High Phenotypic Plasticity, but Low Signals of Local Adaptation to Climate in a Large-Scale Transplant Experiment of Picea abies (L.) Karst. in Europe
- 1Chair of Forest Growth and Woody Biomass Production, TU Dresden, Tharandt, Germany
- 2Thuenen Institute of Forest Genetics, Grosshansdorf, Germany
The most common tool to predict future changes in species range are species distribution models. These models do, however, often underestimate potential future habitat, as they do not account for phenotypic plasticity and local adaptation, although being the most important processes in the response of tree populations to rapid climate change. Here, we quantify the difference in the predictions of future range for Norway spruce, by (i) deriving a classic, occurrence-based species distribution model (OccurrenceSDM), and (ii) analysing the variation in juvenile tree height and translating this to species occurrence (TraitSDM). Making use of 32 site locations of the most comprehensive European trial series that includes 1,100 provenances of Norway spruce originating from its natural and further beyond from its largely extended, artificial distribution, we fit a universal response function to quantify growth as a function of site and provenance climate. Both the OccurrenceSDM and TraitSDM show a substantial retreat towards the northern latitudes and higher elevations (−55 and −43%, respectively, by the 2080s). However, thanks to the species’ particularly high phenotypic plasticity in juvenile height growth, the decline is delayed. The TraitSDM identifies increasing summer heat paired with decreasing water availability as the main climatic variable that restricts growth, while a prolonged frost-free period enables a longer period of active growth and therefore increasing growth potential within the restricted, remaining area. Clear signals of local adaptation to climatic clines spanning the entire range are barely detectable, as they are disguised by a latitudinal cline. This cline strongly reflects population differentiation for the Baltic domain, but fails to capture the high phenotypic variation associated to the geographic heterogeneity in the Central European mountain ranges paired with the species history of postglacial migration. Still the model is used to provide recommendations of optimal provenance choice for future climate conditions. In essence, assisted migration may not decrease the predicted range decline of Norway spruce, but may help to capitalize on potential opportunities for increased growth associated with warmer climates.
Introduction
Climate change significantly alters geographical distribution and composition of the European forest ecosystems, as already observed in terms of species migration, range shifts (Petit et al., 2004; Delzon et al., 2013) and increased tree mortality (Taccoen et al., 2019) attributed to contemporary warming and decreases in growing season precipitation. However, it remains uncertain, whether tree populations will become extinct, migrate, or persist facing the anticipated speed of change in environmental conditions (Aitken et al., 2008). The future extent of their distribution is a much sought-after goal of statistical modeling in order to aid forest management to develop strategies of conservation, passive or active adaptation to maintain desired forest ecosystem services (Bolte et al., 2009). The most widely used tool are species distribution models (SDMs) that predict the potential distribution of species based on the correlation between observed presence of species and the corresponding environmental conditions (Zurell et al., 2020). For tree species in particular, they have been used to predict future species distributions (van der Maaten et al., 2017; Dyderski et al., 2018; Buras and Menzel, 2019; Pecchi et al., 2020; Chakraborty et al., 2021), to model potential future migration rates (Meier et al., 2012), to identify areas relevant for conservation (Nakao et al., 2013; Barnes and Delborne, 2019), or to develop management strategies with climate change (Gray and Hamann, 2013; Isaac-Renton et al., 2014).
Despite few exceptions that first divide species into metapopulations based on climatic and geographic homogeneity to then predict which metapopulation shows best suitability under predicted future climate (Gray and Hamann, 2013; Isaac-Renton et al., 2014), the SDM studies generally follow the key assumptions that (1) there is no intra-specific variability, i.e., all populations across the distribution range perform similarly; and (2) species are assumed to produce homogenous responses to climate variations across their range, i.e., they are usually calibrated with species occurrence or species presence-absence as the only variable factor for a given species over its range (Zurell et al., 2020). Widespread tree species are, however, not one homogeneous genetic entity, they rather consist of genetically differentiated populations that are often narrowly adapted to their local environments (Morgenstern, 1996; Alberto et al., 2013). Further, as long-lived organisms trees are exposed to temporal variation in environmental conditions throughout their life time and do show considerable high amounts of phenotypic plasticity allowing them to physiologically or morphologically respond to changing environments (Kramer, 1995). Local adaptation produces new genotypes via natural selection that are better suited to cope with the local environment (Kawecki and Ebert, 2004; Savolainen et al., 2007), while plasticity enables a given genotype to express different phenotypes in response to a changing environment (Bradshaw, 1965). Both are the most important processes in the response of tree populations to rapid climate change (Aitken et al., 2008; Franks et al., 2014), but are scarcely considered by classical occurrence-based SDMs.
Provenance trails, being large and systematic transplant experiments, suit well as experimental base to assess local adaptation and plasticity. Originally established by forest geneticists since the 1950’s, and maintained with the goal of identifying superior genotypes for reforestation, these trials have emerged as useful climate change laboratories (Mátyás, 1994). For example, an earlier leaf unfolding or greater height growth expressed after translocation to warmer climate than the source climate may be interpreted as a potential reaction to climate warming. Using height of a range-wide collection of Pinus contorta assessed across 60 sites, Wang et al. (2010) were the first, who developed a predictive model combining reaction norms that model quantitative trait variation as a function of provenance climate (past climate experienced at seed source origin) to which populations are genetically adapted, and simultaneously as a function of site climate (current climate experienced at common-garden) to which they show plastic responses. These so called Universal Response Functions (URFs) were also successfully applied to predict future growth potential of Picea mariana and Pinus strobus (Yang et al., 2015), Pseudotsuga menziesii (Chakraborty et al., 2015), and Platycladus orientalis (Hu et al., 2019). Great advantage is their ability to predict the performance of any population growing in any climate (Wang et al., 2010). While URFs are basically linear models, several recent studies have extended this approach to the mixed model framework, which allows to consider the experimental design as random effects in modeling intraspecific variation in single (Fréjaville et al., 2020; Vizcaíno-Palomar et al., 2020) and multiple traits (Gárate-Escamilla et al., 2019). In this respect, Benito-Garzón et al. (2019) proposed the term ΔTraitSDM to summarize all models considering phenotypic trait variation (e.g., survival, growth, and phenology) as key dimension in the response of populations to rapid environmental change.
In this study, we develop a universal response function to predict growth potential of Norway spruce [Picea abies (L.) Karst.] that accounts for both local adaptation and phenotypic plasticity. Due to its economic versatility paired with the ease of propagation Norway spruce became the most preferred option to reforest degraded areas over the last three centuries (Jansen et al., 2017), which has significantly enlarged its native range. However, with ongoing climate change, growing conditions are increasingly becoming unsuitable and climatically stressed trees are predisposed to various abiotic and biotic threats. Occurrence-based SDMs anticipate that the species will face great reductions (e.g., van der Maaten et al., 2017; Dyderski et al., 2018). Here, we expand such relatively simple species presence-absence models (henceforth “OccurrenceSDM”) by acknowledging the tolerance and adaptive capacity of Norway spruce to environmental changes. More specifically, we use extensive international transplant experiments to (1) identify the climatic parameters of seed source (provenance) and growing environment (site) that trigger observed variation in juvenile height, to then (2) develop a trait-based model (henceforth “TraitSDM”) to predict future height growth potential. Model predictions of the developed TraitSDM are translated to species presence and compared with those of the OccurrenceSDM to test the hypothesis that phenotypic plasticity supports persistence on a much larger area than expected by occurrence-based predictions (cf. Benito-Garzón et al., 2019). Finally, the TraitSDM is used to identify areas, where an active seed transfer may have a positive effect on growth potential.
Materials and Methods
Common Gardens
We assessed population response of Norway spruce to climate change by a Europe-wide transplant experiment, the International Provenance Test 1964/68 with Norway spruce (IPTNS) initiated by the international Union of Forest Research Organizations (IUFRO). A total of 1,100 stands was sampled across Europe (Figure 1) and sown in spring 1964 (complete list of provenances may be found in Ujvári-Jármay et al., 2016). After uniform pre-treatment at one single nursery in Germany the material was distributed to be planted at 20 experiments established in 13 countries. Due to the large number of populations, the complete set was split into 11 blocks of 100 populations each, which were assembled, based on a stratified sampling to contain material from the entire sampled area, approximately representing a comparable climate space. These blocks can be regarded as a complete trial by themselves and were assumed to be equal in mean and within-block variance, thus easily comparable (Krutzsch, 1992). For seven experiments all 11 blocks were established at one site (e.g., EXP 7, 8 in Sweden), while others were established at two (e.g., EXP 5 in Norway and EXP 16 in Scotland) or even up to a maximum of nine sites for EXP 15 in Germany. Trials were designed in a similar way with single tree plots planted in a randomized complete block design with 20 or 25 replicates, at a spacing of 2 × 2 m. One exception is EXP 16 in Scotland, where six trees are planted per plot in four replications. All trials were managed independently by the various partner institutions, according to their own guidelines and silvicultural practices.
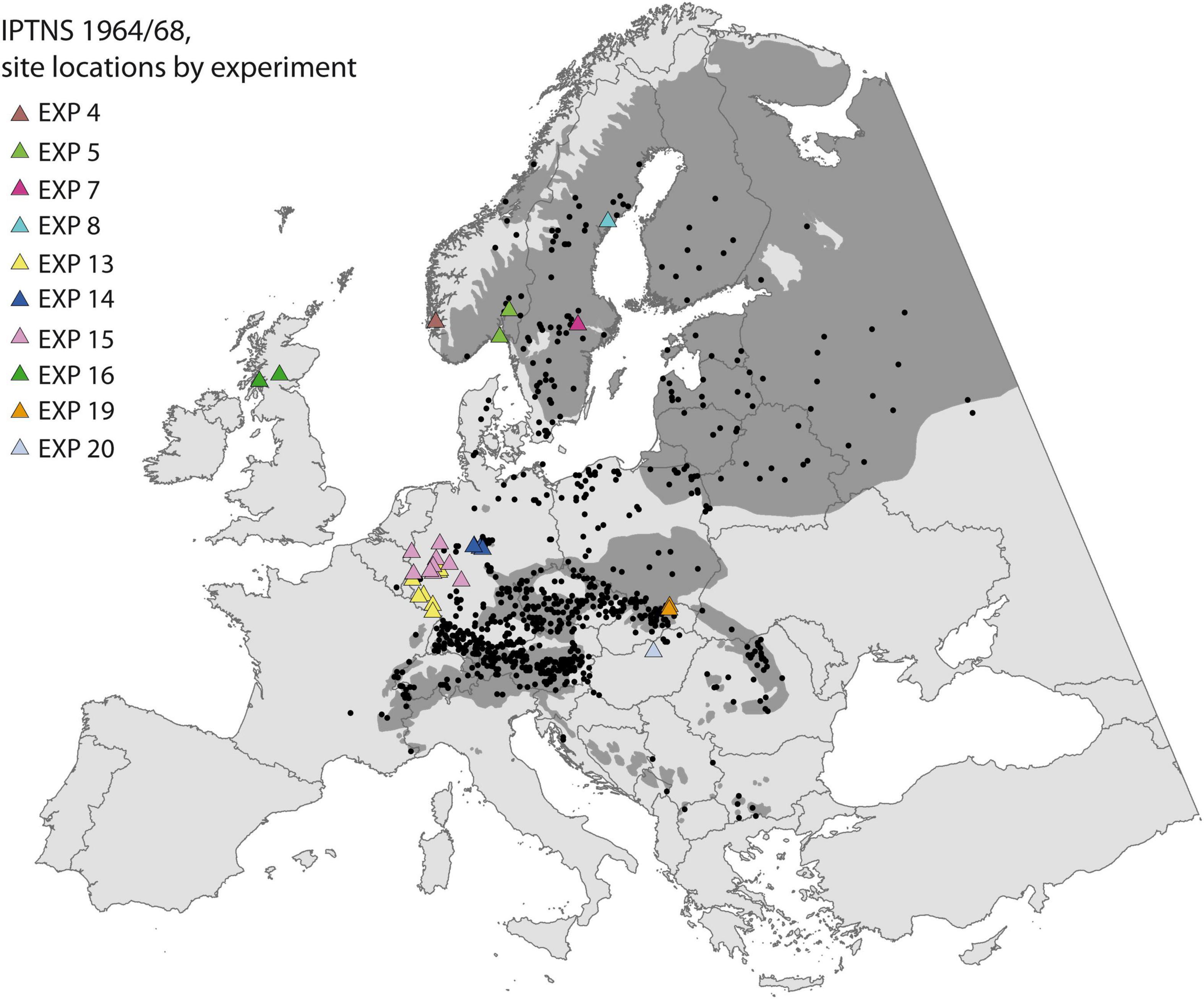
Figure 1. Sampled populations (black points) and trial sites (triangles, colored by correspondence to experiment) of the International Provenance Test 1964/68 of Norway spruce (IPTNS) used to calibrate the TraitSDM. The natural distribution of Norway spruce is shaded in dark gray (Skrøppa, 2003).
