Exploring the key factors affecting the seasonal variation of phytoplankton in the coastal Yellow Sea
- 1Key Laboratory of Sustainable Development of Marine Fisheries, Ministry of Agriculture and Rural Affairs, Yellow Sea Fisheries Research Institute, Chinese Academy of Fishery Sciences, Qingdao, China
- 2Laboratory for Marine Fisheries Science and Food Production Processes, Pilot National Laboratory for Marine Science and Technology (Qingdao), Qingdao, China
- 3Qingdao Marine Management Support Center, Qingdao, China
Marine phytoplankton play crucial roles in the ocean’s biological pump and have great impacts on global biogeochemical cycles, yet the knowledge of environmental variables controlling their seasonal dynamics needs to be improved further, especially in the coastal ecosystems. In order to explore the determinants affecting the seasonal variation of phytoplankton, here we conducted three surveys during spring, summer and autumn along the coastal Yellow Sea. Among the phytoplankton community, 49 species of diatoms and 9 species of dinoflagellates were observed in spring, 63 species of diatoms and 10 species of dinoflagellates in summer, and 62 species of diatoms and 11 species of dinoflagellates in autumn. These results thus suggested that there were obvious differences in the number of species across the three seasons, of which diatoms were the most diverse group, followed by dinoflagellates. Additionally, diatoms were the most dominant species of the phytoplankton community and varied largely during different seasons. According to the redundancy analysis, the abundance of phytoplankton community was mainly related to water temperature and dissolved inorganic nitrogen (DIN) during the three seasons, indicating that water temperature and DIN could be the key factors controlling the seasonal variability of phytoplankton community along the coastal Yellow Sea. Also, significant correlations were observed between phytoplankton abundance and heavy metals Zn, As, and Hg during the three seasons, suggesting that these metals also had potential influences on the seasonal dynamics of phytoplankton community in the coastal Yellow Sea.
Introduction
Marine phytoplankton account for only 0.2% of primary producer biomass in the biosphere, but contribute about 50% of global net primary production (Longhurst et al., 1995; Field et al., 1998; Agawin et al., 2000) and are important food sources for zooplankton as well as marine fisheries (Schloss et al., 2012; Barton et al., 2013; Johnson et al., 2013). Hence, by using light as a primary source of energy, the growth of marine phytoplankton meets the energy demand of higher trophic levels and supports the normal operation of marine ecosystems. Also, phytoplankton diversity is a best predictor of resource use efficiency in ecosystems and has a stabilizing effect on ecosystem functioning over considerable environmental gradients (Ptacnik et al., 2008; Wei et al., 2020). Therefore, phytoplankton, as the main primary producers in marine ecosystems, play crucial roles in food chains, energy supply, element cycling, ecosystem functioning as well as the global biogeochemical cycles (Irigoien et al., 2004; Barton et al., 2010; Acevedo-Trejos et al., 2014; Litchman et al., 2015). However, marine phytoplankton have the characteristics of short reproductive cycle and sensitivity to environmental changes (Niehoff et al., 2002; Riebesell, 2004; Rost et al., 2008). Generally, changes in environmental conditions can directly affect the phytoplankton community structure and diversity, which can indirectly reflect the combined effects of habitats (Findlay et al., 2006; Staehr and Sand-Jensen, 2006; Thomas et al., 2017; Xiao et al., 2018). For example, Staehr and Sand-Jensen (2006) have demonstrated that seasonal variations in temperature and nutrients can affect the photosynthesis, structure, and diversity of natural phytoplankton community. Thomas et al. (2017) have suggested that the interactions of temperature and nutrients could exacerbate the sensitivity of phytoplankton to ocean warming. Xiao et al. (2018) have documented that warming and eutrophication combine to restructure diatoms and dinoflagellates in the East China Sea. Overall, the characteristics of phytoplankton community structure and diversity are important indicators for evaluating the nutrient level, pollution status, resource status, production potential and stability of marine ecosystems. Assessing their community structure and diversity is, therefore, of great significance for understanding changes in the marine environments as well as the structure and function of marine ecosystems (Guo et al., 2014; Liu et al., 2015; Wei et al., 2020).
The Yellow Sea, which has an average water depth of 44 meters and an area of 40 × 104 km2, is one of the largest shallow continental shelf areas in China seas, and is also a typical coastal sea affected by the terrigenous diluted waters (Teng et al., 2012; Hwang et al., 2014). Along with population growth and rapid economic development, environmental pollution (e.g., eutrophication and toxic metal pollution) in the coastal Yellow Sea has become increasingly prominent in recent years, which also alters the physicochemical and biological characteristics in the region over time (Xu et al., 2011; Xiao et al., 2017; Wang et al., 2018). As such, the community structure and diversity of phytoplankton along the coastal Yellow Sea are inevitably affected by its changing marine environment, especially eutrophication (Lin et al., 2005; Wei et al., 2017; Kong et al., 2018; Wei et al., 2022a; Pujari et al., 2022). For instance, Kong et al. (2018) have observed the simultaneous occurrence of green tide, golden tide and red tide along the coastal Yellow Sea during the spring and summer, as a result of the excessive nutrient loads. Also, Keesing et al. (2011) and Ye et al. (2011) have observed large-scale green tides along the coastal Yellow Sea in spring and summer, respectively. In the past decades, major progress has been made in the study of phytoplankton dynamics in the Yellow Sea, and various theoretical arguments have suggested that their variability and habitat preferences there can be described in terms of environmental variables, such as temperature, nutrient availability, degree of mixing and so forth (Wei et al., 2017; Wang et al., 2018; Sun et al., 2019). Nevertheless, more studies are needed to further increase the knowledge of the processes for marine environments controlling the phytoplankton dynamics (including community structure and diversity), especially in coastal areas where environmental variables are comparatively complex (Guo et al, 2014; Wei et al., 2020).
Previous studies have demonstrated that diatoms are dominant during much of the year along the coastal waters of the Yellow Sea, but their seasonal dynamics have been confirmed to be relevant to physical processes (Liu et al., 2015). Furthermore, Zhang et al. (2016) and Wei et al. (2017) have revealed the community composition of phytoplankton in the coastal Yellow Sea, and suggested that nutrients (especially N and P) are the key environmental factors affecting their community composition and distribution. However, a caveat is that those previous studies regarding the effects of environmental factors on phytoplankton community in the coastal Yellow Sea focused on only one season, i.e., there are few studies which assessed the key environmental factors responsible for affecting the seasonal shift in the community of phytoplankton. In the present study, we conducted 3 cruises in three seasons along the coastal Yellow Sea to explore the seasonal variation of phytoplankton in relation to environmental parameters, and to address the following questions: (i) what are the community structure and abundance of phytoplankton along the coastal Yellow Sea? (ii) what are key determinants for the seasonal dynamics of phytoplankton abundance? (iii) how do the key determinants affect their abundance variance in the study region? We hope that this study could provide a more comprehensive picture of seasonal variability of phytoplankton, especially in highly anthropogenic influenced coastal waters.
2. Materials and methods
2.1. Study area and sampling stations
Three oceanographic cruises in the coastal Yellow Sea, China (35.67°-36.34°N, 120.38°-121.25°E) were conducted in March, August, and October 2021, representing spring, summer, and fall, respectively (Figure 1). A total of 78 samples from 26 stations were investigated, and all the locations of the sampling stations were consistent in each season.
2.2. Sample collection and analysis
2.2.1. Environmental parameters
Seawater samples were collected in the surface layer (~2 m) using 10 L Niskin bottles. The in situ measurements of water temperature, salinity, dissolved oxygen (DO), and pH were determined using a YSI multiparameter water quality meter (ProDSS, USA). Those parameters were calibrated according to the standard method of Wei et al. (2022b). Samples for nutrients were filtered through a 0.45 µm cellulose acetate membrane filter to remove large particles, then immediately frozen at -20°C and analyzed as soon as possible. Nutrient concentrations, including nitrate (NO3–N), nitrite (NO2–N), ammonia (NH4+-N), and phosphate (PO4–P), were determined using a Technicon AA3 Auto-Analyzer (Bran + Luebbe, Norderstedt, Germany) based on the methods described by Crouch and Malmstadt (1967) and Verdouw et al. (1978). The concentration of dissolved inorganic nitrogen (DIN) was calculated as the sum of the concentrations of NO3-, NO2-, and NH4+ (Wei et al., 2017; Wei et al., 2022a).The dissolved inorganic phosphorus (DIP) was generalized as PO4-. Besides, we set a minimum nutrient concentration of 0.001 mg L-1 to avoid detection limit issues. For heavy metal analysis, the Cu, Zn, Pb, and Cd samples (~500 mL) were collected in PE bottles, filtered and acidified with nitric acid solution (HNO3). Differently, the Hg and As samples (~500 mL) were collected in glass bottles and acidified with sulfuric acid (H2SO4). The analytical methods for all heavy metals were described by Zhu et al. (2020) and Wei et al. (2022a).
