights 8, 109–125.
McGovern, E., Kenny, D. A., Mccabe, M. S., Fitzsimons, C., Mcgee, M., Kelly, A. K., et al. (2018). 16S rRNA sequencing reveals relationship between potent cellulolytic genera and feed efficiency in the rumen of bulls. Front. Microbiol. 9:1842. doi: 10.3389/fmicb.2018.01842
McMurdie, P. J., and Holmes, S. (2013). phyloseq: an R package for reproducible interactive analysis and graphics of microbiome census data. PLoS One 8:e61217. doi: 10.1371/journal.pone.0061217
Mi, L., Yang, B., Hu, X., Luo, Y., Liu, J., Yu, Z., et al. (2018). Comparative analysis of the microbiota between sheep rumen and rabbit cecum provides new insight into their differential methane production. Front. Microbiol. 9:575. doi: 10.3389/fmicb.2018.00575
Myer, P. R., Wells, J. E., Smith, T. P. L., Kuehn, L. A., and Freetly, H. C. (2015). Microbial community profiles of the colon from steers differing in feed efficiency. SpringerPlus 4, 454–454.
Newbold, C. J., De La Fuente, G., Belanche, A., Ramos-Morales, E., and Mcewan, N. R. (2015). The role of ciliate protozoa in the rumen. Front. Microbiol. 6:1313. doi: 10.3389/fmicb.2015.01313
Newbrook, K., Staton, G. J., Clegg, S. R., Birtles, R. J., Carter, S. D., and Evans, N. J. (2017). Treponema ruminis sp. nov., a spirochaete isolated from the bovine rumen. Int. J. Syst. Evol. Microbiol. 67, 1349–1354. doi: 10.1099/ijsem.0.001812
Nkrumah, J. D., Okine, E. K., Mathison, G. W., Schmid, K., Li, C., Basarab, J. A., et al. (2006). Relationships of feedlot feed efficiency, performance, and feeding behavior with metabolic rate, methane production, and energy partitioning in beef cattle1. J. Anim. Sci. 84, 145–153. doi: 10.2527/2006.841145x
Oksanen, J., Blanchet, F. G., Friendly, M., Kindt, R., Legendre, P., and Mcglinn, D. (2019). vegan: Community Ecology Package. R package version 2.5-3. 2018. Available online at: https://cran.r-project.org/web/packages/vegan/vegan.pdf
Palakawong Na Ayudthaya, S., Van De Weijer, A. H. P., Van Gelder, A. H., Stams, A. J. M., De Vos, W. M., and Plugge, C. M. (2018). Organic acid production from potato starch waste fermentation by rumen microbial communities from Dutch and Thai dairy cows. Biotechnol. Biofuels 11:13.
Pan, X., Xue, F., Nan, X., Tang, Z., Wang, K., Beckers, Y., et al. (2017). Illumina sequencing approach to characterize thiamine metabolism related bacteria and the impacts of thiamine supplementation on ruminal microbiota in dairy cows fed high-grain diets. Front. Microbiol. 8:1818. doi: 10.3389/fmicb.2017.01818
Parks, D. H., Tyson, G. W., Hugenholtz, P., and Beiko, R. G. (2014). STAMP: statistical analysis of taxonomic and functional profiles. Bioinformatics 30, 3123–3124. doi: 10.1093/bioinformatics/btu494
Paz, H. A., Hales, K. E., Wells, J. E., Kuehn, L. A., Freetly, H. C., Berry, E. D., et al. (2018). Rumen bacterial community structure impacts feed efficiency in beef cattle. J. Anim. Sci. 96, 1045–1058. doi: 10.1093/jas/skx081
Perea, K., Perz, K., Olivo, S. K., Williams, A., Lachman, M., Ishaq, S. L., et al. (2017). Feed efficiency phenotypes in lambs involve changes in ruminal, colonic, and small-intestine-located microbiota1. J. Anim. Sci. 95, 2585–2592. doi: 10.2527/jas.2016.1222
Petri, R. M., Forster, R. J., Yang, W., Mckinnon, J. J., and Mcallister, T. A. (2012). Characterization of rumen bacterial diversity and fermentation parameters in concentrate fed cattle with and without forage. J. Appl. Microbiol. 112, 1152–1162. doi: 10.1111/j.1365-2672.2012.05295.x
Petri, R. M., Schwaiger, T., Penner, G. B., Beauchemin, K. A., Forster, R. J., Mckinnon, J. J., et al. (2013). Characterization of the core rumen microbiome in cattle during transition from forage to concentrate as well as during and after an acidotic challenge. PLoS One 8:e83424. doi: 10.1371/journal.pone.0083424
Pokusaeva, K., Fitzgerald, G. F., and Van Sinderen, D. (2011). Carbohydrate metabolism in Bifidobacteria. Genes Nutr. 6, 285–306. doi: 10.1007/s12263-010-0206-6
Pulina, G., Milán, M. J., Lavín, M. P., Theodoridis, A., Morin, E., Capote, J., et al. (2018). Invited review: current production trends, farm structures, and economics of the dairy sheep and goat sectors. J. Dairy Sci. 101, 6715–6729. doi: 10.3168/jds.2017-14015
Quince, C., Walker, A. W., Simpson, J. T., Loman, N. J., and Segata, N. (2017). Shotgun metagenomics, from sampling to analysis. Nat. Biotechnol. 35, 833–844. doi: 10.1038/nbt.3935
Ren, H., Su, X., Bai, H., Yang, Y., Wang, H., Dan, Z., et al. (2019). Specific enrichment of microbes and increased ruminal propionate production: the potential mechanism underlying the high energy efficiency of Holstein heifers fed steam-flaked corn. AMB Express 9:209.
