The Impact of Climate Change on Potential Invasion Risk of Oryctes monoceros Worldwide
- 1Department of Biological, Physical and Mathematical Sciences, School of Natural and Environmental Sciences, University of Environment and Sustainable Development, Somanya, Ghana
- 2State Key Laboratory of Resources and Environmental Information Systems, Institute of Geographic Sciences and Natural Resources Research, Chinese Academy of Sciences, Beijing, China
- 3College of Resources and Environment, University of Chinese Academy of Sciences, Beijing, China
- 4Council for Scientific and Industrial Research (CSIR), Oil Palm Research Institute, Coconut Research Programme, Sekondi, Ghana
- 5Centre for Development Research (ZEF), University of Bonn, Bonn, Germany
As a significant threat to agriculture, pests have caused a great disservice to crop production and food security. Understanding the mechanisms of pests’ outbreaks and invasion is critical in giving sound suggestions on their control and prevention strategies. The African rhinoceros beetle, Oryctes monoceros (Olivier), as the most damaging pest of palms, banana, sugarcane, and pineapple, severely threatens their production due to its ability to kill both young and matured hosts. Analyzing the effect of climate change on major parameters of O. monoceros life history has been an important issue recently, given its sensitivity to thermal conditions. However, information on how climate change alters geographical distribution of O. monoceros is poorly understood. By combining environmental variables and occurrence records, we were able to assess environmental risk factors for O. monoceros and create risk maps for the pest using the Boosted Regression Tree model. Our results significance of environmental variables showed that the annual temperature variation (39.45%), seasonality of temperature (23.00%), the isothermality (18.76%), precipitation of the hottest quarter months (6.07%), average variation of day time temperature (3.27%), were relatively important environmental factors that affected the distribution O. monoceros. We also found that the projected potential distributions of the pest’s habitats in all future global warming scenarios exceeded its present known distribution. The model predicts that habitat suitability for O. monoceros is predominantly concentrated along Africa’s west and east coastlines, Asia’s south coasts, South America’s north and east coasts, and a few locations spread over North America’s southern coasts and coastal regions. These outputs provide a solid theoretical foundation for O. monoceros risk evaluations and control.
Introduction
The African rhinoceros beetle, Oryctes monoceros (Olivier; Coleoptera: Scarabaeidae), as a serious pest of palms such as Phoenix dactylifera L. (Kabiru et al., 2014), Elaeis guineensis, Jacq. (Bedford, 2014), and Cocos nucifera L., is responsible for about 40% of coconut losses in tropical Africa (Allou et al., 2006). Apart from palms, O. monoceros attacks non-palm hosts, such as Saccharum officinarum L., Ananas comosus L., and Musa paradisiaca L. O. monoceros inflicts damage to palms in both direct and indirect ways. The beetle directly stunts the growth of the palms by boring and laying eggs in the soft growing points (Bedford, 2013). Indirectly, O. monoceros feeding exposes palms to secondary infestation by the African palm weevil, Rhynchophorus phoenicis L., which enters the palms via holes or galleries created by O. monoceros. At present, O. monoceros has been reported primarily in Africa (Philippe and Dery, 2004; Allou et al., 2006; Edijala et al., 2009; Bila et al., 2019; EPPO, 2021), and Middle East (Arrow, 1937; NHM, 1938; Lepesme, 1947; EPPO, 2021). However, predictions of potential suitable climate regions for preventive and quarantine measures are generally lacking for O. monoceros.
There are over 40 Oryctes species worldwide (Bedford, 1976). Despite the species’ similarities, morphometric features on different life stages, such as adults and larvae, have been critical in distinguishing these species. For example, in O. monoceros, the anteroventral border of the prothoracic shield is rectilinear, whereas in Oryctes rhinoceros Linnaeus, it is scalloped and more concave (Hurpin and Fresneau, 1970). Adults of O. rhinoceros are gregarious, however just a single O. monoceros is seen feeding on the growing point of palms in most occasions (Philippe and Dery, 2004). O. monoceros is found across Africa, whereas O. rhinoceros is widely distributed in South and Southeast Asia (EPPO, 2021). O. monoceros differs from other Oryctes species like Oryctes boas Fabricius and Oryctes gigas Verkrüzen by having a third tooth on the right mandible between the scissorial and molar sections. Furthermore, O. monoceros has raster teges with 78–120 small sharp setae and a lower anal lip with 60–80 similar setae (Bedford, 1979). On the other hand, each claw of O. gigas has three to five long setae, whereas each claw of O. boas has two long setae. The diameter of the O. boas prothoracic shield is almost equal to its length, according to Hurpin (1969), with rectilinear and parallel anterior and posterior edges.
Changes in the earth’s temperature due to an increase in greenhouse gases have been shown to alter the distribution of species (Dhaliwal et al., 2010; Netherer and Schopf, 2010). Insect life cycles and population dynamics will undoubtedly be affected by global warming, including their growth rate, voltinism, and dispersal patterns (Bale et al., 2002), which influence the distribution and seasonal activity of species (Halsch et al., 2021). It can also induce changes in the habitat suitability of agricultural pests, thereby increasing invasion or exacerbating outbreaks and eventually optimizing the likelihood of damage to food production and food security.
Environmental niche models (ENMs) combine species occurrence records and environmental datasets, build a model using a machine learning algorithm, simulate environmental requirements of species, and project the analysis at a different time and space to predict its habitat suitability (Warren and Seifert, 2011; Valencia-Rodríguez et al., 2021). Environmental niche modeling uses correlation and mechanistic or process-based models to estimate species’ habitat requirements. The mechanistic species distribution model (e.g., CLIMEX) considers how environmental conditions constrain the physiological characteristics of species at a given location (Louvrier et al., 2020). In contrast, correlative models establish mathematical correlations between observable species distributions (presence or absence) and environmental variables [e.g., Maximum Entropy, Boosted Regression Trees (BRT), and Random Forest (RF)] (Phillips et al., 2006; Yu et al., 2020). Each model is theoretically distinct, has distinct data requirements, and employs unique analytic techniques. However, the BRT model is considered as a more robust technique than the other ecological niche models (Yu et al., 2020). The model works well with large datasets or when there are a lot of environmental factors compared to the number of observations (Elith et al., 2008). No prior data modification or outlier removal is required to fit the BRT model’s complicated non-linear relationships and automatically manage predictor interaction effects (De’Ath, 2007). The main limitation of single tree models is its low predictive powers, which can be improved by using BRT to fit multiple trees (Elith and Leathwick, 2017). The BRT model has been used to identify habitat suitability for Aedes aegypti and Aedes albopictus (the arbovirus vectors), based on land-cover and environmental variables (Kraemer et al., 2015). The model has also been used to model a mountain pine beetle distribution (Ramazi et al., 2021). The output from such models provides baseline information for monitoring, surveillance, and development of ecologically friendly management strategies (Mu et al., 2013).
Several studies on O. monoceros have focused on its biology and ecology (Ukeh et al., 2003; Allou et al., 2006; Edijala et al., 2009; Kabiru et al., 2014; Gbangboche et al., 2016), and cultural management practices, such as farm sanitation by destroying felled wood and palms or covering them with cover crops to minimize larval breeding (Mariau and Calvez, 1973).