2.2.2. Biological parameters
Samples for phytoplankton analysis were collected by vertical trawl using a shallow water Type III plankton net (~20 µm mesh size), then fixed with 2% buffered formalin and stored in darkness (Guo et al., 2014; Wei et al., 2017). Thereafter, the preserved samples were concentrated in laboratory using a 100 mL settlement column for 24-48 hours. Phytoplankton taxa (> 5 μm) were then identified and counted under an inverted microscope at 400× and/or 200× magnification (Motic AE2000, China) according to the method described by Utermöhl (1931). Samples (~500 mL) for chlorophyll a (Chl a) extraction and quantification were filtered using a Whatman GF/F filter (25 mm) under low vacuum pressure (<0.04 MPa), and then quickly frozen at -20°C (Guo et al., 2014). The Chl a extraction was carried out in 5 mL 90% acetone (4°C for 24 h in the dark). After removing the filters, the Chl a concentrations were quantified on a Turner designs fluorometer following the fluormetric method of Welschmeyer (1994). All the biological and environmental parameters were measured on water collected from the same Niskin bottles.
2.3. Statistical analysis
The diversity, evenness, and dominance of phytoplankton community along the coastal Yellow Sea were calculated according to the Shannon-Wiener diversity index (H’), the Pielou’s evenness index (J), and the dominance index (Yi), respectively (Fu et al., 2021). In the present study, we took the top five species as the dominant species.
where S represents the total number of phytoplankton species in the collected samples, i represents the species number, Pi represents the relative cell abundance of a species, ni is the cell number of species i, N represents the total number of individual cells in the samples, i is the frequency of occurrence of species i in each sample.
All data are given as values ± standard deviation. Box plots of seasonal changes of environmental parameters, line graphs of heavy metal concentration levels in each season, and bar charts of abundance distribution of dominant species in each season were made by software Origin (v. 2021). Horizontal distributions of environmental parameters, diversity index and evenness index in each season were performed by Ocean Data View (v. 5.6.2). Spatial distributions of phytoplankton dominant species abundance in each season was made by ArcMap (v. 10.7). Redundancy analysis (RDA) correlation analysis was used to assess the relationship between dominant species and environmental parameters (Canoco v. 5).
3. Results
3.1. Environmental parameters
The measured environmental parameters in surface seawater are illustrated in Figure 2, and the horizontal distributions of these environmental parameters in the study area can be found in the Supplementary Materials. The range of water temperature in this study region was between 14.70 and 28.30°C, with the highest in summer and the lowest in spring (Figure 2 and Table 1). The temperature in coastal water was much lower than that of the offshore water in three seasons, which may be driven by the inputs of coastal runoffs. The salinity of this region decreased gradually from spring to autumn (Figure 2), and the salinity was lower in coastal water than offshore water, which may be related to the encroachment of the diluted waters. Similarly, the dissolved oxygen (DO) content (6.21-7.87 mg L-1; Table 1) showed a temporally decreasing trend in the three seasons (Figure 2). The horizontal distribution of DO content was generally higher in the coastal water than the offshore water, but there were low values at stations 4 and 5 in summer, which may be related to the phytoplankton bloom. The pH in this region varied between 7.96 and 8.36, whereas the pH in spring was significantly higher than that in summer and autumn (Table 1). Chl a content in summer was higher than those in spring and autumn (Figure 2), and high values also appeared at stations 4 and 5 in summer, corresponding to the low value of DO content there. The DIN and DIP concentrations increased gradually with the three seasons, ranging 7.95-139.31 and 1.90-24.38 µg L-1, respectively (Figure 2 and Table 1).
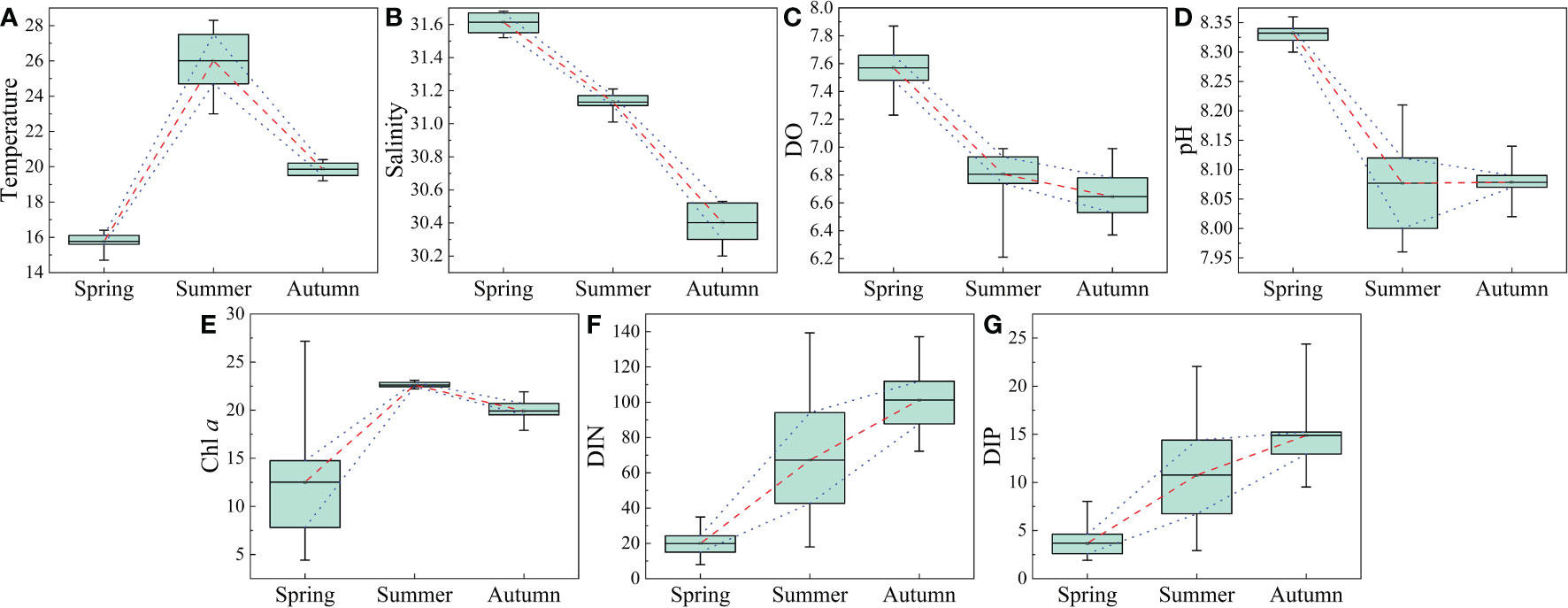
Figure 2 Seasonal variations of various environmental parameters in the coastal Yellow Sea. (A) temperature (°C), (B) salinity, (C) DO (mg L-1), (D) pH, (E) Chl a (µg L-1), (F) DIN (µg L-1), and (G) DIP (µg L-1).
In addition, a seasonal comparison of heavy metal concentrations (i.e., Cu, Zn, Pb, Cd, Hg, As) at each station were conducted, and the results are shown in Figure 3. Generally, the concentration of Cu was the highest in spring and the lowest in summer, with relatively high values at stations 4, 5, and 6 near the shore. For the concentration of Zn, it was higher in autumn, followed by spring and summer. The highest Pb concentration occurred in spring. In terms of regional distribution, the concentration of Pb in the northeastern part of the survey area was higher in summer than in autumn. The Cd concentration fluctuated greatly in each season, but the seasonal difference was not obvious. In terms of regional distribution, however, the concentration in the northeastern part of the survey area during spring was lower than that during summer and autumn. Hg concentration showed no significant fluctuation in autumn, except for stations 3 and 4, and in general, the concentration in summer was higher than those in spring and fall. The concentration of As was extremely low during spring, and the concentration in autumn was higher than that in summer except for the northeastern part of the study area.