Measurements were taken at recurring intervals, but their timing varied among institutions for the same reason. Here, we concentrate at tree height as the most commonly assessed proxy for fitness, in which multiple ecophysiological traits affected by the environment are cumulated, e.g., taller trees compete more efficiently for light, water, and nutrients and are likely to have higher reproductive success at maturity (Aitken and Bemmels, 2016). We use juvenile tree height of 11 years available from 10 experiments, with different numbers of provenance blocks being distributed to a total of 32 site locations (97 blocks of 100 provenances) (Figure 1). Supplementary Table 1 provides further detail about site locations and reports the corresponding references to the used provenance mean data. Heights were either directly measured or interpolated to the common measurement age based on two concurrent measurements of a maximum age difference of 5 years (age 9–14 for EXP 20) using the following equation:
where HT = height, i and j = age of measurement, with i measured before j. The distribution of HT11 used in the analysis is displayed by trial site location in Supplementary Figure 1.
National Forest Inventories
Current occurrence of Norway spruce across Europe was obtained from EU-Forest by Mauri et al. (2017), the most comprehensive Dataset on European tree species occurrence up to date, which harmonizes national forest inventories from 21 European countries and aggregates them to an INSPIRE-compliant 1 × 1 km grid. All records of Norway spruce were treated as true presences (70,310 occurrences), while all other species records were summarized to a grid cell basis from which an equivalent number of pseudo-absences was selected (prevalence = 0.5). Similar to Iturbide et al. (2015), we first set an exclusion buffer of 10 km around the presence points, to then randomly select the pseudo-absences among the remaining target group absences. Barbet-Massin et al. (2012) found this to result in the highest predictive accuracy when using classification techniques.
Climatic and Geographic Data
Biologically relevant annual climate data was generated with the ClimateEU v4.63 software, publicly available at http://tinyurl.com/ClimateEU, based on methodology described by Marchi et al. (2020) (Table 1).
Climate parameters of provenance (suffix “p”) and test site (suffix “s”) for the calibration of the TraitSDM were extracted for different time intervals as described by Sáenz-Romero et al. (2019). The normal period of 1941–1970 was used for provenance climate characterizing the conditions to which the sourced provenances had adapted in the past. To characterize the climate of the test sites, mean values of climate estimates were calculated for the period 1968–1975. The OccurrenceSDM was calibrated with the widely used reference period 1961–1990 that largely predates a significant anthropogenic warming signal. For each of the three sets of predictor variables we performed a principal component analysis to inspect patterns of multicollinearity (Supplementary Figure 2).
Provenances were further characterized by geographic parameters to account for other factors affecting among population variation such as gene flow, genetic drift, or postglacial colonization in the TraitSDM that may result in spatial patterns independent of climate (Wang et al., 2010). These included latitude (LAT), longitude (LONG), and elevation (ELEV) and their transformations and interactions (i.e., LAT2, LONG2, ELEV2, LAT × LONG, LAT × ELEV, LONG × ELEV, (LAT × LONG)2, (LAT × ELEV)2, (LONG × ELEV)2, LAT2 × LONG, LAT2 × ELEV, LONG2 × ELEV, LAT × LONG2, LAT × ELEV2, and LONG × ELEV2).
For spatial habitat modeling of both the OccurrenceSDM and TraitSDM, we used 1 × 1 km resolution climate grids in Albers Equal Area projection. To make predictions for the 1961–1990 normal period, and for three projected time slices, the 2020s (2011–2040), the 2050s (2041–2070), and the 2080s (2071–2100) ensemble projections provided by ClimateEU were used. These ensembles average across 15 Atmosphere-Ocean General circulation models corresponding to the IPCC Assessment Report 5 (CanESM2, ACCESS1.0, IPSL-CM5A-MR, MIROC5, MPI-ESM-LR, CCSM4, HadGEM2-ES, CNRM-CM5, CSIRO Mk 3.6, GFDL-CM3, INM-CM4, MRI-CGCM3, MIROC-ESM, CESM1-CAM5, and GISS-E2R), representing all clusters of similar AOGCMs, and having high validation statistics in their CMIP3 equivalents (Marchi et al., 2020). Each time period is predicted for a Representative Concentration Pathway (RCP) with a radiative forcing value of +8.5 W/m2. This forcing value results in a projected global warming of approximately +2.0°C (±0.6) by the 2050s and +3.7°C (±0.9) by the 2080s (Marchi et al., 2020).
Occurrence-Based Species Distribution Model
We followed the ODMAP protocol proposed by Zurell et al. (2020) to build the OccurrenceSDM that forecasts species occurrence probability based on observed species presence from the described national forest inventory data. First, those climate predictors of Table 1, which explain most of the variation in observed presence and absence were identified by assessing univariate variable importance in terms of Akaike information criterion (AIC). If these variables showed issues of multicollinearity, as visible by their grouping in the PCA (Supplementary Figure 2), the less important variable was removed from each pair of variables having a correlation |r| > 0.8 (Dormann et al., 2013).
The OccurrenceSDM was fitted using an ensemble approach accounting for different assumptions and extrapolations to new environments by different algorithms (Araújo et al., 2019). We implemented five popular algorithms, namely generalized linear models (GLM), classification tree analysis (CTA), generalized boosted regression models (GBM), artificial neural networks (ANN), and random forest for classification and regression (RF) using the multi-method platform biomod2 (Thuiller et al., 2020) implemented in R statistical environment (R Core Team, 2019). Default settings that are designed to balance model complexity and overfitting were used for all modeling algorithms, despite for GLM, where we specifically allowed pairwise interactions. Model output were occurrence probabilities, which were then ensembled to a consensus model by combining the median probability over the selected models. The median was chosen as it is known to be less sensitive to outliers than the mean. For each model as well as the final ensemble, true skill statistics (TSS), area under the receiver operating characteristic (ROC), model sensitivity (ability of the model to predict true presence), and model specificity (ability of the model to predict true absence) were recorded. To account for model uncertainty, the data was partitioned to 75% of the data to train the model while 25% was retained for model testing. This was done for three independent runs, each with a randomly selected set of training and testing data. For each model run model evaluation statistics were recorded and finally averaged for reporting.
The variable importance of the climatic predictors was assessed by an internal function of biomod2, which relies on the comparison of model predictions derived from the original dataset with predictions from permutated datasets (Thuiller et al., 2012, 2020). Permutated datasets are created by randomizing one environmental variable at the time, while keeping all others as original to then create a new prediction. Predicted occurrence probabilities resulting from the original dataset and the permutated dataset are then compared by calculating a Pearson correlation coefficient. A small correlation coefficient indicates a high importance, i.e., the predictor variable strongly affects the prediction, while a high coefficient indicates minor importance. This procedure was repeated three times for each model run and mean correlation values were derived across the three independent model runs. The finally reported variable importance corresponds to 1 minus the average correlation coefficient, so that high values reveal a high importance and low values a low importance. This procedure was repeated for each predictor variable and model algorithm.
Trait-Based Species Distribution Model
To identify the climatic conditions of trial site location and provenance origin that are the main drivers of growth performance across the species range, we used a URF according to Wang et al. (2010). This function basically integrates response functions, being reaction norms of individual provenances to environmental variation, and individual transfer functions that express among population variation as genetic clines into a single model. In addition, other factors such as gene flow, demographic history and colonization events can have a considerable effect on among-population variation resulting in spatial patterns independent of climate. As stated by Wang et al. (2010), “these spatial genetic effects may be explained to some extent by geographical proximity of provenance, assuming population proximity is correlated with relatedness, and shared evolutionary history,” and were further included to the function. The mean tree height HT of population j at site i was fitted according to Equation 2:
where β represents the intercept and regression coefficients, Si and Pj are one or more climate variables for site i and provenance j, respectively, Si×Pj is the interaction between Si and Pj, Gjis one or more geographic variables of provenance j, and eij is the residual error.
Multiple regression models were constructed using different variable combinations with a maximum of two climate variables for site and provenance and one additional geographic variable for provenance. We removed models showing high collinearity between climatic variables (Pearson coefficient r ≥ 0.70) and approved that the quadratic terms were of convex form (decreasing tree height towards both ends of climatic gradients), which is biological plausible and allows to estimate provenance climatic optima (β2 < 0, β4 < 0, or β7 < 0). Significance levels for the predictors to stay in the model were set to α ≤ 0.001. Finally, we selected the best model based on the highest proportion of variance explained by partial R2 and lowest AIC.
In order to validate the derived model, it was used to predict height growth performance for a set of independent data. These were 15 additional trial site locations (47 blocks of 100 provenances) of the IPTNS 1964/68 series, corresponding to seven experiments from six countries (Austria, Belgium, Czech Republic, France, Norway, and Sweden) that were not included in model calibration due to a different age of the available data (age 9, 10, 12, or 14 depending on trial site, Supplementary Table 1). Predicted heights were compared to observed tree heights using Spearman’s rank correlation as proposed by Chakraborty et al. (2016).
Spatially explicit predictions at the continental scale are derived according to the procedure described by Wang et al. (2010). Predictions are made once using “local” provenance, i.e., provenances originate from the climate conditions that are the same as at the planting site, as well as “optimal” provenance, where the, according to the provenance term in the model, best performing provenance is assumed to be planted. Growth of local provenance under current climate is predicted by setting values for site climate in the URF equal to provenance climate (TD_p = TD_s). To estimate growth under the three future scenarios (2020s, 2050s, and 2080s) current local climate is used for provenance and future climate for site. To identify the best performing provenances under reference and future climate conditions, the first order partial derivatives of the URF were drawn for climatic and geographic variables of provenance. These partial derivatives were set to zero and then solved to obtain the values for the chosen variables of the provenance that yields greatest growth at the site, i.e., at any given grid cell of the study area (Wang et al., 2010). As a log-transformation was applied on the response variable height for model fitting, predictions were back transformed prior to spatial mapping.
Species Range Change
To translate occurrence probability from the OccurrenceSDM to binary presence/absence we identified the maximum sum of sensitivity and specificity as optimal threshold (Liu et al., 2005).
The TraitSDM predicts growth performance, which may not be directly compared to binary species occurrence and therefore requires conversion. Predictions were translated to binary presence/absence applying a threshold surface accounting for decreasing growth potential towards the margins (high elevations, northern, and southern edges) of the distribution. For example, average performance on trial sites in western Germany is over 2 m at age 11, while only about 0.5 m at the northern most site in Lappkojberget, which needs to be accounted for when translating predictions of height growth potential into species presence and absence. Therefore, we applied a productivity-oriented threshold according to the following procedure: First, all provenance means were standardized and a quadratic regression was fitted based on geographic latitude and elevation. Elevation was only used as linear term, as a negative linear cline with increasing elevation is commonly observed. The derived regression was then applied spatially, giving each pixel across the landscape a specific threshold value according to its latitude and elevation. Height growth predictions by TraitSDM were likewise standardized and compared to the threshold value to segregate presence (higher than threshold) and absence (lower than threshold).
Finally, the spatial extent of species presence predicted by different models (OccurrenceSDM and TraitSDM) and projected time slices (2020s, 2050s, and 2080s) was compared to a common baseline: the spatial extent of the binary presence for the reference period 1961–1990 as modeled by the OccurrenceSDM. The change in species range was quantified with the biomod2 built-in function BIOMOD_RangeSize().
