Can environmental information disclosure reduce air pollution? Evidence from China
- 1Accounting School, Chongqing University of Technology, Chongqing, China
- 2Nanyang Technopreneurship Centre, Nanyang Technological University, Singapore, Singapore
- 3Academy of Guangzhou Development, Guangzhou University, Guangzhou, China
- 4School of Economics, Huazhong University of Science and Technology, Wuhan, China
Previous studies have focused on the reduction effect of regulation-based instruments and economic-based instruments on air pollution, ignoring the importance of environmental information disclosure. Based on the Ambient Air Quality Standards (AAQS), a quasi-natural policy implemented in 2012, this paper assesses the effect of environmental information disclosure on air pollution through a dynamic spatial difference-in-difference (DID) model using panel data of 269 cities from 2006 to 2017 in China. We find that the implementation of the AAQS results in a 3% reduction of local PM2.5 concentration and a 3.3% reduction of PM2.5 concentration in the surrounding cities. Further analysis suggests that environmental information disclosure reduces air pollution through enhancing public environmental concerns, green innovation, and industrial upgrading. We also explore the moderating effect of government environmental regulation and heterogeneity analysis in different regions. Our findings suggest that government should further develop the scope and quality of environmental quality information disclosure. Moreover, the local government should provide more support for the green transformation of enterprises and provide necessary support during the transition period. In addition, long-term sustainable environmental protection policies must be able to mobilize regional initiatives for green development.
1 Introduction
In recent years, China’s rapid economic growth has also brought about serious environmental pollution problems (Yu et al., 2021; Bao and Liu, 2022; Duan et al., 2022; Wu et al., 2022). Especially since 2011, air pollution, represented by haze, has received increasing attention. The most important task to deal with haze pollution and improve air quality is to control PM2.5 concentration (Wang et al., 2021b; Hopke and Hill, 2021; Jin et al., 2022; Lin et al., 2022; Renard et al., 2022; Che et al., 2023). As an air pollutant with small particles, PM2.5 can enter the respiratory system from the lungs, thus leading to an increased prevalence of respiratory and neurological diseases (Varotsos, 2013; Bu et al., 2021; Yang et al., 2021; Liu et al., 2022a; Gan et al., 2022; Yuan et al., 2022). In addition, increased PM2.5 concentration significantly limit all types of outdoor activities, resulting in economic losses (Wang et al., 2020). Therefore, how to control PM2.5-based air pollution has become an important academic topic.
Governmental environmental regulation is considered to be an important measure to control air pollution (Chen et al., 2022). In general, government policy instruments for implementing environmental pollution control can be divided into three main categories (Hanley et al., 2016), which are regulation-based instruments, economic-based instruments, and information-based instruments. The evolution of these three policy instruments represents the developmental stages of government environmental regulation (Ding et al., 2022). In the first stage, environmental pollution was restricted mainly through regulation-based instruments. The second stage further promotes the green transformation of firms by raising the costs of high polluting enterprises through market mechanisms. The third stage requires cities or firms to disclose more detailed environmental information to be monitored by the government and the public. In recent years, China’s rapid economic growth has been underpinned by the increasingly serious problem of air pollution (Gangwar et al., 2019; Li and Lu, 2020). The excessive focus on economic development has led local governments to pay less attention to pollution control, resulting in a large number of firms circumventing environmental regulation and emitting various air pollutants illegally (Zhang et al., 2020; Zhang et al., 2022b). Thus, relying only on regulation-based instruments or economic-based instruments is still unable to effectively control air pollution in China. Due to the lack of information disclosure on air pollution in China, the public is unable to effectively assess the air quality in their location, and the government does not have sufficient incentive to strengthen environmental regulation (Ouyang et al., 2019; Zhang et al., 2022a). This is one of the reasons for the limited effect of regulation-based instruments and economic-based instruments on air pollution reduction. Therefore, China implemented the Ambient Air Quality Standards (AAQS) in 2012 to publish real-time PM2.5 concentration in each city, expecting to enhance public awareness of environmental protection and local government regulation to improve air pollution.
Most of the literature focused the reduction effect of regulation-based instruments on air pollution. For example, based on cross-country panel data for 30 OECD countries from 1998 to 2015, the study of Ouyang et al. (2019) shows that the effect of environmental regulation on PM2.5 emissions is non-linear. Using panel data for 248 cities from 2003 to 2016, the study of Wang et al. (2021c) shows that an enhancement of regulation-based instruments significantly reduces air pollution. Some of the literature has also assessed the impact of measures such as the collection of emission fees on pollution control from the perspective of economic-based instruments. The study of Cui et al. (2021) holds that carbon emission trading implementation can significantly reduce your pollution emissions at the firm level. However, there is still a paucity of literature that analyzes the impact of environmental information disclosure on reducing air pollution from the perspective of environmental information disclosure. Most studies on environmental information disclosure have focused on the firm level, mainly discussing which factors enhance corporate environmental information disclosure or how corporate environmental information disclosure affects their business behavior and financial status (Liu and Anbumozhi, 2009; Zhang et al., 2010; Hassan and Ibrahim, 2012). The direct effect of environmental information disclosure on environmental pollution, which is the original purpose of environmental information disclosure, has not been emphasized. Some studies have used the Pollution Information Transparency Index (PITI) for annual evaluation of 113 Chinese cities as a quasi-natural experiment to assess its impact on environmental pollution management (Zhong et al., 2021; Zhang et al., 2022a). However, such NGO evaluation indicators often suffer from subjectivity, timeliness, and comprehensiveness (Brulle et al., 2007). Moreover, 110 of the 113 cities selected for the PITI evaluation are national environmental protection priority cities, and bias in the selection of evaluation targets may lead to biased results on effectiveness of pollution control. In addition, Liu et al. (2021a) used National Ambient Air Quality Monitoring Network (NAAQMN) as a quasi-natural experiment to evaluate its impact on reducing air pollution. The results showed that implementation of NAAQMN resulted in a reduction of PM2.5 concentration by 1.325 mg/m3. However, this study only focuses on the emission reduction effect of PM2.5 monitoring station establishment and ignores the impact of environmental information disclosure from the monitoring stations. The information disclosure of PM2.5 concentration data at monitoring stations can directly affect the public and government concerns and the effectiveness of air pollution control. Therefore, it is necessary to explore in depth the effect of environmental information disclosure, an important initiative, in reducing air pollution.
