Tolerance for Wolves in the United States
- School of Environment and Natural Resources, The Ohio State University, Columbus, OH, United States
This study applies a psychological hazard-acceptance model to U.S. wolf conservation. Where most prior studies have focused on human populations most likely to interact with wolves (e.g., people who reside in wolves’ range), we sought to model tolerance among the general public throughout the United States, with representative samples from two regions with ongoing recovery efforts (i.e., the Northern Rocky Mountains and Western Great Lakes) as well as the rest of the country. As opposed to typical, attitudinal measures of tolerance (e.g., wildlife acceptance capacity) we sought to model supportive and oppositional behavior among the U.S. public as a function of perceptions of risk, benefit, and control, trust in the U.S. Fish and Wildlife Service, and affect toward wolves. At the national level, results predict a moderate amount of the variance for tolerant, stewardship behaviors (r2 = 0.22–0.25) and intolerant, oppositional behaviors to wolf conservation (r2 = 0.14–0.22). Most respondents (55%) did not intend to engage in either supportive or oppositional actions, and 23% indicated a preference for wolf populations to increase nationally. These preferences varied slightly by sample region when weighted to reflect regional demographics, with about one in three respondents in the Northern Rocky Mountains preferring for wolf populations to increase (32%), and slightly fewer saying the same in the Western Great Lakes region (30%) and rest of the United States (27%). We performed a post hoc logistic regression to identify factors that predisposed U.S. residents nationally to engage in any behavior toward wolves (tolerant or intolerant). This analysis suggested that the perceived importance of the wolf issue was most predictive of intentions to engage in behavior relevant to wolf conservation. Analyses indicate high levels of tolerance for wolves nationally, some support for their restoration, and only small minorities engaging in oppositional behavior. With the recent shift to individual state-level management, a more diverse policy matrix will increase the importance of understanding how human tolerance for wolves varies spatially (at the local level), and what factors drive tolerance at both the individual and group level.
Introduction
In recent decades, large carnivores have begun to reoccupy parts of Europe and North America where they were previously eradicated (e.g., Chapron et al., 2014). As these species move into human-dominated landscapes, coexistence requires some degree of human tolerance of their presence and associated risks (Bruskotter et al., 2014). Indeed, in some cases, human-causes represent the overwhelming source of mortality for carnivores (Treves et al., 2017). Thus, a variety of recent scholarship has sought to address the question: what makes people more or less tolerant of wildlife (e.g., Carter et al., 2012; Slagle et al., 2012; Zajac et al., 2012; Browne-Nuñez et al., 2015; Inskip et al., 2016; Kansky et al., 2016; Marino et al., 2021). As large carnivores represent a novel hazard to many human populations, Bruskotter and Wilson (2014) adapted theory explaining individual-level variation in the “acceptance” of hazards (e.g., Siegrist, 2000; Siegrist and Cvetkovich, 2000) to explain variation in acceptance (or tolerance—we use these terms interchangeably here) of carnivores. Here we test a hazard acceptance model of carnivore conservation with a national sample in the United States, using the gray wolf as our model species.
Conventional wisdom suggests that charismatic megafauna can act as “flagship species”—animals that become symbols or rallying points for conservation action (Ducarme et al., 2013). However, large terrestrial mammals are also prone to conflict with humans’ interests. Through normal expressions of behavior these animals represent potential threats to our crops, our pets and occasionally our lives (Nyhus, 2016). In the language of researchers who study risk, they are hazards. Studies on hazard acceptance indicate that acceptance is driven in large part by the perceived risks and benefits individuals associate with hazards (Siegrist, 2000; Visschers et al., 2011; Ascher et al., 2013), a finding that generally mirrors research on tolerance for wildlife (for reviews see: Kansky et al., 2016; Slagle and Bruskotter, 2019). Although describing acceptance in terms of risk and benefit implies a considered and rational cost/benefit approach to managing a carnivore species, in reality it is the human perceptions of these risks and benefits that matter (Slovic, 1987; Alhakami and Slovic, 1994; Siegrist, 2000), and these perceptions are not always objectively accurate (Slovic, 1999). While research on perceived risks and benefits related to carnivore conservation is recent, findings generally follow similar patterns as other environmental hazards in that higher risk perceptions correlate to lower acceptance (e.g., Riley and Decker, 2000; Zajac et al., 2012), and higher perceptions of benefits generally correlate to greater acceptance (e.g., Siemer et al., 2009; Carter et al., 2012). Where studies have employed more extensive models of tolerance for carnivores, perceptions of risk and benefit work in a converse relationship (as they do in other hazard contexts: Alhakami and Slovic, 1994), though perceived benefits may be as or more predictive of tolerance than risk perceptions across varied contexts of carnivore conservation (e.g., Carter et al., 2012; Slagle et al., 2012; Inskip et al., 2016).
Perceptions of risk and benefit are, in turn, affected by individuals’ general feelings surrounding a hazard, also known as affect (Johnson and Tversky, 1983; Peters, 2006). Affect serves to bias subsequent judgments concerning hazards such that negative affect can increase risk perceptions and lower benefit perceptions, while positive affect can have the opposite effect (Finucane et al., 2000), with evidence of this in the tolerance literature (e.g., Slagle et al., 2012; Vaske J. et al., 2021). Affect as a heuristic enables us to sift through information and come to a decision more efficiently than an endless tabulation of pros and cons might (Peters, 2006).
Also critical to understanding perceptions of risk is one’s perception of control over the hazard, where a perceived lack of control drives up risk perception (Slovic, 1987), and inhibits our intended behaviors (Armitage and Conner, 2001; Ajzen, 2002). The tolerance literature supports these findings, where lower personal control was associated with increased risk perceptions of black bears (Ursus americanus), and in turn, decreased acceptance of black bears in Ohio (Zajac et al., 2012). In Norway, residents that had lower perceptions of control found carnivore behaviors in general to be less acceptable (Kleiven et al., 2004), and sheep farmers with a higher sense of control had fewer negative attitudes toward carnivores (Bjerke et al., 2000). Focusing on tigers (Panthera tigris) in Nepal, local residents’ lower perceived ability to adapt to or avoid risks was associated with a preference for fewer tigers (Carter et al., 2012).
Finally, in modern societies, citizens shift responsibility and management for hazards to local, state and federal agencies charged with mitigating risk on behalf of the public (think nuclear power, prescription drugs, or forest fires). Because the actions of these agencies affect how hazards are managed, they can also impact the extent to which hazards are judged as risky or beneficial. Specifically, trust in the agency acts as a decision-making shortcut, similar to affect, in that it simplifies our choices. When agencies are trusted, individuals tend to view hazards as less risky and more beneficial; when they are not trusted individuals tend toward the opposite (e.g., Siegrist, 2000; Siegrist and Cvetkovich, 2000; Zajac et al., 2012). Trust in the management agency is partially composed of calculative trust or confidence: do we believe the agency is capable in their management? If so, we entrust them with responsibilities ranging from saving endangered carnivores to protecting us from recovered carnivore populations. Similarly, trust as salient values similarity, also known as relational trust, is the perception that the agency holds the same values as we do in this issue and will act in a similar manner as we would. When we perceive a similarity, we trust the agency to do the right thing, whatever we perceive that to be, because we expect those who think like us to act like us. Relational trust is based in knowing the intentions of the agency and is resilient because of its basis in shared values.