However, due to the high planting density of palms and the expensive expense of these management measures, they are rarely adopted (Allou et al., 2006). Attempts to manage the pests using biological control have been unsuccessful because none of the parasitoids or pathogens (Baculovirus oryctes) have provided a long-lasting solution to the threat posed by O. monoceros (Seguni et al., 1999). In addition, application of synthetic pesticides has not been recommended due to environmental and health risks (Parven et al., 2021). In Ghana, a new method for controlling O. monoceros in coconut plantations is extracting adults using a metal mini-harpoon from their feeding galleries (Philippe and Dery, 2004). This mechanical control strategy is labor-intensive and costly in high-density palm plantations. Also, since preventive measures are economically cheaper than post entry management (Arthur et al., 2015), creating risk maps would serve as an early warning against the future spread, especially in areas where the pest is currently absent.
The average global temperatures would rise ranging from 2 and 9.7°F (1.1 to 5.4°C) by 2100 in a variety of greenhouse gas emission scenarios (Herring, 2012). In recent decades, it is accepted that a rise in global temperature is considered as one of the causes of the drastic change in climate (Soytas and Sari, 2006; Karki, 2007). Climate change is an important factor restricting insects’ survival and population growth (Vanhanen et al., 2007). However, there is a scarcity of information on the effects of climate change on the global distribution of O. monoceros as well as identifying environmental factors driving the geographical distribution for surveillance, monitoring and preventive programs is generally lacking. To address this research gap, we simulated the potential distribution of O. monoceros under different climate change scenarios to identify climate suitable areas for biosecurity and risk assessment.
Materials and Methods
Our research included two key procedures: (i) obtaining occurrence records and climate datasets; and (ii) predicting the pest’s existing and future potential environmental niche shifts. The BRT modeling framework is a prominent technique to ecological studies that has been used to accurately forecast the potential geographic range of many species (Jiang et al., 2019; Kraemer et al., 2019; Chen et al., 2020; Ding et al., 2020). The BRT modeling approach combines the advantages of regression trees (Breiman et al., 1984) and gradient boosting (Friedman, 2001), and has a good ability in fitting complex non-linear response functions from outcomes based on given environmental covariates. To assess the effects of climate change on environmental suitability for O. monoceros, we used the following datasets as key input data for modeling analysis: (a) the assembled contemporary occurrence records of O. monoceros, and a set of pseudo-absence points of pest; (b) a set of environmental covariates reflecting a baseline scenario for the year 2020; and (c) a set of environmental covariates reflecting two representative concentration pathways (RCPs) (R 4.5 and 8.5) scenarios on future periods (the 2030s, 2050s, and 2080s).
Occurrence Records and Pseudo-Absence Points
A 3-year nationwide survey for O. monoceros was conducted in palm plantations in Ghana to obtain the pest’s occurrence records. The sampling consisted of visual observation for the presence of any developmental stage (eggs, larvae, pupae, and adults), as well as symptomatic fronds, feeding galleries, and debris. A handheld GPS device (Garmin eTrex 22x), was used to obtain GPS coordinates of locations where O. monoceros was collected in the field. Having enough data points for accurate modeling is critical for optimal model performance, therefore the field data were supplemented by a comprehensive scientific literature search using internet sources, such as Web of Science, Science Direct, Google Scholar, and PubMed (Arrow, 1937; NHM, 1938; Lepesme, 1947; Julia and Mariau, 1976; Lomer, 1986; Gries et al., 1994; Seguni et al., 1999; Ukeh et al., 2003; Philippe and Dery, 2004; Allou et al., 2006, 2008, 2012; Edijala et al., 2009; Bedford, 2013; Kabiru et al., 2014; Gbangboche et al., 2016; Séré et al., 2018; Bila et al., 2019; Idowu et al., 2019). Google Earth Pro version 7.3 was used to extract the coordinates (i.e., latitudes and longitudes) and elevation information if only locations were provided. In addition, we manually deleted redundant and ambiguous datasets, and those with evident geocoding issues. Finally, 323 O. monoceros records were discovered worldwide (Supplementary Table 1). An equivalent number of pseudo-absence points were randomly selected from a previously described approach (Senay et al., 2013). The following steps were used to obtain the pseudo-absence records; to begin with, the pseudo-absence locations were randomly selected from the background points. In addition, the pseudo-absence locations were created within a delimited geographical distance from recorded occurrence points. Finally, we selected pseudo-absence locations with environmentally dissimilar conditions from the occurrence points.
Environmental Variables
The environmental factors chosen as the starting variables to be used in the modeling in this study are shown in Table 1, for the various reasons described above. The environmental variables, which included 19 bioclimatic variables, were downloaded with a spatial resolution of 5 km from the WorldClim Global environmental database.1 In its AR5 assessment, the Intergovernmental Panel on Climate Change (IPCC) chose four RCPs to depict the future climate scenario, namely RCPs 2.6, 4.5, 6.0, and RCP8.5 (Remya et al., 2015). The future environmental variables generated by the MIROC-ESM-CHEM model for two climatic scenarios (RCPs 4.5 and 8.5) were taken from the Climatic Change, Agriculture and Food Security website.2
Modeling Analysis
The “dismo” package was used to run the modeling analysis process in the R 3.3.3 statistical programming environment. Also, recommendations from scientific literature were consulted for the BRT model projections (Jiang et al., 2019, 2021; Zheng et al., 2019). This allowed us to specify key BRT model parameter values. As an initial step, we used O. monoceros records and pseudo-absence points as well as environmental variables to create a baseline scenario for 2020. Pseudo-absence points were minimized by constructing an ensemble of 30 BRT models, and this helped make our modeling technique more resilient. Then, the BRT models were merged with a set of future environmental covariates to generate O. monoceros environmental suitability maps for the future. By averaging 30 BRT models, the maps of the world’s future geographic distribution were created. In order to avoid overfitting, a tenfold cross-validation area under the receiver operating characteristic curve (ROC-AUC) was chosen. The ROC curve and the area under the ROC curve were used to evaluate the model’s accuracy. AUC values range between 0 and 1. As a general rule, an AUC less than 0.9 implies that a model’s prediction accuracy is low, while an AUC more than 0.9 suggests that the model’s accuracy is outstanding. As this value approaches 1, the model’s performance improves (Phillips et al., 2006). We utilized the ArcGIS software’s inbuilt Tabulate Area tool (version 10.1) to calculate the habitat suitability for O. monoceros.
Results
Performance of the Model and the Significance of Environmental Variables
The O. monoceros model prediction accuracy was shown to be “excellent” for the current period (AUC mean = 0.992). The results showed that the variables chosen correctly predicted the current O. monoceros distribution. Table 1 listed the relative contribution of the environmental variables, which showed that the annual temperature variation contributed most to the model (39.45 ± 11.40%), followed by the seasonality of temperature (23.00% ± 8.5%), the isothermality (18.76% ± 12%), precipitation of the hottest quarter months (6.07% ± 3.46%), and average variation of day time temperature (3.27% ± 1.95%) (Table 2). The other variables accounted for less than 1% of the total contribution.
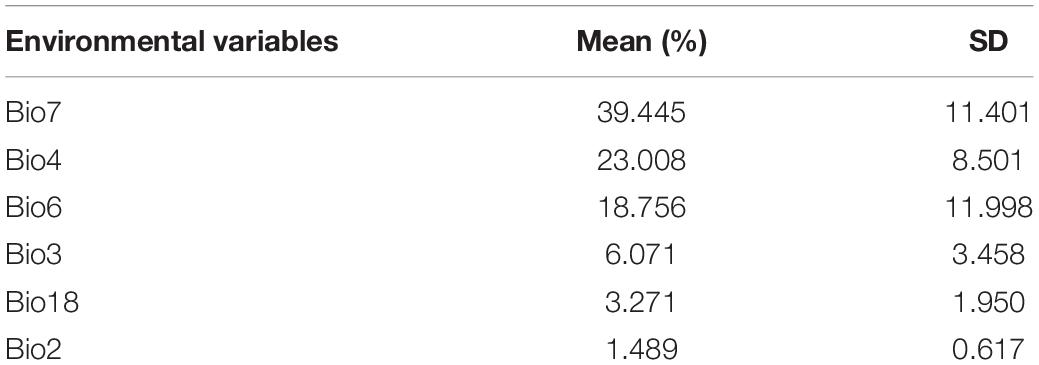
Table 2. Environmental variables’ proportional contributions to predicting global habitat suitability for O. monoceros occurrence.