All statistical analysis was conducted in the R programming environment for statistical computing and visualization (R Core Team, 2019).
Results
Habitat Predictions by Occurrence-Based Species Distribution Model
After the inspection of multicollinearity in the predictor variables of the presence/absence data, four weakly correlated climate variables remained for fitting the OccurrenceSDM, namely climate moisture deficit (CMD), mean coldest month temperature (MCMT), mean driest month precipitation (MDMP), and the temperature difference between mean warmest and coldest month (TD) as indicator of continentality. MCMT and CMD contribute strongly to correctly predicting presence and absence in the forest inventory data, with GLM, CTA, and GBM depicting CMD as most relevant followed by MCMT, while it was the opposite for the algorithms ANN and RF (Table 2). Partial response curves are provided in Supplementary Figure 3 illustrating the sensibility of each algorithm to the climatic predictors. The predictive performance of the models was excellent with both training and testing data, with mean TSS ranging from 0.87 to 0.95 and mean ROC ranging from 0.97 to 0.99 (Table 3).
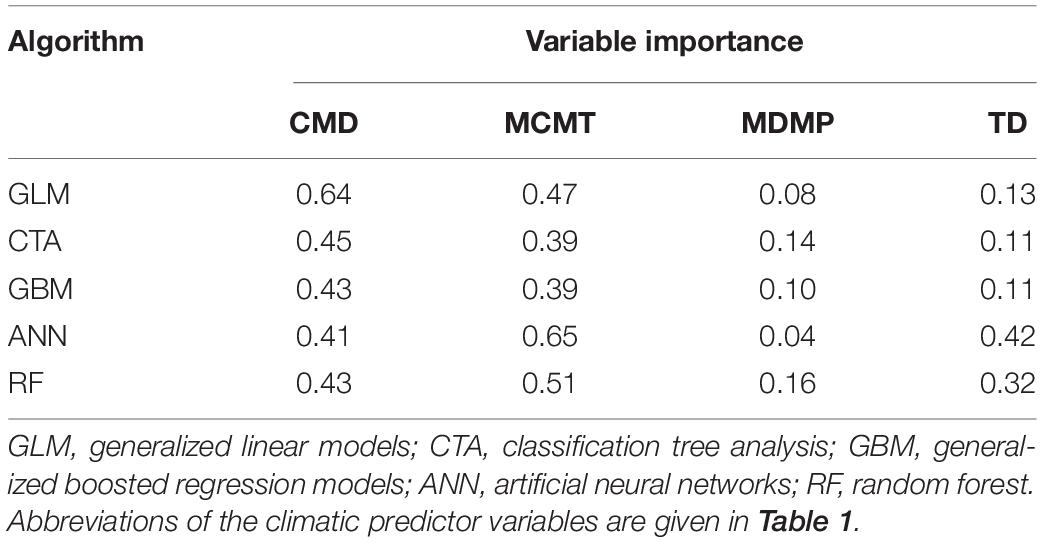
Table 2. Variable importance by algorithm of the climatic predictor variables used in the OccurenceSDM.
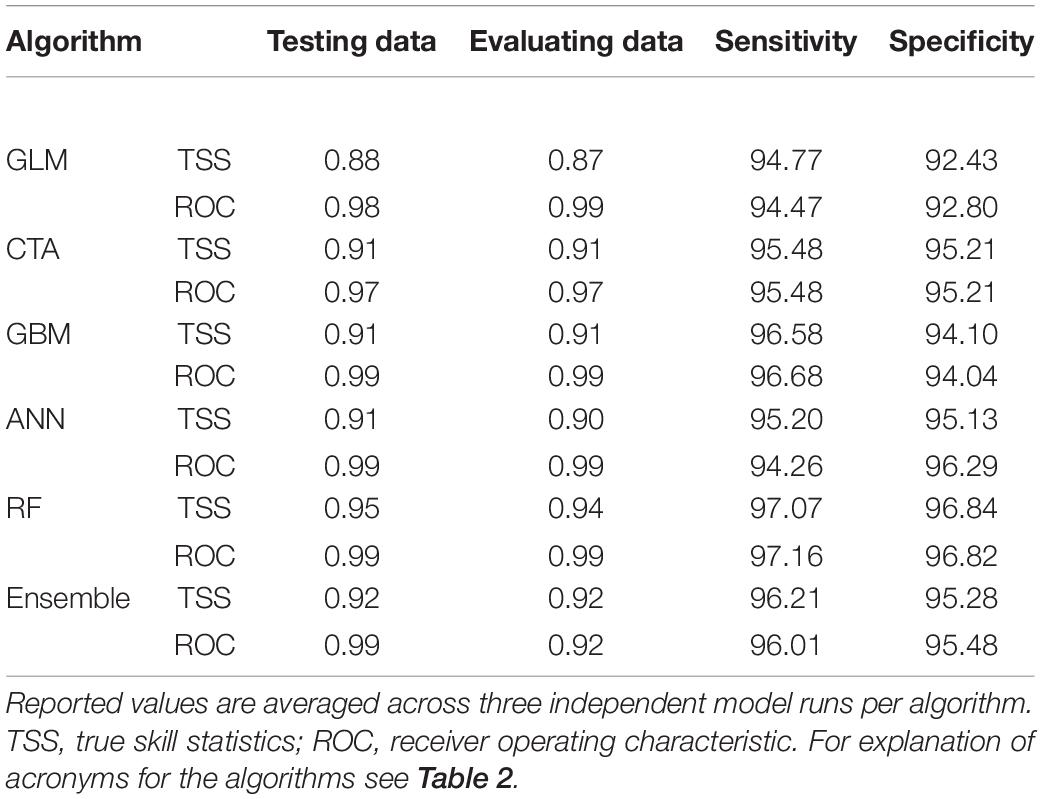
Table 3. Statistics of evaluation for the OccurrenceSDM ensemble, and each of the five individual algorithms used to develop the ensemble.
According to the OccurrenceSDM, the range of Norway spruce decreases for all predicted climate periods (2020s, 2050s, and 2080s) as compared to the reference period, first back towards the extents of the natural distribution and thereafter even further (Figure 2). Habitat suitability declines particularly in Southern, Central, and Eastern Europe, whereas it remains stable in the in the North (>60°N) and at higher elevations (e.g., in the Alps).
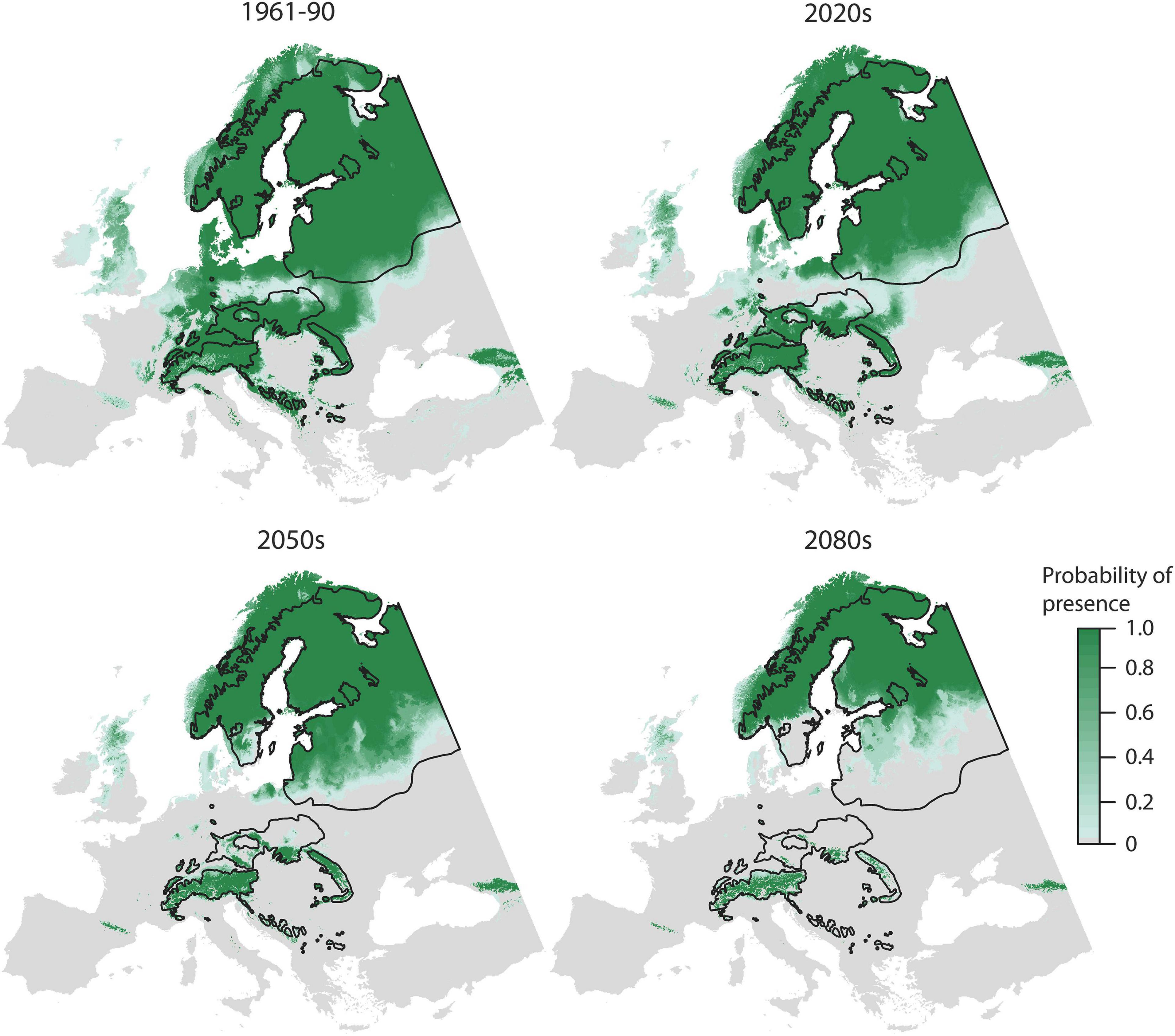
Figure 2. Probability of presence according to OccurrenceSDM for the 1961–1990 reference period and three projections for the 2020s, 2050s, and 2080s. Black lines indicate the natural distribution.
Growth Predictions by Trait-Based Species Distribution Model
From the 14 climate variables tested for their performance in predicting height growth, two variables of the trial site, one climatic and one geographic variable for the provenance, as well as one interaction between site and provenance climate were found to be significant predictors (Table 4). The iterative process of comparative model selection is illustrated in Supplementary Table 2 (step 1–7). The final model explained 75.8% of the variance in the data and included the length of the frost-free period (FFP_s), which can be considered an indicator for the length of the growing season, as well as the summer-heat-moisture index (SHM_s). For provenance, it included the temperature difference between warmest and coldest month as indicator of continentality (TD_p) and the geographic latitude (LAT_p). As interaction term FFP_s × TD_p stayed in the model (the second interaction term tested SHM_s × TD_p was rejected as it did not fulfill α ≤ 0.001). Site climatic variables explain large amounts of the variation in tree growth with 82.7%, while 16.8% is explained by geography and climate of the provenance. Herein, provenance climate could only capture a limited proportion of 2.2%, whereas provenance latitude and its squared term shape a great proportion of the observed variation with 14.5% (Table 4). Despite being significant the interaction term between site and provenance climate accounts for less than 1%.
