Nitrogen deposition in forests: Statistical modeling of total deposition from throughfall loads
- 1Institute for Applied Plant Biology AG, Witterswil, Switzerland
- 2Department of Environmental Control, Northwest German Forest Research Institute (NW-FVA), Göttingen, Germany
- 3Ecotoxicology of Air Pollution, Research Centre for Energy, Environment and Technology (CIEMAT), Madrid, Spain
- 4Federal Office for the Environment, Bern, Switzerland
- 5Czech Hydrometeorological Institute, Prague, Czechia
- 6IVL Swedish Environmental Research Institute, Gothenburg, Sweden
- 7State Agency for Nature, Environment and Consumer Protection of North Rhine-Westphalia, Recklinghausen, Germany
- 8WSL-Swiss Federal Institute for Forest, Snow and Landscape Research, Birmensdorf, Switzerland
Introduction: Nitrogen (N) gradient studies in some cases use N deposition in throughfall as measure of N deposition to forests. For evaluating critical loads of N, however, information on total N deposition is required, i.e., the sum of estimates of dry, wet and occult deposition.
Methods: The present paper collects a number of studies in Europe where throughfall and total N deposition were compared in different forest types. From this dataset a function was derived which allows to estimate total N deposition from throughfall N deposition.
Results: At low throughfall N deposition values, the proportion of canopy uptake is high and thus the underestimation of total deposition by throughfall N needs to be corrected. At throughfall N deposition values > 20 kg N ha–1 yr–1 canopy uptake is getting less important.
Conclusion: This work shows that throughfall clearly underestimates total deposition of nitrogen. With the present data set covering large parts of Europe it is possible to derive a critical load estimate from gradient studies using throughfall data.
1 Introduction
Excess atmospheric deposition of nitrogen, either in wet, dry or occult form, can result in a cascade of environmental effects impacting ecosystems diversity and functioning. While the wet deposition flux (WD) of nitrogen (N) is easily measured, the dry deposition flux (DD) is an important but very uncertain component in total N deposition flux (TD) estimations. The determination of the TD from the throughfall deposition flux measurements (TF) requires the quantification of canopy exchange (Draaijers and Erisman, 1995). There are large conceptually weaknesses and uncertainties in the application of canopy budget models for the canopy exchange quantification (Ahrends et al., 2020). Other methods applied are micrometeorological measurement and inferential modeling (IFM). The latter method is an approach by which deposition rates can be estimated on a routine basis, using measured concentrations of gasses and particles in the ambient air at a defined reference height and site- and element-specific deposition velocities (Hicks et al., 1991; Wesely and Hicks, 2000). A variety of approaches is used to determine deposition velocities (vd), ranging from dynamic models based on stomatal conductance and atmospheric conditions to land-use specific empirical long-term averaged vd (e.g., Schrader and Brümmer, 2014; Bytnerowicz et al., 2015; García-Gómez et al., 2018). At an intermediate level of complexity, published vd are adapted for site specific conditions based on semi-empirical correction factors (Schmitt et al., 2005; Kirchner et al., 2014).
In addition, DD of N to forests may be estimated based on measurements with surrogate surfaces, such as e.g., Teflon strings placed under of roof, in combination with net throughfall estimates of an element that is regarded as biologically inert, such as e.g., sodium (Ferm and Hultberg, 1999; Karlsson et al., 2019). Following Lindberg and Owens (1992) the net throughfall flux (netTF) is the difference between throughfall deposition flux (TF) and WD (netTF = TF-WD) and represent the DD of an inert element to the forest canopies. Concentration ratios to sodium from the surrogate surface samples then give the DD of the element of interest in relation to the DD of sodium. This approach was tested for a wide range of N DD levels across Sweden (Karlsson et al., 2019, 2022).
For assessing the effect of N deposition on forest ecosystems, nitrogen gradient studies can provide an important knowledge contribution. These gradients are either based on modeled TD with varying spatial grid sizes (see the discussion on this issue in Braun et al., 2017) or on TF measured on the site. For example, TF and open field deposition measurements (together with stemflow in beech stands) are the mandatory methods for plots operated under the UNECE International Co-operative Program on Assessment and Monitoring of Air Pollution Effects on Forests (ICP Forests) (ICP Forests, 2022). Measuring TF is a cheap, simple and low-maintenance method. Accordingly, the number of sites with long-term TF measurements is many times higher than the number of sites with air concentration measurements for applying the IF method (e.g., Ahrends et al., 2020, supplement). Therefore, many gradient studies rely on the results of TF. TF consists of the precipitation collected below canopy using open funnel-type samplers (McDowell et al., 2020). In parallel to throughfall measurements, precipitation is collected in the open field. Mostly, continuously open funnel-type samplers are used, and the open-field deposition is then called bulk deposition (BD). The BD samplers collect mainly WD with a small contribution of DD to the sampling equipment. In trees with a smooth bark like beech, stemflow (SF) is sampled, too, which is the substance flux with the water running down the stem. It contains similar components of inorganic ions as throughfall and can make up 5–20% of the sum of TF and SF (Neal et al., 1993; Chang and Matzner, 2000; Staelens et al., 2007) but it has not been included in gradient studies (e.g. van der Linde et al., 2018 which used the data of Waldner et al. (2014). Stemflow is less important for coniferous tree species, in particular trees with downward pointing branches, such as Norway spruce (Moldan et al., 1998; Kovács and Horváth, 2004; Małek, 2010). Similarly, in broadleaf evergreen trees, stemflow only contributes 3% to total rainfall (Rodrigo et al., 2003).
Throughfall collects WD, occult deposition (OD) and the part of the DD to the forest canopy, but canopy processes can also affect the deposition estimation. The fraction of DD collected varies depending on the atmospheric species and canopy exchange processes (Fenn et al., 2015). Part of the deposited N can be taken up by the forest canopy (Draaijers et al., 1996; Harrison et al., 2000; Adriaenssens et al., 2012; Karlsson et al., 2019), leading to an underestimation of TD by TF. To estimate the N fraction taken up by the canopy, canopy budget models can be applied which were developed by Ulrich (1994) and modified many times (Draaijers and Erisman, 1995; De Vries et al., 2001; Staelens et al., 2008). However, these models cannot estimate the direct uptake of gaseous compounds through the stomata (NH3, NO2) or the cuticle (NH3). Moreover, canopy budget models might not be suitable to represent canopy processes in forests growing in semi-arid environments such as in the Mediterranean biogeographical region (Aguillaume et al., 2017). Further complications arise from transformation into organic N, which is also part of the canopy exchange process (Zimmermann et al., 2006; Cape et al., 2010; Massad et al., 2010; Adriaenssens et al., 2012) or from transformation of reduced forms of N into nitrate by epiphytic nitrifiers (Guerrieri et al., 2020). Other canopy processes such as NH3 emission from leaves (Massad et al., 2010) or atmospheric N fixation by phyllosphere microorganisms (Rico et al., 2014) represent further inputs of N into the canopy whose possible contribution to TF N enrichment needs further research.
At the end, DD calculated with the different approaches needs to be combined with estimations of WD and OD to yield TD. Including OD, i.e., the deposition via cloud or fog water, is particularly relevant in mountain forests which are merged in clouds during a substantial part of the year (Bridges et al., 2002; Blaś et al., 2012; Hůnová et al., 2021a,b).