Global Potential Distribution Oryctes monoceros
Under the current time, the prediction of habitat suitability for O. monoceros is illustrated in Figure 1. The model results are in line with the present-day known distribution of the pest. According to the model, suitable areas for O. monoceros are largely found in Africa’s east, south, and west coastlines, South America’s north and east coasts, Asia’s south and east coasts, and Oceania’s north coast. The prediction demonstrates that the habitat suitability for the pest spans all continents, although the high suitability areas are found in Asia, Africa, Europe, and the Americas. The major oil palm producing countries, such as Thailand, Indonesia, Malaysia, Nigeria, and Colombia, are predicted to be favorable for O. monoceros. Similarly, the model forecasts habitat suitability in Indonesia, the Philippines, India, Brazil, and Sri Lanka, all of which produce considerable quantities of coconut. Finally, the predictions show that generally Egypt, Algeria, Iran, and Saudi Arabia, the world’s leading producers of date palms are not suitable for O. monoceros.
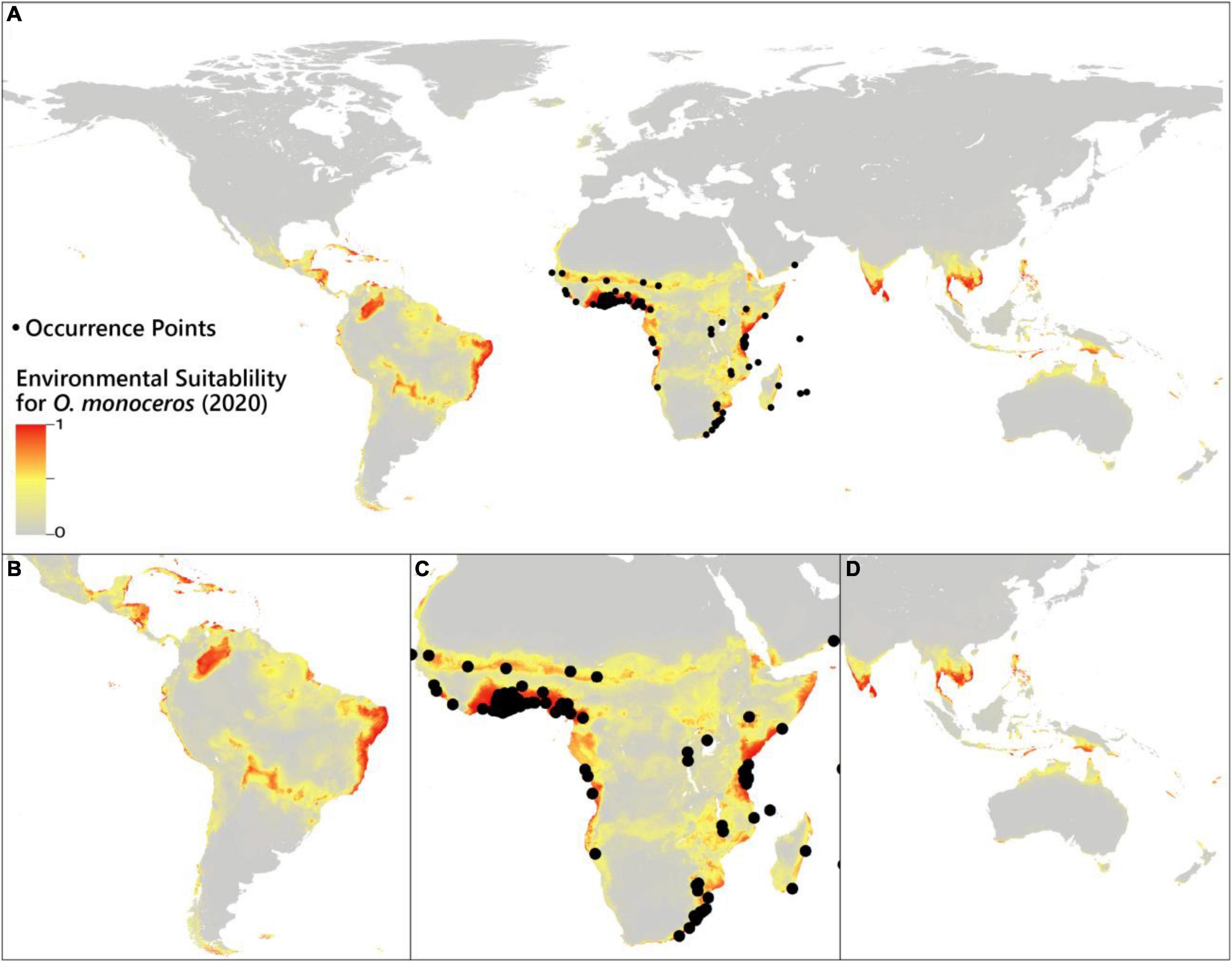
Figure 1. Suitability of the environment for the occurrence of O. monoceros. Data for (A) global probability (B) South America and sections of Central America, (C) Africa, and (D) Asia and Oceania.
In the RCP 4.5 scenario, the BRT model predicted that high suitability areas are mainly distributed in northern parts of southern America, central Africa, southern Asia, and southeastern Asia. In addition, most contraction of suitability was focused on parts of South America, and scattered throughout Africa. While suitability in parts of Africa and Asia shows an expanded trend. The potential geographical distribution of O. monoceros was quantified and the results presented in Table 3. The potential global suitable areas of O. monoceros are shown in Figures 2A–C and presented in Table 3. The model predicts changes in zones of areas at risk of O. monoceros invasion (Figures 2D–F). Also, the model predicts more suitable areas in future (7,236,100 km2) than the current time (6,525,300 million km2).