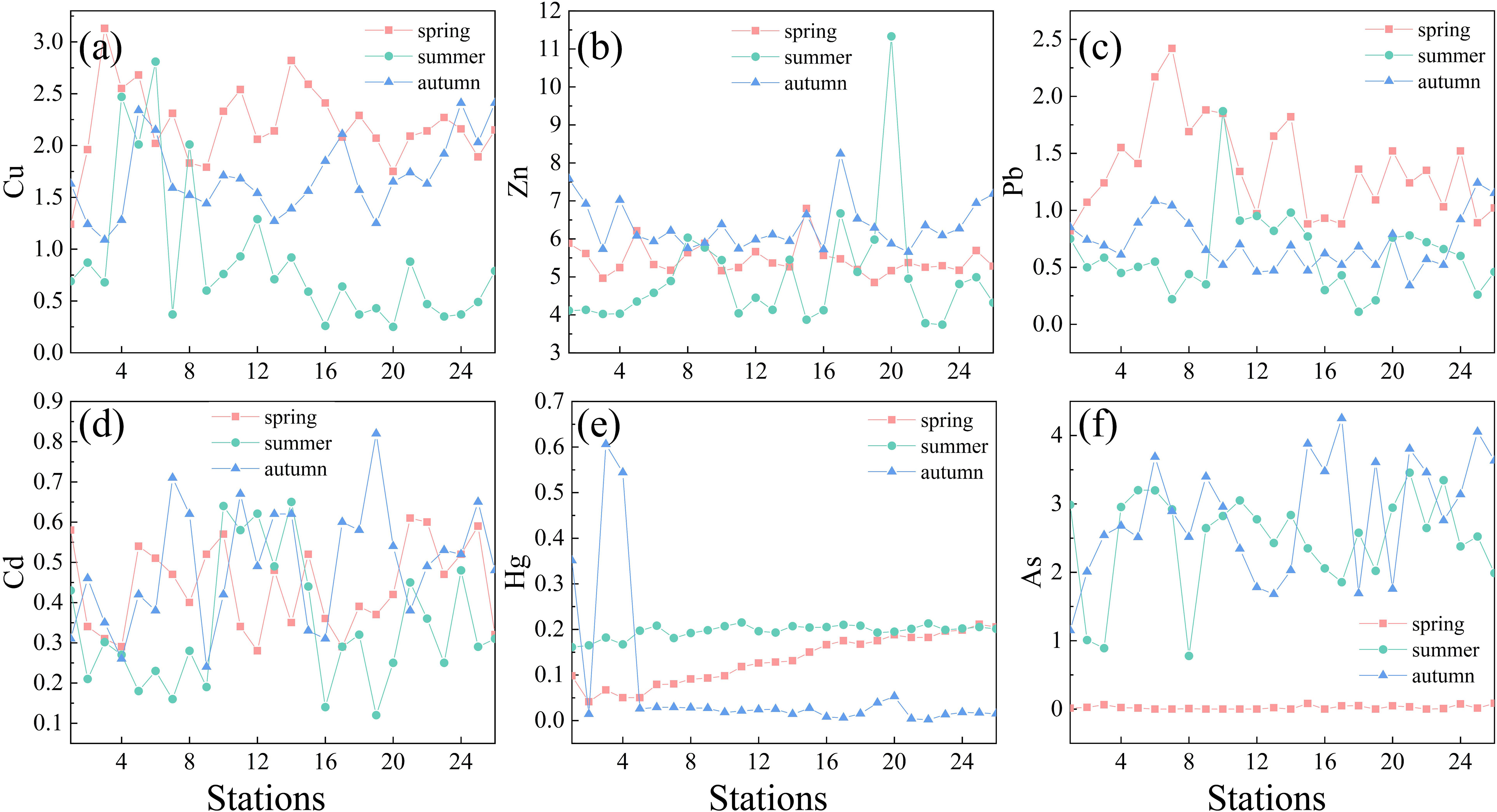
Figure 3 Heavy metal concentrations in different stations during spring, summer and autumn. (A) Cu (µg L-1), (B) Zn (µg L-1), (C) Pb (µg L-1), (D) Cd (µg L-1), (E) Hg (µg L-1), and (F) As (µg L-1).
3.2. Phytoplankton community composition
During the study interval, a total of 115 phytoplankton taxa (> 5 μm) belonging to 3 phyla (i.e., Bacillariophyta, Dinophyta, and Chrysophyta) were identified in the coastal Yellow Sea (Table 2). 95 diatom species were accurately differentiated in the three seasons, accounting for 82.60% of the total phytoplankton taxa. Dinoflagellates were the second diverse group (19 species), representing 16.52% of the total species. Species in other groups were recorded more sparsely (<1%), including 1 taxon in 1 genus of Chrysophyta (i.e., Dictyocha fibula) during the three seasons. These results thus suggested that phytoplankton community were mainly composed of diatoms and dinoflagellates, but dominated primarily by diatoms along the coastal Yellow Sea. The specific classification of phytoplankton taxa in each season is shown in Table 2.
3.3. Phytoplankton diversity, abundance, and distribution
3.3.1. Species diversity
The H’ and J indices were applied to evaluate the complexity of the community structure and, to estimate whether the community is stable, respectively (Equations 1 and 2). In the study region, The H’ and J indices showed a similar distribution pattern in each season along the coastal Yellow Sea (Figure 4). The lower values were mainly observed in the coastal water, while the higher values were primarily found in the offshore water during the three seasons. These results indicated that the coastal region exhibited lower biodiversity and more uneven species distribution patterns in the three seasons. In terms of seasonal variation, the H’ index was highest in summer, followed by autumn and spring, indicating that the biodiversity is highest in summer. However, there was no significant difference in J index among the three seasons.
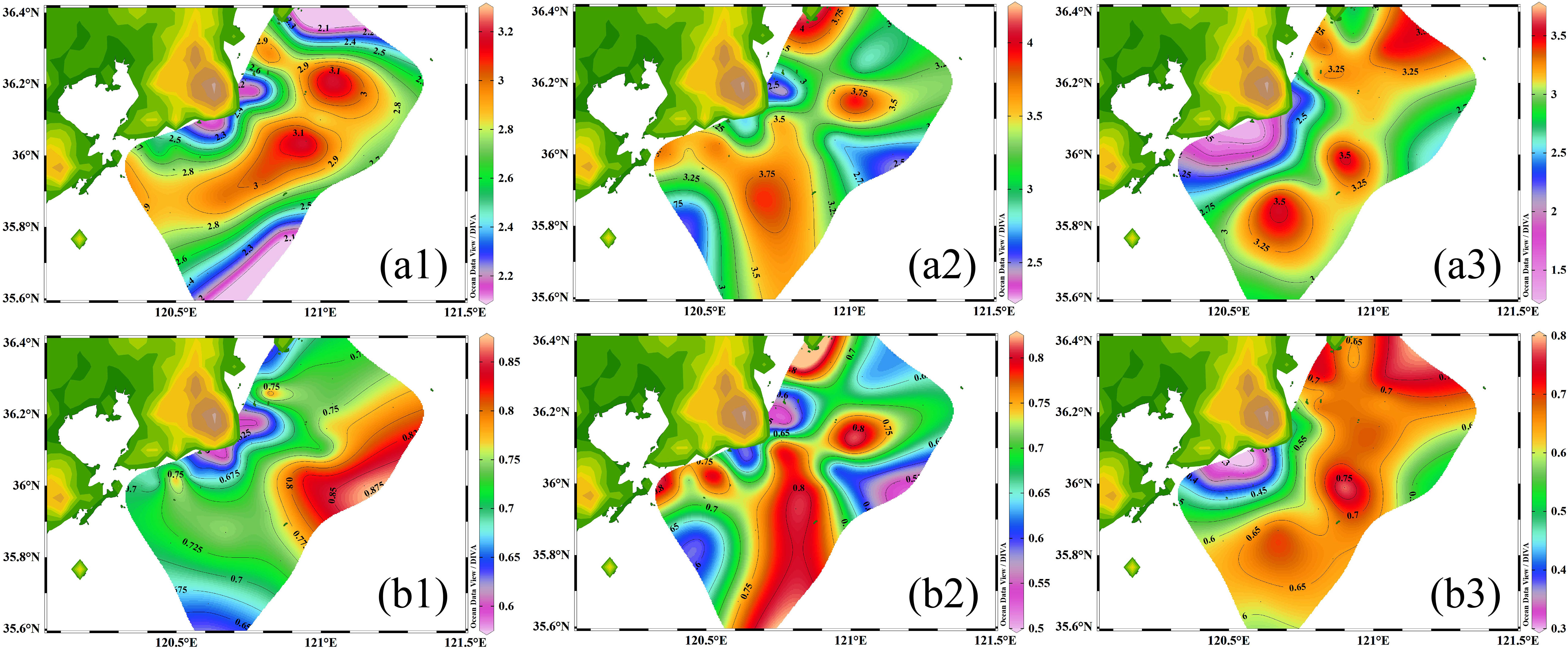
Figure 4 Spatial distributions of Shannon-Wiener diversity index (H’) and Pielou’s evenness index (J) during the three seasons along the coastal Yellow Sea. (a1), (a2) and (a3) represent the H’ index in spring, summer and autumn, respectively. (b1), (b2) and (b3) represent the J index in spring, summer and autumn, respectively.
3.3.2. Dominant species and distribution
The top five dominant species selected in each season in the present study are shown in Table 3. Among the dominant species, most of which were diatoms in the three season, except for Noctiluca scintillans which mainly occurred in the spring. These results further confirmed that the phytoplankton community along the coastal Yellow Sea was dominated by diatoms. As shown in Figure 5, the species richness was the highest in autumn, followed by summer, and the lowest in spring. It is evident that there was an algal bloom phenomenon in the coastal water in autumn. The horizontal distribution of phytoplankton abundance is shown in Figure 6. The high abundance zones of phytoplankton were found in the nearshore water during the three seasons. In addition, in autumn, the diversity of dominant species in nearshore waters declined, and Coscinodiscus granii blooms occurred (Figure 6).