TF based N gradient studies give valuable information on N effects in ecosystems (e.g., van der Linde et al., 2018). To set critical loads of N, however, information on TD is needed. In the last years various studies were conducted to compare the measurement of N in TF to estimations of TD (García-Gómez et al., 2018; Karlsson et al., 2019; Thimonier et al., 2019; Ahrends et al., 2020). This allows finding relations between TF and TD and deriving a transfer function. The aim of this study is to provide TD estimates for forests where only TF of N is available.
2 Materials and methods
Data were collected from studies which measured total N deposition either using the IFM for DD estimates (Spain: García-Gómez et al., 2018; Switzerland: Thimonier et al., 2019; Germany: Ahrends et al., 2020) or an equivalent estimate using teflon strings (Sweden: Karlsson et al. (2019), along with BD (or WD) and TF. The Thimonier et al. (2019) dataset was amended with TF from an additional site from Braun et al. (2018). Additional datasets included were from Germany (Zimmermann et al., 2006) and Belgium (Neirynck et al., 2007), various NitroEurope sites across Europe (Flechard et al., 2011), Finland (Korhonen et al., 2013) as well as 36 plots from the Czech Republic (Hůnová et al., 2016). The final dataset consisted of 103 coniferous, 33 deciduous, 4 broadleaved evergreen and 2 mixed stands with data from 1–15 years each. The distribution of the plots is shown in Figure 1, a description of the datasets is given in Supplementary Table 1. Since stemflow has not been considered in many gradient studies (Waldner et al., 2014), it was not included even when it was measured.
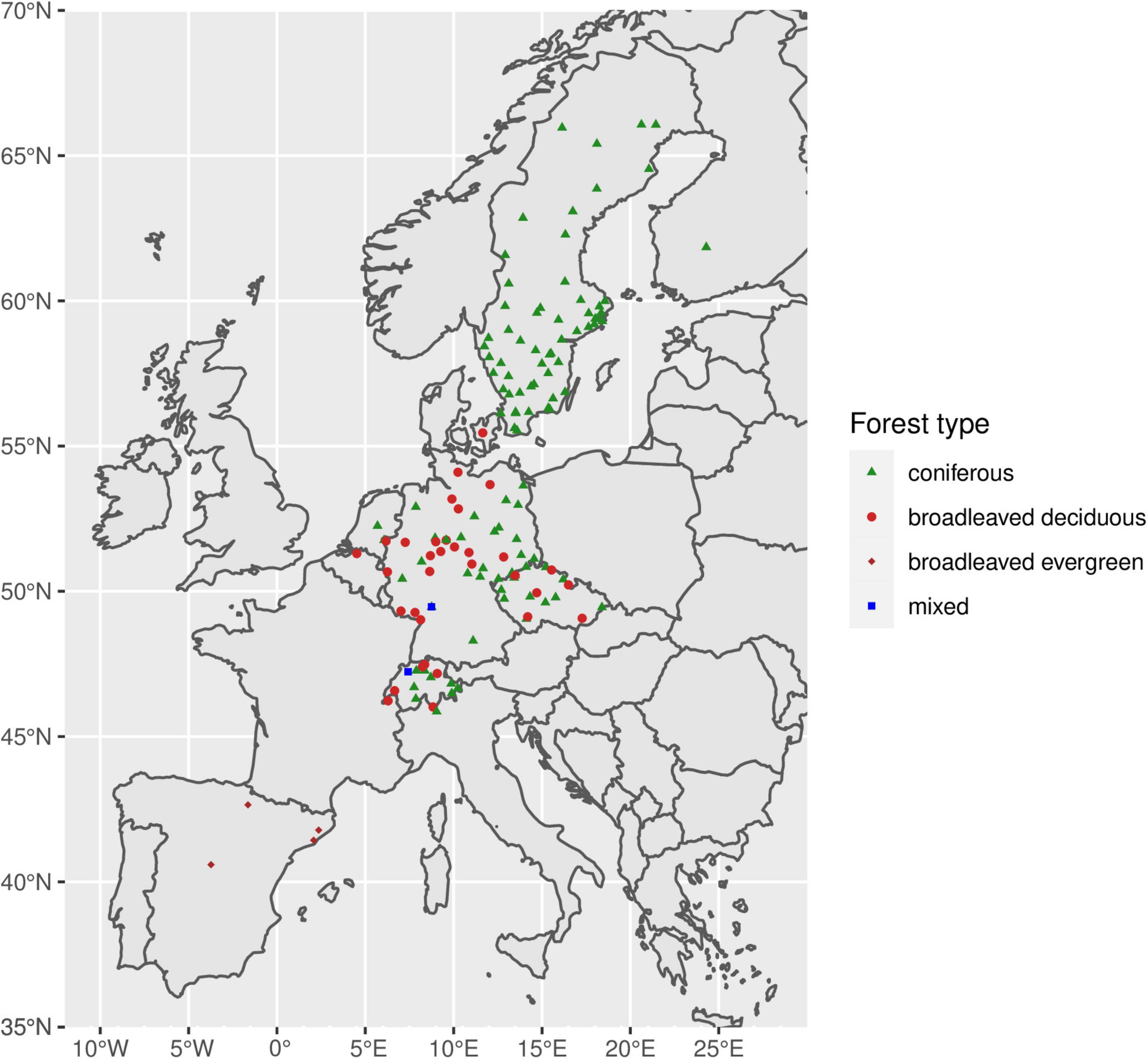
Figure 1. Location of the plots included in the current study. Broadleaf deciduous, broadleaf evergreen, and mixed were combined to one forest group in the analysis.
Response variable of the regression analysis was total N deposition. Explanatory variables were included according to theoretical considerations and availability. The initial model included covariates such as precipitation, altitude, annual temperature, longitude, forest type, year of the measurement and, to cover for differences in measurement methodology, also country. We calculated a Variance Inflating Factor (VIF) to check for collinearity. The data were subjected to an explorative analysis using mixed regression, function glmer in R, and polynomial functions for non-linearity (Bates et al., 2015). Predictors increasing the Akaike information criterion, AIC (Burnham and Anderson, 2002) were removed, and transformations of TD or TF were tested to yield a uniform and normal distribution of the residuals. The model resulting from this selection procedure used loge transformation for both TD and TF, forest type as well as year of measurement and country as random variables. Forest type was included as binary variable (0 = coniferous forests, 1 = broadleaf deciduous, broadleaf evergreen and mixed forests). The final multivariate mixed model (MMM) has the form:
However, during model setup, it was noticed that the inclusion of covariates led to mismatch of the intercept with the data, resulting in systematically overestimation of low values. The final selected model had therefore the form:
without any random effects and covariates. The residuals were checked for outliers, homoscedasticity and normal distribution. However, the model with covariates is listed for information, too.
3 Results
Table 1 lists the output from the MMM model. Forest type (coniferous vs. broadleaved deciduous, broadleaved evergreen and mixed) and geographical longitude were significant predictors. The VIF analysis suggested, however, that longitude was too strongly correlated with TF so it was removed. The coefficient for forest type suggested that the estimates for TD are lower for deciduous stands than for coniferous stands. The model in Table 1 resulted in an estimate of the intercept which was higher than the majority of the data points (Figure 2). Therefore, the model was simplified to the one shown in Table 2, which considerably improved the intercept estimates (Figure 3). Because of the many points from Sweden with low TD, the intercept could be calculated rather precisely. As an example, the estimate for 2 kg N ha–1 yr–1 TF is 6 kg N ha–1 yr–1 TD. For 5 kg N ha–1 yr–1 TF, the estimate for TD is on average 10 kg N ha–1 yr–1.