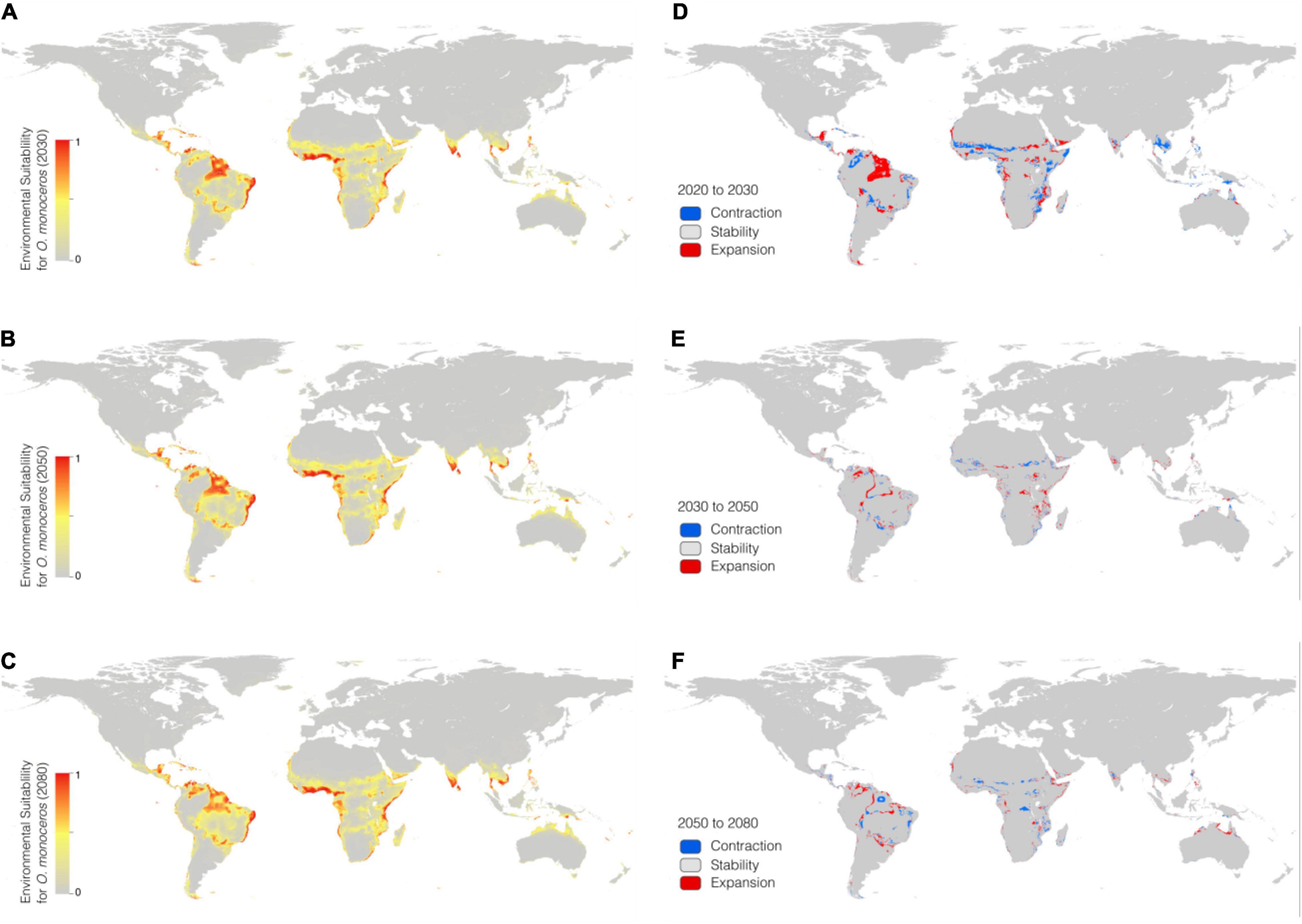
Figure 2. Geographical distribution of O. monoceros predicted by RCP4.5 for the future worldwide [2030 (A), 2050 (B), and 2080 (C)]. Changes in zones expected to be at risk (D–F).
In the RCP 8.5 scenario (Figures 3A–C), the suitable areas will increase from the current time (6,525,300 km2) to 2080 (7,236,100 km2) (Table 3). The suitable areas of O. monoceros were found in all continents, with the model predicting either contraction or expansion of suitable areas (Figures 3D–F). The future expansion of suitable areas suggests that there is a need for regular monitoring and surveillance, especially where it is currently absent. Although the model predicts changes in suitable areas in the RCP 4.5 and 8.5 scenarios, more areas will continue to be suitable for O. monoceros in the future, especially coastal belts (Figures 2A–F, 3A–F).
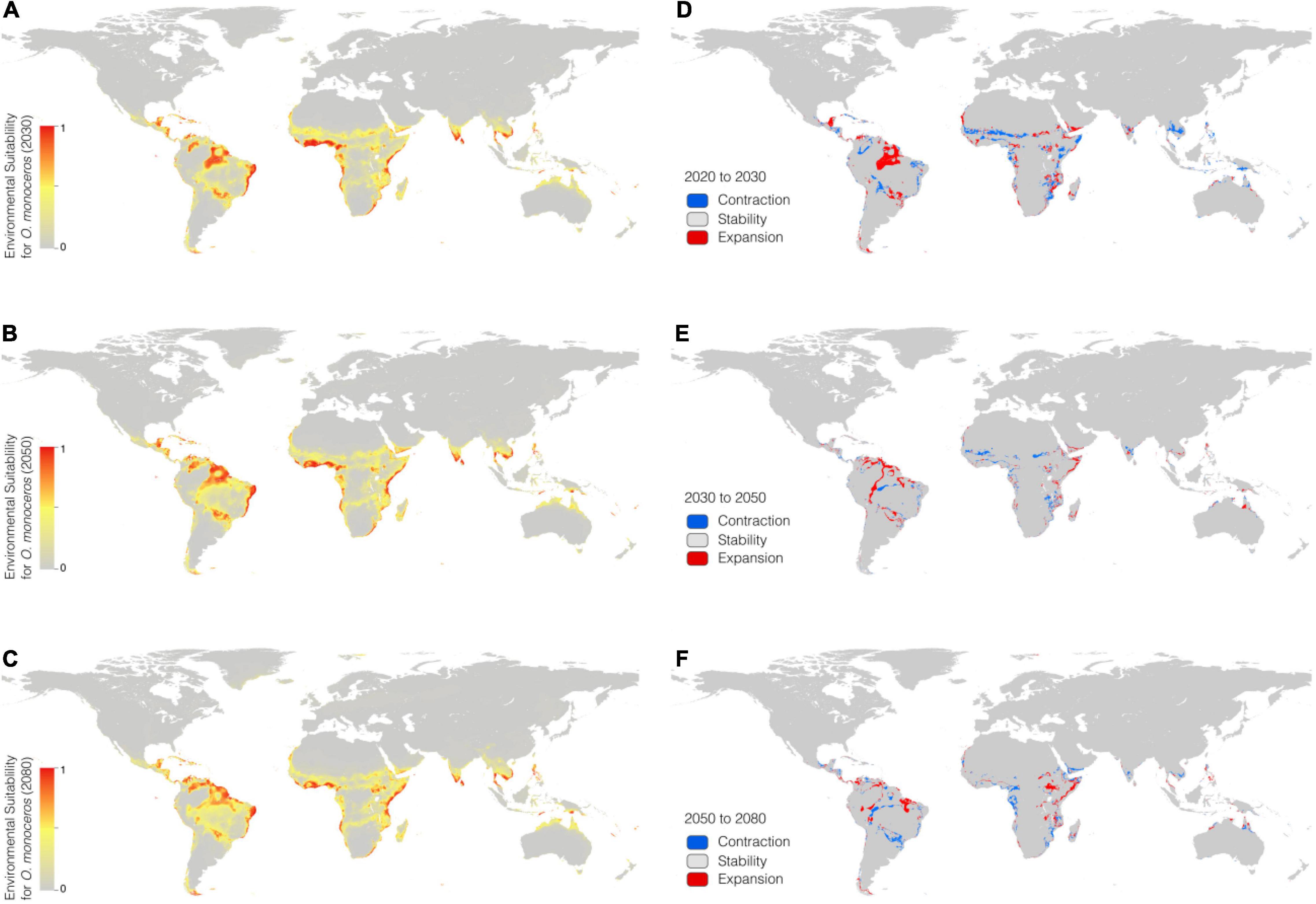
Figure 3. Geographical distribution of O. monoceros predicted by RCP8.5 for the future worldwide [2030 (A), 2050 (B), and 2080 (C)]. Changes in zones expected to be at risk (D–F).