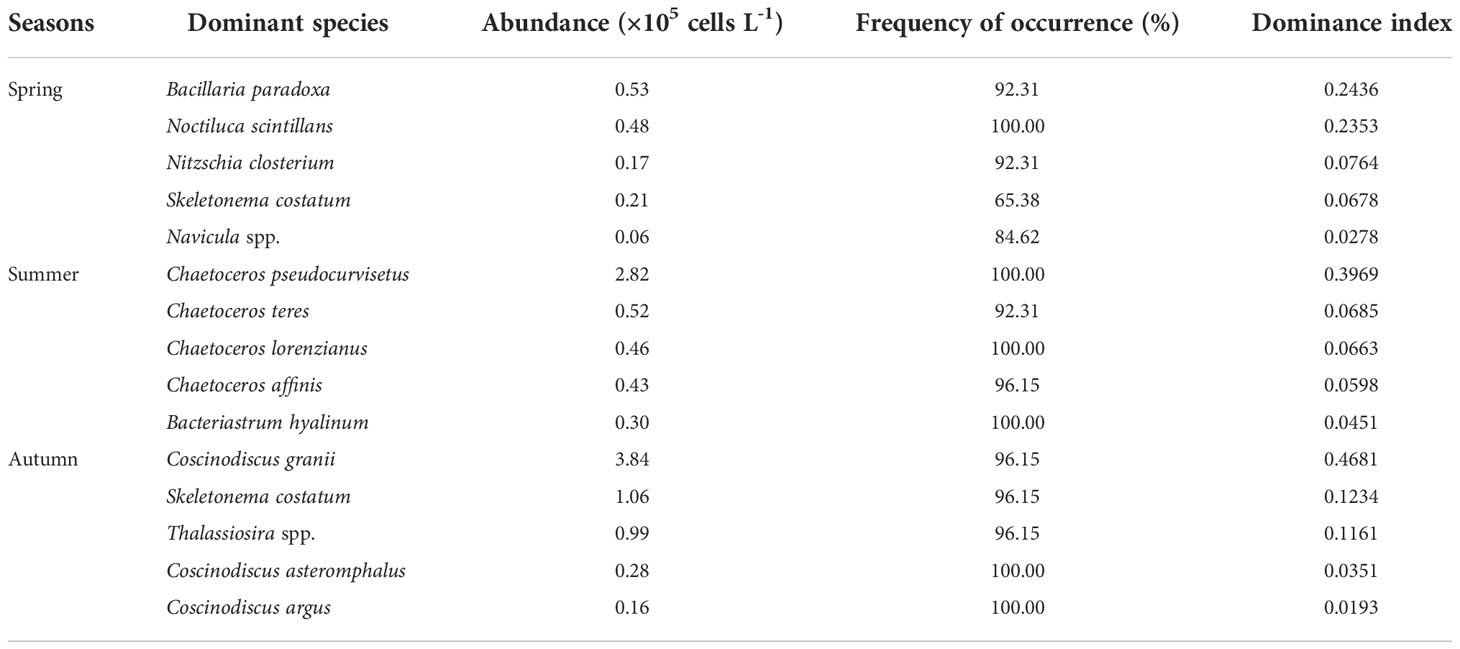
Table 3 The abundance, frequency of occurrence, and dominance index of dominant species among the phytoplankton community in spring, summer, and autumn along the coastal Yellow Sea.
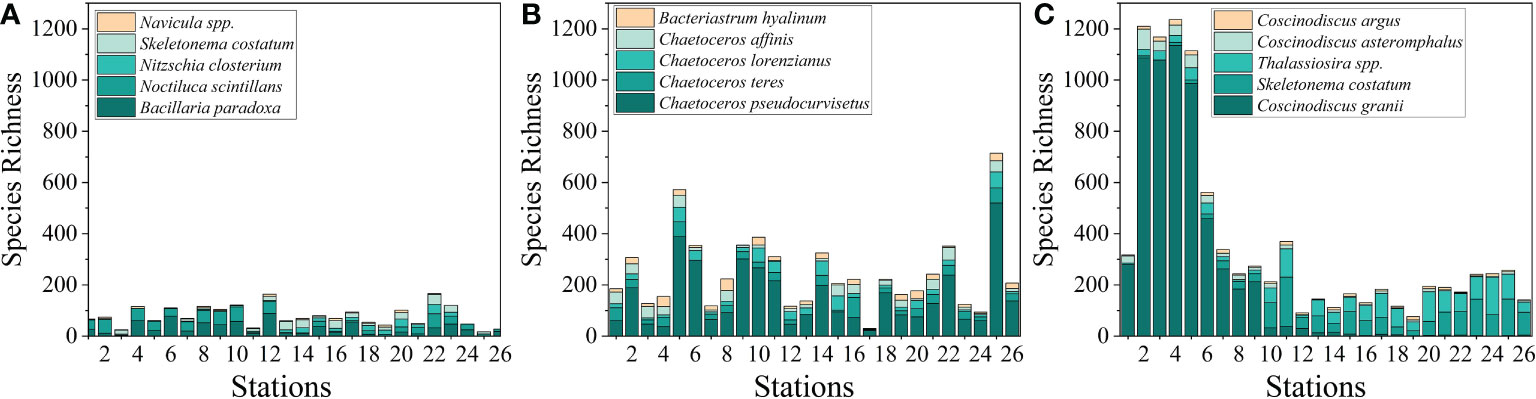
Figure 5 Species richness distribution of dominant species in different stations during the three seasons. (A) spring, (B) summer, and (C) autumn.
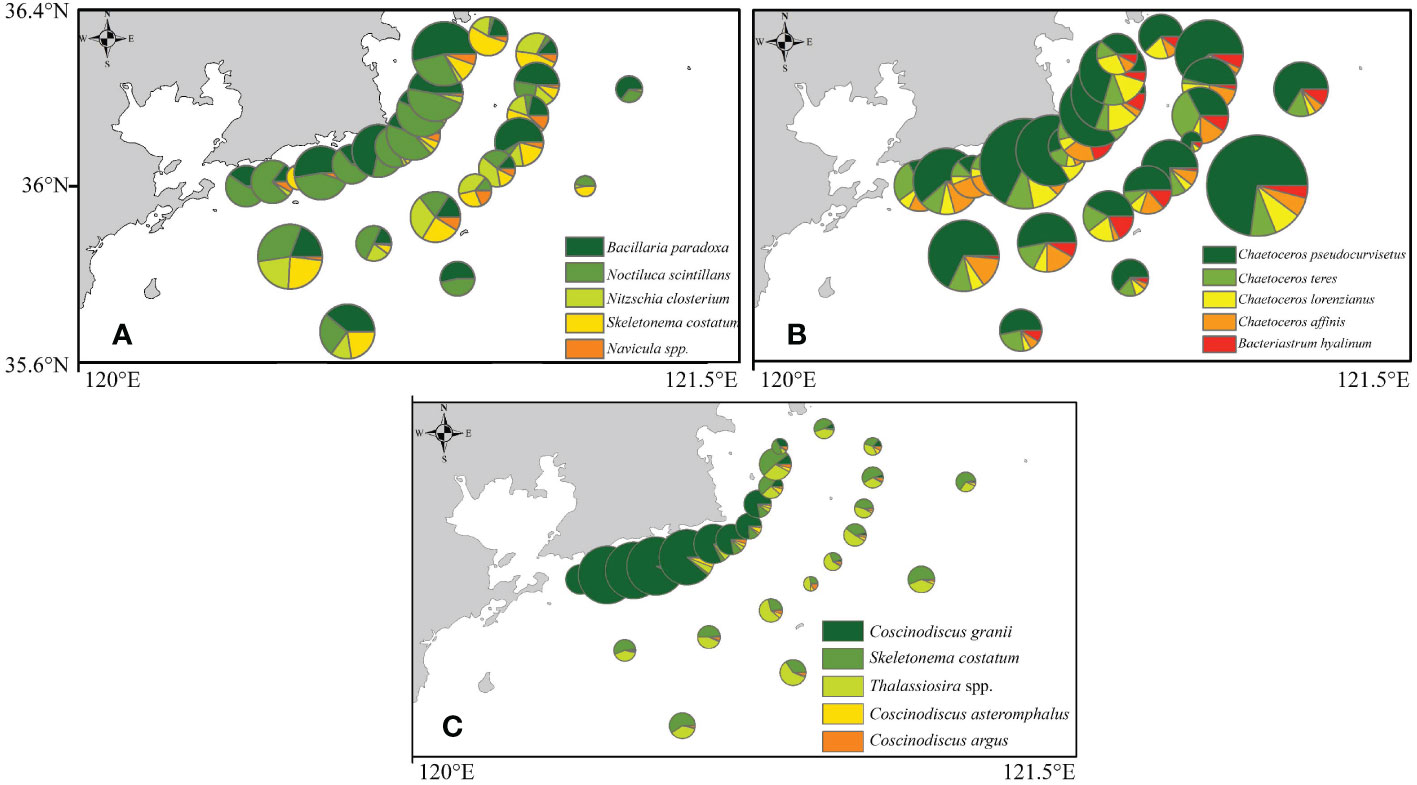
Figure 6 Horizontal distributions for the abundance of dominant species during different seasons along the coastal Yellow Sea. (A) spring, (B) summer and (C) autumn.