Pulling together the antecedents of acceptance—trust, control, affect, risk, benefit—into a sequential model provides additional insight to how judgments concerning the acceptability of carnivores are made, particularly the relationships between these judgments (Figure 1). In prior work, we designed and experimentally evaluated outreach aimed at increasing these antecedents as a means of increasing tolerance. We found evidence of the causal nature of this framework in that messages focused on heightening a sense of personal control and perceived benefits increased reported acceptance of black bears in Ohio (Slagle et al., 2013).
However, recently scholars have questioned the adequacy of relying on traditional, attitudinal measures of acceptance (e.g., attitudes or “Wildlife Acceptance Capacity”) to gauge tolerance of wildlife (e.g., Bruskotter and Fulton, 2012; Heberlein, 2012; Brenner and Metcalf, 2020). Psychological researchers have long understood that a general attitude toward a target (general classes of entities such as peoples of certain nationalities or animals of a particular species) tends to be weakly associated with any instance of behavior, though the strength of association improves when broad classes of behavior are examined (Fishbein and Ajzen, 1974; Weigel and Newman, 1976). Bruskotter and Fulton (2012) argued that beyond the problem of prediction, single-item measures do not capture the broad array of actions people can take either in support of or opposition to carnivores. They proposed a behavioral measure, where researchers assess respondents intentions to engage in a broad range of supportive and oppositional actions. Slagle et al. (2012) demonstrated that, similar to attitudinal measures of acceptance, risk and benefit judgments can be useful for explaining intentions to engage in supportive and oppositional behaviors directed at carnivores. However, their study is limited in that it (i) did not contain all of the components of the hazard model discussed by Bruskotter and Wilson (2014), and (ii) relied on a sample of highly involved individuals (i.e., an “issue public”). Thus, in this study we seek to test the hazard-acceptance model of tolerance among a sample of the general public in the United States, using a behavioral measure of tolerance. We use gray wolves (Canis lupus) as a model species in this analysis. Gray wolves are an ideal model because they (i) are among the widest-ranging large, terrestrial carnivore (Wolf and Ripple, 2017); (ii) are capable of living near human settlement (Linnell et al., 2001; Mech and Boitani, 2003); and (iii) occasionally attack humans, our pets and our livestock (Linnell et al., 2001, 2021). Moreover, wolves have recently expanded into parts of their former ranges in Europe and the United States where they have not been for decades, prompting questions about how to coexist with this species (Bruskotter et al., 2014; Chapron et al., 2014).
Materials and Methods
Sampling and Data Collection
We conducted an online survey of a representative sample from a panel maintained by GfK (now maintained by Ipsos), a marketing research firm. In order to approximate traditional mail samples, GfK recruits members via multiple contacts at their mailing address. To overcome coverage issues plaguing online samples due to a lack of internet access, GfK provides internet access to recruits that do not have access in exchange for their participation in the online panel. Recruits that already have internet access are compensated with points, which translate to roughly $4–$6 per month, a nominal remuneration unlikely to bias responses. GfK then draws a sample of respondents from their recruited panel members for study. Our samples were roughly evenly recruited from three regions of the United States: (1) The Northern Rocky Mountains, (2) the Western Great Lakes, and (3) the rest of the United States. To ensure sample representativeness at the national level, post hoc sampling weights were constructed using United States. Census Bureau data, accounting for respondent age, race and/or ethnicity, level of education, household income, census region, metropolitan area residence, and whether the respondent had household access to the Internet. Research on this method of sampling suggests that it is almost identical to telephone surveys (Berrens et al., 2003), but lacks other biases associated with telephone surveys (coverage bias due to cellphone use and social desirability bias; Chang and Krosnick, 2009; for more detail on panel construction, see Berrens et al., 2003; The GfK Group, 2013.
The Ohio State University’s Office of Responsible Research Practices reviewed and approved the methods used in this research (protocol number 2013E0553). Prior to the full survey period, we pre-tested the survey instrument for function and length, and found it necessary to reduce survey burden. To achieve this, we limited respondents’ assessment of trust in the USFWS to those respondents at least somewhat familiar with the agency. Respondents not at all familiar with the agency skipped to the next bank of questions, and thus were removed for SEM analyses due to data not missing at random. Additionally, responses to the 10-item bank of benefits and risks to wolf recovery were randomly assigned, such that each respondent received a random set of 8 of the 10 questions. These changes resulted in a final average survey length of 12.5 min. We gathered all responses using Qualtrics, an online survey platform, and the full survey period occurred over an 11-day period in February 2014. Respondents were contacted up to 3 times, twice by email and a final automated telephone call (for further details on this study, please see (Slagle et al., 2017).
There are several variables of interest in this study: affect, benefits, risks, control, trust, and tolerance (specific measures can be found in Table 1). Measurement of affect followed the method proposed by Peters and Slovic (1996, 2007), where respondents are asked to write down the first thought or image that comes to mind when considering wolves. They are then asked to rate on a bipolar scale how positive or negative they feel about what they wrote. To increase the measure’s usefulness and follow existing literature, the measure was asked a second time, immediately following the question rating the first thought or image (Peters and Slovic, 2007).
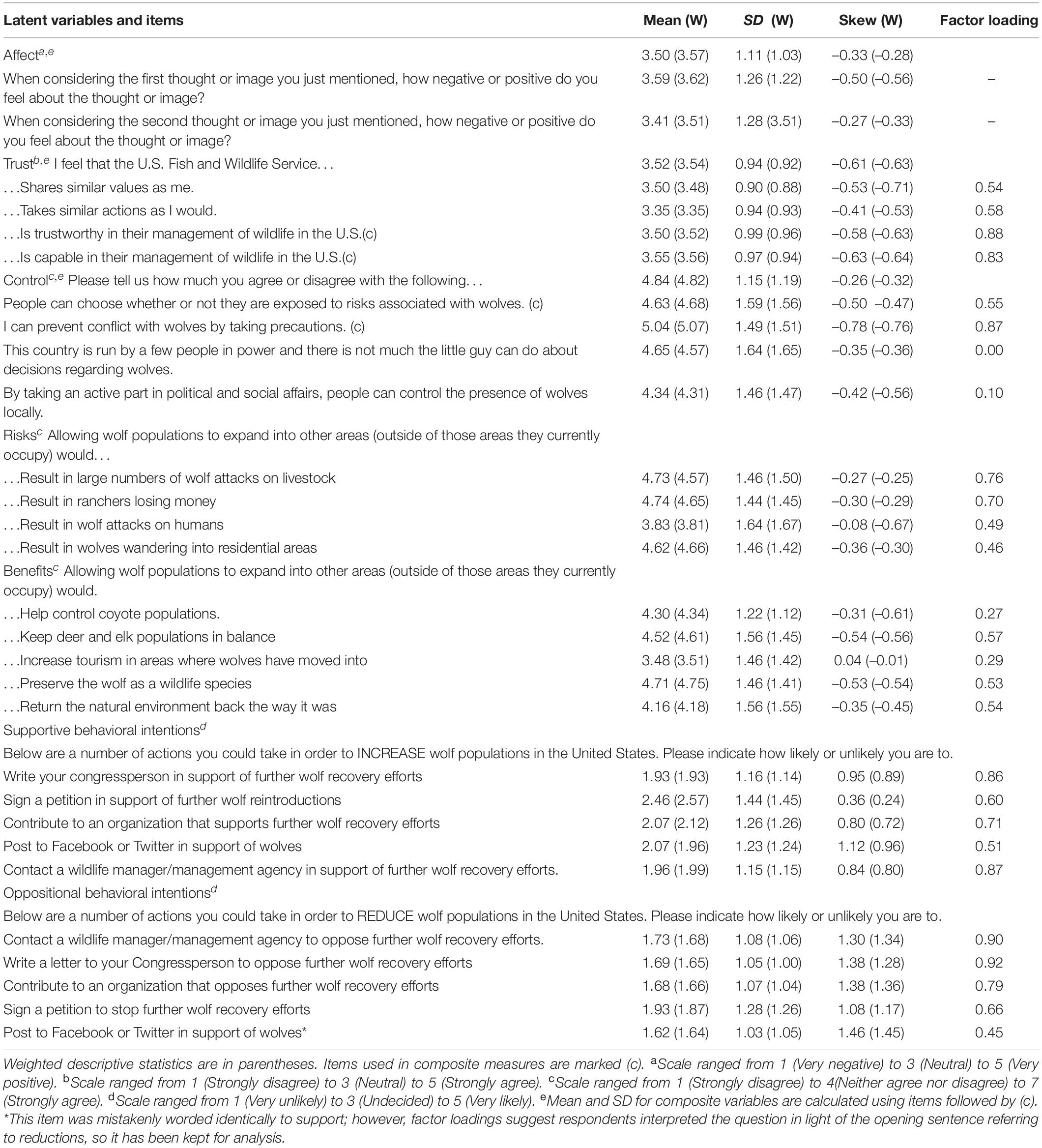
Table 1. Factor loadings, unweighted and weighted descriptive statistics of items used to measure latent model variables.