Discussion
The occurrence datasets used in the modeling were mostly taken from scientific papers. This is consistent with the open science movement’s definition, which encourages data reuse for further research and decision-making (Allen and Mehler, 2019). As a result, some studies have used historical species data to estimate invasion risk areas (Jiang et al., 2018; Kraemer et al., 2019; Guo et al., 2021; Zhou et al., 2021; Aidoo et al., 2022a,b). The model outputs from such studies have been utilized in developing surveillance, monitoring, and preventive measures against invasive pests (Hao et al., 2022).
The African rhinoceros beetle has been observed in Africa and Asia (Ukeh et al., 2003), showing an expanding trend in recent decades. O. monoceros, an important pest of palms in tropical Africa (Allou et al., 2006), causes server damage to these plants, thus leading to economic losses. Therefore, predicting O. monoceros suitable areas can help monitor the pest, and develop early warning, and management programmes wherever it occurs. In this study, we used the BRT model and ArcGIS to understand the potential climatic risk factors and estimate the global distribution of O. monoceros under climate change.
The environmental factors that influence the pest distribution were annual temperature variation, followed by the seasonality of temperature, the isothermality, precipitation of the hottest quarter months, and average variation of day temperature. This suggests that temperature factors contribute more to the global geographical distribution of the pest, which may explain why temperatures and relative humidity ranging from 27 to 29°C and 85 to 95%, respectively, are ideal for larval growth (Bedford, 1980).
The African rhinoceros beetle has been reported in two Asian countries: Yemen and Saudi Arabia. According to our predictions, extensive areas of Asia, though remaining uninfested at present, are highly favorable to the pest. For example, the southeastern parts of India, Vietnam, Cambodia, and Thailand are predicted to be suitable for O. monoceros. In the present study, our model predicts high habitat suitability in the world’s largest coconut-producing countries, such as Indonesia, Philippines, India, Sri Lanka, Brazil, Vietnam, Papua New Guinea, Mexico, Thailand, and Myanmar (FAOSTAT, 2019). These largest coconut-producing countries will remain suitable to O. monoceros in the future. In addition, Indonesia, Malaysia, Thailand, Colombia, Nigeria, Guatemala, Honduras, Papua New Guinea, Ecuador, and Brazil, as the leading producers of oil palm (FAOSTAT, 2019), were all predicted to have suitable areas for the pest. Our predictions show habitat suitability for O. monoceros in Sudan and Saudi Arabia, which is quite discouraging for the date palm industry in these regions since these countries are among the major date palm producing countries in the world. These regions should be alert to the possibility of O. monoceros invasion.
In this study, the BRT model predicted habitat suitability for O. monoceros in areas where the Asiatic rhinoceros beetle O. rhinoceros Linnaeus (Coleoptera: Scarabaeidae) is currently present. O. monoceros and O. rhinoceros are native to Africa and Asia, respectively (Hinckley, 1973). Oryctes rhinoceros has spread outside its native range inflicting damage to economically important palms in the Americas, Oceania and Africa (Giblin-Davis, 2001). The countries affected by O. rhinoceros include Mauritius, Nigeria, and Reunion in Africa; India, Indonesia, Philippines, Sri Lanka, and Vietnam in Asia; Texas and Hawaii in the Americas; Papua New Guinea and American Samoa in Oceania (Catley, 1969; Onyeike et al., 2005; Okaraonye and Ikewuchi, 2009; Olowu et al., 2012; EPPO, 2022). O. monoceros has not been reported from Oceania, Southeast Asia, and the Americas. As predicted in this study, parts of these areas have medium to high habitat suitability for O. monoceros. Given the substantial economic impacts of Oryctes species, the invasion of O. monoceros in countries where O. rhinoceros occurs could pose a severe threat to food security and biodiversity conservation. Therefore, our risk maps would guide regular monitoring and surveillance of O. monoceros, thereby controlling the pest in an ecologically friendly.
Global warming would cause changes in species distributions (Kiritani, 2006; Freeman et al., 2018; Chen et al., 2019). Our results indicated that future predicted suitable areas occurred outside the present distribution of O. monoceros. The new areas were mainly found in South America, the Caribbean, Asia, and Oceania. On the one hand, our predictions revealed that the distribution of O. monoceros would expand under future climate scenarios (present < 2030 < 2050 < 2080), indicating that there would be more suitable habitats for O. monoceros distribution in the future. The range shift was more noticeable in Central America than in any other region. The predicted range contraction for O. monoceros in this study could be useful for pest control and eradication. The range of habitat suitability will potentially contrast in some parts of the world, making this pest less competitive and possibly contributing to its eradication. On the other hand, a few areas are predicted to remain suitable and may require regular monitory and surveillance for early detection and control, especially in regions such as parts of Australia that currently have no occurrence records of O. monoceros. The predicted suitable areas are primarily in line with earlier pest occurrence records and show expansion of the pest’s geographical distribution.
Our study identifies the regions where O. monoceros would most likely occur, as well as the relationship between the environmental indicators of this pest and the risk of its invasion, especially in areas where it has not yet been observed. It is important to note that there are some limitations in this study. Firstly, the environmental suitability maps generated from the ensemble BRT models can be interpreter to predict the potential geographical distribution of the O. monoceros, rather than where the pest will be spread in the future. Secondly, factors such as the interaction of the invader’s characteristics (e.g., high propagule output), the abiotic environment (especially disturbance), and biotic interactions within novel environments (e.g., enemy-release) (Sakai et al., 2001; Keane and Crawley, 2002; Shea and Chesson, 2002) were not included in the BRT model because of unavailability of data. However, these biotic and abiotic factors are involved in the ability of a pest to establish in a new geographical area. Among them, competitors, the ability to survive in the absence of hosts, and phenotypic plasticity are considered important influencers of the establishment of species outside their native range (Lee and Lee, 2006; Aidoo et al., 2021). Thirdly, local policies, such as the inspection of plants, plant parts, farm machinery, and plant by-products at entry ports, can affect the establishment of this species outside of its natural range (McNeely, 2000; Pyšek et al., 2020). It is our recommendation that the implementation of plant protection and regulatory procedures, the development of programs to monitor the quality of plant products and byproducts, as well as an increase in academic awareness and the transfer of technology can help prevent the spread of this pest in areas where it has not yet been detected (Shine, 2007; Early et al., 2016).
Conclusion
In this study, we have combined the BRT models with O. monoceros occurrence and environmental data to predict the potential geographical distribution of the pest. The AUC value of the model was excellent, suggesting that our model predictions are accurate and can be used to inform policy formulation and preventive measures. From now until 2080, we found that the worldwide habitat suitability for O. monoceros increased, as predicted by the BRT model. Therefore, to prevent more O. monoceros invasions in the face of present and future climate change scenarios, many of the world’s largest oil palm, coconut, and date palm plantations must take preventative steps.
Data Availability Statement
The original contributions presented in this study are included in the article/Supplementary Material, further inquiries can be directed to the corresponding authors.
Author Contributions
OFA, MH, FD, TM, and YQ wrote the manuscript. FD, OFA, TM, NY, CB, ET, and KD conceived and designed the research. FD, DJ, TM, OFA, DW, MH, and TM analyzed the data. All authors reviewed the manuscript.
Funding
This research was supported and funded by the Strategic Priority Research Program of the Chinese Academy of Sciences (XDA20010203).
Conflict of Interest
The authors declare that the research was conducted in the absence of any commercial or financial relationships that could be construed as a potential conflict of interest.