3.4. Relationships between abundance and environmental parameters
The Redundancy analysis (RDA) was conducted to identify which environmental variables affected the seasonal variation of phytoplankton and to gain some insights into the determinants controlling the seasonal dynamics of phytoplankton abundance along the coastal Yellow Sea (Figure 7). According to our RDA analysis, the abundance of dominant species in spring was positively correlated with DIN and DO content, but negatively correlated with water temperature (Figure 7A). Similarly, the abundance of dominant species showed a positive correlation with DIN during summer (Figure 7B). In contrast, significant positive correlation was observed between the abundance of most dominant species and water temperature, but the effect of DIN concentration on their abundance was significantly negative in autumn (Figure 7C). These results thus indicated that water temperature and DIN may be the key environmental variables affecting the seasonal dynamics of phytoplankton abundance in the coastal Yellow Sea. In addition, our RDA analysis demonstrated that heavy metals also had significant influences on the seasonal dynamics of phytoplankton abundance along the coastal Yellow Sea (Figures 7D–F). During the three seasons, there were significant correlations between Zn, As, and Hg and phytoplankton abundance, the implication is that Zn, As, and Hg were the potential metals that affected the seasonal dynamics of phytoplankton community. However, this result was in line with a previous study that heavy metals Hg, Zn, and As had more or less diverse consequences for the dynamics of phytoplankton in the Bohai Sea (Wei et al., 2022a), a region that is adjacent to the Yellow Sea and that is also highly affected by anthropogenic activities (Wang et al., 2018; Wei et al., 2022b).
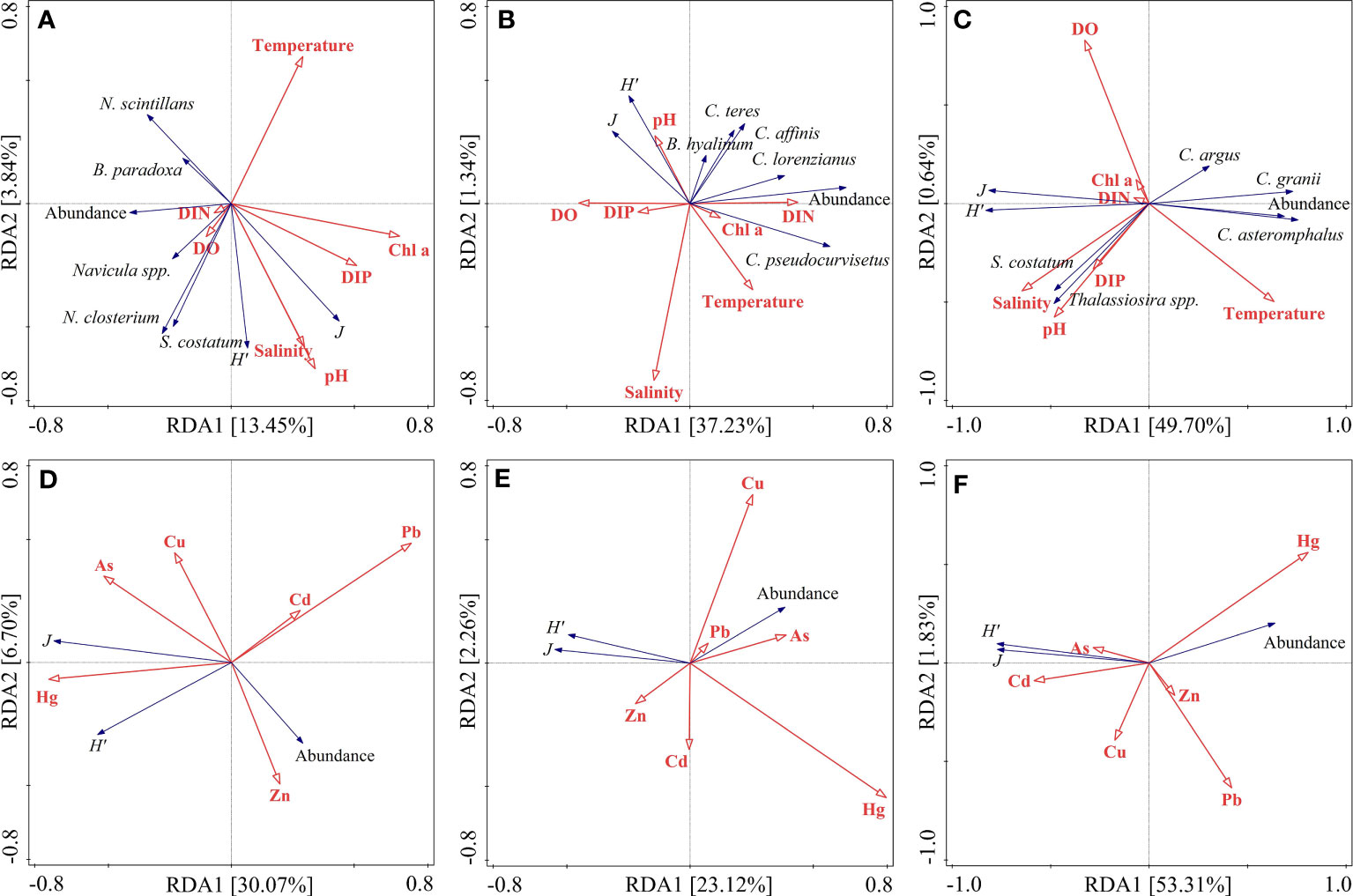
Figure 7 The RDA analysis for the relationships between phytoplankton abundance and environmental variables as well as heavy metals during the three seasons along the coastal Yellow Sea. (A–C) show the relationships between phytoplankton abundance and environmental variables in spring, summer, and autumn, respectively. (D–F) indicate the relationships between phytoplankton abundance and heavy metals in spring, summer, and autumn, respectively.
4. Discussion
4.1. Key factors affecting the phytoplankton community composition
In the present study, the phytoplankton community were mainly contributed by diatoms and dinoflagellates, but diatoms absolutely dominated throughout the coastal Yellow Sea (Tables 2, 3). Among the top five dominant species of each season, similarly, most of them were also diatoms, except for Noctiluca scintillans which only occurred in the spring. However, our results are quite consistent with previous studies in the China seas (Guo et al., 2014; Wei et al., 2017; Zhong et al., 2021; Wei et al., 2022a). For example, Guo et al. (2014) have explored the seasonal variation of phytoplankton in the East China Sea (ECS), and concluded that the phytoplankton community in the ECS were mainly composed of diatoms and dinoflagellates. Wei et al. (2017) have classified the phytoplankton community into six ecological provinces in the Bohai Sea and Yellow Sea, where the spatial distribution of phytoplankton was depicted by diatoms. Zhong et al. (2021) have examined the phytoplankton assemblages in the Pearl River estuary of the northern South China Sea, and revealed that chain-forming diatoms dominated the phytoplankton community. Moreover, Wei et al. (2022a) have documented the response of phytoplankton community to current changing coastal environment in the Bohai Sea, and suggested that diatoms were the most abundant and diverse group during the past three years, representing ~78% and ~82% of total abundance and taxa on average, respectively. Taken together, these data demonstrate that our results within this study can be typical of eutrophication conditions in the coastal ecosystems.
Various theoretical arguments based on laboratory experiments and field studies have suggested that the community composition of phytoplankton is always correlated with the fluctuation of physico-chemical environmental variables (Xiao et al., 2018). Based on the raw data, our RDA analysis showed that DIN was strongly related to the dominant species that were mainly composed of diatoms (Figure 7 and Table 2). This close relationship in fact seems reasonable, as diatoms tend to have a competitive advantage when nutrient concentrations are high, compared to dinoflagellates. For diatoms, they are typically R-strategists and tend to have higher maximum rates of carbon-specific nutrient uptake (Alves-de-Souza et al., 2008; Edwards et al., 2012). As a mixotrophic group, however, dinoflagellates are very insensitive to high-nutrient conditions. Therefore, the higher nutrient concentrations (especially DIN) along the coastal Yellow Sea favored the diatom growth (Figure 2 and Table 1). We also found that water temperature had a potential impact on the dynamics of dominant diatoms (Figure 7). The temperature sensitivity of diatoms is consistent with a previous found that diatoms adapt to a wide range of temperatures, i.e., diatoms have a wide thermal tolerance in the global scenario (Chen, 2015). Certainly, our RDA analysis showed that both temperature and DIN had potential effects on the phytoplankton community composition, indicating that the temperature effect was very dependent on nutritional status, which can be either the result of species specific variation or the interaction of temperature and nutrient on the same dominant species (Anderson, 2000; Xiao et al., 2018; Wei et al., 2022a). In particular, the RDA results showed that the phytoplankton abundance dominated by diatoms had positive correlations with high temperature at high DIN concentration in summer (Figure 7B). The implication is that the response of diatoms to high temperature may not be a result of innate metabolic intolerance to super-optimal temperatures, as long as temperature stress is not accompanied by the additional stress of nutrient limitation. In contrast, dinoflagellate blooms often occur at temperatures of ~18 °C when nutrient concentrations are low (Graneli et al., 2011). In the coastal Yellow Sea, however, there is a continuous input of terrestrial nutrients (especially DIN), causing eutrophic conditions, which may thus benefit diatoms and inhibit dinoflagellates. Altogether, the interactions of temperature and DIN as well as different nutritional strategies favor the growth of diatoms but have apparent negative effect on dinoflagellate species along the coastal Yellow Sea.