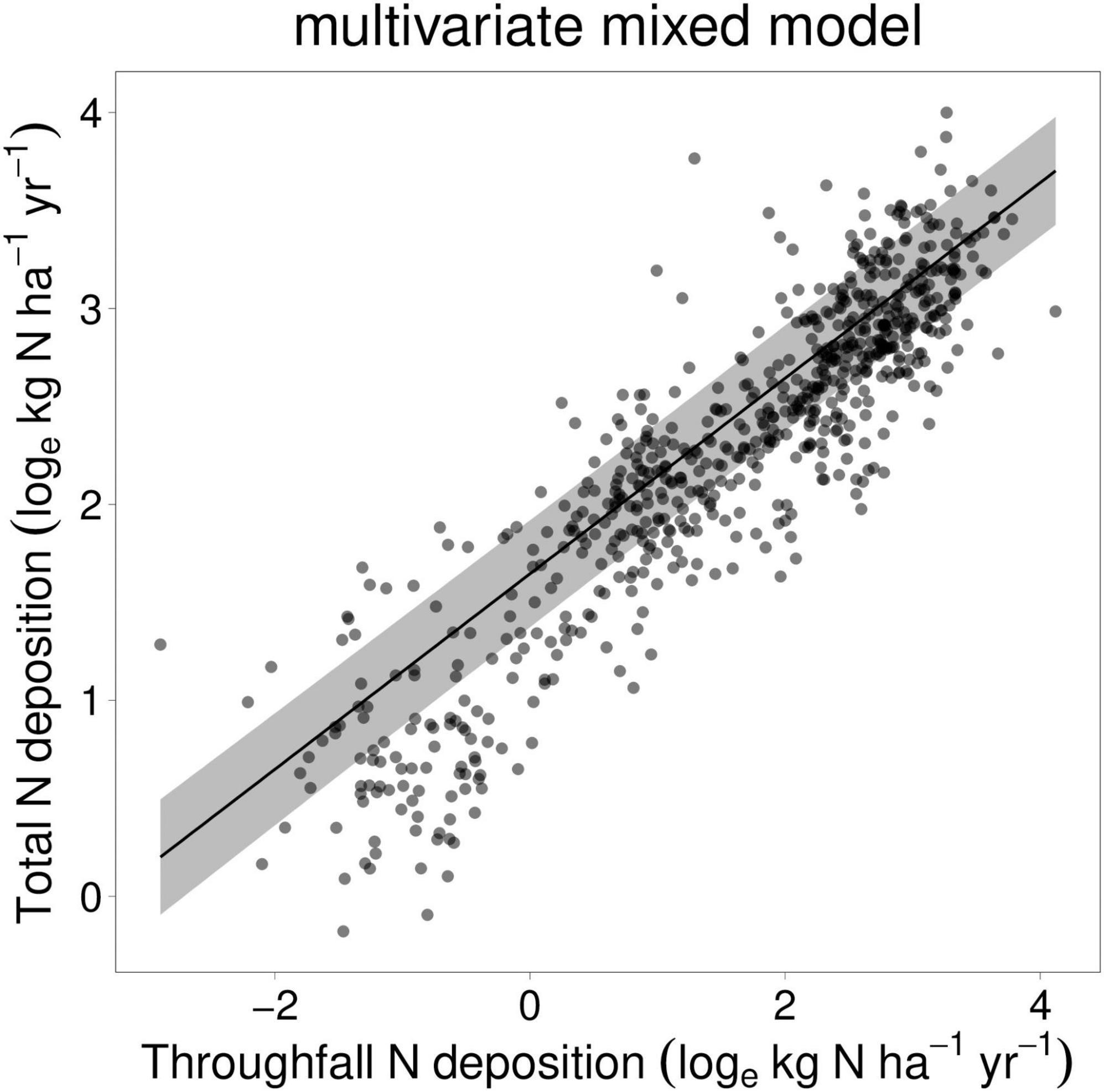
Figure 2. Output of the multivariate mixed model: raw data plot and regression with 95%-confidence intervals as gray fields. Points are semi-transparent, so darker dots indicate overlap of several points.
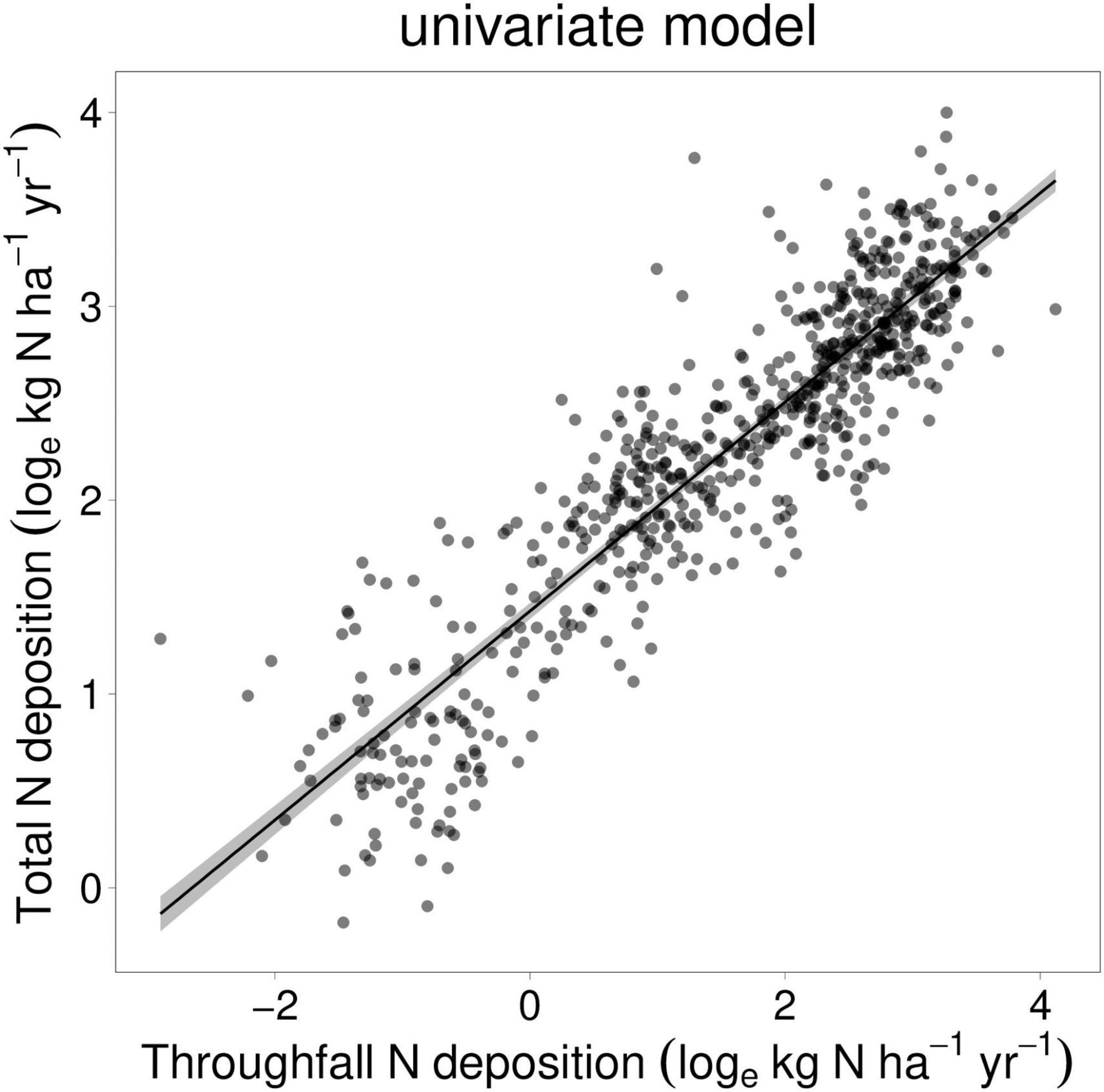
Figure 3. Output of the univariate model: raw data plot and regression with 95%-confidence intervals as gray fields. Points are semi-transparent, so darker dots indicate overlap of several points.