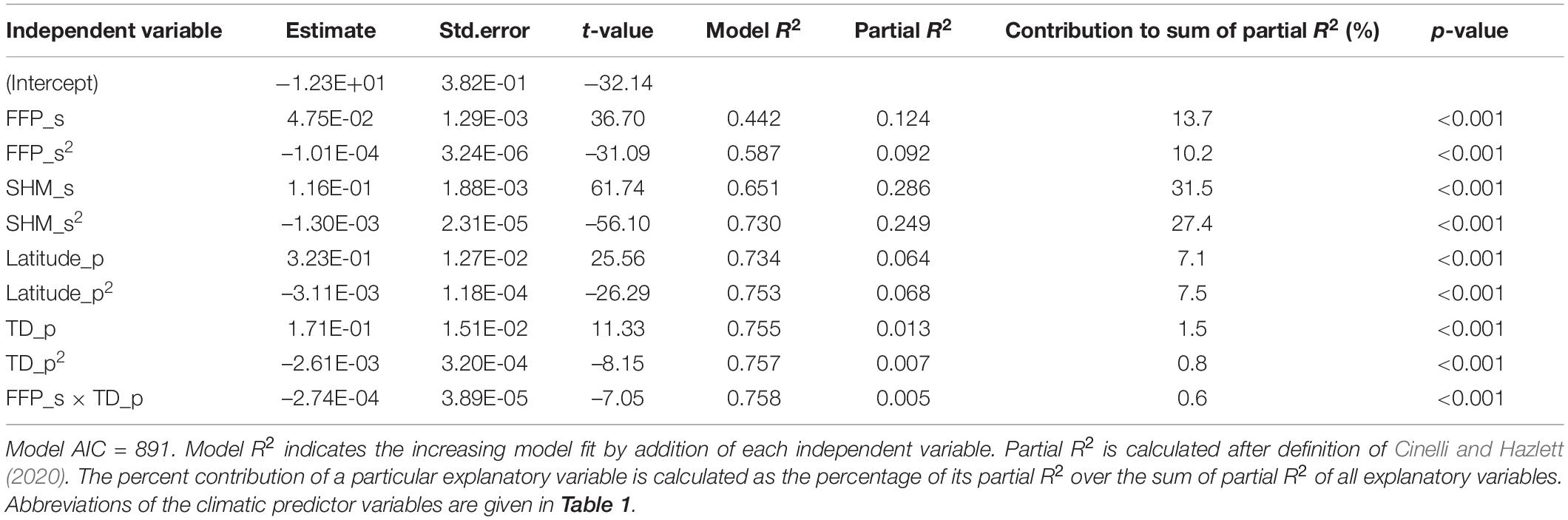
Table 4. Summary statistics of the TraitSDM predicting tree height of Norway spruce populations at age 11 based on climate of site, as well as climate and geography of and provenance origin.
The model was evaluated with independent data from 15 locations (47 blocks of 100 provenances), well distributed across the study area (Supplementary Figure 4). Spearman’s rank correlations between predicted and observed provenance height had positive coefficients ranging between 0.06 and 0.70, with r > 0.5 for 29 blocks of 100 provenances. The full model (equal to Table 4) performs moderate to well for sites located within the southern center of the natural distribution, those close to the western border of spruce’s artificial distribution in northern France and Belgium, as well as for the southern edge of Norway. However, model performance is poor for Hjuleberg in Southern Sweden (r < 0.4 for six of 11 provenance blocks). The combination of geographic and climatic variables for provenance in the model improved the predictive power of the model, as illustrated by the comparison with two reduced models that exclude either provenance climate or provenance geography from the full model (Supplementary Figure 4).
Predictions of juvenile height based on the TraitSDM are illustrated in Figure 3. All areas with a positive height growth potential basically represent the fundamental niche of Norway spruce, i.e., the potential distribution of the species being restricted by climatic constraints. For the reference period 1961–1990 it covers the greatest extent, with large parts of this potentially inhabited area being located outside the natural distribution. Height growth performance is particularly high in the center, while it decreases towards high elevations and the margins. Areas above 60°N are characterized by a sharp growth decline (<70 cm), while countries along the east side of the Baltic sea (Estonia, Latvia, and Lithuania) and large parts of Central and Eastern Europe (Poland, Germany, Czech Republic, Slovakia, Hungary, and Belarus) are predicted to have superior growth (>200 cm). With changing climate conditions there is a substantial decline in height growth potential across the study area, particularly for areas of low elevation. Former disjunctions (e.g., the Polish disjunction) do again become a spruceless belt. The range with superior height growth >200 cm retreats towards the natural distribution and further within (from 0.87 × 106 km2 for 1961–1990 to 0.08 × 106 km2 by 2080s, −91%, Table 5). This also applies for the southeastern part of the Baltic part of the species distribution, while some small improvements in height are predicted for areas above 60°N. Higher elevations, e.g., in the Alps or Carpathians will profit from climate warming by an increased growth potential.
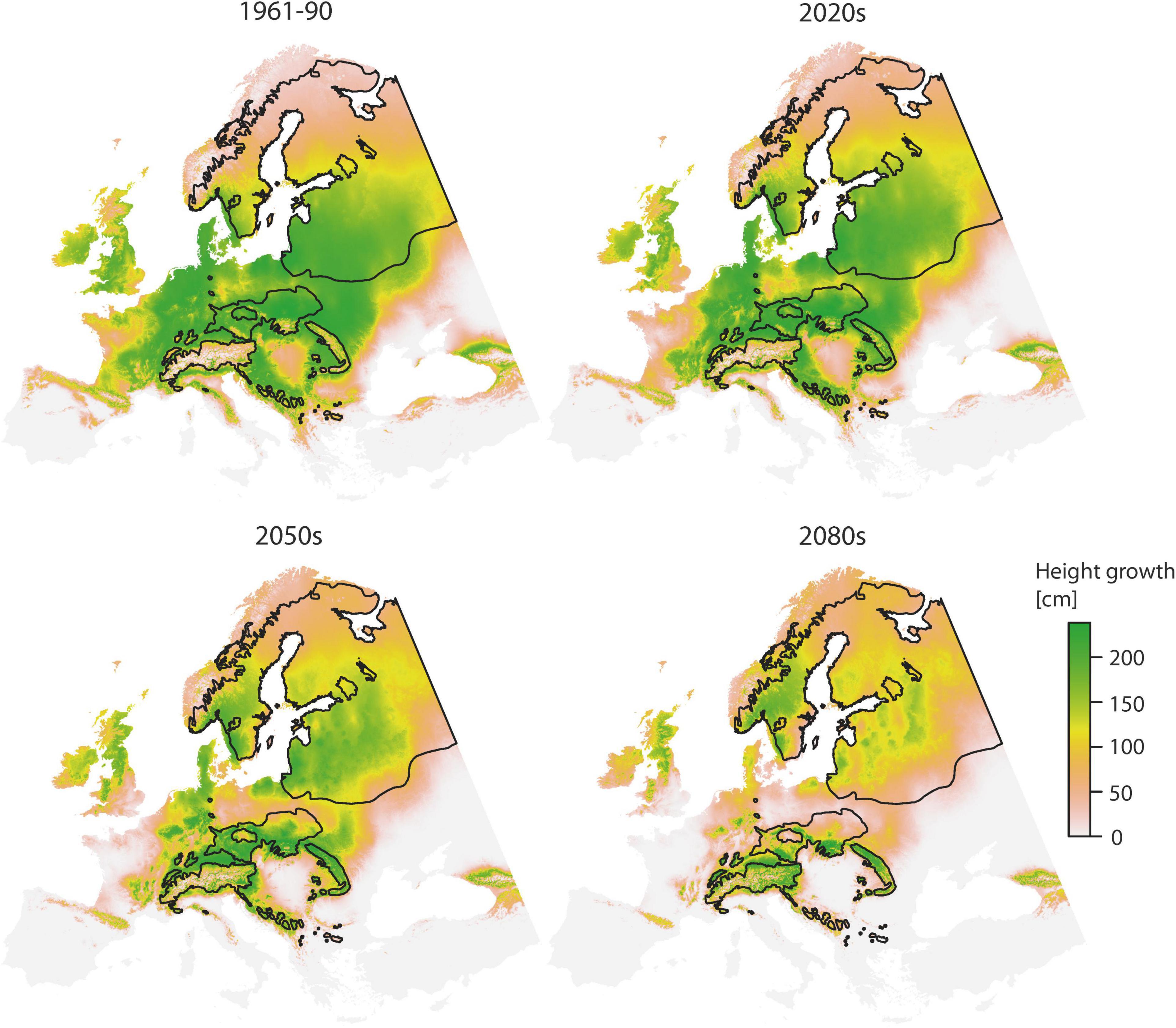
Figure 3. Spatial prediction of the TraitSDM for height age 11 using “local” provenance for the 1961–1990 reference scenario and three predicted scenarios for the 2020s, 2050s, and 2080s. Black lines indicate the natural distribution.
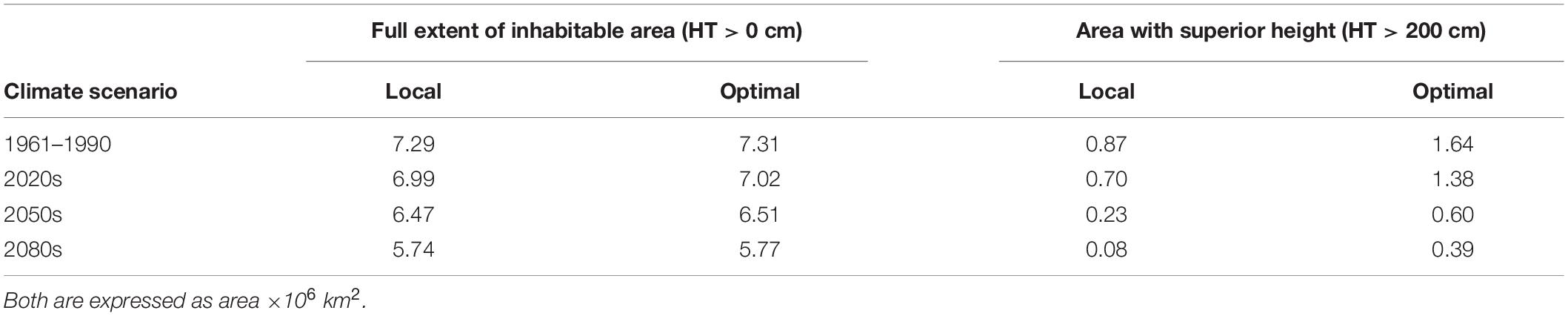
Table 5. Full extent of the area potentially inhabitable for Norway spruce according to the TraitSDM and the area within expected to show superior juvenile height growth potential ≥200 cm.
Choosing “optimal” provenances has a positive effect on height growth (Figure 4). Towards the second half of the century, potential height gains are particularly prevalent at high latitudes. Compared to the first half of the century, total area where height gains may be achieved will then decrease (Table 5), but the absolute gain will increase (Figure 4). By the 2080s, it will be possible to achieve superior height growth on an area of 0.39 × 106 km2, if provenances were chosen according to the derived model. However, these areas are mainly restricted to the higher elevations and the north.