Water turbulence may also have a direct impact on the community composition of phytoplankton in the coastal Yellow Sea (Guo et al., 2014; Wei et al., 2017). As a result of the complex hydrological characteristics of the Yellow Sea, internal waves are a common phenomenon along the coastal Yellow Sea. For example, many in situ observations (Liu et al., 2009) and satellite images (Zhao et al., 2014) have reflected the existence of internal waves in the Yellow Sea. Numerous studies have shown that the rupture of internal solitary waves can generate intensified turbulence within the thermocline (Klymak and Moum, 2003; Moum et al., 2003; Liu et al., 2009). However, the integrity and metabolism of dinoflagellate cells are susceptible to turbulence, such as cell division, morphology, and the nutrient-retrieval migration, while diatoms can thrive in strong turbulence due to the protection of their cell walls (Sullivan et al., 2003; Peters et al., 2006; Clarson et al., 2009; Guo et al., 2014). This can be further confirmed by previous studies, which have revealed that changes in ocean circulation and water masses can affect the composition of phytoplankton community (Wei et al., 2017; Wei et al., 2020). Also, Eriksen et al. (2018) have proposed that when turbulence levels increase, the composition of phytoplankton community changes from dinoflagellates to diatoms. Taken together, water turbulence may be another factor responsible for the phytoplankton community composition.
4.2. Key factors affecting the seasonal variation of phytoplankton
Previous studies have demonstrated that the seasonal variation in phytoplankton abundance is mainly affected by various environmental parameters such as nutrient, temperature, salinity, light availability and so forth (Chen et al., 2003; Edwards et al., 2012; Johnson et al., 2013; Guo et al., 2014; Eriksen et al., 2018; Wei et al., 2022a). According to our RDA analysis in this study, the abundance of phytoplankton across the three seasons was closely related to water temperature and DIN concentration (Figure 7), indicating that water temperature and DIN may be the determinants controlling the seasonal variability of phytoplankton abundance along the coastal Yellow Sea. This result, however, is quite consistent with previous studies in the Yellow Sea (Lin et al., 2005; Fu et al., 2009; Zhang et al., 2016; Wei et al., 2017; Liu et al., 2019). For instance, Lin et al. (2005) have investigated the ecological responses of phytoplankton abundance and primary production to environmental changes in the Yellow Sea during 1976-2000, and suggested that the seasonal dynamics of phytoplankton abundance are strongly related to DIN and N:P ratios. Fu et al. (2009) have reported that temperature is an important regulation factor for the seasonal variability of phytoplankton biomass size structure in the Southern Yellow Sea. Zhang et al. (2016) and Wei et al. (2017) have revealed the different ecological distribution of phytoplankton abundance during spring in the Yellow Sea, based on the regional variations of water temperature and nutrients (especially DIN and DIP). Based on a time series of satellite data on Chl a concentration, Liu et al. (2019) have determined the mechanisms of phytoplankton variation in recent decade in the Yellow Sea during 2003-2015, and found an intimate relationship between sea surface temperature and phytoplankton. In addition, diatoms, as the dominant species of phytoplankton along the coastal Yellow Sea, are typically R-strategists in nutrient uptake and have a wide thermal tolerance as discussed above (Alves-de-Souza et al., 2008; Edwards et al., 2012; Guo et al., 2014; Chen, 2015). For example, diatoms can accelerate N assimilation under nitrate-rich conditions and thus have inherently high growth rates (Edwards et al., 2012; Chen, 2015). It is noteworthy that the effect of DIN concentration on phytoplankton abundance was significantly negative in autumn (Figure 7C). In fact, the DIN concentration in the coastal Yellow Sea was much higher in autumn than in spring and summer (Figure 2). The implication is that DIN was no longer the potential limiting nutrient for the growth of phytoplankton in the coastal Yellow Sea, instead of a inhibited factor. Consequently, the significant correlations between phytoplankton abundance and temperature and DIN during the three seasons suggest that the seasonal variation of phytoplankton is associated with the seasonal differences in temperature and DIN in the coastal Yellow Sea. Certainly, some physical processes (e.g., Yellow Sea Warm Current, YSWC; Liu et al., 2015) or other environmental factors (e.g., light availability; Findlay et al., 2006; Fu et al., 2009; Zhang et al., 2016) in the Yellow Sea may affect the seasonal variability of phytoplankton abundance, but those would not be discussed in detail due to the lack of data within our study.
According to the RDA analysis (Figure 7), we also observed that the phytoplankton abundance was strongly correlated with heavy metals (especially Zn, As, and Hg) during the three seasons, indicating that heavy metals also had significant influences on the seasonal dynamics of phytoplankton. In other words, Zn, As, and Hg were the main possible metals that affected the seasonal dynamics of phytoplankton along the coastal Yellow Sea. This result, however, is in line with a previous study that metals Zn, As, and Hg have diverse consequences for the dynamics of phytoplankton in the Bohai Sea (Wei et al., 2022a). It is well known that some heavy metals are essential micronutrients but some have no known metabolic function for phytoplankton growth (Abdou and Tercier-Waeber, 2022; Wei et al., 2022a). For instance, Zn is an essential element in key phytoplankton biochemical functions within an optimal concentration range. Recent studies have also suggested that Zn may be involved in the biological function and adaptive evolution of phytoplankton (Mikhaylina et al., 2022; Ye et al., 2022). In contrast, Hg is thought to be a hazardous metal that can be accumulated by phytoplankton as well as zooplankton, but zooplankton are much more sensitive to Hg than phytoplankton (Zhu et al., 2020; Abdou and Tercier-Waeber, 2022). The positive correlation between phytoplankton abundance and metal Hg in fall may be a result of the harmful effect of Hg on zooplankton, which thus reduces the predation pressure of zooplankton on phytoplankton. Although As was also related to the phytoplankton dynamic in summer (Figure 7E), it is generally considered as a non-essential toxic metal with no known metabolic function, and thus more data are required for future work. Overall, heavy metals can impact the seasonal dynamics of phytoplankton along the coastal Yellow Sea with effects that may differ physiologically and functionally.
Additionally, Coscinodiscus granii bloom was observed at several stations along the coastal Yellow Sea during the autumn (Figure 5). Analogously, the autumnal blooms in phytoplankton have also been observed in many eutrophic ecosystems around the world (Song et al., 2010). The classic theory suggests that phytoplankton blooms in autumn are related to the vertical mixing in autumn (Chen et al., 2003; Findlay et al., 2006). This is because the increased vertical mixing and subsequent decomposition of stratification in autumn can lead to the inflow of nutrients into the upper layers of the ocean. The ocean surface has sufficient light to support photosynthesis, which is a key for the massive growth of phytoplankton. In addition, the grazing of zooplankton is affected by the increase of vertical mixing, it may further promote the growth and proliferation of phytoplankton (Irigoien et al., 2004; Findlay et al., 2006; Song et al., 2010). In theory, the combination of the above factors can thus initiate a bloom of phytoplankton in autumn.
5. Conclusions
Marine phytoplankton, as primary producers in marine ecosystems, play crucial roles in food chains, energy supply, element cycling, ecosystem functioning as well as the global biogeochemical cycles. The characteristics of phytoplankton community and diversity are thus important indicators for evaluating the nutrient level, pollution status, resource status, production potential and stability of marine ecosystems. Major progress has been made in the study of phytoplankton dynamics in the past decades. However, more studies are needed to further increase the knowledge of the processes for marine environments affecting the phytoplankton dynamics, especially in coastal regions where environmental variables are comparatively complex. In this study, we conducted 3 cruises during three different seasons in the coastal Yellow Sea to suggest that temperature and DIN may be the determinants controlling the seasonal variability of phytoplankton abundance in this coastal region. We also found that metals Zn, As, and Hg had potential affects on the seasonal dynamics of phytoplankton community along the coastal Yellow Sea. Accordingly, we suggested that the interaction of water temperature, DIN, and metals Zn, As, and Hg might be key in driving the seasonal variation of phytoplankton community that was dominated by diatoms.
Data availability statement
The original contributions presented in the study are included in the article/Supplementary Material. Further inquiries can be directed to the corresponding author.
Author contributions
YuW contributed to the theoretical designs. YiW, YL, XC, KQ, and ZC performed all experiments. YiW and YL analyzed all data. YiW wrote the manuscript with the help of all authors. All authors contributed to the article and approved the submitted version.
Acknowledgments
This work was financially supported by the National Natural Science Foundation of China (42206103), the Project funded by China Postdoctoral Science Foundation (2021M703590), the Shandong Postdoctoral Innovation Talent Support Program (SDBX2021014), the Shandong Natural Science Foundation (ZR2022QD133), and the Central Public-interest Scientific Institution Basal Research Fund, YSFRI, CAFS (20603022022010). Also, we would like to show our great gratitude to all members of Yellow Sea & Bohai Sea Fishery Ecological Environment Monitoring Center for their assistance in our sampling and analysis processes.
Conflict of interest
The authors declare that this article was conducted in the absence of any commercial or financial relationships that could be construed as a potential conflict of interest.
Publisher’s note
All claims expressed in this article are solely those of the authors and do not necessarily represent those of their affiliated organizations, or those of the publisher, the editors and the reviewers. Any product that may be evaluated in this article, or claim that may be made by its manufacturer, is not guaranteed or endorsed by the publisher.