Benefits and risks were measured by agreement with statements regarding various outcomes of a recovered wolf population (Bright and Manfredo, 1996; Slagle et al., 2012). Five statements described negative outcomes like depredation, effects on game species and rancher losses. Five statements described positive outcomes like a return of “naturalness,” benefits of tourism, and “balanced” wildlife populations.
Control was measured through agreement with a series of four statements that assess both control over the risk from wolves themselves (adapted from Zajac et al., 2012), as well as control over the policy process (adapted from Johansson and Karlsson, 2011).
Agency trust was assessed through measures aimed at both relational trust and calculative trust in the U.S. Fish and Wildlife Service (Earle, 2010). Salient values statements tapping relational trust focused on the extent to which an individual feels the agency in question shares their values and would take similar action (Earle and Cvetkovich, 1995; Cvetkovich and Winter, 2003). Confidence statements or calculative trust, was measured in statements regarding the agency’s ability to manage wildlife.
Finally, tolerance for wolves was measured as the intention to perform a variety of politically relevant behaviors. Tolerance can be thought of as a continuous scale ranging from stewardship of wildlife to passive tolerance to active intolerant behaviors (Bruskotter et al., 2015). Here, tolerance is measured with a set of behavioral intentions to oppose and support wolf recovery, which are replicated from Slagle et al. (2012). These include writing one’s Congressperson, signing a petition, and contributing to a non-profit organization. We added 2 more behaviors that might be more accessible or typical of the general public: contacting a wildlife manager and making a post to social media related to wolf conservation.
Model Measurement and Analyses
Testing the hazard acceptance model required measuring 6 latent variables via 30 survey items: trust, control, affect, risk, benefit, and tolerance (divided into support and opposition for purposes of analysis; Table 1). Latent psychological variables are impossible to measure directly, and as such, are observed through their influence on other human behaviors (here, responding to items on a survey questionnaire). Structural equation modeling allows for these latent—observed variable relationships to be maintained by building confirmatory factor analyses into the measurement models, while also testing for the theoretical relationships between the latent variables themselves (Schumacker and Lomax, 2004). Model fit was assessed using multiple indices, specifically the Comparative Fit Index (CFI), Root Mean Squared Error of Approximation (RMSEA), and the Standardized Root-Mean Squared Residual (SRMR). Following Diamantopoulos and Siguaw (2000) and Schumacker and Lomax (2004), RMSEA and SRMR values between 0.05 and 0.08 were seen as having “reasonable fit,” while anything less than 0.05 was a “good fit.” We sought CFI values of 0.95 or greater, but levels of 0.90 or greater were considered as having “reasonable fit” (Hu and Bentler, 1999).
Structural equation modeling requires data missingness to be explicitly handled prior to analysis, and this study explicitly contained data missing completely at random due to the need to reduce response burden (see “Sampling and Data Collection” section for detail). In order to apply weights approximating a national sample, we used linear interpolation method using ordinary least squares regression in IBM SPSS (IBM Corp, 2013) to recover planned missingness in benefit and risk (10 items total, between 20 and 24% missing completely at random), as well as random missingness in the remaining model variables (18 items total, between 2 and 6% missing at random). This method uses regression to model missing data for each respondent, imputing the value suggested by the regression line (Allison, 2003). After imputing the data in SPSS, it was transferred to R for all structural equation model analyses. Measurement confirmatory factor analyses and structural equation model analyses were performed in R using the lavaan and lavaan.survey packages (Rosseel, 2012; Oberski, 2014; R Development Core Team, 2016). We used weights generated by GfK to adjust our sample to approximate the demographics of the U.S. national population and investigate the generalizability of the hazard-acceptance model to a broader context. Descriptive analyses and post hoc regressions were performed in IBM SPSS (IBM Corp, 2013).
Results
GfK contacted a total of 2,020 potential respondents and received 1,287 completed surveys for a response rate of 63.7%. Prior to weighting procedures, respondents were more female than male (46.0% male), majority white (83.5%), from metropolitan statistical areas (73.0%) and had internet access separate from GfK (84.5%). The average age was 50.8 years, and 50.9% of respondents reported a household income of less than $59,000 per year. Weights were created using demographics from the 2009 to 2011 American Community Survey conducted by the United States Census Bureau, and the minimal impacts of adding sociodemographic weights to descriptive analyses for observed variables can be seen in Table 1, where fairly small or non-existent changes to means, standard deviations, and skewness are evident. After national weighting, 30% of respondents preferred for wolves to increase nationwide, and when weighted to reflect regional demographics, about one in three respondents in the Northern Rocky Mountains preferred for wolf populations to increase nationwide (32%), with slightly fewer saying the same in the Western Great Lakes region (30%) and rest of the United States (27%).
Bearing the thresholds for factor loadings and model fit indices in mind, we aimed to ensure good measurement models while not discarding observed variables out of hand. We assessed affect with two items, which required an averaged composite measure rather than a latent variable model. Trust was measured via 4 items, and responses to these items was limited to those individuals who reported being at least somewhat familiar with the U.S. Fish and Wildlife Service. Still, a measurement model resulted in poor fit (CFI = 0.87, RMSEA = 0.46, SRMR = 0.07), but indicated high factor loadings (> 0.80) for the two items focused on trustworthiness and capability in management. These items were averaged for a composite measure of trust, or more specifically, calculative trust or confidence. Control was also measured through 4 items, resulting in marginally acceptable fit of the overall measurement model (CFI = 0.94, RMSEA = 0.15, SRMR = 0.05), but poor factor loadings for 2 items of < 0.10. Again, we created a composite measure for control by averaging the 2 items with factor loadings of > 0.50, measuring personal control over exposure to the risk and prevention of conflict with wolves. The remaining 4 latent variable measurement models (benefit, risk, support and opposition) all had acceptable fits for at least 2 of the 3 fit indices used here (CFI > 0.95, SRMR < 0.05), and factor loadings greater than 0.16 for each observed variable, and thus were maintained in full (Table 1).