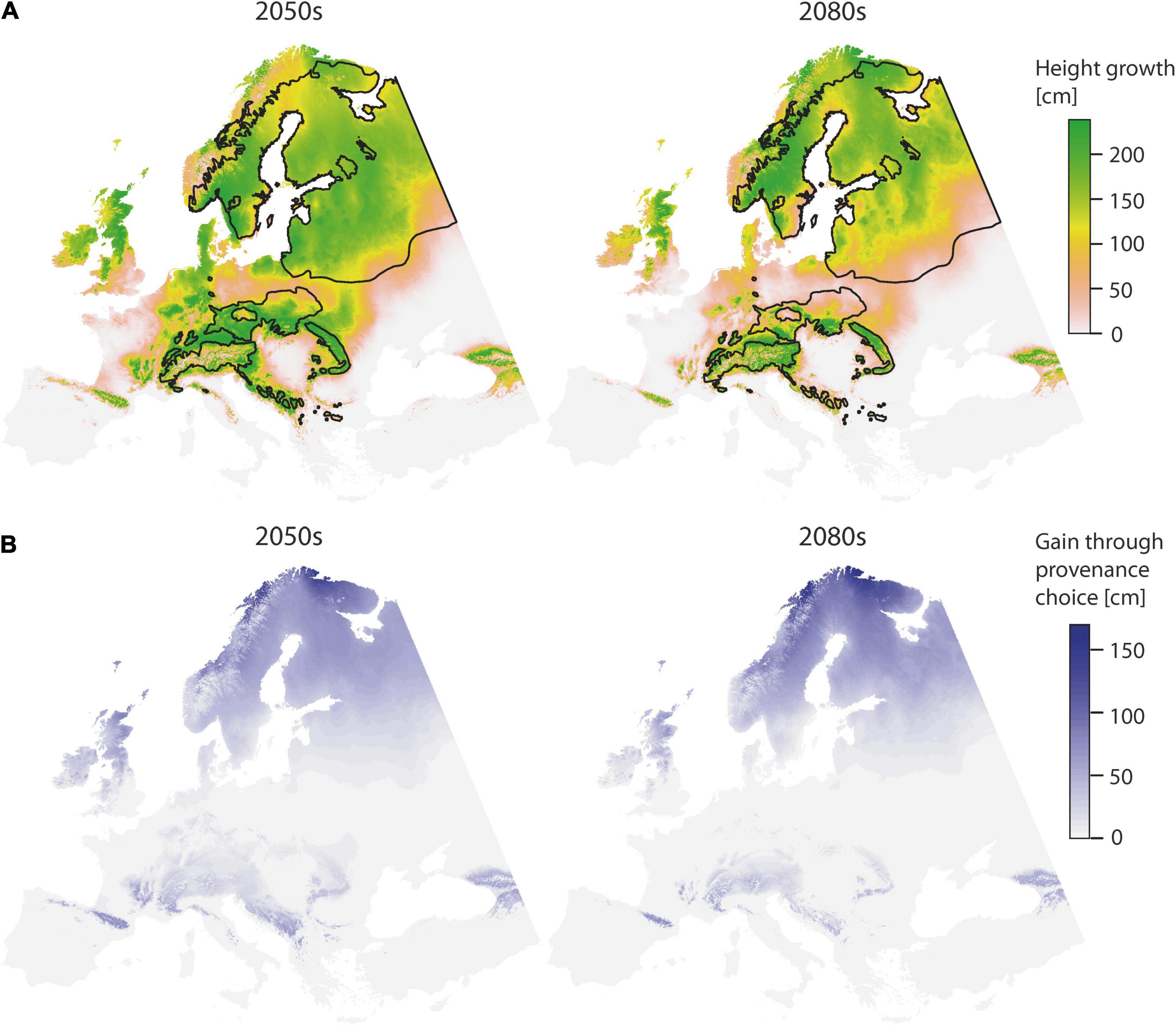
Figure 4. Spatial prediction of the TraitSDM for height age 11 using “optimal” provenance, as identified by solving the first order partial derivatives for climatic and geographic variables for provenance in the model (TD_p and Lat_p), for the predicted scenarios 2050s and 2080s (A). The potential of height gain through model-based provenance choice is indicated (B). Black lines indicate the natural distribution.
Comparison of Species Range
For the ensemble OccurrenceSDM, the optimal threshold (based on true skill statistics) to translate occurrence probability to binary presence/absence corresponds to a probability of 0.412. Growth potential predicted by the TraitSDM is likewise translated using a productivity-oriented threshold (surface shown in Supplementary Figure 5), which assigns a specific threshold value to each grid cell according to its latitude and elevation.
The largest suitable habitat according to the OccurrenceSDM is predicted for the reference period 1961–1990, covering an area of 3.95 × 106 km2, that is used as common baseline scenario (Table 6). During this time period, the TraitSDM identifies an area of 5.24 × 106 km2 as suitable habitat for “local” provenances, which is 15.9% more than the baseline scenario. The 10.3% range loss compared to the OccurrenceSDM do represent mainly subalpine and alpine zones in the Alps that become unsuitable due to their low productivity and at the south-east facing range margin in Russia, which the TraitSDM predicts to run slightly shifted northwards. On the other hand, it indicates a range gain of 26.2%, with a potential spread to the Massif Central in France and further south to the Pyrenees, as well as some additional habitat along the Dinaric Alps and the Eastern Carpathians. With forthgoing climate change the border of suitable Norway spruce habitat climbs up in elevation, as growth potential increases.
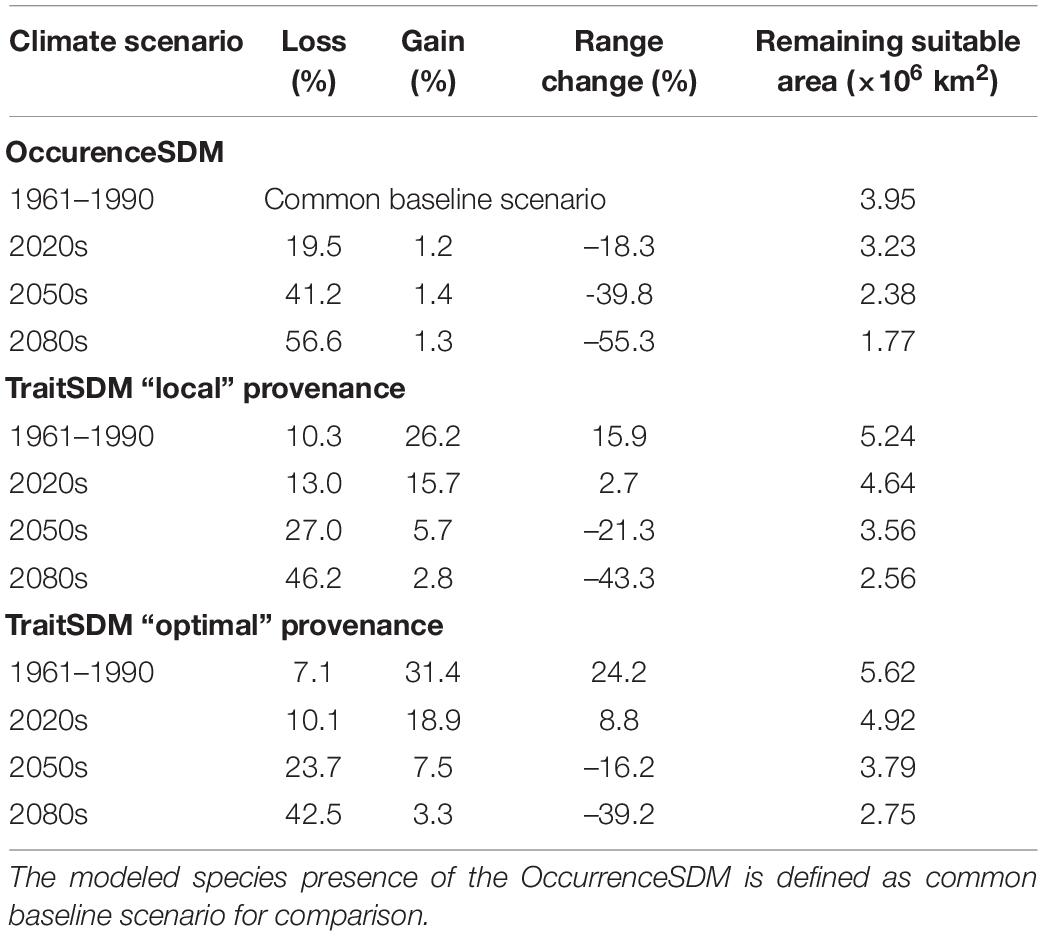
Table 6. Species range change predicted by OccurrenceSDM and TraitSDM using “local” or “optimal” provenances.
Range size of both OccurrenceSDM and TraitSDM decrease over time, facing the same direction with a retreat towards the higher elevations and the northern latitudes. The former does already decrease by 18.3% in the 2020s (Table 6), while the latter has approximately the size of the baseline scenario to then pass the baseline extent shortly after. According to the OccurrenceSDM Norway spruce will lose 39.8% of its current range by 2050s and as much as 55.3% by the 2080s. This equals to an area that remains suitable of approximately 1.77 × 106 km2. The retreat of suitable area proceeds likewise according to the TraitSDM with a remaining suitable area of 2.56 × 106 km2 in the 2080s (−43.3%). With only 1% range gain for each time slice predicted by the OccurrenceSDM, the species will not be able to expand to new suitable habitats, as those are, for example, restricted by the northern edge of Scandinavia.
If there had been a model-based provenance transfer during stand establishment in the past using “optimal” provenance, the species range might have been even 24% larger than the baseline scenario 1961–1990. Choosing “optimal” provenance for future stand establishments may lead to substantial increase in height growth potential (Figure 3), however, this has only a marginal effect to slow down species range change. By the 2080s the area potentially occupied by Norway spruce will also be 39% smaller (approx. 2.75 × 106 km2 remain suitable).
Discussion
Phenotypic Plasticity Largely Determines Height Growth
Site climatic variables contribute 82.7% to partial R2. This is a strong indicator for a particularly high phenotypic plasticity in height growth of young Norway spruce. The two most important drivers of the plastic response identified by the TraitSDM are the frost-free period (FFP_s) and the summer heat-moisture-index (SHM_s). The quadratic pattern with SHM shows great growth declines towards both ends of the quadratic response within the climate range of observed sites. Low values of SHM generally represent cold and moist conditions, while high values imply warm and dry conditions. In essence, increasing temperatures paired with decreasing water availability, particularly during the summer, do strongly restrict future growth potential of Norway spruce (Figure 3). The consequences of this effect were already noticed in the species’ reaction to drought events, where it shows low resistance and resilience (Vitali et al., 2017). According to projections of future climate, SHM will greatly increase in the second half of the century, with a negative effect on Norway spruce, as conditions will surpass the maximum of the quadratic relationship (SHMmax = ∼45) after which height growth will drop quickly. Sites with an SHM exceeding 85, e.g., large parts of the North European Plain, become completely unsuitable for Norway spruce. Kapeller et al. (2012) likewise identified a heat moisture index, i.e., the annual heat moisture index, as best variable to predict tree height applying response functions for aggregated clusters of Austrian provenances. They hardly found areas throughout Austria, where conditions will be already too warm and too dry for Norway spruce by the 2080s. This is corroborated by our observation that the alpine mountain ranges, including Austria, will remain suitable for Norway spruce into the 2080s.
For these areas, FFP_s has an additional positive effect. With an increasing length of the frost-free period, height growth potential will increase, which is particularly noticeable at the higher elevations and the northern latitudes. Biologically this may relate to bud phenology in spring, which is largely driven by heat sum accumulation in Norway spruce (Hannerz, 1999). If the frost period ends earlier and conditions get warmer, required heat sums are fulfilled earlier and buds break. As budset on the other hand is mainly driven by the photoperiod, which remains constant, the length of available growing season between budbreak and budset increases, resulting in a higher growth potential. Considering the climatic range of the test sites included in our analysis, Norway spruce has just reached the upper limit at which a further extending frost-free period may not provide further benefit.
The observed dominant effect of environmental variables on the variation in height growth has been reported previously for Norway spruce (Chakraborty et al., 2019a,b) as well as for other conifer tree species (Wang et al., 2010; Rehfeldt et al., 2014; Chakraborty et al., 2015). Despite this, these studies still identified a noticeable effect of local adaptation to provenance climate. Chakraborty et al. (2015) could attribute a contribution of 18% of the observed variation in height at age 24 to the mean annual temperature of provenance for Pseudotsuga menziesii, and Wang et al. (2010) attributed 21% to the same climatic variable for Pinus contorta. In our TraitSDM of Norway spruce, provenance climate was found to have only a minor influence (partial R2 = 2.2%). Provenance latitude, however, had a much larger effect (partial R2 = 14.5%) and may be interpreted as a sign of local adaptation as well.