Santana, M. H. A., Rossi, P., Almeida, R., and Cucco, D. C. (2012). Feed efficiency and its correlations with carcass traits measured by ultrasound in Nellore bulls. Livestock Sci. 145, 252–257. doi: 10.1016/j.livsci.2012.02.012
Schären, M., Kiri, K., Riede, S., Gardener, M., Meyer, U., Hummel, J., et al. (2017). Alterations in the rumen liquid-, particle- and epithelium-associated microbiota of dairy cows during the transition from a silage- and concentrate-based ration to pasture in spring. Front. Microbiol. 8:744. doi: 10.3389/fmicb.2017.00744
Schliep, K. P. (2011). phangorn: phylogenetic analysis in R. Bioinformatics 27, 592–593. doi: 10.1093/bioinformatics/btq706
Shabat, S. K. B., Sasson, G., Doron-Faigenboim, A., Durman, T., Yaacoby, S., Berg Miller, M. E., et al. (2016). Specific microbiome-dependent mechanisms underlie the energy harvest efficiency of ruminants. ISME J. 10, 2958–2972. doi: 10.1038/ismej.2016.62
Shakya, M., Lo, C.-C., and Chain, P. S. G. (2019). Advances and challenges in metatranscriptomic analysis. Front. Genet. 10:904. doi: 10.3389/fgene.2019.00904
Shi, W., Moon, C. D., Leahy, S. C., Kang, D., Froula, J., Kittelmann, S., et al. (2014). Methane yield phenotypes linked to differential gene expression in the sheep rumen microbiome. Genome Res. 24, 1517–1525. doi: 10.1101/gr.168245.113
Stanton, T. B. (1984). Glucose metabolism of Treponema bryantii, an anaerobic rumen spirochete. Can. J. Microbiol. 30, 526–531. doi: 10.1139/m84-080
Tapio, I., Snelling, T. J., Strozzi, F., and Wallace, R. J. (2017). The ruminal microbiome associated with methane emissions from ruminant livestock. J. Anim. Sci. Biotechnol. 8:7.
Thomas, N. A., Olvera-Ramírez, A. M., Abecia, L., Adam, C. L., Edwards, J. E., Cox, G. F., et al. (2019). Characterisation of the effect of day length, and associated differences in dietary intake, on the gut microbiota of Soay sheep. Arch. Microbiol. 201, 889–896. doi: 10.1007/s00203-019-01652-w
Thornton, P. K. (2010). Livestock production: recent trends, future prospects. Philos. Trans. R. Soc. Lond. Ser. B Biol. Sci. 365, 2853–2867. doi: 10.1098/rstb.2010.0134
Trabi, E. B., Seddik, H.-E., Xie, F., Lin, L., and Mao, S. (2019). Comparison of the rumen bacterial community, rumen fermentation and growth performance of fattening lambs fed low-grain, pelleted or non-pelleted high grain total mixed ration. Anim. Feed Sci. Technol. 253, 1–12. doi: 10.1016/j.anifeedsci.2019.05.001
United Nations/Framework Convention on Climate Change [UNFCCC] (2015). “Adoption of the Paris Agreement”, in Proceedings of the 21st Conference of the Parties, Paris.
Wallace, R. J., Rooke, J. A., Mckain, N., Duthie, C.-A., Hyslop, J. J., Ross, D. W., et al. (2015). The rumen microbial metagenome associated with high methane production in cattle. BMC Genomics 16:839. doi: 10.1186/s12864-015-2032-0
Whitman, W. B. (2015). Bergey’s Manual of Systematics of Archaea and Bacteria. Hoboken, NJ: Wiley Online Library.