Publisher’s Note
All claims expressed in this article are solely those of the authors and do not necessarily represent those of their affiliated organizations, or those of the publisher, the editors and the reviewers. Any product that may be evaluated in this article, or claim that may be made by its manufacturer, is not guaranteed or endorsed by the publisher.
Acknowledgments
We thank the University of Environment and Sustainable Development and Council for Scientific and Industrial Research, both in Ghana, and the State Key Laboratory of Resources and Environmental Information Systems, Institute of Geographical Sciences and Natural Resources Research, Chinese Academy of Sciences, Beijing, China for supporting this study. We also thank the reviewers for the constructive and valuable comments and the editors for their assistance in refining this article.
Supplementary Material
The Supplementary Material for this article can be found online at: https://www.frontiersin.org/articles/10.3389/fevo.2022.895906/full#supplementary-material
Footnotes
References
Aidoo, O. F., Cunze, S., Guimapi, R. A., Arhin, L., Ablormeti, F. K., Tettey, E., et al. (2021). Lethal yellowing disease: insights from predicting potential distribution under different climate change scenarios. J. Plant. Dis. Prot. 1-13, doi: 10.1007/s41348-021-00488-1
Aidoo, O. F., da Silva, R. S., Santana Junior, P. A., Souza, P. G. C., Kyerematen, R., Owusu-Bremang, F., et al. (2022a). Model-based prediction of the potential geographical distribution of the invasive coconut mite, Aceria guerreronis Keifer (Acari: Eriophyidae) based on MaxEnt. Agric. For. Entomol. [Preprint]. doi: 10.1111/afe.12502
Aidoo, O. F., Souza, P. G. C., da Silva, R. S., Santana, P. A., Picanço, M. C., Kyerematen, R., et al. (2022b). Climate-induced range shifts of invasive species (Diaphorina citri Kuwayama). Pest Manage. Sci. 78, 2534–2549. doi: 10.1002/ps.6886
Allen, C., and Mehler, D. M. (2019). Open science challenges, benefits and tips in early career and beyond. PLoS Biol. 17:e3000246. doi: 10.1371/journal.pbio.3000246
Allou, K., Issali, A. E., Lekadou, T., Konan, K. J. L., Zakra, N., Kouassi, K. P., et al. (2012). Comparative synergetic effect of coconut palm (Cocos nucifera L.) slices and bunches residue of oil palm (Elaeis guineensis Jacq.) associated with two kinds of pheromone traps on Oryctes monoceros Olivier trapping in Côte d’Ivoire. Int. J. Emerg. Tech. Adv. Eng. 2, 1–6.
Allou, K., Morin, J. P., Kouassi, P., N’klo, F. H., and Rochat, D. (2006). Oryctes monoceros trapping with synthetic pheromone and palm material in Ivory Coast. J. Chem. Ecol. 32, 1743–1754. doi: 10.1007/s10886-006-9106-z
Allou, K., Morin, J. P., Kouassi, P., N’klo, F. H., and Rochat, D. (2008). Sex ratio and female sexual status of the coconut pest, Oryctes monoceros (Coleoptera: Dynastidae), differ in feeding galleries and pheromone-baited traps. Bull. Entomol. Res. 98, 581–586. doi: 10.1017/S0007485308005890
Arrow, G. J. (1937). “Pars 156: Scarabaeidae: Dynastinae,” in Coleopterorum Catalogus. Volumen XXI. Scarabaeidae III, ed. S. Schenkling (Berlin: W Junk), 1–124.
Arthur, T., Summerson, R., and Mazur, K. (2015). A comparison of the costs and effectiveness of prevention, eradication, containment and asset protection of invasive marine species incursions. Canberra: ABARES.
Bale, J. S., Masters, G. J., Hodkinson, I. D., Awmack, C., Bezemer, T. M., Brown, V. K., et al. (2002). Herbivory in global climate change research: direct effects of rising temperature on insect herbivores. Glob. Change Biol. 8, 1–16. doi: 10.1046/j.1365-2486.2002.00451.x
Bedford, G. O. (1976). Observations on the biology and ecology of Oryctes rhinoceros and Scapanes australis (Coleoptera: Scarabaeidae: Dynastinae): pests of coconut palms in Melanesia. Aust. J. Entomol. 15, 241–251.
Bedford, G. O. (1979). Description of the larva of Oryctes monoceros (Olivier) (Coleoptera: Scarabpidae: Dynastinp) from the Seychelles. Bull. Entomol. Res. 69, 599–604.
Bedford, G. O. (1980). Biology, ecology and control of palm rhinoceros beetles. Annu. Rev. Entomol 25, 309–339. doi: 10.1146/annurev.en.25.010180.001521
Bedford, G. O. (2013). Biology and management of palm dynastid beetles: recent advances. Annu. Rev. Entomol 58, 353–372. doi: 10.1146/annurev-ento-120710-100547
Bedford, G. O. (2014). Advances in the control of rhinoceros beetle. Oryctes rhinoceros in oil palm. J. Oil Palm Res. 26, 183–194.
Bila, J., Mondjana, A., Samils, B., Santos, L., and Hogberg, N. (2019). Integrated Management of Coconut Lethal Yellowing Phytoplasma Disease in Mozambique: Current Challenges and Future Perspectives. In Sustainable Management of Phytoplasma Diseases in Crops Grown in the Tropical Belt. Cham.: Springer, 233–249.
Breiman, L., Friedman, J. H., Olshen, R. A., and Stone, C. J. (1984). Classification And Regression Trees, 1st Edn. Milton Park: Routledge, doi: 10.1201/9781315139470
Catley, A. (1969). The coconut rhinoceros beetle Oryctes rhinoceros (L) [Coleoptera: Scarabaeidae: Dynastinae]. PANS Pest Articles News Summ. 15, 18–30. doi: 10.1080/04345546909415075
Chen, L., Huang, J. G., Ma, Q., Hänninen, H., Tremblay, F., and Bergeron, Y. (2019). Long-term changes in the impacts of global warming on leaf phenology of four temperate tree species. Glob. Change Biol. 25, 997–1004. doi: 10.1111/gcb.14496
Chen, S., Ding, F., Hao, M., and Jiang, D. (2020). Mapping the potential global distribution of red imported fire ant (Solenopsis invicta Buren) based on a machine learning method. Sustainability 12:10182. doi: 10.3390/su122310182
De’Ath, G. (2007). Boosted trees for ecological modeling and prediction. Ecology 88, 243–251. doi: 10.1890/0012-9658(2007)88[243:BTFEMA]2.0.CO;2
Dhaliwal, G. S., Jindal, V., and Dhawan, A. K. (2010). Insect pest problems and crop losses: changing trends. Ind. J. Ecol. 37, 1–7.
Ding, F., Ma, T., Hao, M., Wang, Q., Chen, S., Wang, D., et al. (2020). Mapping Worldwide Environmental Suitability for Artemisia annua L. Sustainability. 12:1309. doi: 10.3390/su12041309
Early, R., Bradley, B. A., Dukes, J. S., Lawler, J. J., Olden, J. D., Blumenthal, D. M., et al. (2016). Global threats from invasive alien species in the twenty-first century and national response capacities. Nat. Commun. 7:12485. doi: 10.1038/ncomms12485
Edijala, J. K., Egbogbo, O., and Anigboro, A. A. (2009). Proximate composition and cholesterol concentrations of Rhynchophorus phoenicis and Oryctes monoceros larvae subjected to different heat treatments. Afr. J. Biotechnol. 8, 2346–2348.