The geographic latitude of the provenance closely resembles patterns of local adaptation in the northern part of the distribution where latitudes are >55°N (Supplementary Figure 6), but fails to picture the high observed variation in height growth for the southern part of the range. We interpret this as an effect of the post-glacial migration history of the species. Fossil pollen (Latałowa and van der Knaap, 2006) and genetic analysis [RAPD (Collignon et al., 2002); mtDNA (Tollefsrud et al., 2008)] suggest postglacial colonization from at least three geographically distinct refugia in the present-day area of Moscow, the Carpathians and the Dinaric Alps. From these starting points, the so-called Baltic, Hercyno-Carpathian and Alpine domain colonized the European continent. Tollefsrud et al. (2008) explain the high population differentiation along the pronounced latitudinal gradient in the Baltic domain as a result of post-glacial migration in form of a front-like spread at high population density, paired with repeated bottlenecks resulting in low within population diversity. Considerable differences in the annual amplitude of the photoperiod (up to 8 h between 55° and 65°N) strongly shaped natural selection along the way (Collignon et al., 2002). The southern range of the species is dominated by the Central European mountain ranges that compromise migration. The Western Alps are characterized by decreasing genetic diversity at relatively short distance, as high mountain ridges and dry valleys acted as physical barriers and competing species introduced from the north (Fagus) and south (Abies). In contrast to this effect stand genetic patterns in the West Carpathians and the Bohemian Massif with low differentiation among, but high within population variation, which are most likely the result of admixture with high levels of geneflow between different colonization routes (Tollefsrud et al., 2008).
Despite these strong effects of postglacial migration mirrored in the model by the geographic latitude, the continentality (TD_p) appeared to be the most important provenance climate variable in predicting juvenile tree height. It significantly interacts with the frost-free period, which basically represents a form of genotype × environment interaction. Populations originating from milder climate (smaller difference between mean warmest and mean coldest month temperature) grow better at sites with a longer frost-free period, while those of harsher climate are better suited for sites with a short frost-free period, e.g., in Central Sweden.
Occurrence-Based Species Distribution Model Underestimates Species Range Predicted by Trait-Based Species Distribution Model
Generally speaking, the TraitSDM estimates the fundamental niche, the full range of environmental conditions that a species can occupy and use, without any other limiting factors such as competition, biotic threats, or dispersal barriers. The size of the predicted fundamental niche of Norway spruce appears quite large with 7.29 × 106 km2 (Table 5 and Figure 4), and largely exceeds the suitable habitat predicted by the OccurrenceSDM. However, the OccurrenceSDM basically predicts the realized niche, making this direct comparison inappropriate. This has been recognized previously by Chakraborty et al. (2019b), who used true skill statistics to identify a single value as optimal height threshold segregating presence from absence. While this approach worked well in their case for Pseudotsuga menziesii, an introduced species in Europe and mainly distributed at the low elevations, it was not expedient here for Norway spruce, a species naturally associated with the harsh, cold, and moist conditions of the higher elevations and latitudes. By the use of a regression surface as threshold (Supplementary Figure 5) to approximate the realized niche, we were able to account for the different site potential and, naming just one example, could successfully identify Northern Scandinavia as suitable habitat despite the species’ low growth potential in this area.
Comparing the approximated realized niche of the TraitSDM with that of the OccurrenceSDM, the latter does underestimate the range Norway spruce may actually inhabit thanks to its high phenotypic plasticity. This high plasticity, paired with the ease of propagation, made the species the most preferred option to reforest degraded areas over the last two centuries (Jansen et al., 2017), which has significantly enlarged the species range. Still, our TraitSDM identifies an area of +16%, where there might have been further potential for range expansion in the past (Table 6). Obviously, Norway spruce will not realize this further range expansion any more now. However, when it comes to the future, the range decline may be delayed. According to the OccurrenceSDM Norway spruce will lose as much as 55% of its range by the 2080s, while it will lose only 43% according to the TraitSDM. This difference translates to a remaining suitable area of 2.56 × 106 km2 instead of only 1.77 × 106km2.
Despite this difference, both models do agree on a substantial range decline, first back towards the extents of the natural distribution and then further within. The general direction and spatial pattern of this decline to the north, i.e., towards the Boreal forest, and the high elevations closely resembles the predictions from other SDMs, regardless their use of different sets of predictor variables, global climate models, or even species occurrence data (van der Maaten et al., 2017; Dyderski et al., 2018; Chakraborty et al., 2021).
Limitations of the Trait-Based Species Distribution Model
Despite the fact that a broad application of TraitSDMs across species would provide great benefit to support forest management decisions, they are limited by the availability of provenance trials spanning large geographic gradients (Urban et al., 2016). It is crucial to use data of the same age, which greatly restricts their application. To date, very few comparable studies were able to assemble even aged datasets of age 20 (Wang et al., 2010) or older (Chakraborty et al., 2015), while most studies use observations available from younger age (Leites et al., 2012; Gárate-Escamilla et al., 2019; Hu et al., 2019). In our case the most abundant comparative data is height at an age of 11 years, which is relatively low compared to a full rotation of Norway spruce between 50 and 120 years (rotation length largely depends on site potential, planting material, and management recommendations; e.g., Felton et al., 2017). However, juvenile growth is considered to be closely connected to fitness, as intraspecific competition among trees is strongest among seedlings and juvenile trees (Kapeller et al., 2017). Further, Norway spruce shows strong age-age correlations, which makes it a suitable candidate for early selection (Isik et al., 2010). Bałut and Sabor (2002) found stable provenance reactions between 11 and 25 years at the polish site of the IPTNS 1964/68 series. And at an unthinned site in Germany, provenance ranks were found to be consistent for an even longer time period. Correlations between height of age 10 and DBH of age 51 – an age difference of 41 years – still had a coefficient of 0.75, while they ranged between 0.83 and 0.98 for shorter time intervals (Liepe et al., 2020).
Norway spruce has been largely translocated during past reforestation waves, far beyond the natural distribution, but likewise within (Jansen et al., 2017). Artificial populations were included in the presented trial series on purpose, as it was intended as an inventory to “test as many provenances as possible, regardless whether the seed sources were autochthonous or not” (Krutzsch, 1974). This likely introduces some bias to the model as artificially translocated populations may have only adapted for one generation to the climatic conditions at seed source location. While it is impossible to identify artificially planted populations within the natural distribution, those located outside may be spotted in the data and removed easily. However, when fitting a TraitSDM using a dataset reduced by those artificial populations, we identified the same climatic predictor variables (analysis not shown), and therefore do present here the analysis using the complete set of provenances.
The two statistical models used in the present study only consider climatic effects as potential drivers and delimiters of height growth potential and species occurrence. However, it is expected that other disturbance agents will strongly limit successful cultivation of Norway spruce. Drought stress causes sharp decreases in vitality (Marini et al., 2017), significantly lowering tree resistance against the colonization by bark beetles (mainly Ips typographus) (Schuldt et al., 2020). These do by themselves already profit from warmer temperatures with an earlier propagation in spring and subsequent exponential increase in population size. In addition, devastating wind throw events are expected to become more frequent (Gardiner et al., 2010), but their timing and strength are unpredictable.
Apart from climatic or climatically induced effects, population demography, structure and dynamics, dispersal, and competition with other species significantly shape future species distributions. Process-based models that simulate these aspects based on observations on individuals in natural populations or experiments have been proposed as biologically more realistic (Morin et al., 2007). However, these models are very “data-hungry,” limiting their broad application (Guisan and Thuiller, 2005). In case of our study species, Takolander et al. (2019) observed a high accordance between predictions of a process-based dynamic vegetation model and those of a bioclimatic envelope model. Further, their predictions closely resemble our predictions, allowing the assumption that the OccurrenceSDM reflects the processes responsible for niche realization close enough.
Implications for Forest Management
In the past, Norway spruce was planted far beyond its ecological niche limits (Figure 2, 1961–1990). In the future, the suitable range will decrease drastically towards the natural distribution and further within (Figures 2, 3 and Table 5). Norway spruce will be limited to a restricted area, remaining mainly at the high elevations and latitudes, which basically represent its core habitat in the boreal and subalpine conifer forests (Schmidt-Vogt, 1977; Caudullo et al., 2016). Here, form the practitioners point of view, Norway spruce may actually profit from the effects of climate change with better growth potential, as growth is promoted by the extending frost-free period, and there is still room for improvement in terms of an increasing summer-heat-moisture index (cf. Kapeller et al., 2012).
The presented TraitSDM was also used to identify “optimal” populations solving the partial derivatives for the provenance variables in the model. The height gain indicated in Figure 4 quantifies the potential merits of assisted migration, which has been proposed as key forest management strategy to mitigate negative impacts of climate change on the one hand, while making use of the potential opportunities for increased growth associated with warmer climate on the other (Pedlar et al., 2012; Williams and Dumroese, 2013).
Solving the model for latitude of the provenance, one particular area is identified to provide optimal growth across planting sites, i.e., the northern edge of the Hercyno-Carpathian domain. Provenances from this area do actually frequently stand out by their superior growth vigor, e.g., on sites of the presented IPTNS 1964/68 trial series (Liesebach et al., 2001; Ujvári-Jármay et al., 2016; Liepe et al., 2020), but likewise in numerous other provenance trials (e.g., Rau, 1983; Schmidt-Vogt, 1986; Giertych, 2007; Zeltinš et al., 2019). Further the interaction between provenance continentality and frost-free period affects the choice of the “optimal” provenance, in a way that provenances from milder, less continental climate than currently observed at the planting location are assigned as the frost-free period extends.
Our TraitSDM does suggest a movement of provenances northwards to enhance growth in the northern part of the distribution. Such a movement has already been successful in the past, for example, with provenances translocated from the Carpathians to southern Sweden achieving superior height and diameter in these new environments (Milesi et al., 2019). We do, however, caution this movement to span too large latitudinal gradients, despite the predicted high potential for improvement. The highly consistent shape of individual transfer functions across sites up to a latitude of 60°N, does not apply to the northern most site in Lappkojberget (63.4°N, Supplementary Figure 6). At this site, local provenances appear to be better adapted to the harsh climatic conditions. For the southern part of the range provenance choice may further enhance growth in those mountainous areas, where the increasing frost-free period already shows some positive effect. In terms of range decline, the choice of optimal provenances for reforestation may have some positive effect (total range loss of 39% compared to 43% using “local” provenance), but it may not significantly change the general pattern (Figures 3, 4).
Even though bark beetles have caused vast devastations, e.g., during the extraordinary dry summers 2018–2020, Norway spruce may still present a preferable option in certain areas, if some means of caution are applied. For example, in the German Harz mountains or the Sauerland, where the OccurrenceSDM already indicates great range loss by the 2050s, it may still be possible to make use of the densely emerging natural regeneration, as height growth potential is predicted to remain high in the 2050s (Figures 3, 4). As long as the conversion towards less susceptible mixed forests is initiated to mitigate the unforeseeable risks and increase stand resistance, one short generation of Norway spruce may be exhausted as productive element drawing upon her economic value (Paul et al., 2019). Heterogeneity (instead of largely common homogeneity in species and age in the past) appears to rule the future. This is recognized by the forest practice: management prescriptions urge for species mixtures, uneven-aged structures, small scale patches, or a mixture of the different approaches.
Data Availability Statement
The original contributions presented in the study are included in the article/Supplementary Material, further inquiries can be directed to the corresponding author.
Author Contributions
KL and ML conceived and designed the study and carried out data curation. KL performed the analysis and prepared the original draft. EM and MM-T provided critical feedback and helped to shape research, analysis, and manuscript. All authors reviewed the article and gave their approval to the final version to be published.
Funding
This research was in part conducted during the project “FitForClim” funded by the German Federal Ministry of Food and Agriculture and the Federal Ministry of the Environment, Nature Conservation and Nuclear Safety following a decision by the German Bundestag (Waldklimafonds, Grant number 22WB400704).
Conflict of Interest
The authors declare that the research was conducted in the absence of any commercial or financial relationships that could be construed as a potential conflict of interest.
Publisher’s Note
All claims expressed in this article are solely those of the authors and do not necessarily represent those of their affiliated organizations, or those of the publisher, the editors and the reviewers. Any product that may be evaluated in this article, or claim that may be made by its manufacturer, is not guaranteed or endorsed by the publisher.
Acknowledgments
Analysis comparable to those presented here, are only possible through international collaborations, long-term maintenance and measurement of test sites, and the publication of their results. Our acknowledgment reaches out to all people involved in the IPTNS 1968/64 series for their immense efforts. At Thünen Institute, this particularly includes Armin König and Carla Dühring, who managed these trials over several years. We further thank the two reviewers for their valuable comments to improve an earlier version of the manuscript.