The unweighted model achieved a reasonable fit for both support and opposition (support: CFI = 0.92, RMSEA = 0.06, SRMR = 0.06; opposition: CFI = 0.93, RMSEA = 0.06, SRMR = 0.06; Figure 2A), explained 25 and 22% of the variance, respectively, and all relationships were in the expected directions. Affect did not significantly predict opposition, but trust had a small relationship with risk (β = –0.13).
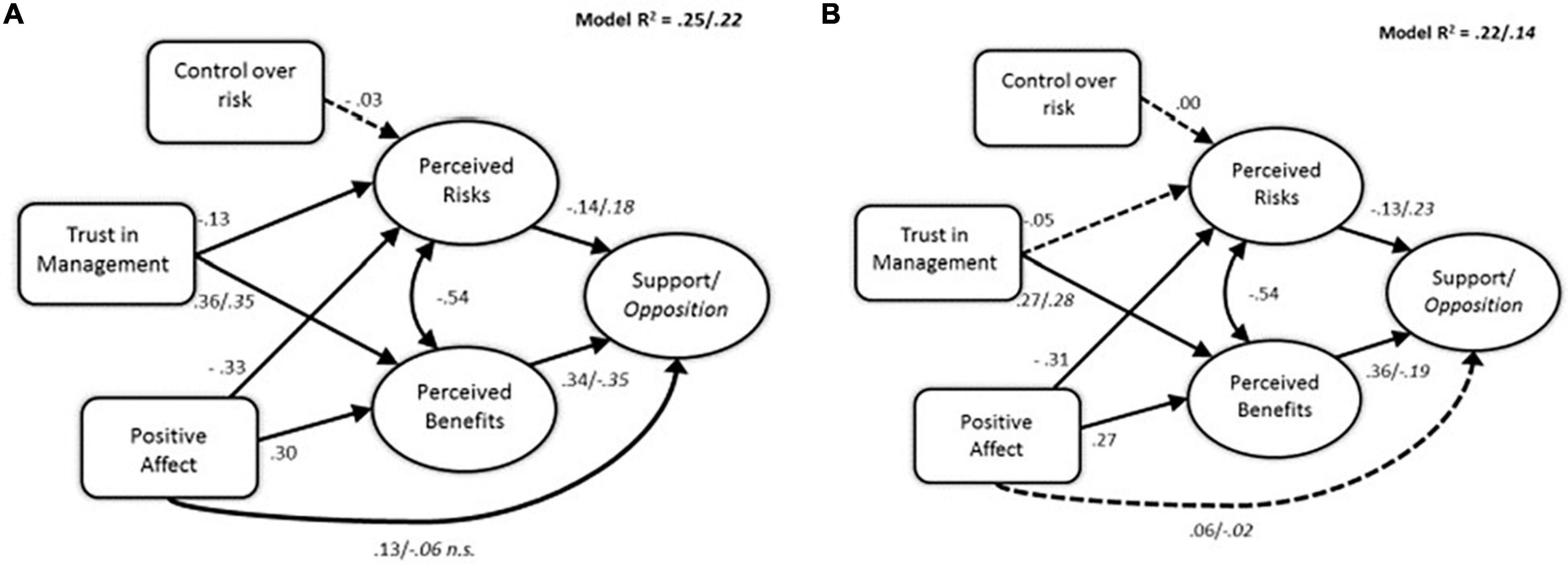
Figure 2. Structural equation model coefficients estimated using unweighted data (A) and weighted data (B) imputed with linear interpolation for both support and opposition. Where different, the opposition model coefficients are in italics. Bolded lines represent pathways significant at the p < 0.01 level for both models, dotted lines represent non-significant paths in both models. Discrepancies in path significance between models is noted with n.s. following the appropriate path coefficient. Observed (directly measured) variables are in rectangles, while latent variables are in ovals.
Finally, we tested the model on weighted, imputed data to understand the generalizability of such a model among a national public. Model fit dropped to a marginal fit for support and a poor fit for opposition (support: CFA = 0.89, RMSEA = 0.08, SRMR = 0.06; opposition: CFI = 0.86, RMSEA = 0.09, SRMR = 0.07; Figure 2B), and explained 22 and 14% of the variance, respectively. After weighting, the trust relationship with risk was reduced and no longer significant and affect no longer had a significant direct influence on support. While the model fit is poor for opposition, an interesting shift in the relationship between risk, benefit, and opposition occurs in this model: Risks and benefits are almost equally predictive of opposition (risk β = 0.23; benefit β = –0.19), where in the previous unweighted model the influence of benefits on support and opposition dwarfed the influence of risk (Figure 2A). It should be noted that the unweighted model would be more heavily influenced by the respondents from the Northern Rocky Mountains and the Western Great Lakes regions—i.e., places where wolves are present and, presumably, a subject of more regular discussion and debate.
Given the overall poor fit of the weighted model, we conducted post hoc analyses to determine which variables might predict any likely action regarding wolves nationwide. We combined supportive and oppositional intended behaviors into one measure, where all unlikely responses were coded as 0, and all neither or likely responses were coded as 1. After combining behaviors, we then collapsed them into a dichotomous variable where any respondents who were unlikely to take any actions were coded as 0, and all others were coded as 1. We then used composite mean measures of trust, control, risk, benefit, and affect, as well as region (Northern Rocky Mountains, Western Great Lakes, and the rest of the United States) and dichotomized perceived importance of the wolf issue (0 = not at all or slightly important, 1 = moderately or very important) to predict any likely intended behavior in a logistic regression using pairwise deletion to handle all missing data (Table 2). Analyses weighted for a national sample suggest that when perceptions of both risk and benefit are high, any action is more likely, and when importance is high, any action is more likely. All other variables in the model were not significant.