4 Discussion
The measurement of TD is complicated as there is a number of components contributing to it with varying importance for different ecosystems. The IFM for measuring TD uses a number of assumptions on vd but is well established as reference method. In some plots it has been validated with more complicated eddy flux measurements (Neirynck et al., 2007). The teflon string method used in Scandinavia relies on another principle but seems to yield comparable results (Karlsson et al., 2019).
The results of this study clearly confirm that canopy uptake occurs, and that it is significant. The resulting shape, with values above the 1:1 line for TF < 20 kg N ha–1 yr–1 (Figure 4), might be explained by gradients of N deposition and demand in Europe. In far northern latitudes, both the demand and the supply are low, hence the difference between TF and TD will be small in absolute terms (Karlsson et al., 2019). At more central latitudes in Europe, the conditions for physiological activity are better, while the N deposition covers a large range from deposition hotspots to less polluted areas. At some of the less polluted sites, N demand is high while N is not available in sufficient quantities, resulting in a large canopy uptake rate and, correspondingly, in a large difference between TF and TD (with TF < TD). The difference between TF and TD is largest between 4 and 8 kg N ha–1 yr–1 in TF (see also Karlsson et al., 2019). At these more polluted sites the uptake will be low, and hence the difference will be low. In Mediterranean areas, as published in Meixner and Fenn (2004) and García-Gomez et al. (2016), canopy uptake and retention strongly varies throughout the year. During dry periods (mainly summer, but also winter), when climatic conditions determine low physiological activity and N uptake, there is an accumulation of deposited atmospheric N, resulting in N-enriched TF. On the other hand, during spring, there is a higher N demand, reducing the content of N in TF. This asynchrony between N deposition and demand in Mediterranean ecosystems could cause a strong variability on the relationship between TF and TD. Since this regression has been obtained from a large geographical range of studies within Europe, it is meant to be a generalized tool to estimate TD in cases where only TF is measured.
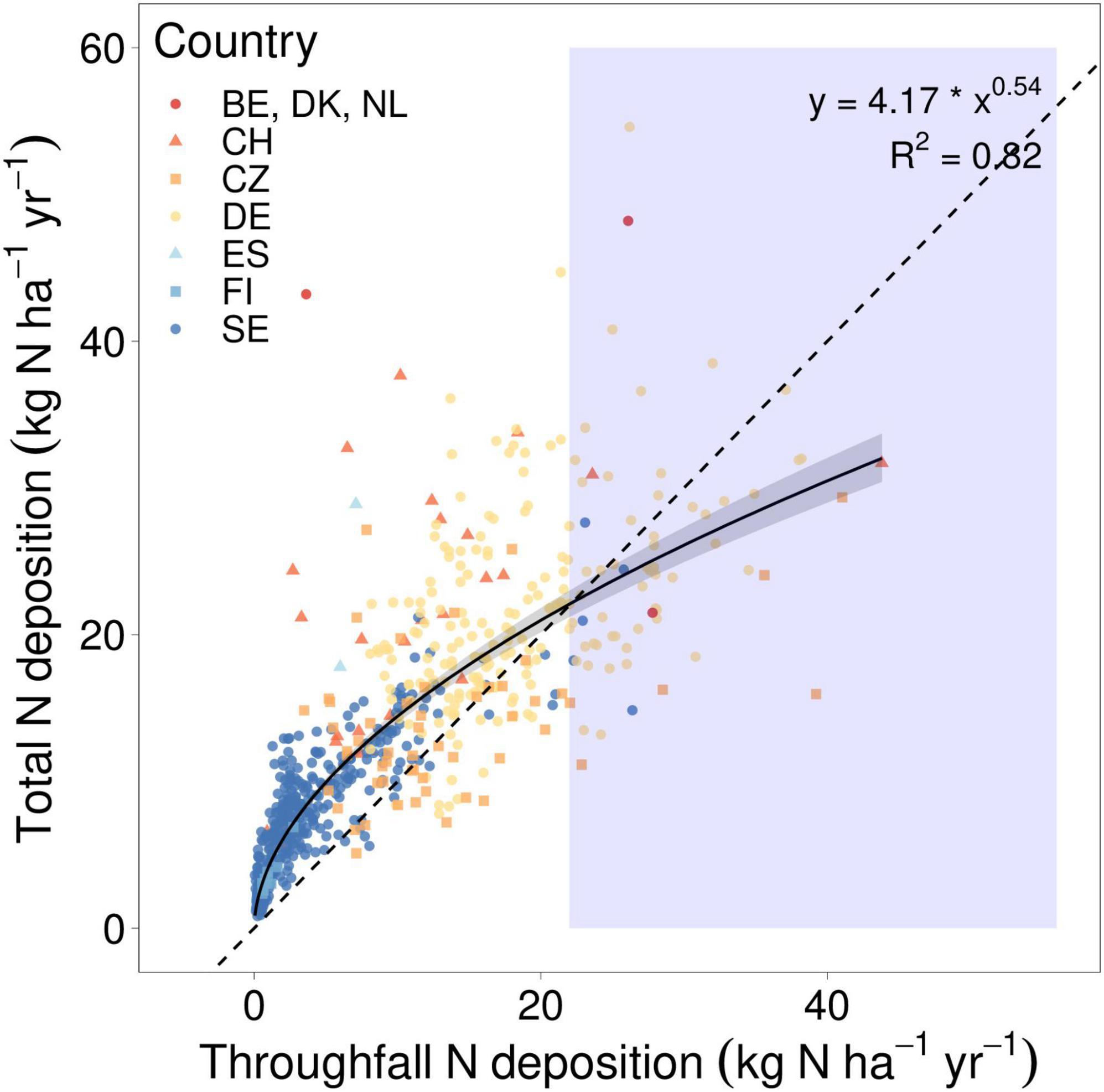
Figure 4. Total N deposition regressed against throughfall N deposition after back-transformation to linear scales (univariate model). The solid line shows the regression. gray range 95% confidence interval. Dashed line: 1:1 line. Blue field: Range where the regression should not be used (TF > TD). BE, Belgium; DK, Denmark; NL, Netherlands; CH, Switzerland; CZ, Czech Republic; DE, Germany; ES, Spain; FI, Finland; SE, Sweden.