Supplementary Material
The Supplementary Material for this article can be found online at: https://www.frontiersin.org/articles/10.3389/ffgc.2022.804857/full#supplementary-material
References
Aitken, S. N., and Bemmels, J. B. (2016). Time to get moving: assisted gene flow of forest trees. Evol. Appl. 9, 271–290. doi: 10.1111/eva.12293
Aitken, S. N., Yeaman, S., Holliday, J. A., Wang, T., and Curtis-McLane, S. (2008). Adaptation, migration or extirpation: climate change outcomes for tree populations. Evol. Appl. 1, 95–111. doi: 10.1111/j.1752-4571.2007.00013.x
Alberto, F. J., Aitken, S. N., Alía, R., González-Martínez, S. C., Hänninen, H., Kremer, A., et al. (2013). Potential for evolutionary responses to climate change – evidence from tree populations. Glob. Chang. Biol. 19, 1645–1661. doi: 10.1111/gcb.12181
Araújo, M. B., Anderson, R. P., Barbosa, A. M., Beale, C. M., Dormann, C. F., Early, R., et al. (2019). Standards for distribution models in biodiversity assessments. Sci. Adv. 5:eaat4858. doi: 10.1126/sciadv.aat4858
Bałut, S., and Sabor, J. (2002). Inventory Provenance Test of Norway Spruce (Picea abies (L.) Karst.) IPTNS-IUFRO 1964/68 in Krynica. Part II: Test Results of 1968-1984. Geographical Variability of Traits in the Whole Range of Species. Kraków: Norway Spruce Provenances and Breeding.
Barbet-Massin, M., Jiguet, F., Albert, C. H., and Thuiller, W. (2012). Selecting pseudo-absences for species distribution models: how, where and how many? Methods Ecol. Evol. 3, 327–338. doi: 10.1111/j.2041-210X.2011.00172.x
Barnes, J. C., and Delborne, J. A. (2019). Rethinking restoration targets for American chestnut using species distribution modeling. Biodivers. Conserv. 28, 3199–3220. doi: 10.1007/s10531-019-01814-8
Benito-Garzón, M., Robson, T. M., and Hampe, A. (2019). ΔTraitSDMs: species distribution models that account for local adaptation and phenotypic plasticity. New Phytol. 222, 1757–1765. doi: 10.1111/nph.15716
Bolte, A., Ammer, C., Löf, M., Madsen, P., Nabuurs, G. J., Schall, P., et al. (2009). Adaptive forest management in central Europe: climate change impacts, strategies and integrative concept. Scand. J. For. Res. 24, 473–482. doi: 10.1080/02827580903418224
Bradshaw, A. D. (1965). Evolutionary significance of phenotypic plasticity in plants. Adv. Genet. 13, 115–155. doi: 10.1016/S0065-2660(08)60048-6
Buras, A., and Menzel, A. (2019). Projecting tree species composition changes of european forests for 2061–2090 under RCP 4.5 and RCP 8.5 scenarios. Front. Plant Sci. 9:1986. doi: 10.3389/fpls.2018.01986
Caudullo, G., Tinner, W., and de Rigo, D. (2016). “Picea abies in Europe: distribution, habitat, usage and threats,” in European Atlas of Forest Tree Species, eds A. San-Miguel-Ayanz, J. de Rigo, D. Caudullo, G. H. Durrant, and T. Mauri (Luxembourg: Publications Office of the European Union), e012300. doi: 10.2788/4251
Chakraborty, D., Jandl, R., Kapeller, S., and Schueler, S. (2019a). Disentangling the role of climate and soil on tree growth and its interaction with seed origin. Sci. Total Environ. 654, 393–401. doi: 10.1016/j.scitotenv.2018.11.093
Chakraborty, D., Móricz, N., Rasztovits, E., Dobor, L., and Schueler, S. (2021). Provisioning forest and conservation science with high-resolution maps of potential distribution of major European tree species under climate change. Ann. For. Sci. 78:26. doi: 10.1007/s13595-021-01029-4
Chakraborty, D., Schueler, S., Lexer, M. J., and Wang, T. (2019b). Genetic trials improve the transfer of Douglas-fir distribution models across continents. Ecography (Cop.) 42, 88–101. doi: 10.1111/ecog.03888
Chakraborty, D., Wang, T., Andre, K., Konnert, M., Lexer, M. J., Matulla, C., et al. (2015). Selecting populations for non-analogous climate conditions using universal response functions: the case of Douglas-fir in Central Europe. PLoS One 10:e0136357. doi: 10.1371/journal.pone.0136357
Chakraborty, D., Wang, T., Andre, K., Konnert, M., Lexer, M. J., Matulla, C., et al. (2016). Adapting Douglas-fir forestry in Central Europe: evaluation, application, and uncertainty analysis of a genetically based model. Eur. J. For. Res. 135, 919–936. doi: 10.1007/s10342-016-0984-5
Cinelli, C., and Hazlett, C. (2020). Making sense of sensitivity: extending omitted variable bias. J. R. Stat. Soc. Ser. B Stat. Methodol. 82, 39–67. doi: 10.1111/rssb.12348
Collignon, A.-M., Van de Sype, H., and Favre, J.-M. (2002). Geographical variation in random amplified polymorphic DNA and quantitative trais in Norway Sprouce. Can. J. For. Res. 32, 266–282. doi: 10.1139/x01-198
Delzon, S., Urli, M., Samalens, J. C., Lamy, J. B., Lischke, H., Sin, F., et al. (2013). Field evidence of colonisation by holm oak, at the Northern Margin of its distribution range, during the anthropocene period. PLoS One 8:e0080443. doi: 10.1371/journal.pone.0080443
Dormann, C. F., Elith, J., Bacher, S., Buchmann, C., Carl, G., Carré, G., et al. (2013). Collinearity: a review of methods to deal with it and a simulation study evaluating their performance. Ecography (Cop.) 36, 27–46. doi: 10.1111/j.1600-0587.2012.07348.x
Dyderski, M. K., Paź, S., Frelich, L. E., and Jagodziński, A. M. (2018). How much does climate change threaten European forest tree species distributions? Glob. Chang. Biol. 24, 1150–1163. doi: 10.1111/gcb.13925
Felton, A., Sonesson, J., Nilsson, U., Lämås, T., Lundmark, T., Nordin, A., et al. (2017). Varying rotation lengths in northern production forests: implications for habitats provided by retention and production trees. Ambio 46, 324–334. doi: 10.1007/s13280-017-0909-7
Franks, S. J., Weber, J. J., and Aitken, S. N. (2014). Evolutionary and plastic responses to climate change in terrestrial plant populations. Evol. Appl. 7, 123–139. doi: 10.1111/eva.12112
Fréjaville, T., Vizcaíno-Palomar, N., Fady, B., Kremer, A., and Marta, B.-G. (2020). Range margin populations show high climate adaptation lags in European trees. Glob. Chang. Biol. 26, 484–495. doi: 10.1111/gcb.14881
Gárate-Escamilla, H., Hampe, A., Vizcaíno-Palomar, N., Robson, T. M., and Benito-Garzón, M. (2019). Range-wide variation in local adaptation and phenotypic plasticity of fitness-related traits in Fagus sylvatica and their implications under climate change. Glob. Ecol. Biogeogr. 28, 1336–1350. doi: 10.1111/geb.12936
Gardiner, B., Blennow, K., Carnus, J., Fleischer, P., Ingemarson, F., Landmann, G., et al. (2010). Destructive Storms in European Forests: Past and Forthcoming Impacts. Final Report to European Commission – DG Environment. Wallingford: CABI, 138.
Giertych, M. (2007). “Provenance variation and inheritance,” in Biology and Ecology of Norway Spruce, 1st Edn, eds M. G. Tjoelker, A. Boratyński, and W. Bugała (Dodtrecht: Springer), 115–147.
Gray, L. K., and Hamann, A. (2013). Tracking suitable habitat for tree populations under climate change in western North America. Clim. Change 117, 289–303. doi: 10.1007/s10584-012-0548-8
Guisan, A., and Thuiller, W. (2005). Predicting species distribution: offering more than simple habitat models. Ecol. Lett. 8, 993–1009. doi: 10.1111/j.1461-0248.2005.00792.x
Hannerz, M. (1999). Evaluation of temperature models for predicting bud burst in Norway spruce. Can. J. For. Res. Can. Rech. For. 29, 9–19. doi: 10.1139/x98-175
Hu, X.-G., Mao, J., El-Kassaby, Y. A., Jia, K.-H., Jiao, S.-Q., Zhou, S.-S., et al. (2019). Local adaptation and response of Platycladus orientalis (L.) franco populations to climate change. Forests 10, 1–19. doi: 10.3390/f10080622
Isaac-Renton, M. G., Roberts, D. R., Hamann, A., and Spiecker, H. (2014). Douglas-fir plantations in Europe: a retrospective test of assisted migration to address climate change. Glob. Chang. Biol. 20, 2607–2617. doi: 10.1111/gcb.12604
Isik, K., Kleinschmit, J., and Steiner, W. (2010). Age-age correlations and early selection for height in a clonal genetic test of Norway spruce. For. Sci. 56, 212–221. doi: 10.1093/forestscience/56.2.212
Iturbide, M., Bedia, J., Herrera, S., del Hierro, O., Pinto, M., and Gutiérrez, J. M. (2015). A framework for species distribution modelling with improved pseudo-absence generation. Ecol. Modell. 312, 166–174. doi: 10.1016/j.ecolmodel.2015.05.018
Jansen, S., Konrad, H., and Geburek, T. (2017). The extent of historic translocation of Norway spruce forest reproductive material in Europe. Ann. For. Res. 74, 1–17. doi: 10.1007/s13595-017-0644-z
Kapeller, S., Dieckmann, U., and Schueler, S. (2017). Varying selection differential throughout the climatic range of Norway spruce in Central Europe. Evol. Appl. 10, 25–38. doi: 10.1111/eva.12413
Kapeller, S., Lexer, M. J., Geburek, T., Hiebl, J., and Schueler, S. (2012). Intraspecific variation in climate response of Norway spruce in the eastern Alpine range: selecting appropriate provenances for future climate. For. Ecol. Manage. 271, 46–57. doi: 10.1016/j.foreco.2012.01.039
Kawecki, T. J., and Ebert, D. (2004). Conceptual issues in local adaptation. Ecol. Lett. 7, 1225–1241. doi: 10.1111/j.1461-0248.2004.00684.x
Kramer, K. (1995). Phenotypic plasticity of the phenology of seven European tree species in relation to climatic warming. Plant Cell Environ. 18, 93–104. doi: 10.1111/j.1365-3040.1995.tb00356.x
Krutzsch, P. (1974). The IUFRO 1964-68 provenance test with Norway Spruce (Picea abies (L.) Karst.). Silvae Genet. 23, 58–62.
Krutzsch, P. (1992). IUFRO’s role in coniferous tree improvement: Norway spruce (Picea abies (L.) Karst.). Silvae Genet. 41, 143–150.