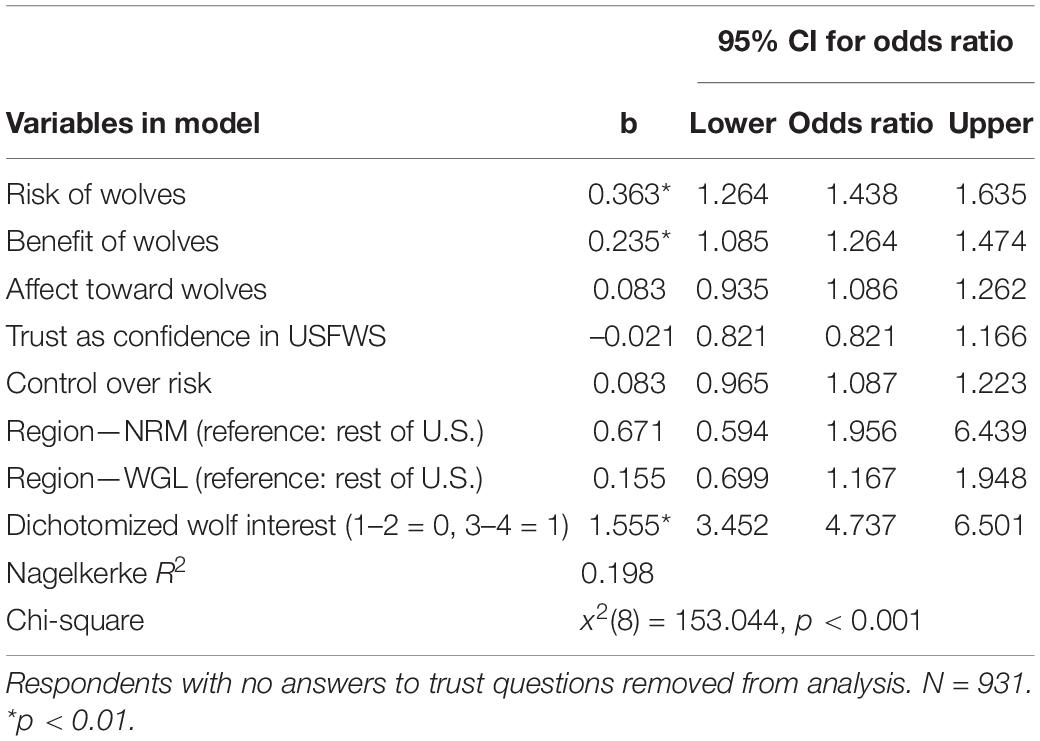
Table 2. Weighted logistic regression results predicting any likely or uncertain action reported by respondents (respondents unlikely to take any action coded as 0).
Discussion
A variety of studies have demonstrated that perceived risks and benefits are important for understanding acceptance of wildlife. However, studies of acceptance are often conducted among particular populations, i.e., those most likely to be impacted by a particular species. Moreover, it is unclear the extent to which the factors associated with attitudinal measures of “acceptance” are likewise associated with the broad classes of behavior (measured as intentions) that may impact the management of wildlife. Having demonstrated the usefulness of affect and perceived risk and benefit for explaining behavior in a sample of highly interested and involved individuals (see Slagle et al., 2012), we sought to determine the applicability of the full hazard-acceptance model among the general population of residents of the United States.
The structural model showed a reasonable fit to the data, predicting 22–25% of the variance in intentions to oppose or support wolf conservation, but became marginal to poor once weights adjusting for demographics within our sample were applied. While our model did not do nearly as well at explaining variance in behavior as it did among the interested and involved respondents, the explained variance is similar to other behavioral models. For example, a recent study found the Theory of Planned Behavior (TPB) and Value-Belief-Norm Theory explained 25 and 16% of variance, respectively, in willingness to pay for conservation of a specific park (López-Mosquera and Sánchez, 2012). Likewise, a recent meta-analysis of the TPB’s application to another risk-related behavior (food safety), found model components explained 22% of the variance in intention (Lin and Roberts, 2020). Moreover, TPB studies typically take advantage of the “principle of compatibility” to align measures to refer to a specific target behavior, conducted at a specific time in a particular context (Ajzen, 2005). Unfortunately, while this alignment makes them ideal for understanding instances of a behavior, it also means they are not well-suited to understand broad classes of behavior. Our model performs similarly well to these models and is designed to be used with a broad class of behaviors of interest to conservationists.
At least a couple of factors potentially explain why our model performed less well among the general public (this manuscript) than in an interested public (Slagle et al., 2012). First, it is likely that geography dictates the relevance of a subject. In this case, geography determines who interacts with wolves under what conditions. We know that people generally acquire the various beliefs and attitudes they possess through repeated rehearsals, i.e., interactions with the target of the attitude or with other people over that target (see generally, Eagly and Chaiken, 1993). At the time this survey was administered, fewer than 10 states had gray wolf populations. Moreover, wolves tend to be in the least populated regions of states—meaning very few people are “exposed” to wolves as a hazard. As experience with wolves increase, we expect beliefs about them and the risks and benefits they pose to become practiced and thus, more stable and accessible in memory (Petty and Krosnick, 2014). Second, our study used a panel of paid respondents, which led to much higher response rates (> 60%) than are normally witnessed in traditional mail and phone surveys. While this could be an indication that our sample is more representative than these other surveys, non-response can act as a type of “filter” whereby un/less interested individuals are less likely to respond (Thomson, 1991). If interested individuals have more stable and accessible thoughts about wolves, we can expect greater associations between their risk/benefit perceptions and behavior (because lack of reliability attenuates statistical relationships; Block, 1963).
Although, all relationships were in the expected hypothesized directions, in every model, the impacts of trust and control on risk were minimal, and the direct effects of affect on behavior were minimal to non-significant. Trust was a moderate predictor of benefits in every structural model, and for every model save the weighted opposition model, benefits maintained a moderate relationship with both supportive and oppositional behaviors. Structural model fit for opposition was consistently poorer than that for support, and possibly related to the mildly non-normal nature of those observed variables. In essence, very few people indicated they were likely or very likely to perform oppositional behaviors (less than or equal to 14% for any given action). Indeed, 33% of our sample were unlikely or very unlikely to perform any of the behaviors we included in our study, although this means 67% were at least uncertain of whether they would act and at most very likely to act to influence wolf policy. This finding is consistent with other work on the general public’s engagement in political behaviors meant to address environmental concerns (see Ballew et al., 2019 where the strong majority never contact policy makers about climate change).
Overall, this research suggests when placed in context with other models of human behavior, the hazard acceptance model provides similar insight into the way the general public approaches wolf management, and perhaps more broadly, carnivore management. Namely, that calculative or non-relational trust in the managing agency can increase the perceived benefits of the carnivores (for those familiar with the agency), and in turn, increase politically supportive behaviors.
One-third of our respondents were unlikely to perform any of the behaviors we assessed, and could be deemed “tolerant,” in the sense that they are not choosing to impact wolves in any fashion (Bruskotter and Fulton, 2012; Bruskotter et al., 2015). Understanding inaction may be just as important for future research as understanding active stewardship or intolerance, and given the limitations of our final weighted models, we chose to explore this idea in a post hoc logistic regression. Results suggest that interest in the wolf issue is a primary driver of action. Interest was associated with both increased risk and benefit perceptions, as well as increased likelihood of engaging in any action.
Comprehensive measures of trust in the area of risk and decision making rely on different measures (Earle, 2010), however due to survey length, we chose to both limit the number of items used to measure it and allow respondents unfamiliar with the agency to skip the question of trust altogether. Excluding unfamiliar respondents from analysis resulted in a significant relationship between trust and benefit, suggesting for those familiar with an agency, greater trust could increase perceptions of benefits. This relationship was no longer significant once sampling weights were applied, calling into question the public’s ability to make trust judgments due to a lack of familiarity and limiting generalizability of the finding. However, the relationship between trust and benefit remained throughout all iterations of the hazard acceptance model, emphasizing not only previous recommendations for agencies to include benefits as part of their outreach efforts to increase tolerance (Slagle et al., 2013; Bruskotter and Wilson, 2014), but also the importance of building trust with the public. The inverse relationship between risk and benefit indicates that an increase in perceived benefits could subsequently serve to lower risk perceptions and increase tolerance for carnivores both directly and indirectly. To increase trust, agencies, or other entities, might choose to emphasize their effectiveness in wolf management and any other successes in wildlife conservation. Here, greater trust related to greater perceptions of benefit, suggesting higher trust in the agency’s ability to manage successfully may promote a greater belief in the benefits of wolf conservation.