Wilkinson, T. J., Huws, S. A., Edwards, J. E., Kingston-Smith, A. H., Siu-Ting, K., Hughes, M., et al. (2018). CowPI: a rumen microbiome focussed version of the PICRUSt functional inference software. Front. Microbiol. 9:1095. doi: 10.3389/fmicb.2018.01095
Willis, C., Desai, D., and Laroche, J. (2019). Influence of 16S rRNA variable region on perceived diversity of marine microbial communities of the Northern North Atlantic. FEMS Microbiol. Lett. 366:fnz152.
Wilson-Welder, J. H., Alt, D. P., and Nally, J. E. (2015). Digital dermatitis in cattle: current bacterial and immunological findings. Animals 5, 1114–1135. doi: 10.3390/ani5040400
Xie, F., Zhang, L., Jin, W., Meng, Z., Cheng, Y., Wang, J., et al. (2019). Methane emission, rumen fermentation, and microbial community response to a nitrooxy compound in low-quality forage fed hu sheep. Curr. Microbiol. 76, 435–441. doi: 10.1007/s00284-019-01644-5
Xie, X., Yang, C., Guan, L. L., Wang, J., Xue, M., and Liu, J. X. (2018). Persistence of cellulolytic bacteria fibrobacter and treponema after short-term corn stover-based dietary intervention reveals the potential to improve rumen fibrolytic function. Front. Microbiol. 9:1363. doi: 10.3389/fmicb.2018.01363
Yu, Z., and Morrison, M. (2004). Comparisons of different hypervariable regions of rrs genes for use in fingerprinting of microbial communities by PCR-denaturing gradient gel electrophoresis. Appl. Environ. Microbiol. 70, 4800–4806. doi: 10.1128/aem.70.8.4800-4806.2004
Zeineldin, M., Barakat, R., Elolimy, A., Salem, A. Z. M., Elghandour, M. M. Y., and Monroy, J. C. (2018). Synergetic action between the rumen microbiota and bovine health. Microb. Pathog. 124, 106–115. doi: 10.1016/j.micpath.2018.08.038
Zhang, J., Shi, H., Wang, Y., Cao, Z., Yang, H., and Li, S. (2018). Effect of limit-fed diets with different forage to concentrate ratios on fecal bacterial and archaeal community composition in holstein heifers. Front. Microbiol. 9:976. doi: 10.3389/fmicb.2018.00976
Zhang, J., Shi, H., Wang, Y., Li, S., Cao, Z., Ji, S., et al. (2017). Effect of dietary forage to concentrate ratios on dynamic profile changes and interactions of ruminal microbiota and metabolites in holstein heifers. Front. Microbiol. 8:2206. doi: 10.3389/fmicb.2017.02206
Zhou, J., He, Z., Yang, Y., Deng, Y., Tringe, S. G., and Alvarez-Cohen, L. (2015). High-throughput metagenomic technologies for complex microbial community analysis: open and closed formats. mBio 6:e02288-14. doi: 10.1128/mBio.02288-14
Zhou, M., Hernandez-Sanabria, E., and Guan, L. L. (2010). Characterization of variation in rumen methanogenic communities under different dietary and host feed efficiency conditions, as determined by PCR-denaturing gradient gel electrophoresis analysis. Appl. Environ. Microbiol. 76, 3776–3786. doi: 10.1128/aem.00010-10
Keywords: Greenhouse Gas, feed efficiency, sheep, ruminant, microbiome, metagenomics, 16S RNA
Citation: McLoughlin S, Spillane C, Claffey N, Smith PE, O’Rourke T, Diskin MG and Waters SM (2020) Rumen Microbiome Composition Is Altered in Sheep Divergent in Feed Efficiency. Front. Microbiol. 11:1981. doi: 10.3389/fmicb.2020.01981
Received: 05 April 2020; Accepted: 27 July 2020;
Published: 25 August 2020.
Edited by:
Zhongtang Yu, The Ohio State University, United StatesReviewed by:
Sang Suk Lee, Sunchon National University, South KoreaMinseok Kim, Chonnam National University, South Korea
Copyright © 2020 McLoughlin, Spillane, Claffey, Smith, O’Rourke, Diskin and Waters. This is an open-access article distributed under the terms of the Creative Commons Attribution License (CC BY). The use, distribution or reproduction in other forums is permitted, provided the original author(s) and the copyright owner(s) are credited and that the original publication in this journal is cited, in accordance with accepted academic practice. No use, distribution or reproduction is permitted which does not comply with these terms.
*Correspondence: Charles Spillane, charles.spillane@nuigalway.ie; Sinéad M. Waters, sinead.waters@teagasc.ie