Latałowa, M., and van der Knaap, W. O. (2006). Late Quaternary expansion of Norway spruce Picea abies (L.) Karst. in Europe according to pollen data. Quat. Sci. Rev. 25, 2780–2805. doi: 10.1016/j.quascirev.2006.06.007
Leites, L. P., Rehfeldt, G. E., Robinson, A. P., Crookston, N. L., and Jaquish, B. C. (2012). Possibilities and limitations of using historic provenance tests to infer forest species growth to climate change. Nat. Resour. Model. 25, 409–433. doi: 10.1111/j.1939-7445.2012.00129.x
Liepe, K. J., Mittelberg, H. S., and Liesebach, M. (2020). Der IUFRO-Fichtenherkunftsversuch von 1964/68 - Eine erste abschließende Auswertung der deutschen Flächen nach Herkunftsgruppen. Thünen Rep. 76, 60–72. doi: 10.3220/REP1584625360000
Liesebach, M., König, A. O., and Ujvári-Jármay, É (2001). “Provenance-environment interactions of Norway spruce (Picea abies [L.] Karst.) on German and Hungarian test sites,” in Genetic Response of Forest Systems to Changing Environmental Conditions. Vol. 70 (For. Sci.), eds G. Müller-Starck and R. Schubert (Dordrecht: Kluwer Academic Publishers), 353–363. doi: 10.1007/978-94-015-9839-2_29
Liu, C., Berry, P. M., Dawson, T. P., and Pearson, R. G. (2005). Selecting thresholds of occurrence in the prediction of species distributions. Ecography (Cop.) 28, 385–393. doi: 10.1111/j.0906-7590.2005.03957.x
Marchi, M., Castellanos-Acuña, D., Hamann, A., Wang, T., Ray, D., and Menzel, A. (2020). ClimateEU, scale-free climate normals, historical time series, and future projections for Europe. Sci. Data 7:428. doi: 10.1038/s41597-020-00763-0
Marini, L., Økland, B., Jönsson, A. M., Bentz, B., Carroll, A., Forster, B., et al. (2017). Climate drivers of bark beetle outbreak dynamics in Norway spruce forests. Ecography (Cop.) 40, 1426–1435. doi: 10.1111/ecog.02769
Mátyás, C. (1994). Modeling climate change effects with provenance test data. Tree Physiol. 14, 797–804. doi: 10.1093/treephys/14.7-8-9.797
Mauri, A., Strona, G., and San-Miguel-Ayanz, J. (2017). EU-Forest, a high-resolution tree occurrence dataset for Europe. Sci. Data 4:160123. doi: 10.1038/sdata.2016.123
Meier, E. S., Lischke, H., Schmatz, D. R., and Zimmermann, N. E. (2012). Climate, competition and connectivity affect future migration and ranges of European trees. Glob. Ecol. Biogeogr. 21, 164–178. doi: 10.1111/j.1466-8238.2011.00669.x
Milesi, P., Berlin, M., Chen, J., Orsucci, M., Li, L., Jansson, G., et al. (2019). Assessing the potential for assisted gene flow using past introduction of Norway spruce in Southern Sweden: local adaptation and genetic basis of quantitative traits in trees. Evol. Appl. 12, 1946–1959. doi: 10.1101/481614
Morgenstern, E. K. (1996). Geographic Variation in Forest Trees: Genetic Basis and Application of Knowledge in Silviculture. Vancouver, BC: University of British Columbia Press.
Morin, X., Augspurger, C., and Chuine, I. (2007). Process-based modeling of species’ distributions: what limits temperate tree species’ range boundaries? Ecology 88, 2280–2291. doi: 10.1890/06-1591.1
Nakao, K., Higa, M., Tsuyama, I., Matsui, T., Horikawa, M., and Tanaka, N. (2013). Spatial conservation planning under climate change: using species distribution modeling to assess priority for adaptive management of Fagus crenata in Japan. J. Nat. Conserv. 21, 406–413. doi: 10.1016/j.jnc.2013.06.003
Paul, C., Brandl, S., Friedrich, S., Falk, W., Härtl, F., and Knoke, T. (2019). Climate change and mixed forests: how do altered survival probabilities impact economically desirable species proportions of Norway spruce and European beech? Ann. For. Sci. 76, 1–15. doi: 10.1007/s13595-018-0793-8
Pecchi, M., Marchi, M., Moriondo, M., Forzieri, G., Ammoniaci, M., Bernetti, I., et al. (2020). Potential impact of climate change on the forest coverage and the spatial distribution of 19 key forest tree species in Italy under RCP4.5 IPCC trajectory for 2050s. Forests 11, 1–19. doi: 10.3390/F11090934
Pedlar, J. H., McKenney, D. W., Aubin, I., Beardmore, T., Beaulieu, J., Iverson, L., et al. (2012). Placing forestry in the assisted migration debate. Bioscience 62, 835–842. doi: 10.1525/bio.2012.62.9.10
Petit, R. J., Bialozyt, R., Garnier-Géré, P., and Hampe, A. (2004). Ecology and genetics of tree invasions: from recent introductions to Quaternary migrations. For. Ecol. Manage. 197, 117–137. doi: 10.1016/j.foreco.2004.05.009
R Core Team (2019). R: A Language and Environment for Statistical Computing. Vienna, Austria: R Foundation for Statistical Computing. https://www.r-project.org/
Rau, H.-M. (1983). Entwicklung des deutsch-polnischen Fichten-Provenienzversuches in Hessen. Ergebnisse aus der Anzuchtphase und von den Versuchsflachen bis zum Alter 7. Forstarchiv 54, 15–19.
Rehfeldt, G. E. (2006). A Spline Model of Climate for the Western United States. General Technical Report RMRS-GTR-165. Fort Collins, CO: U.S. Department of Agriculture, Forest Service, Rocky Mountain Research Station, 21. doi: 10.2737/RMRS-GTR-165
Rehfeldt, G. E., Jaquish, B. C., Sáenz-Romero, C., Joyce, D. G., Leites, L. P., St Clair, J. B., et al. (2014). Comparative genetic responses to climate in the varieties of Pinus ponderosa and Pseudotsuga menziesii: reforestation. For. Ecol. Manage. 324, 147–157. doi: 10.1016/j.foreco.2014.02.040
Sáenz-Romero, C., Kremer, A., Nagy, L., Újvári-Jármay, É, Ducousso, A., Kóczán-Horváth, A., et al. (2019). Common garden comparisons confirm inherited differences in sensitivity to climate change between forest tree species. PeerJ 7:e6213. doi: 10.7717/peerj.6213
Savolainen, O., Pyhäjärvi, T., and Knürr, T. (2007). Gene flow and local adaptation in trees. Annu. Rev. Ecol. Evol. Syst. 38, 595–619. doi: 10.1146/annurev.ecolsys.38.091206.095646
Schmidt-Vogt, H. (1977). Die Fichte. Band I: Taxonomie, Verbreitung, Morphologie, Ökologie, Waldgesellschaften. Hamburg: Verlag Paul Parey.
Schmidt-Vogt, H. (1986). Die Fichte. Band II/1: Wachstum, Züchtung, Boden, Umwelt, Holz. Hamburg: Verlag Paul Parey.
Schuldt, B., Buras, A., Arend, M., Vitasse, Y., Beierkuhnlein, C., Damm, A., et al. (2020). A first assessment of the impact of the extreme 2018 summer drought on Central European forests. Basic Appl. Ecol. 45, 86–103. doi: 10.1016/J.BAAE.2020.04.003
Skrøppa, T. (2003). EUFORGEN Technical Guidelines for genetic conservation and use for Norway spruce (Picea abies). Rome: International Plant Genetic Resources Institute.
Taccoen, A., Piedallu, C., Seynave, I., Perez, V., Gégout-Petit, A., Nageleisen, L. M., et al. (2019). Background mortality drivers of European tree species: climate change matters. Proc. R. Soc. B Biol. Sci. 286, 1–10. doi: 10.1098/rspb.2019.0386
Takolander, A., Hickler, T., Meller, L., and Cabeza, M. (2019). Comparing future shifts in tree species distributions across Europe projected by statistical and dynamic process-based models. Reg. Environ. Chang. 19, 251–266. doi: 10.1007/s10113-018-1403-x
Thuiller, W., Georges, D., Engler, R., and Lafourcade, B. (2012). BIOMOD: Tutorial. Available online at: http://www.will.chez-alice.fr/pdf/BiomodTutorial.pdf (accessed October 2, 2021).
Thuiller, W., Georges, D., Gueguen, M., Engler, R., and Breiner, F. (2021). biomod2: Ensemble Platform for Species Distribution Modeling. R Package Version 3.5.1. https://CRAN.R-project.org/package=biomod2 (accessed June 11, 2021).
Tollefsrud, M. M., Kissling, R., Gugerli, F., Johnsen, Ø, Skrøppa, T., Cheddadi, R., et al. (2008). Genetic consequences of glacial survival and postglacial colonization in Norway spruce: combined analysis of mitochondrial DNA and fossil pollen. Mol. Ecol. 17, 4134–4150. doi: 10.1111/j.1365-294X.2008.03893.x
Ujvári-Jármay, É, Nagy, L., and Mátyás, C. (2016). The IUFRO 1964/68 inventory provenance trial of Norway spruce in Nyírjes, Hungary – Results and conclusions of five decades. Acta Silv. Lignaria Hungarica 12:177. doi: 10.1515/aslh-2016-0001
Urban, M. C., Bocedi, G., Hendry, A. P., Mihoub, J. B., Pe’er, G., Singer, A., et al. (2016). Improving the forecast for biodiversity under climate change. Science 353:aad8466. doi: 10.1126/science.aad8466
van der Maaten, E., Hamann, A., van der Maaten-Theunissen, M., Bergsma, A., Hengeveld, G., van Lammeren, R., et al. (2017). Species distribution models predict temporal but not spatial variation in forest growth. Ecol. Evol. 7, 2585–2594. doi: 10.1002/ece3.2696
Vitali, V., Büntgen, U., and Bauhus, J. (2017). Silver fir and Douglas fir are more tolerant to extreme droughts than Norway spruce in south-western Germany. Glob. Chang. Biol. 23, 5108–5119. doi: 10.1111/gcb.13774
Vizcaíno-Palomar, N., Fady, B., Alía, R., Raffin, A., Mutke, S., and Marta, B.-G. (2020). The legacy of climate variability over the last century on populations’ phenotypic variation in tree height. Sci. Total Environ. 749, 1–13. doi: 10.1016/j.scitotenv.2020.141454
Wang, T., Hamann, A., Spittlehouse, D. L., and Murdock, T. Q. (2012). ClimateWNA-high-resolution spatial climate data for western North America. J. Appl. Meteorol. Climatol. 51, 16–29. doi: 10.1175/JAMC-D-11-043.1
Wang, T., O’Neill, G. A., and Aitken, S. N. (2010). Integrating environmental and genetic effects to predict responses of tree populations to climate. Ecol. Appl. 20, 153–163. doi: 10.1890/08-2257.1
Williams, M. I., and Dumroese, R. K. (2013). Preparing for climate change: forestry and assisted migration. J. For. 111, 287–297. doi: 10.5849/jof.13-016
Yang, J., Pedlar, J. H., McKenney, D. W., and Weersink, A. (2015). The development of universal response functions to facilitate climate-smart regeneration of black spruce and white pine in Ontario, Canada. For. Ecol. Manage. 339, 34–43. doi: 10.1016/j.foreco.2014.12.001
Zeltinš, P., Katreviès, J., Gailis, A., Maaten, T., Desaine, I., and Jansons, A. (2019). Adaptation capacity of Norway spruce provenances in Western Latvia. Forests 10, 1–15. doi: 10.3390/f10100840
Keywords: assisted migration, climate change, height growth, local adaptation, phenotypic plasticity, provenance trial, species distribution model, universal response function
Citation: Liepe KJ, van der Maaten E, van der Maaten-Theunissen M and Liesebach M (2022) High Phenotypic Plasticity, but Low Signals of Local Adaptation to Climate in a Large-Scale Transplant Experiment of Picea abies (L.) Karst. in Europe. Front. For. Glob. Change 5:804857. doi: 10.3389/ffgc.2022.804857
Received: 29 October 2021; Accepted: 07 March 2022;
Published: 29 March 2022.
Edited by:
Christian Messier, Université du Québec à Montréal, CanadaReviewed by:
Maxime Cailleret, Institut National de Recherche pour l’Agriculture, l’Alimentation et l’Environnement (INRAE), FranceRomà Ogaya, Ecological and Forestry Applications Research Center (CREAF), Spain
Copyright © 2022 Liepe, van der Maaten, van der Maaten-Theunissen and Liesebach. This is an open-access article distributed under the terms of the Creative Commons Attribution License (CC BY). The use, distribution or reproduction in other forums is permitted, provided the original author(s) and the copyright owner(s) are credited and that the original publication in this journal is cited, in accordance with accepted academic practice. No use, distribution or reproduction is permitted which does not comply with these terms.
*Correspondence: Katharina Julie Liepe, katharina.liepe@thuenen.de