The fitted relation of TD vs. TF indicates that TF is lower than TD at TF rates above around 20 kg N ha–1 yr–1. To our knowledge, the leaching of inorganic nitrogen from forest canopies has not been reported in the literature until now. On the contrary, numerous reports indicate that inorganic nitrogen may be taken up by canopy foliage, epiphytic lichens or other microflora (Sparks, 2009; Butterbach-Bahl et al., 2011; Woods et al., 2012; Guerrieri et al., 2021). In view of the large scatter of modeled TD at high TF rates, we hypothesize that the data points with TD < TF (i.e., data points below the 1:1 line in Figure 4, at TF values > 20 kg N ha–1 yr–1) reflect the uncertainty inherent in both the TF measurements and the TD estimations. It is thus not advisable to use the relation reported in our study at TF rates beyond 20 kg N ha–1 yr–1. Instead, TF should be used as a lower boundary estimate of TD in regions where our relation indicates TD < TF. This recommendation is motivated by the realization that the analyzed dataset contains a wide cascade of uncertainties that can accumulate with each measurement and/or calculation step of deposition estimation:
(A) Uncertainty in throughfall sampling and missing stemflow: The stand deposition flux (SD) is the sum of throughfall and stemflow and would be the better basis for comparison with TD. However, stemflow is not always provided in the ICP Forests measurements, and gradient studies usually work with TF only, thus introducing a systematic underestimation of stand deposition in deciduous stands with a smooth bark (e.g., beech). However, this mainly concerns only the beech tree species (Clarke et al., 2016). As in the present study the data analysis had to be restricted to throughfall, the term “throughfall” is used in this study even when “stand deposition” would be more appropriate. At dry sites, precipitation might be insufficient to mobilize the N compounds intercepted by the canopy during dry periods, which leads to lower throughfall fluxes than theoretically expected (Bytnerowicz et al., 2015; Thimonier et al., 2019).
The ICP Forests manual (Clarke et al., 2016) suggests a number of samplers depending on forest stand heterogeneity such that the resulting uncertainty in throughfall measurements is 20% (Draaijers et al., 1998; Thimonier, 1998; Maes et al., 2014). For various reasons, such as occasional contamination by biological material in the samplers, etc., this might be rather a lower boundary estimate.
(B) Uncertainty in TD of those N deposition pathways covered by the methods. Flechard et al. (2011) demonstrated that discrepancies between N deposition estimates from different IFM at one site can be several times larger than between sites. One possible reason for this is that the IFM usually is a combination of observations and modeling with numerous assumptions. The gaseous components NO2 and NH3 were assessed at the intensive monitoring sites. The accuracy level of these measurements is stated to be about ± 30% (Schaub et al., 2016). Other aspects, such as the low sampling frequency of passive samplers, may cause biased results (Schrader et al., 2018). A probably larger uncertainty is harbored in the parameterization of vd. Several mechanistic models for vd exist, which e.g., utilize detailed information on meteorology and soil water availability to model stomatal opening. This allows for the parameterization of stomatal exchange if ambient air concentrations compared to foliar concentrations are known (Wichink Kruit et al., 2012). While the complexity of these comprehensive models offers the opportunity for an accurate quantification of DD fluxes, additional uncertainty arises from assumptions about input parameters. The studies used in this paper choose a different approach, starting from typical vd from the literature. These forest type specific values were adjusted using the most important determinants for vd in order to derive “semi-empirical correction factors.” However the different authors have used various vd for the IFM: some such as Ahrends et al. (2020) used site-specific modifications on the vd to account for differences in exposition, wind speed, etc., others differentiated the vd with forest type (Thimonier et al., 2019) or simply used the annual mean vd (Hůnová et al., 2016). Thus, the resulting vd for gaseous and particulate substances are not easily comparable and can only be considered as a very rough estimate. Furthermore, the DD of HNO3, particulate NH4+ and particulate NO3– was often calculated from open field deposition based on an empirical relation, because local concentration measurements were rarely available. At the same time we are fully aware of the fact that vd belongs to key factors in DD estimate, is highly variable due to its dependence on surface, meteorological conditions, season and time of the day (Brook et al., 1997; Zhang et al., 2009), and thus can introduce a substantial uncertainty in the deposition estimate.
(C) Some N deposition pathways not covered by the methods: Inorganic N deposition can be converted to organic N in the canopy by microorganisms (e.g., Cape et al., 2010). This N deposition pathway is accounted for in the TD estimates but is missing in the measured stand deposition of inorganic N. Similarly, the stomatal canopy uptake of NH3 and NO2 is accounted for in the TD estimates but not in the TF. The magnitude of these effects probably strongly varies depending on site-specific aspects such as plant species, meteorological conditions and soil water availability.
The final regression model gave a reasonable fit to the data and can serve to translate TF measurements into TD estimates in the desired context of gradient studies of forest ecosystem responses to N deposition. A number of covariates were tested in the regression model. Precipitation was not significant but forest type was a significant predictor in the mixed model, and the inclusion of country and year as random variables improved the model, too. Longitude was too closely related with throughfall deposition and confounded forest type. This is, however, without practical consequence for the final selected model as the inclusion of covariates affected the intercept estimates, resulting in a systematic overestimation of the N deposition at low throughfall values. The knowledge on a longitude effect would also not help to improve the result of a gradient study where detailed site information is not available anyway.
In their comparison of TD estimations from the EMEP chemical transport model (Simpson et al., 2020) with TF from ICP forest plots, Marchetto et al. (2021) came to a similar conclusion as the present study. They report that EMEP-modeled TD of NH4+ is on average 77% higher and modeled TD of NO3– is on average 72% higher compared to TF measurements at the same site. Their scatter plots show the largest differences at NH4+ loads in TF between 5 and 10 kg N ha–1 yr–1 and at NO3– loads in TF between 3 and 8 kg N ha–1 yr–1. As the EMEP estimate of total N deposition is now available at 0.1° grid size (which corresponds to between 3.5 km and 8.5 km in Europe), it can provide additional information for gradient studies. For example it can help to set limits to estimates from throughfall studies in low deposition areas. However, it has to be taken into account that in the EMEP grid various receptors in the grid cell are weighted. For the use with forests, the data can be downloaded with specific vd for coniferous and deciduous forests (EMEP, 2022).
5 Conclusion
TF clearly underestimates TD of nitrogen. This underestimation is largest in areas with low to medium deposition. With the present data set covering large parts of Europe it is possible to derive a critical load estimate from gradient studies using TF data (Bobbink et al., 2022).
Data availability statement
The datasets presented in this study can be found in online repositories. The names of the repository/repositories and accession number (s) can be found below: doi: 10.5061/dryad.2z34tmpqc.
Author contributions
SA and SB made the concept of the study. SB, BA, AS, and PK collected the data. RA, HG-G, IH, GK, and AT contributed to the data. All authors contributed to the article and approved the submitted version.
Funding
This evaluation was partly based on data that was collected by partners of the official UNECE ICP Forests Network (http://icp-forests.net/page/participating-countries). Part of the data was Co-financed by the European Commission. Partial funding of data collection was provided by the European Union Under Council Regulation (EEC) 3528/86 on the Protection of Forests Against Atmospheric Pollution, the Regulation (EC) 2152/2003 concerning monitoring of forests and environmental interactions in the community (Forest Focus) and by the project LIFE 07 ENV/D/000218 Further Development and Implementation of an EU-Level Forest Monitoring System (FutMon). The German part of evaluation was part of the project “Site adapted management in privately owned small forests” funded by BMEL/FNR (FKZ: 2220NR269C). The Swedish data was collected within the Swedish Throughfall Monitoring Network (SWETHRO). SWETHRO was run by IVL Swedish Environmental Research Institute and funded by Regional Air Quality Protection Associations, County Administrative Boards, and the Swedish Environmental Protection Agency. The Swiss study was partly funded by the Swiss Federal Office for the Environment (FOEN), the environmental departments of the cantons GR, AG, BE, GE, SO and TI, and OSTLUFT. The Spanish data were collected in the framework of the EDEN-Med Project (FEDER/MICINN–AEI CGL2017-84687-C2-1-R) and the collaboration in this study was co-funded by the Framework on atmospheric pollution and persistent organic pollutants between DGCEA and CIEMAT (ACTUA-MITERD). The Czech data used for the analysis were provided by the Czech Hydrometeorological Institute, Czech Geological Survey, and Forestry and Game Management Research Institute.