Supplementary material
The Supplementary Material for this article can be found online at: https://www.frontiersin.org/articles/10.3389/fmars.2022.1076975/full#supplementary-material
References
Abdou M., Tercier-Waeber M. L. (2022). New insights into trace metal speciation and interaction with phytoplankton in estuarine coastal waters. Mar. pollut. Bull. 181, 113845. doi: 10.1016/j.marpolbul.2022.113845
Acevedo-Trejos E., Brandt G., Steinacher M., Merico A. (2014). A glimpse into the future composition of marine phytoplankton communities. Front. Mar. Sci. 1, 15. doi: 10.3389/fmars.2014.00015
Agawin N. S., Duarte C. M., Agustí S. (2000). Nutrient and temperature control of the contribution of picoplankton to phytoplankton biomass and production. Limnology oceanography 45 (3), 591–600. doi: 10.4319/lo.2000.45.3.0591
Alves-de-Souza C., González M. T., Iriarte J. L. (2008). Functional groups in marine phytoplankton assemblages dominated by diatoms in fjords of southern Chile. J. Plankton Res. 30 (11), 1233–1243. doi: 10.1093/plankt/fbn079
Anderson N. J. (2000). Diatoms, temperature and climatic change. Eur. J. Phycology 35 (4), 307–314. doi: 10.1017/S0967026200002857
Barton A. D., Dutkiewicz S., Flierl G., Bragg J., Follows M. J. (2010). Patterns of diversity in marine phytoplankton. Science 327 (5972), 1509–1511. doi: 10.1126/science.1184961
Barton A. D., Finkel Z. V., Ward B. A., Johns D. G., Follows M. J. (2013). On the roles of cell size and trophic strategy in north Atlantic diatom and dinoflagellate communities. Limnology Oceanography 58 (1), 254–266. doi: 10.4319/lo.2013.58.1.0254
Chen B. (2015). Patterns of thermal limits of phytoplankton. J. Plankton Res. 37 (2), 285–292. doi: 10.1093/plankt/fbv009
Chen C., Zhu J., Beardsley R. C., Franks P. J. (2003). Physical-biological sources for dense algal blooms near the changjiang river. Geophysical Res. Lett. 30 (10), 22–25. doi: 10.1029/2002GL016391
Clarson S. J., Steinitz-Kannan M., Patwardhan S. V., Kannan R., Hartig R., Schloesser L., et al. (2009). Some observations of diatoms under turbulence. Silicon 1 (2), 79–90. doi: 10.1007/s12633-009-9018-y
Crouch S. R., Malmstadt H. V. (1967). Mechanistic investigation of molybdenum blue method for determination of phosphate. Analytical Chem. 39 (10), 1084–1089. doi: 10.1021/ac60254a027
Edwards K. F., Thomas M. K., Klausmeier C. A., Litchman E. (2012). Allometric scaling and taxonomic variation in nutrient utilization traits and maximum growth rate of phytoplankton. Limnology Oceanography 57 (2), 554–566. doi: 10.4319/lo.2012.57.2.0554
Eriksen R., Trull T. W., Davies D., Jansen P., Davidson A. T., Westwood K., et al. (2018). Seasonal succession of phytoplankton community structure from autonomous sampling at the Australian southern ocean time series (SOTS) observatory. Mar. Ecol. Prog. Ser. 589, 13–31. doi: 10.3354/meps12420
Field C. B., Behrenfeld M. J., Randerson J. T., Falkowski P. (1998). Primary production of the biosphere: integrating terrestrial and oceanic components. science 281 (5374), 237–240. doi: 10.1126/science.281.5374.237
Findlay H. S., Yool A., Nodale M., Pitchford J. W. (2006). Modelling of autumn plankton bloom dynamics. J. plankton Res. 28 (2), 209–220. doi: 10.1093/plankt/fbi114
Fu X., Sun J., Wei Y., Liu Z., Xin Y., Guo Y., et al. (2021). Seasonal shift of a phytoplankton (> 5 µm) community in bohai Sea and the adjacent yellow Sea. Diversity 13 (2), 65. doi: 10.3390/d13020065
Fu M., Wang Z., Li Y., Li R., Sun P., Wei X., et al. (2009). Phytoplankton biomass size structure and its regulation in the southern yellow Sea (China): Seasonal variability. Continental Shelf Res. 29 (18), 2178–2194. doi: 10.1016/j.csr.2009.08.010
Graneli E., Vidyarathna N. K., Funari E., Cumaranatunga P. R. T., Scenati R. (2011). Can increases in temperature stimulate blooms of the toxic benthic dinoflagellate ostreopsis ovata? Harmful algae 10 (2), 165–172. doi: 10.1016/j.hal.2010.09.002
Guo S., Feng Y., Wang L., Dai M., Liu Z., Bai Y., et al. (2014). Seasonal variation in the phytoplankton community of a continental-shelf sea: the East China Sea. Mar. Ecol. Prog. Ser. 516, 103–126. doi: 10.3354/meps10952
Hwang J. H., Van S. P., Choi B. J., Chang Y. S., Kim Y. H. (2014). The physical processes in the yellow Sea. Ocean Coast. Manage. 102, 449–457. doi: 10.1016/j.ocecoaman.2014.03.026
Irigoien X., Huisman J., Harris R. P. (2004). Global biodiversity patterns of marine phytoplankton and zooplankton. Nature 429 (6994), 863–867. doi: 10.1038/nature02593
Johnson M. D., Stoecker D. K., Marshall H. G. (2013). Seasonal dynamics of mesodinium rubrum in Chesapeake bay. J. plankton Res. 35 (4), 877–893. doi: 10.1093/plankt/fbt028
Keesing J. K., Liu D., Fearns P., Garcia R. (2011). Inter-and intra-annual patterns of ulva prolifera green tides in the yellow Sea during 2007–2009, their origin and relationship to the expansion of coastal seaweed aquaculture in China. Mar. pollut. Bull. 62 (6), 1169–1182. doi: 10.1016/j.marpolbul.2011.03.040
Klymak J. M., Moum J. N. (2003). Internal solitary waves of elevation advancing on a shoaling shelf. Geophysical Res. Lett. 30 (20), 3–9. doi: 10.1029/2003GL017706
Kong F. Z., Jiang P., Wei C. J., Zhang Q. C., Li J. Y., Liu Y. T., et al. (2018). Co-Occurrence of green tide, golden tide and red tides along the 35° n transect in the yellow Sea during spring and summer in 2017. Oceanologia Limnologia Sin. 49 (5), 1021–1030. doi: 10.11693/hyhz20180400082
Lin C. L., Ning X. R., Su J. L., Lin Y., Xu B. (2005). Environmental changes and the responses of the ecosystems of the yellow Sea during 1976–2000. J. Mar. Syst. 55 (3-4), 223–234. doi: 10.1016/j.jmarsys.2004.08.001
Litchman E., de Tezanos Pinto P., Edwards K. F., Klausmeier C. A., Kremer C. T., Thomas M. K. (2015). Global biogeochemical impacts of phytoplankton: A trait-based perspective. J. Ecol. 103 (6), 1384–1396. doi: 10.1111/1365-2745.12438
Liu X., Huang B., Huang Q., Wang L., Ni X., Tang Q., et al. (2015). Seasonal phytoplankton response to physical processes in the southern yellow Sea. J. Sea Res. 95, 45–55. doi: 10.1016/j.seares.2014.10.017
Liu C., Sun Q., Xing Q., Wang S., Tang D., Zhu D., et al. (2019). Variability in phytoplankton biomass and effects of sea surface temperature based on satellite data from the yellow Sea, China. PloS One 14 (8), e0220058. doi: 10.1371/journal.pone.0220058
Liu Z., Wei H., Lozovatsky I. D., Fernando H. J. S. (2009). Late summer stratification, internal waves, and turbulence in the yellow Sea. J. Mar. Syst. 77 (4), 459–472. doi: 10.1016/j.jmarsys.2008.11.001
Longhurst A., Sathyendranath S., Platt T., Caverhill C. (1995). An estimate of global primary production in the ocean from satellite radiometer data. J. plankton Res. 17 (6), 1245–1271. doi: 10.1093/plankt/17.6.1245
Mikhaylina A., Ksibe A. Z., Wilkinson R. C., Smith D., Marks E., Coverdale J. P., et al. (2022). A single sensor controls large variations in zinc quotas in a marine cyanobacterium. Nat. Chem. Biol. 18, 1–9. doi: 10.1038/s41589-022-01051-1
Moum J. N., Farmer D. M., Smyth W. D., Armi L., Vagle S. (2003). Structure and generation of turbulence at interfaces strained by internal solitary waves propagating shoreward over the continental shelf. J. Phys. Oceanography 33 (10), 2093–2112. doi: 10.1175/1520-0485(2003)033<2093:SAGOTA>2.0.CO;2
Niehoff B., Madsen S., Hansen B., Nielsen T. (2002). Reproductive cycles of three dominant calanus species in disko bay, West Greenland. Mar. Biol. 140 (3), 567–576. doi: 10.1007/s00227-001-0731-3