While recent work suggests a critical role for emotions or affect in understanding wildlife interactions (Jacobs and Vaske, 2019; Vaske J. J. et al., 2021), similar assessments of models for tolerance either omit affect entirely (Lischka et al., 2020), or only assess it as worry or concern (Carter et al., 2012; Inskip et al., 2016). In a reduced model tested on a sample highly interested in and knowledgeable about wolves, a generalized affect measure had a direct effect on both supportive and oppositional behaviors (Slagle et al., 2012), suggesting a role for motivated reasoning among this group of political sophisticates (Taber and Lodge, 2006, 2016). With motivated reasoning, affect acts as a contagion in our minds, spreading throughout cognitive schema and influencing the way in which we consider new information. This influence comes mostly in the form of discounting information that disconfirms our existing biases and placing greater value on information that confirms our initial affective evaluation. Here, however, among a national sample, the expected relationships between affect, risk, and benefit were maintained in all the models, but the direct relationship between affect and conservation related behavior was either weak or unsupported. When affect was predictive of conservation behavior, it was positively predictive of supportive behavior in the present sample. Key to the differences between a national public and an engaged and informed issue public, the contagion effect of affect resulting in motivated reasoning is indeed more pronounced among political sophisticates like those represented by the issue public—those one might intuitively presume to be most immune to it but in fact are often the most subject to such effects (Kahan et al., 2012). Less engaged individuals on the wolf issue, and likely other carnivore conservation issues, may lack the existing strong affective reactions or cognitive schema that drive the potentially motivated reasoning of an issue public, and could give more careful consideration and cognitive effort to weighing risk and benefit, though this is admittedly rare (Taber and Lodge, 2016).
From a more practical perspective, no more than 13% of our respondents were willing to contact a wildlife manager or agency in support or opposition to wolf recovery. Informal conversations with wildlife managers and agency personnel suggest that they fret over being contacted by members of the public, and sometimes take such calls as reflective of the general population. Managers and the agencies they serve in would do well to maintain perspective regarding contacts from the general public—it was a very small group in our sample that would consider this a viable action for impacting a charismatic and heavily contested carnivore species. One might conclude that contact from constituents regarding lower profile species or issues probably represent an even smaller portion of the general public. While these contacts from the public could be interpreted as a sign of bigger issues, such interpretations should be investigated further before warranting major shifts in policy or protocol.
The hazard acceptance model demonstrated acceptable fit with unweighted data from a nationally representative sample, indicating that it is useful for explaining some of the individual choice to impact wolf conservation. Our unweighted sample would have more heavily reflected regional populations (i.e., Northern Rocky Mountains and Western Great Lakes), and likely provided a better fit due to the contextual relevance for these respondents. At the national scale, many of the respondents in our sample were unlikely to undertake any of the behaviors we investigated, suggesting either a passive tolerance of wolves on their part or that our list of behaviors needs to be more inclusive. Where Kansky and Knight (2014) found the most predictive strength in intangible and tangible risks when considering attitudes toward large mammals among nearby communities, we found tangible and intangible benefits to be better predictors of intentions to behave among a broader, spatially distant sample. We also note the important differences between measuring attitudes toward conservation and conservation-related behaviors—predicting human behavior is more difficult, but better links to existing social science theory can better guide policy makers, and allow for stronger linkages to conservation outcomes via impacts on policy. We echo previous calls for additional exploration of the importance of explaining benefits of carnivore conservation, and issue caution to managers heavily weighting the contacts they receive from the public, as this likely represents a very small portion of their constituents.
Conclusion
Public tolerance for wolves in a general public sample does not appear to be driven by positive or negative affect toward wolves, but rather by perceptions of the benefits of expanded wolf populations, which were moderately predictive of both oppositional, intolerant behaviors and supportive, tolerant behaviors. These benefits may also be driven by confidence or calculative trust in the agency’s ability or those familiar with the managing agency. As might be expected by the compatibility principle, the hazard acceptance model tested at a large geographical scale here did not perform as well as similar models of tolerance at the state and local level, although it provides useful insights into the possibility of building acceptance through trust and perceived benefits even among a less engaged audience. We would expect that researchers and conservationists at the local level might find the model even more predictive and informative for public engagement around issues that are more proximal and relevant to a local population.
Data Availability Statement
The raw data supporting the conclusions of this article will be made available by the authors, without undue reservation.
Ethics Statement
The studies involving human participants were reviewed and approved by the Institutional Review Board at The Ohio State University. Written informed consent for participation was not required for this study in accordance with the national legislation and the institutional requirements.
Author Contributions
KS, RW, and JB equally contributed to conception and design of the study. KS performed the statistical analysis and wrote the first draft of the manuscript. All authors contributed to manuscript revision, read, and approved the submitted version.
Funding
Funding for data collection was provided by startup funds from the School of Environment and Natural Resources at The Ohio State University.
Conflict of Interest
The authors declare that the research was conducted in the absence of any commercial or financial relationships that could be construed as a potential conflict of interest.
Publisher’s Note
All claims expressed in this article are solely those of the authors and do not necessarily represent those of their affiliated organizations, or those of the publisher, the editors and the reviewers. Any product that may be evaluated in this article, or claim that may be made by its manufacturer, is not guaranteed or endorsed by the publisher.
Acknowledgments
This work was completed as part of KS’s doctoral dissertation, and we wish to acknowledge her committee member, Eric Toman for his service. We also thank Ryan Zajac for his support of the initial structural equation modeling that inspired this manuscript, as well as the many respondents who took the time to complete this survey.