Acknowledgments
We thank Christian Schindler, Swiss Tropical and Public Health Institute, University of Basel, Switzerland, for statistical advice. We also thank Christophe Flechard, Johan Neirynck, Janne Korhonen, Klaus Butterbach-Bahl and Pasi Kolari for their assistance in locating additional data sets. We thank the numerous staff involved in operating the deposition monitoring in the different countries in the last decades. We acknowledge the Dry Deposition Group initiative within the Expert Panel on Deposition of ICP Forests (http://icp-forests.net/group/dry-deposition), which stimulated this collaboration.
Conflict of interest
The authors declare that the research was conducted in the absence of any commercial or financial relationships that could be construed as a potential conflict of interest.
Publisher’s note
All claims expressed in this article are solely those of the authors and do not necessarily represent those of their affiliated organizations, or those of the publisher, the editors and the reviewers. Any product that may be evaluated in this article, or claim that may be made by its manufacturer, is not guaranteed or endorsed by the publisher.
Supplementary material
The Supplementary Material for this article can be found online at: https://www.frontiersin.org/articles/10.3389/ffgc.2022.1062223/full#supplementary-material
References
Adriaenssens, S., Hansen, K., Staelens, J., Wuyts, K., De Schrijver, A., Baeten, L., et al. (2012). Throughfall deposition and canopy exchange processes along a vertical gradient within the canopy of beech (Fagus sylvatica L.) and Norway spruce (Picea abies (L.) Karst). Sci. Total Environ. 420, 168–182. doi: 10.1016/j.scitotenv.2011.12.029
Aguillaume, L., Izquieta-Rojano, S., García-Gómez, H., Elustondo, D., Santamaría, J. M., Alonso, R., et al. (2017). Dry deposition and canopy uptake in Mediterranean holm-oak forests estimated with a canopy budget model: A focus on N estimations. Atmos. Environ. 152, 191–200. doi: 10.1016/j.atmosenv.2016.12.038
Ahrends, B., Schmitz, A., Prescher, A.-K., Wehberg, J., Geupel, M., Andreae, H., et al. (2020). Comparison of methods for the estimation of total inorganic nitrogen deposition to forests in Germany. Front. For. Glob. Chang. 3:103. doi: 10.3389/ffgc.2020.00103
Bates, D., Maechler, M., Bolker, B., and Walker, S. (2015). Fitting linear mixed-effects models using lme4. J. Stat. Softw. 67, 1–48.
Blaś, M., Sobik, M., Polkowska, Z., Cichala-Kamrowska, K., and Namieśnik, J. (2012). Water and chemical properties of hydrometeors over central European mountains. Pure Appl. Geophys. 169, 1067–1081. doi: 10.1007/s00024-011-0359-2
Bobbink, R., Loran, C., and Tomassen, H. (2022). Review and revision of empirical critical loads of nitrogen for Europe. Dessau: German Environmental Agency.
Braun, S., Achermann, B., De Marco, A., Pleijel, H., Karlsson, P. E., Rihm, B., et al. (2017). Epidemiological analysis of ozone and nitrogen impacts on vegetation – critical evaluation and recommendations. Sci. Total Environ. 603–604, 785–792. doi: 10.1016/j.scitotenv.2017.02.225
Braun, S., Hopf, S.-E., and de Witte, L. C. (2018). Wie geht es unserem wald? 34 jahre jahre walddauerbeobachtung. Schönenbuch: Institut für angewandte Pflanzenbiologie.
Bridges, K. S., Jickells, T. D., Davies, T. D., Zeman, Z., and Hunova, I. (2002). Aerosol, precipitation and cloud water chemistry observations on the czech krusne hory plateau adjacent to a heavily industrialised valley. Atmos. Environ. 36, 353–360. doi: 10.1016/S1352-2310(01)00388-0
Brook, J. R., Di-Giovanni, F., Cakmak, S., and Meyers, T. P. (1997). Estimation of dry deposition velocity using inferential models and site-specific meteorology - uncertainty due to siting of meteorological towers. Atmos. Environ. 31, 3911–3919. doi: 10.1016/S1352-2310(97)00247-1
Burnham, K. P., and Anderson, D. R. (2002). Model selection and multimodel inference. A practical information - theoretic approach. Berlin: Springer.
Butterbach-Bahl, K., Gundersen, P., Ambus, P., Augustin, J., Beier, C., Boeckx, P., et al. (2011). “Nitrogen processes in terrestrial ecosystems,” in The European nitrogen assessment, eds M. Sutton, C. M. Howard, J. W. Erisman, G. Billen, A. Bleeker, H. van Grinsven, et al. (Cambridge: Cambridge University Press), 99–125.
Bytnerowicz, A., Johnson, R. F., Zhang, L., Jenerette, G. D., Fenn, M. E., Schilling, S. L., et al. (2015). An empirical inferential method of estimating nitrogen deposition to Mediterranean-type ecosystems: The san bernardino mountains case study. Environ. Pollut. 203, 69–88. doi: 10.1016/j.envpol.2015.03.028
Cape, J. N., Sheppard, L. J., Crossley, A., van Dijk, N., and Tang, Y. S. (2010). Experimental field estimation of organic nitrogen formation in tree canopies. Environ. Pollut. 158, 2926–2933. doi: 10.1016/j.envpol.2010.06.002
Chang, S. C., and Matzner, E. (2000). The effect of beech stemflow on spatial patterns of soil solution chemistry and seepage fluxes in a mixed beech/oak stand. Hydrol. Process. 14, 135–144. doi: 10.1002/(SICI)1099-1085(200001)14:1<135::AID-HYP915<3.0.CO;2-R
Clarke, N., Zlindra, D., Ulrich, E., Mosello, R., Derome, J., Derome, K., et al. (2016). “Part XIV: Sampling and analysis of deposition,” in Manual on methods and criteria for harmonized sampling, assessment, monitoring and analysis of the effects of air pollution on forests, ed. UNECE ICP Forests Programme Co-ordinating Centre (Eberswalde: Thünen Institute of Forest Ecosystems).
De Vries, W., Reinds, G. J., van der Salm, C., Draaijers, G. P. J., Bleeker, A., Erisman, J. W., et al. (2001). Intensive monitoring of forest ecosystems in europe, 2001 technical report. Brussels: EC, UN/ECE, 177.
Draaijers, G. P. J., and Erisman, J. W. (1995). A canopy budget model to assess atmospheric deposition from throughfall measurements. Water Air Soil Pollut. 85, 2253–2258. doi: 10.1007/s11356-017-8861-4
Draaijers, G. P. J., Erisman, J. W., Lövblad, G., Spranger, T., and Vel, E. (1998). Quality and uncertainty aspects of forest deposition estimation using throughfall, stemflow and precipitation measurements. Apeldoorn: TNO.
Draaijers, G. P. J., Erisman, J. W., Spranger, T., and Wyers, G. P. (1996). The application of throughfall measurements for atmospheric deposition monitoring. Atmos. Environ. 30, 3349–3361. doi: 10.1016/1352-2310(96)00030-1
EMEP. (2022). EMEP deposition data. Available online at: https://emep.int/mscw/mscw_moddata.html (accessed December 11, 2022).