Peters F., Arin L., Marrasé C., Berdalet E., Sala M. M. (2006). Effects of small-scale turbulence on the growth of two diatoms of different size in a phosphorus-limited medium. J. Mar. Syst. 61 (3-4), 134–148. doi: 10.1016/j.jmarsys.2005.11.012
Ptacnik R., Solimini A. G., Andersen T., Tamminen T., Brettum P., Lepistö L., et al. (2008). Diversity predicts stability and resource use efficiency in natural phytoplankton communities. Proc. Natl. Acad. Sci. 105 (13), 5134–5138. doi: 10.1073/pnas.0708328105
Pujari L., Kan J., Xin Y., Zhang G., Noman M. A., Nilajkar S., et al. (2022). Deciphering the diversity and distribution of chromophytic phytoplankton in the bohai Sea and the yellow Sea via RuBisCO genes (rbcL). Mar. pollut. Bull. 184, 114193. doi: 10.1016/j.marpolbul.2022.114193
Riebesell U. (2004). Effects of CO2 enrichment on marine phytoplankton. J. Oceanography 60 (4), 719–729. doi: 10.1007/s10872-004-5764-z
Rost B., Zondervan I., Wolf-Gladrow D. (2008). Sensitivity of phytoplankton to future changes in ocean carbonate chemistry: current knowledge, contradictions and research directions. Mar. Ecol. Prog. Ser. 373, 227–237. doi: 10.3354/meps07776
Schloss I. R., Abele D., Moreau S., Demers S., Bers A. V., González O., et al. (2012). Response of phytoplankton dynamics to 19-year, (1991–2009) climate trends in potter cove (Antarctica). J. Mar. Syst. 92 (1), 53–66. doi: 10.1016/j.jmarsys.2011.10.006
Song H., Ji R., Stock C., Wang Z. (2010). Phenology of phytoplankton blooms in the Nova scotian shelf-gulf of Maine region: remote sensing and modeling analysis. J. Plankton Res. 32 (11), 1485–1499. doi: 10.1093/plankt/fbq086
Staehr P. A., Sand-Jensen K. A. J. (2006). Seasonal changes in temperature and nutrient control of photosynthesis, respiration and growth of natural phytoplankton communities. Freshw. Biol. 51 (2), 249–262. doi: 10.1111/j.1365-2427.2005.01490.x
Sullivan J. M., Swift E., Donaghay P. L., Rines J. E. (2003). Small-scale turbulence affects the division rate and morphology of two red-tide dinoflagellates. Harmful Algae 2 (3), 183–199. doi: 10.1016/S1568-9883(03)00039-8
Sun D., Huan Y., Wang S., Qiu Z., Ling Z., Mao Z., et al. (2019). Remote sensing of spatial and temporal patterns of phytoplankton assemblages in the bohai Sea, yellow Sea, and east China sea. Water Res. 157, 119–133. doi: 10.1016/j.watres.2019.03.081
Teng S. K., Yu H., Tong L., Choi C. I., Kang D., Liu H., et al. (2012). Yellow Sea, global international waters assessment, GIWA, regional assessment (Kalmar, Sweden: University of Kalmar) 34.
Thomas M. K., Aranguren-Gassis M., Kremer C. T., Gould M. R., Anderson K., Klausmeier C. A., et al. (2017). Temperature–nutrient interactions exacerbate sensitivity to warming in phytoplankton. Global Change Biol. 23 (8), 3269–3280. doi: 10.1111/gcb.13641
Utermöhl V. H. (1931). Neue wege in der quantitativen erfassung des plankton. (Mit besonderer berücksichtigung des ultraplanktons.). Mit 4 Abbildungen im Text. Internationale Vereinigung für theoretische und angewandte Limnologie: Verhandlungen 5 (2), 567–596. doi: 10.1080/03680770.1931.11898492
Verdouw H., Van Echteld C. J. A., Dekkers E. M. J. (1978). Ammonia determination based on indophenol formation with sodium salicylate. Water Res. 12 (6), 399–402. doi: 10.1016/0043-1354(78)90107-0
Wang B., Xin M., Wei Q., Xie L. (2018). A historical overview of coastal eutrophication in the China seas. Mar. pollut. Bull. 136, 394–400. doi: 10.1016/j.marpolbul.2018.09.044
Wei Y., Cui H., Hu Q., Bai Y., Qu K., Sun J., et al. (2022b). Eutrophication status assessment in the laizhou bay, bohai Sea: Further evidence for the ecosystem degradation. Mar. pollut. Bull. 181, 113867. doi: 10.1016/j.marpolbul.2022.113867
Wei Y., Ding D., Gu T., Jiang T., Qu K., Sun J., et al. (2022a). Different responses of phytoplankton and zooplankton communities to current changing coastal environments. Environ. Res. 215, 114426. doi: 10.1016/j.envres.2022.114426
Wei Y., Liu H., Zhang X., Xue B., Munir S., Sun J. (2017). Physicochemical conditions in affecting the distribution of spring phytoplankton community. Chin. J. Oceanology Limnology 35 (6), 1342–1361. doi: 10.1007/s00343-017-6190-6
Wei Y., Sun J., Zhang G., Wang X., Wang F. (2020). Environmental factors controlling the dynamics of phytoplankton communities during spring and fall seasons in the southern sunda shelf. Environ. Sci. pollut. Res. 27 (18), 23222–23233. doi: 10.1007/s11356-020-08927-6
Welschmeyer N. A. (1994). Fluorometric analysis of chlorophyll a in the presence of chlorophyll b and pheopigments. Limnology oceanography 39 (8), 1985–1992. doi: 10.4319/lo.1994.39.8.1985
Xiao C., Jian H., Chen L., Liu C., Gao H., Zhang C., et al. (2017). Toxic metal pollution in the yellow Sea and bohai Sea, China: distribution, controlling factors and potential risk. Mar. pollut. Bull. 119 (1), 381–389. doi: 10.1016/j.marpolbul.2017.03.027
Xiao W., Liu X., Irwin A. J., Laws E. A., Wang L., Chen B., et al. (2018). Warming and eutrophication combine to restructure diatoms and dinoflagellates. Water Res. 128, 206–216. doi: 10.1016/j.watres.2017.10.051
Xu K., Choi J. K., Lei Y., Yang E. J. (2011). Marine ciliate community in relation to eutrophication of coastal waters in the yellow Sea. Chin. J. Oceanology Limnology 29 (1), 118–127. doi: 10.1007/s00343-011-9106-x
Ye N., Han W., Toseland A., Wang Y., Fan X., Xu D., et al. (2022). The role of zinc in the adaptive evolution of polar phytoplankton. Nat. Ecol. Evol. 6, 1–14. doi: 10.1038/s41559-022-01750-x
Ye N. H., Zhang X. W., Mao Y. Z., Liang C. W., Xu D., Zou J., et al. (2011). ‘Green tides’ are overwhelming the coastline of our blue planet: taking the world’s largest example. Ecol. Res. 26 (3), 477–485. doi: 10.1007/s11284-011-0821-8
Zhang S., Leng X., Feng Y., Ding C., Yang Y., Wang J., et al. (2016). Ecological provinces of spring phytoplankton in the yellow Sea: species composition. Acta Oceanologica Sin. 35 (8), 114–125. doi: 10.1007/s13131-016-0872-3
Zhao Z., Liu B., Li X. (2014). Internal solitary waves in the China seas observed using satellite remote-sensing techniques: a review and perspectives. Int. J. Remote Sens. 35 (11-12), 3926–3946. doi: 10.1080/01431161.2014.916442
Zhong Q., Xue B., Noman M. A., Wei Y., Liu H., Liu H., et al. (2021). Effect of river plume on phytoplankton community structure in zhujiang river estuary. J. Oceanology Limnology 39 (2), 550–565. doi: 10.1007/s00343-020-9213-7
Keywords: phytoplankton, seasonal variation, nutrient, temperature, heavy metal, Yellow Sea
Citation: Wang Y, Liu Y, Chen X, Cui Z, Qu K and Wei Y (2022) Exploring the key factors affecting the seasonal variation of phytoplankton in the coastal Yellow Sea. Front. Mar. Sci. 9:1076975. doi: 10.3389/fmars.2022.1076975
Received: 22 October 2022; Accepted: 24 November 2022;
Published: 06 December 2022.
Edited by:
Qicheng Meng, Ministry of Natural Resources, ChinaReviewed by:
Laxman Pujari, Council of Scientific and Industrial Research (CSIR), IndiaYongyu Zhang, Qingdao Institute of Bioenergy and Bioprocess Technology, Chinese Academy of Sciences (CAS), China
Copyright © 2022 Wang, Liu, Chen, Cui, Qu and Wei. This is an open-access article distributed under the terms of the Creative Commons Attribution License (CC BY). The use, distribution or reproduction in other forums is permitted, provided the original author(s) and the copyright owner(s) are credited and that the original publication in this journal is cited, in accordance with accepted academic practice. No use, distribution or reproduction is permitted which does not comply with these terms.
*Correspondence: Yuqiu Wei, weiyuqiu@163.com
†These authors have contributed equally to this work