References
Ajzen, I. (2002). Perceived behavioral control, self efficacy, locus of control, and the theory of planned behavior. J. Appl. Soc. Psychol. 32, 665–683. doi: 10.1111/j.1559-1816.2002.tb00236.x
Alhakami, A. S., and Slovic, P. (1994). A psychological study of the inverse relationship between perceived risk and perceived benefit. Risk Anal. 14, 1085–1096. doi: 10.1111/j.1539-6924.1994.tb00080.x
Allison, P. D. (2003). Missing data techniques for structural equation modeling. J. Abnorm. Psychol. 112, 545–557. doi: 10.1037/0021-843X.112.4.545
Armitage, C. J., and Conner, M. (2001). Efficacy of the theory of planned behaviour: a meta-analytic review. Br. J. Soc. Psychol. 40, 471–499. doi: 10.1348/014466601164939
Ascher, T. J., Wilson, R. S., and Toman, E. (2013). The importance of affect, perceived risk and perceived benefit in understanding support for fuels management among wildland–urban interface residents. Int. J. Wildland Fire 22:267. doi: 10.1071/WF12026
Ballew, M. T., Goldberg, M. H., Rosenthal, S. A., Cutler, M. J., and Leiserowitz, A. (2019). Climate change activism among Latino and white Americans. Front. Commun. 3:58. doi: 10.3389/fcomm.2018.00058
Berrens, R. P., Bohara, A. K., Jenkins-Smith, H., Silva, C., and Weimer, D. L. (2003). The advent of internet surveys for political research: a comparison of telephone and internet samples. Polit. Anal. 11, 1–22. doi: 10.1093/pan/11.1.1
Bjerke, T., Vittersø, J., and Kaltenborn, B. P. (2000). Locus of control and attitudes toward large carnivores. Psychol. Rep. 86, 37–46. doi: 10.2466/pr0.2000.86.1.37
Block, J. (1963). The equivalence of measures and the correction for attenuation. Psychol. Bull. 60, 152–156. doi: 10.1037/h0041811
Brenner, L. J., and Metcalf, E. C. (2020). Beyond the tolerance/intolerance dichotomy: incorporating attitudes and acceptability into a robust definition of social tolerance of wildlife. Hum. Dimens. Wildl. 25, 259–267. doi: 10.1080/10871209.2019.1702741
Bright, A. D., and Manfredo, M. J. (1996). A conceptual model of attitudes toward natural resource issues: a case study of wolf reintroduction. Hum. Dimens. Wildl. 1, 1–21. doi: 10.1080/10871209609359048
Browne-Nuñez, C., Treves, A., MacFarland, D., Voyles, Z., and Turng, C. (2015). Tolerance of wolves in Wisconsin: a mixed-methods examination of policy effects on attitudes and behavioral inclinations. Biol. Conserv. 189, 59–71. doi: 10.1016/j.biocon.2014.12.016
Bruskotter, J. T., and Fulton, D. C. (2012). Will hunters steward wolves? A comment on Treves and Martin. Soc. Nat. Resour. 25, 97–102. doi: 10.1080/08941920.2011.622735
Bruskotter, J. T., Singh, A., Fulton, D. C., and Slagle, K. (2015). Assessing tolerance for wildlife: clarifying relations between concepts and measures. Hum. Dimens. Wildl. 20, 255–270. doi: 10.1080/10871209.2015.1016387
Bruskotter, J. T., Vucetich, J. A., Enzler, S., Treves, A., and Nelson, M. P. (2014). Removing protections for wolves and the future of the U.S. Endangered Species Act (1973): removing protections for wolves. Conserv. Lett. 7, 401–407. doi: 10.1111/conl.12081
Bruskotter, J. T., and Wilson, R. S. (2014). Determining where the wild things will be: using psychological theory to find tolerance for large carnivores. Conserv. Lett. 7, 158–165. doi: 10.1111/conl.12072
Carter, N. H., Riley, S. J., and Liu, J. (2012). Utility of a psychological framework for carnivore conservation. Oryx 46, 525–535. doi: 10.1017/S0030605312000245
Chang, L., and Krosnick, J. A. (2009). National surveys via RDD telephone interviewing versus the internet: comparing sample representativeness and response quality. Public Opin. Q. 73, 641–678. doi: 10.1093/poq/nfp075
Chapron, G., Kaczensky, P., Linnell, J. D., von Arx, M., Huber, D., Andrén, H., et al. (2014). Recovery of large carnivores in Europe’s modern human-dominated landscapes. Science 346, 1517–1519. doi: 10.1126/science.1257553
Cvetkovich, G., and Winter, P. L. (2003). Trust and social representations of the management of threatened and endangered species. Environ. Behav. 35, 286–307. doi: 10.1177/0013916502250139
Diamantopoulos, A., and Siguaw, J. A. (2000). Introducing LISREL: A Guide for the Uninitiated. London: SAGE.
Ducarme, F., Luque, G. M., and Courchamp, F. (2013). What are “charismatic species” for conservation biologists. Biosci. Master Rev. 10, 1–8.
Eagly, A. H., and Chaiken, S. (1993). The Psychology of Attitudes. Fort Worth, TX: Harcourt Brace Jovanovich College Publishers.
Earle, T. C. (2010). Trust in risk management: a model-based review of empirical research. Risk Anal. 30, 541–574. doi: 10.1111/j.1539-6924.2010.01398.x
Earle, T. C., and Cvetkovich, G. (1995). Social Trust: Toward a Cosmopolitan Society. Westport, WA: Praeger Publishers.
Finucane, M. L., Alhakami, A., Slovic, P., and Johnson, S. M. (2000). The affect heuristic in judgments of risks and benefits. J. Behav. Decis. Mak. 13, 1–17. doi: 10.1002/(sici)1099-0771(200001/03)13:1<1::aid-bdm333>3.0.co;2-s
Fishbein, M., and Ajzen, I. (1974). Attitudes towards objects as predictors of single and multiple behavioral criteria. Psychol. Rev. 81, 59–74. doi: 10.1037/h0035872
Hu, L., and Bentler, P. M. (1999). Cutoff criteria for fit indexes in covariance structure analysis: conventional criteria versus new alternatives. Struct. Equ. Model. 6, 1–55. doi: 10.1080/10705519909540118
Inskip, C., Carter, N., Riley, S., Roberts, T., and MacMillan, D. (2016). Toward human-carnivore coexistence: understanding tolerance for tigers in Bangladesh. PLoS One 11:e0145913. doi: 10.1371/journal.pone.0145913
Jacobs, M., and Vaske, J. J. (2019). “Understanding emotions as opportunities for and barriers to coexistence with wildlife,” in Human–Wildlife Interactions, eds B. Frank, J. A. Glikman, and S. Marchini (Cambridge: Cambridge University Press), 65–84. doi: 10.1017/9781108235730.007
Johansson, M., and Karlsson, J. (2011). Subjective experience of fear and the cognitive interpretation of large carnivores. Hum. Dimens. Wildl. 16, 15–29. doi: 10.1080/10871209.2011.535240
Johnson, E. J., and Tversky, A. (1983). Affect, generalization, and the perception of risk. J. Pers. Soc. Psychol. 45, 20–31. doi: 10.1037/0022-3514.45.1.20
Kahan, D. M., Peters, E., Wittlin, M., Slovic, P., Ouellette, L. L., Braman, D., et al. (2012). The polarizing impact of science literacy and numeracy on perceived climate change risks. Nat. Clim. Change 2, 732–735. doi: 10.1038/nclimate1547
Kansky, R., Kidd, M., and Knight, A. T. (2016). A wildlife tolerance model and case study for understanding human wildlife conflicts. Biol. Conserv. 201, 137–145. doi: 10.1016/j.biocon.2016.07.002
Kansky, R., and Knight, A. T. (2014). Key factors driving attitudes towards large mammals in conflict with humans. Biol. Conserv. 179, 93–105. doi: 10.1016/j.biocon.2014.09.008
Kleiven, J., Bjerke, T., and Kaltenborn, B. P. (2004). Factors influencing the social acceptability of large carnivore behaviors. Biodivers. Conserv. 13, 1647–1658. doi: 10.1177/1471301220932780
Lin, N., and Roberts, K. R. (2020). Using the theory of planned behavior to predict food safety behavioral intention: a systematic review and meta-analysis. Int. J. Hosp. Manag. 90:102612. doi: 10.1016/j.ijhm.2020.102612
Linnell, J. D., Kovtun, E., and Rouart, I. (2021). Wolf Attacks on Humans: An Update for 2002–2020. Trondheim: Norwegian Institute for Nature Research.
Linnell, J. D. C., Swenson, J. E., and Andersen, R. (2001). Predators and people: conservation of large carnivores is possible at high human densities if management policy is favourable. Anim. Conserv. 4, 345–349. doi: 10.1017/s1367943001001408
Lischka, S. A., Teel, T. L., Johnson, H. E., Larson, C., Breck, S., and Crooks, K. (2020). Psychological drivers of risk-reducing behaviors to limit human–wildlife conflict. Conserv. Biol. 34, 1383–1392. doi: 10.1111/cobi.13626
López-Mosquera, N., and Sánchez, M. (2012). Theory of planned behavior and the value-belief-norm theory explaining willingness to pay for a suburban park. J. Environ. Manage. 113, 251–262. doi: 10.1016/j.jenvman.2012.08.029
Marino, F., Kansky, R., Shivji, I., Di Croce, A., Ciucci, P., and Knight, A. T. (2021). Understanding drivers of human tolerance to gray wolves and brown bears as a strategy to improve landholder–carnivore coexistence. Conserv. Sci. Pract. 3:e265.