Fenn, M. E., Bytnerowicz, A., Schilling, S. L., and Ross, C. S. (2015). Atmospheric deposition of nitrogen, sulfur and base cations in jack pine stands in the athabasca oil sands region, Alberta, Canada. Environ. Pollut. 196, 497–510. doi: 10.1016/j.envpol.2014.08.023
Ferm, M., and Hultberg, H. (1999). Dry deposition and internal circulation of nitrogen, sulphur and base cations to a coniferous forest. Atmos. Environ. 33, 4421–4430. doi: 10.1016/S1352-2310(99)00211-3
Flechard, C. R., Nemitz, E., Smith, R. I., Fowler, D., Vermeulen, A. T., Bleeker, A., et al. (2011). Dry deposition of reactive nitrogen to European ecosystems: A comparison of inferential models across the NitroEurope network. Atmos. Chem. Phys. 11, 2703–2728. doi: 10.5194/acp-11-2703-2011
García-Gomez, H., Izquieta-Rojano, S., Aguillaume, L., González-Fernández, I., Valiño, F., Elustondo, D., et al. (2016). Atmospheric deposition of inorganic nitrogen in Spanish forests of Quercus ilex measured with ion-exchange resins and conventional collectors. Environ. Pollut. 216, 653–661. doi: 10.1016/j.envpol.2016.06.027
García-Gómez, H., Izquieta-Rojano, S., Aguillaume, L., González-Fernández, I., Valiño, F., Elustondo, D., et al. (2018). Joining empirical and modelling approaches to estimate dry deposition of nitrogen in Mediterranean forests. Environ. Pollut. 243, 427–436. doi: 10.1016/j.envpol.2018.09.015
Guerrieri, R., Lecha, L., Mattana, S., Cáliz, J., Casamayor, E. O., Barceló, A., et al. (2020). Partitioning between atmospheric deposition and canopy microbial nitrification into throughfall nitrate fluxes in a Mediterranean forest. J. Ecol. 108, 626–640. doi: 10.1111/1365-2745.13288
Guerrieri, R., Templer, P., and Magnani, F. (2021). Canopy exchange and modification of nitrogen fluxes in forest ecosystems. Curr. For. Rep. 7, 115–137. doi: 10.1007/s40725-021-00141-y
Harrison, A. F., Schulze, E.-D., Gebauer, G., and Bruckner, G. (2000). “Canopy uptake and utilization of atmospheric pollutant nitrogen,” in Carbon and nitrogen cycling in European forest ecosystems. Ecological studies, ed. E. Schulze (Berlin: Springer-Verlag), 171–178. doi: 10.1007/978-3-642-57219-7_8
Hicks, B. B., Hosker, R. P., Meyers, T. P., and Womack, J. D. (1991). Dry deposition inferential measurement techniques-I. Design and tests of a prototype meteorological and chemical system for determining dry deposition. Atmos. Environ. Part A Gen. Top. 25, 2345–2359. doi: 10.1016/0960-1686(91)90109-K
Hůnová, I., Brabec, M., Geletič, J., Malý, M., and Dumitrescu, A. (2021a). Statistical analysis of the effects of forests on fog. Sci. Total Environ. 781:146675. doi: 10.1016/j.scitotenv.2021.146675
Hůnová, I., Brabec, M., Malý, M., Dumitrescu, A., and Geletič, J. (2021b). Terrain and its effects on fog occurrence. Sci. Total Environ. 768:144359. doi: 10.1016/j.scitotenv.2020.144359
Hůnová, I., Kurfürst, P., Vlček, O., Stráník, V., Stoklasová, P., Schovánková, J., et al. (2016). Towards a better spatial quantification of nitrogen deposition: A case study for czech forests. Environ. Pollut. 213, 1028–1041. doi: 10.1016/j.envpol.2016.01.061
ICP Forests (2022). Manual on methods and criteria for hamonized sampling, assessment, monitoring and analysis of the effects of air pollution on forests. Niigata: ICP Forests.
Karlsson, P. E., Akselsson, C., Hellsten, S., and Pihl Karlsson, G. (2022). Twenty years of nitrogen deposition to Norway spruce forests in Sweden. Sci. Total Environ. 809:152192. doi: 10.1016/j.scitotenv.2021.152192
Karlsson, P. E., Pihl Karlsson, G., Hellsten, S., Akselsson, C., Ferm, M., and Hultberg, H. (2019). Total deposition of inorganic nitrogen to Norway spruce forests – applying a surrogate surface method across a deposition gradient in Sweden. Atmos. Environ. 217:116964. doi: 10.1016/j.atmosenv.2019.116964
Kirchner, M., Fegg, W., Römmelt, H., Leuchner, M., Ries, L., Zimmermann, R., et al. (2014). Nitrogen deposition along differently exposed slopes in the Bavarian Alps. Sci. Total Environ. 470–471, 895–906. doi: 10.1016/j.scitotenv.2013.10.036
Korhonen, J. F. J., Pihlatie, M., Pumpanen, J., Aaltonen, H., Hari, P., Levula, J., et al. (2013). Nitrogen balance of a boreal scots pine forest. Biogeosciences 10, 1083–1095. doi: 10.5194/bg-10-1083-2013
Kovács, E. A., and Horváth, L. (2004). Determination of sulfur balance between the atmosphere and a Norway spruce forest ecosystem: Comparison of gradient dry + wet and throughfall deposition measurements. J. Atmos. Chem. 48, 235–240. doi: 10.1023/B:JOCH.0000044417.01498.75
Lindberg, S. E., and Owens, J. G. (1992). Throughfall studies of deposition to forest edges and gaps in montane ecosystems. Biogeochemistry 19, 173–194. doi: 10.1007/BF00000877
Maes, S. L., De Frenne, P., Brunet, J., de la Pena, E., Chabrerie, O., Cousins, S. A. O., et al. (2014). Effects of enhanced nitrogen inputs and climate warming on a forest understorey plant assessed by transplant experiments along a latitudinal gradient. Plant Ecol. 215, 899–910. doi: 10.1007/s11258-014-0341-z
Małek, S. (2010). Nutrient fluxes in planted norway spruce stands of different age in southern Poland. Water Air Soil Pollut. 209, 45–59. doi: 10.1007/s11270-009-0180-z
Marchetto, A., Simpson, D., Aas, W., Fagerli, H., Hansen, K., Pihl-Karlsson, G., et al. (2021). Good agreement between modeled and measured sulfur and nitrogen deposition in Europe, in spite of marked differences in some sites. Front. Environ. Sci. 9:734556. doi: 10.3389/fenvs.2021.734556
Massad, R. S., Nemitz, E., and Sutton, M. A. (2010). Review and parameterisation of bi-directional ammonia exchange between vegetation and the atmosphere. Atmos. Chem. Phys. 10, 10359–10386. doi: 10.5194/acp-10-10359-2010
McDowell, W. H., Pérez-Rivera, K. X., and Shaw, M. E. (2020). “Assessing the ecological significance of throughfall in forest ecosystems,” in Forest-water interactions, ed. D. F. Levia (Berlin: Springer International Publishing), 299–318. doi: 10.1007/978-3-030-26086-6_13
Meixner, T., and Fenn, M. (2004). Biogeochemical budgets in a Mediterranean catchment with high rates of atmospheric N deposition – importance of scale and temporal asynchrony. Biogeochemistry 70, 331–356. doi: 10.1007/s10533-003-4106-3
Moldan, F., Andersson, I., Bishop, K. H., and Hultberg, H. (1998). “Catchment-Scale acidification reversal experiment at Gårdsjön, South-west Sweden: Assessment of the experimental design,” in Experimental reversal of acid rain effects: The Gårdsjön roof project, eds H. Hultberg and R. A. Skeffington (Hoboken, NJ: Wiley & Sons), 85–108.