Elith, J., and Leathwick, J. (2017). Boosted Regression Trees for ecological modeling. R Documentation. Available online at: https://cran.r-project.org/web/packages/dismo/vignettes/brt.pdf (accessed on 12 June 2011).
Elith, J., Leathwick, J. R., and Hastie, T. (2008). A working guide to boosted regression trees. J. Anim. Ecol. 77, 802–813.
Freeman, B. G., Lee-Yaw, J. A., Sunday, J. M., and Hargreaves, A. L. (2018). Expanding, shifting and shrinking: The impact of global warming on species’ elevational distributions. Glob. Ecol. Biogeogr. 27, 1268–1276. doi: 10.1111/geb.12774
Friedman, J. H. (2001). Greedy function approximation: a gradient boosting machine. Ann. Stat. 29, 1189–1232. doi: 10.1214/aos/1013203451
Gbangboche, A. B., Tognibo, B. A. C., Kayode, P., Zannou, E. T., and Codjia, J. T. C. (2016). Croissance et valeur alimentaire des larves de Oryctes monoceros. Int. J. Biol. Chem. Sci. 10, 983–992. doi: 10.4314/ijbcs.v10i3.6
Gries, G., Gries, R., Perez, A. L., Oehlschlager, A. C., Gonzales, L. M., and Pierce, H. D. Jr., et al. (1994). Aggregation pheromone of the African rhinoceros beetle, Oryctes monoceros (Olivier) (Coleoptera: Scarabaeidae). Z. Naturforsch. C 49, 363–366. doi: 10.1515/znc-1994-5-614
Guo, S., Ge, X., Zou, Y., Zhou, Y., Wang, T., and Zong, S. (2021). Projecting the Global Potential Distribution of Cydia pomonella (Lepidoptera: Tortricidae) Under Historical and RCP4. 5 Climate Scenarios. J. Insect. Sci. 21:15. doi: 10.1093/jisesa/ieab024
Halsch, C. A., Shapiro, A. M., Fordyce, J. A., Nice, C. C., Thorne, J. H., Waetjen, D. P., et al. (2021). Insects and recent climate change. Proc. Natl. Acad. Sci. U.S.A. 118:e2002543117. doi: 10.1073/pnas.2002543117
Hao, M., Aidoo, O. F., Qian, Y., Wang, D., Ding, F., Ma, T., et al. (2022). Global potential distribution of Oryctes rhinoceros, as predicted by boosted regression tree model. Glob. Ecol. Conserv. 37:e02175. doi: 10.1016/j.gecco.2022.e02175
Herring, D. (2012). Climate Change: Global Temperature Projections. Available online at: https://www.climate.gov/news-features/understanding-climate/climate-change-global-temperature-projections (accessed on 9 April 2022).
Hinckley, A. D. (1973). Ecology of the coconut rhinoceros beetle, Oryctes rhinoceros (L.) (Coleoptera: Dynastidae). Biotropica 111–116.
Hurpin, B., and Fresneau, M. (1970). Etude en laboratoire du developpement larvaire de Oryctes monoceros et O. rhinoceros (Col. scarabaeidae). Soc. Entomol. France Ann. 6, 193–214.
Idowu, A. B., Oliyide, E. O., Ademolu, K. O., and Bamidele, J. A. (2019). Nutritional and anti-nutritional evaluation of three edible insects consumed by the Abeokuta community in Nigeria. Int. J. Trop. Insect Sci. 39, 157–163. doi: 10.1007/s42690-019-00021-w
Jiang, D., Chen, S., Hao, M., Fu, J., and Ding, F. (2018). Mapping the potential global codling moth (Cydia pomonella L.) distribution based on a machine learning method. Sci. Rep. 8:13093. doi: 10.1038/s41598-018-31478-3
Jiang, D., Ma, T., Hao, M., Qian, Y., Chen, S., Meng, Z., et al. (2021). Spatiotemporal patterns and spatial risk factors for visceral leishmaniasis from 2007 to 2017 in Western and Central China: A modelling analysis. Sci. Total Environ. 764:144275. doi: 10.1016/j.scitotenv.2020.144275
Jiang, D., Wang, Q., Ding, F., Fu, J., and Hao, M. (2019). Potential marginal land resources of cassava worldwide: A data-driven analysis. Renew. Sustain. Energ. Rev. 104, 167–173. doi: 10.1016/j.rser.2019.01.024
Julia, J. F., and Mariau, D. (1976). Research on Oryctes monoceros Ol. in the Ivory Coast. I. Biological control. Role of the cover plant. Oléagineux 31, 63–68.
Kabiru, S. M., Abiodun, O. C., and Rahman, Y. S. A. (2014). Control of Oryctes monoceros on date palm field in Sudan-savannah vegetative zone of Nigeria. World 2, 285–290.
Karki, K. B. (2007). Greenhouse gases, global warming and glacier ice melt in Nepal. J. Agric. Environ. Int. Dev. 8, 1–7. doi: 10.3126/aej.v8i0.721
Keane, R. M., and Crawley, M. J. (2002). Exotic plant invasions and the enemy release hypothesis. Trends Ecol. Evol. 17, 164–170. doi: 10.1016/S0169-5347(02)02499-0
Kiritani, K. (2006). Predicting impacts of global warming on population dynamics and distribution of arthropods in Japan. Popul. Ecol. 48, 5–12. doi: 10.1093/jme/tjx200
Kraemer, M. U., Reiner, R. C., Brady, O. J., Messina, J. P., Gilbert, M., Pigott, D. M., et al. (2019). Past and future spread of the arbovirus vectors Aedes aegypti and Aedes albopictus. Nat. Microbiol. 4, 854–863. doi: 10.1038/s41564-019-0376-y
Kraemer, M. U., Sinka, M. E., Duda, K. A., Mylne, A. Q., Shearer, F. M., Barker, C. M., et al. (2015). The global distribution of the arbovirus vectors Aedes aegypti and Ae. albopictus. eLife 4, e08347. doi: 10.7554/eLife.08347
Lee, H. W., and Lee, C. S. (2006). Environmental factors affecting establishment and expansion of the invasive alien species of tree of heaven (Ailanthus altissima) in Seoripool Park, Seoul. Integr. Biosci. 10, 27–40. doi: 10.1080/17386357.2006.9647281
Lomer, C. J. (1986). Release of Baculovirus oryctes into Oryctes monoceros populations in the Seychelles. J. Invertebr. Pathol. 47, 237–246. doi: 10.1016/0022-2011(86)90093-5
Louvrier, J., Papaïx, J., Duchamp, C., and Gimenez, O. (2020). A mechanistic–statistical species distribution model to explain and forecast wolf (Canis lupus) colonization in South-Eastern France. Spat. Stat. 36:100428. doi: 10.1016/j.spasta.2020.100428
Mariau, D., and Calvez, C. (1973). Méthode de lutte contre l’Oryctes en replantations de palmier à huile. Oléagineux 28, 215–218.
McNeely, J. A. (2000). Global strategy for addressing the problem of invasive alien species. A result of the global invasive alien species (GISP). Gland: IUCN-The World Conservation Union.
Mu, J., Luo, N., Chang, W., Huang, K., and Shi, M. (2013). Predicting and mapping the spatial distribution of Chamaecyparis formosensis in central Taiwan in a GIS with species distribution models. Acta Ecol. Sin. 33, 325–331. doi: 10.1016/j.chnaes.2013.09.006
Netherer, S., and Schopf, A. (2010). Potential effects of climate change on insect herbivores in European forests—general aspects and the pine processionary moth as specific example. For. Ecol. Manag. 259, 831–838. doi: 10.1016/j.foreco.2009.07.034
NHM (1938). Specimen record from the collection in the Natural History Museum. London, UK: Natural History Museum.