Mech, L. D., and Boitani, L. (2003). Wolves: Behavior, Ecology, and Conservation. Chicago, IL: University of Chicago Press.
Nyhus, P. J. (2016). Human–wildlife conflict and coexistence. Annu. Rev. Environ. Resour. 41, 143–171. doi: 10.1016/j.jenvman.2021.113125
Oberski, D. (2014). lavaan.survey: an R package for complex survey analysis of structural equation models. J. Stat. Softw. 57, 1–27. doi: 10.1002/9781119579038.ch1
Peters, E. (2006). “The functions of affect in the construction of preferences,” in The Construction of Preference, eds S. Lichtenstein and P. Slovic (New York, NY: Cambridge University Press).
Peters, E., and Slovic, P. (1996). The role of affect and worldviews as orienting dispositions in the perception and acceptance of nuclear Power1. J. Appl. Soc. Psychol. 26, 1427–1453. doi: 10.1111/j.1559-1816.1996.tb00079.x
Peters, E., and Slovic, P. (2007). Affective asynchrony and the measurement of the affective attitude component. Cogn. Emot. 21, 300–329. doi: 10.1080/02699930600911440
Petty, R. E., and Krosnick, J. A. (2014). Attitude Strength: Antecedents and Consequences. Hove: Psychology Press.
R Development Core Team (2016). R: A Language and Environment for Statistical Computing. Vienna: R Foundation for Statistical Computing.
Riley, S. J., and Decker, D. J. (2000). Risk perception as a factor in wildlife stakeholder acceptance capacity for cougars in Montana. Hum. Dimens. Wildl. Int. J. 5, 50–62. doi: 10.1080/10871200009359187
Rosseel, Y. (2012). lavaan: an R package for structural equation modeling. J. Stat. Softw. 48, 1–36. doi: 10.3389/fpsyg.2014.01521
Schumacker, R. E., and Lomax, R. G. (2004). A Beginner’s Guide to Structural Equation Modeling. Mahwah, NJ: Lawrence Erlbaum.
Siegrist, M. (2000). The influence of trust and perceptions of risks and benefits on the acceptance of gene technology. Risk Anal. Int. J. 20, 195–204. doi: 10.1111/0272-4332.202020
Siegrist, M., and Cvetkovich, G. (2000). Perception of hazards: the role of social trust and knowledge. Risk Anal. Int. J. 20, 713–720. doi: 10.1111/0272-4332.205064
Siemer, W. F., Hart, P. S., Decker, D. J., and Shanahan, J. E. (2009). Factors that influence concern about human–black bear interactions in residential settings. Hum. Dimens. Wildl. 14, 185–197. doi: 10.1080/10871200902856138
Slagle, K., Bruskotter, J. T., Singh, A., and Schmidt, R. H. (2017). Attitudes toward predator control in the United States: 1995 and 2014. J. Mammal. 98, 7–16. doi: 10.1093/jmammal/gyw144
Slagle, K., Zajac, R. M., Bruskotter, J. T., Wilson, R. S., and Prange, S. (2013). Building tolerance for bears: a communications experiment. J. Wildl. Manag. 77, 863–869. doi: 10.1002/jwmg.515
Slagle, K. M., and Bruskotter, J. T. (2019). “Tolerance for wildlife: a psychological perspective,” in Human–Wildlife Interactions: Turning Conflict into Coexistence, eds B. Frank and J. A. Glikman (Cambridge: Cambridge University Press), 85–106. doi: 10.1186/1472-6785-12-10
Slagle, K. M., Bruskotter, J. T., and Wilson, R. S. (2012). The role of affect in public support and opposition to wolf management. Hum. Dimens. Wildl. 17, 44–57. doi: 10.1080/10871209.2012.633237
Slovic, P. (1999). Trust, emotion, sex, politics, and science: surveying the risk-assessment battlefield. Risk Anal. 19, 689–701. doi: 10.1023/A:1007041821623
Taber, C. S., and Lodge, M. (2006). Motivated skepticism in the evaluation of political beliefs. Am. J. Polit. Sci. 50, 755–769. doi: 10.1111/j.1540-5907.2006.00214.x
Taber, C. S., and Lodge, M. (2016). The illusion of choice in democratic politics: the unconscious impact of motivated political reasoning. Polit. Psychol. 37, 61–85. doi: 10.1111/pops.12321
Thomson, C. J. (1991). Effects of the avidity bias on survey estimates of fishing effort and economic value. Am. Fish. Soc. Symp. 12, 356–366.
Treves, A., Langenberg, J., Lopez-Bao, J., and Rabenhorst, M. (2017). Gray wolf mortality patterns in Wisconsin from 1979–2012. J. Mammal. 98, 17–32. doi: 10.1093/jmammal/gyw145
Vaske, J. J., Miller, C. A., Pallazza, S., and Williams, B. (2021). Attitudes and emotions as predictors of support for wolf management. J. Environ. Psychol. 78:101695. doi: 10.1016/j.jenvp.2021.101695
Vaske, J., Pallazza, S., Miller, C. A., and Williams, B. (2021). Attitudes, emotions, and acceptance of wolf management in Illinois. Hum. Dimens. Wildl. 1–12. doi: 10.1080/10871209.2021.1967526
Visschers, V. H. M., Keller, C., and Siegrist, M. (2011). Climate change benefits and energy supply benefits as determinants of acceptance of nuclear power stations: investigating an explanatory model. Energy Policy 39, 3621–3629. doi: 10.1016/j.enpol.2011.03.064
Weigel, R. H., and Newman, L. (1976). Increasing attitude-behavior correspondence by broadening the scope of the behavioral measure. J. Pers. Soc. Pscychol. 33, 793–802. doi: 10.1037/0022-3514.33.6.793
Wolf, C., and Ripple, W. J. (2017). Range contractions of the world’s large carnivores. R. Soc. Open Sci. 4:170052. doi: 10.1098/rsos.170052
Keywords: carnivores, risk, hazard, benefit, trust, tolerance, wolves (Canis lupus)
Citation: Slagle KM, Wilson RS and Bruskotter JT (2022) Tolerance for Wolves in the United States. Front. Ecol. Evol. 10:817809. doi: 10.3389/fevo.2022.817809
Received: 18 November 2021; Accepted: 10 March 2022;
Published: 31 March 2022.
Edited by:
Bridget Borg, Denali National Park and Preserve, United StatesReviewed by:
Tom Langen, Clarkson University, United StatesMaria Johansson, Lund University, Sweden
Copyright © 2022 Slagle, Wilson and Bruskotter. This is an open-access article distributed under the terms of the Creative Commons Attribution License (CC BY). The use, distribution or reproduction in other forums is permitted, provided the original author(s) and the copyright owner(s) are credited and that the original publication in this journal is cited, in accordance with accepted academic practice. No use, distribution or reproduction is permitted which does not comply with these terms.
*Correspondence: Kristina M. Slagle, Slagle.44@osu.edu