Neal, C., Robson, A. J., Bhardwaj, C. L., Conway, T., Jeffery, H. A., Neal, M., et al. (1993). Relationships between precipitation, stemflow and throughfall for a lowland beech plantation, Black Wood, Hampshire, southern England: Findings on interception at a forest edge and the effects of storm damage. J. Hydrol. 146, 221–233. doi: 10.1016/0022-1694(93)90277-G
Neirynck, J., Kowalski, A. S., Carrara, A., Genouw, G., Berghmans, P., and Ceulemans, R. (2007). Fluxes of oxidised and reduced nitrogen above a mixed coniferous. Environ. Pollut. 149, 31–43. doi: 10.1016/j.envpol.2006.12.029
Rico, L., Ogaya, R., Terradas, J., and Peñuelas, J. (2014). Community structures of N2-fixing bacteria associated with the phyllosphere of a holm oak forest and their response to drought. Plant Biol. 16, 586–593. doi: 10.1111/plb.12082
Rodrigo, A., Àvila, A., and Rodà, F. (2003). The chemistry of precipitation, throughfall and stemflow in two holm oak (Quercus ilex L.) forests under a contrasted pollution environment in NE Spain. Sci. Total Environ. 305, 195–205. doi: 10.1016/S0048-9697(02)00470-9
Schaub, M., Calatayud, V., Ferretti, M., Brunialti, G., Lövblad, G., Krause, G. H., et al. (2016). “Monitoring of air quality,” in Manual on methods and criteria for harmonized sampling, assessment, monitoring and analysis of the effects of air pollution on forests, ed. ICP Forests Programme Co-ordinating Centre (Eberswalde: Thünen Institute of Forest Ecosystems), 11.
Schmitt, M., Thöni, L., Waldner, P., and Thimonier, A. (2005). Total deposition of nitrogen on swiss long-term forest ecosystem research (LWF) plots: Comparison of the throughfall and the inferential method. Atmos. Environ. 39, 1079–1091. doi: 10.1016/j.atmosenv.2004.09.075
Schrader, F., and Brümmer, C. (2014). Land use specific ammonia deposition velocities: A review of recent studies (2004-2013). Water Air Soil Pollut. 225:2114. doi: 10.1007/s11270-014-2114-7
Schrader, F., Schaap, M., Zöll, U., Kranenburg, R., and Brümmer, C. (2018). The hidden cost of using low-resolution concentration data in the estimation of NH3 dry deposition fluxes. Sci. Rep. 8:969. doi: 10.1038/s41598-017-18021-6
Simpson, D., Bergström, R., Briolat, A., Imhof, H., Johansson, J., Priestley, M., et al. (2020). GenChem v1.0 – a chemical pre-processing and testing system for atmospheric modelling. Geosci. Model Dev. 13, 6447–6465. doi: 10.5194/gmd-13-6447-2020
Sparks, J. P. (2009). Ecological ramifications of the direct foliar uptake of nitrogen. Oecologia 159, 1–13. doi: 10.1007/s00442-008-1188-6
Staelens, J., De Schrijver, A., and Verheyen, K. (2007). Seasonal variation in throughfall and stemflow chemistry beneath a European beech (Fagus sylvatica) tree in relation to canopy phenology. Can. J. For. Res. 37, 1359–1372. doi: 10.1139/X07-003
Staelens, J., Houle, D., De Schrijver, A., Neirynck, J., and Verheyen, K. (2008). Calculating dry deposition and canopy exchange with the canopy budget model: Review of assumptions and application to two deciduous forests. Water Air Soil Pollut. 191, 149–169. doi: 10.1007/s11270-008-9614-2
Thimonier, A. (1998). Measurement of atmospheric deposition under forest canopies: Some recommendations for equipment and sampling design. Environ. Monit. Assess. 52, 353–387. doi: 10.1023/A:1005853429853
Thimonier, A., Kosonen, Z., Braun, S., Rihm, B., Schleppi, P., Schmitt, M., et al. (2019). Total deposition of nitrogen in swiss forests: Comparison of assessment methods and evaluation of changes over two decades. Atmos. Environ. 198, 335–350. doi: 10.1016/j.atmosenv.2018.10.051
Ulrich, B. (1994). “Nutrient and acid-base budget of central European forest ecosystems,” in Effects of acid rain on forest processes, eds D. L. Godbold and A. Hüttermann (New York, NY: Wiley), 1–50.
van der Linde, S., Suz, L. M., Orme, C. D. L., Cox, F., Andreae, H., Asi, E., et al. (2018). Environment and host as large-scale controls of ectomycorrhizal fungi. Nature 558, 243–248. doi: 10.1038/s41586-018-0189-9
Waldner, P., Marchetto, A., Thimonier, A., Schmitt, M., Rogora, M., Granke, O., et al. (2014). Detection of temporal trends in atmospheric deposition of inorganic nitrogen and sulphate to forests in Europe. Atmos. Environ. 95, 363–374. doi: 10.1016/j.atmosenv.2014.06.054
Wesely, M. L., and Hicks, B. B. (2000). A review of the current status of knowledge on dry deposition. Atmos. Environ. 34, 2261–2282. doi: 10.1016/S1352-2310(99)00467-7
Wichink Kruit, R. J., Schaap, M., Sauter, F. J., Van Zanten, M. C., and Van Pul, W. A. J. (2012). Modeling the distribution of ammonia across Europe including bi-directional surface-atmosphere exchange. Biogeosciences 9, 5261–5277. doi: 10.5194/bg-9-5261-2012
Woods, C. L., Hunt, S. L., Morris, D., and Andrew, M. (2012). Epiphytes influence the transformation of nitrogen in coniferous forest canopies. Helsinki: Boreal Environment Publishing Board.
Zhang, L., Vet, R., O’Brien, J. M., Mihele, C., Liang, Z., and Wiebe, A. (2009). Dry deposition of individual nitrogen species at eight Canadian rural sites. J. Geophys. Res. Atmos. 114:D02301. doi: 10.1029/2008JD010640
Keywords: total nitrogen deposition, throughfall, critical loads of nitrogen, canopy uptake, dry deposition of nitrogen, forests
Citation: Braun S, Ahrends B, Alonso R, Augustin S, García-Gómez H, Hůnová I, Karlsson PE, Karlsson GP, Schmitz A and Thimonier A (2022) Nitrogen deposition in forests: Statistical modeling of total deposition from throughfall loads. Front. For. Glob. Change 5:1062223. doi: 10.3389/ffgc.2022.1062223
Received: 05 October 2022; Accepted: 05 December 2022;
Published: 22 December 2022.
Edited by:
Yafeng Zhang, Northwest Institute of Eco-Environment and Resources (CAS), ChinaReviewed by:
Chuan Yuan, Zhejiang Agriculture and Forestry University, ChinaKatarina Zabret, University of Ljubljana, Slovenia
Copyright © 2022 Braun, Ahrends, Alonso, Augustin, García-Gómez, Hůnová, Karlsson, Karlsson, Schmitz and Thimonier. This is an open-access article distributed under the terms of the Creative Commons Attribution License (CC BY). The use, distribution or reproduction in other forums is permitted, provided the original author(s) and the copyright owner(s) are credited and that the original publication in this journal is cited, in accordance with accepted academic practice. No use, distribution or reproduction is permitted which does not comply with these terms.
*Correspondence: Sabine Braun, ✉ sabine.braun@iap.ch