Okaraonye, C. C., and Ikewuchi, J. C. (2009). Nutritional potential of Oryctes rhinoceros larva. Pak. J. Nutrn. 8, 35–38.
Olowu, R. A., Moronkola, B. A., Tovide, O. O., Denloye, A. A., Awokoya, K. N., Sunday, C. E., et al. (2012). Assessment of proximate and mineral status of Rhinoceros beetle larva, Oryctes rhinoceros Linnaeus (1758) (Coleoptera: Scarabaeidae) from Itokin, Lagos State, Nigeria. Res. J. Environ. Sci. 6, 118–124.
Onyeike, E. N., Ayalogu, E. O., and Okaraonye, C. C. (2005). Nutritive value of the larvae of raphia palm beetle (Oryctes rhinoceros) and weevil (Rhyncophorus pheonicis). J. Sci. Food Agric. 85, 1822–1828.
Parven, A., Khan, M. S. I., Prodhan, M. D. H., Venkateswarlu, K., Megharaj, M., and Meftaul, I. M. (2021). Human health risk assessment through quantitative screening of insecticide residues in two green beans to ensure food safety. J. Food Compos. Anal. 103:104121. doi: 10.1016/j.jfca.2021.104121
Philippe, R., and Dery, S. K. (2004). New way of controlling Oryctes monoceros (Coleoptera: Dynastidae), a coconut pest in Ghana. CORD 20, 43–51. doi: 10.37833/cord.v20i01.382
Phillips, S. J., Anderson, R. P., and Schapire, R. E. (2006). Maximum entropy modeling of species geographic distributions. Ecol. Model. 190, 231–259. doi: 10.1016/j.ecolmodel.2005.03.026
Pyšek, P., Hulme, P. E., Simberloff, D., Bacher, S., Blackburn, T. M., Carlton, J. T., et al. (2020). Scientists’ warning on invasive alien species. Biol. Rev. 95, 1511–1534. doi: 10.1111/brv.12627
Ramazi, P., Kunegel-Lion, M., Greiner, R., and Lewis, M. A. (2021). Predicting insect outbreaks using machine learning: A mountain pine beetle case study. Ecol. Evol. 11, 13014–13028. doi: 10.1002/ece3.7921
Remya, K., Ramachandran, A., and Jayakumar, S. (2015). Predicting the current and future suitable habitat distribution of Myristica dactyloides Gaertn. using MaxEnt model in the Eastern Ghats, India. Ecol. Eng. 82, 184–188. doi: 10.1016/j.ecoleng.2015.04.053
Sakai, A. K., Allendorf, F. W., Holt, J. S., Lodge, D. M., Molofsky, J., With, K. A., et al. (2001). The population biology of invasive species. Systematics. Annu. Rev. Ecol. Evol. Syst. 32, 305–332. doi: 10.1146/annurev.ecolsys.32.081501.114037
Seguni, Z., Lohr, B., and Mwaiko, W. (1999). Introducing Baculovirus oryctes Huger into Oryctes monoceros Oliv. populations in Tanzania. J. Appl. Entomol. 123, 427–431. doi: 10.1046/j.1439-0418.1999.00361.x
Senay, S. D., Worner, S. P., and Ikeda, T. (2013). Novel three-step pseudo-absence selection technique for improved species distribution modelling. PLoS One 8:e71218. doi: 10.1371/journal.pone.0071218
Séré, A., Bougma, A., Ouilly, J. T., Traoré, M., Sangaré, H., Lykke, A. M., et al. (2018). Traditional knowledge regarding edible insects in Burkina Faso. J. Ethnobiol. Ethnomed. 14, 1–11. doi: 10.1186/s13002-018-0258-z
Shea, K., and Chesson, P. (2002). Community ecology theory as a framework for biological invasions. Trends Ecol. Evol. 17, 170–176. doi: 10.1016/S0169-5347(02)02495-3
Shine, C. (2007). Invasive species in an international context: IPPC, CBD, European Strategy on Invasive Alien Species and other legal instruments. EPPO Bull. 37, 103–113. doi: 10.1111/j.1365-2338.2007.01087.x
Soytas, U., and Sari, R. (2006). Can China contribute more to the fight against global warming? J. Policy Model. 28, 837–846. doi: 10.1016/j.jpolmod.2006.06.016
Ukeh, D. A., Usua, E. J., and Umoetok, S. B. A. (2003). Notes on the biology of Oryctes monoceros (OLIV.) A pest of palms in Nigeria. Glob. J. Agric. Sci. 2, 33–36. doi: 10.4314/gjass.v2i1.2180
Valencia-Rodríguez, D., Jiménez-Segura, L., Rogéliz, C. A., and Parra, J. L. (2021). Ecological niche modeling as an effective tool to predict the distribution of freshwater organisms: The case of the Sabaleta Brycon henni (Eigenmann, 1913). PLoS One 16:e0247876. doi: 10.1371/journal.pone.0247876
Vanhanen, H., Veteli, T. O., Paivinen, S., Kellomaki, S., and Niemela, P. (2007). Climate change and range shifts in two insect defoliators: gypsy moth and nun moth-a model study. Silva Fenn. 41:621. doi: 10.14214/sf.469
Warren, D. L., and Seifert, S. N. (2011). Ecological niche modeling in Maxent: the importance of model complexity and the performance of model selection criteria. Ecol. Appl. 21, 335–342. doi: 10.1890/10-1171.1
Yu, H., Cooper, A. R., and Infante, D. M. (2020). Improving species distribution model predictive accuracy using species abundance: Application with boosted regression trees. Ecol. Model. 432:109202. doi: 10.1016/j.ecolmodel.2020.109202
Zheng, C., Jiang, D., Ding, F., Fu, J., and Hao, M. (2019). Spatiotemporal patterns and risk factors for scrub typhus from 2007 to 2017 in southern China. Clin. Infect. Dis. 69, 1205–1211. doi: 10.1093/cid/ciy1050
Keywords: African rhinoceros beetle, Boosted Regression Trees, BRT, climate change, Oryctes monoceros
Citation: Aidoo OF, Hao M, Ding F, Wang D, Jiang D, Ma T, Qian Y, Tettey E, Yankey N, Dadzie Ninsin K and Borgemeister C (2022) The Impact of Climate Change on Potential Invasion Risk of Oryctes monoceros Worldwide. Front. Ecol. Evol. 10:895906. doi: 10.3389/fevo.2022.895906
Received: 14 March 2022; Accepted: 15 June 2022;
Published: 06 July 2022.
Edited by:
Mark Maboeta, North-West University, South AfricaReviewed by:
Ji-Zhong Wan, Qinghai University, ChinaMatan Shelomi, National Taiwan University, Taiwan
Copyright © 2022 Aidoo, Hao, Ding, Wang, Jiang, Ma, Qian, Tettey, Yankey, Dadzie Ninsin and Borgemeister. This is an open-access article distributed under the terms of the Creative Commons Attribution License (CC BY). The use, distribution or reproduction in other forums is permitted, provided the original author(s) and the copyright owner(s) are credited and that the original publication in this journal is cited, in accordance with accepted academic practice. No use, distribution or reproduction is permitted which does not comply with these terms.
*Correspondence: Fangyu Ding, dingfy@igsnrr.ac.cn; Tian Ma, mat.19b@igsnrr.ac.cn
†These authors have contributed equally to this work