In this paper, the implementation of AAQS is used as a quasi-natural experiment to assess the impact of environmental information disclosure on reducing air pollution. First, this paper collects balanced panel data of 269 prefecture-level cities from 2006 to 2017 to assess the impact of environmental information disclosure on air pollution using a dynamic spatial DID model. Second, this paper further explores the mechanism of environmental information disclosure affecting air pollution and the moderating effect of government environmental governance. Finally, this paper also conducts some tests to ensure the robustness of the analytical results, including a placebo test, a re-estimation based on PSM-DID and a re-estimation excluding contemporaneous policy interference.
The contributions of this paper can be summarized as follows. First, most studies focus on the impact of environmental regulation on air pollution, but the impact of environmental information disclosure is ignored. With the new Ambient Air Quality Standards as a quasi-natural experiment, this paper the relationship between environmental information disclosure and air pollution. Meanwhile, with the spatial DID model, this paper further investigates the spatial spillover effect which has been ignored in current research. Our findings show that the implementation of AAQS leads to a 3% reduction in PM2.5 concentration not only in the local area, but also in the surrounding area by 3.3%. Therefore, environmental information disclosure should be continuously promoted to improve air quality. Second, this paper discusses in depth the mechanisms by which environmental information disclosure affects air pollution and explores the moderating effect of government environmental regulation. Our findings suggest that environmental information disclosure reduces air pollution by enhancing public environmental concerns, green innovation, and industrial upgrading. In addition, this paper holds that increased government environmental regulation amplifies the air pollution reduction effect of environmental information disclosure. The mechanism analysis is extremely vital since it can be used for government policy making. Finally, this paper explores the differences in the effects of environmental information disclosure across regions. Previous studies related to environmental information disclosure and pollution control have less often analyzed heterogeneity from the perspective of heating and non-heating areas. Our findings suppose that the reduction effect of environmental information disclosure on air pollution is strongest in the eastern region. In addition, the reduction effect of environmental information disclosure on air pollution is much higher in non-heating areas than in heating areas.
The reminder of this study is organized as follows: Section 2 provides the literature review and research hypothesis, Section 3 provides the methods and data, Section 4 provides the results, and Section 5 provides the conclusions and recommendations.
2 Literature review and research hypothesis
2.1 Literature review on air pollution
Air pollution is defined as a phenomenon that occurs when certain substances invade the air in concentrations that are harmful to humans or to the ecosystem on which humans depend (Brauer et al., 2021; Wang et al., 2022c). In recent years, the frequent occurrence of highly hazardous air pollution events has brought about a high level of social concern. To shift the public from concern to better participation in monitoring air quality, a series of measurement standards have been introduced to allow the public to perceive the severity of air pollution at the data level (Lin et al., 2021). Specifically, the concentrations of sulfur dioxide, nitrogen dioxide, carbon monoxide, ozone, PM2.5, and PM10 are commonly chosen to measure air pollution levels (Akbarzadeh et al., 2018). Among these sources, PM2.5 is an important class of pollutants. Due to its small size and ability to be suspended in the air, PM2.5 has the property of carrying pathogens (Bu et al., 2021). When PM2.5 enters the human airways, it not only causes airway obstruction and a series of inflammatory diseases but may even cause cancer through air-blood exchange, which is very destructive to the human body (Yu et al., 2022). Therefore, when people focus on air pollution indicators, they always give priority to PM2.5 concentration.
PM2.5 concentration is influenced by several factors, which can be mainly classified as economic and weather factors (Wu et al., 2020). On the one hand, at the economic level, economic development, population, public transportation, and industrial structure all have an impact on PM2.5 concentration. Ji et al. (2018) used panel data from 2001 to 2010 for 79 developing countries to quantify the socioeconomic drivers of PM2.5 concentration. They found that the effect of urbanization level on PM2.5 concentration differed between low- and high-income zones, showing an inverted U-shaped relationship. Li et al. (2020) studied the relationship between urban spatial structure, especially population distribution, and PM2.5 concentration using a two-way stationary panel regression model based on panel data from 2001 to 2016 for 286 cities in China. Hao et al. (2022) constructed a high-precision estimation model for PM2.5 concentration based on the method of gradient boosted regression tree. They verified the importance of major factors such as traffic conditions, meteorological conditions, and urban spatial layout in PM2.5 concentration estimation and confirmed that public transportation activities contribute significantly to the increase of PM2.5. Xue et al. (2021) reconstructed a high spatial resolution PM2.5 datasets from 2000 to 2018 in Beijing-Tianjin-Hebei region and used vector autoregressive model to investigate the response of PM2.5 to industrial structure. They found that the government needs to promote the optimization of industrial structure through low-carbon technology development or clean energy research to more significantly reduce PM2.5 concentration.
On the other hand, temperature, humidity, precipitation, and sunshine duration are all important factors affecting PM2.5 concentration. Liu et al. (2022b) evaluated the specific contribution of weather patterns to PM2.5 concentration based on spatial effects and health risks. They found that pollutants tend to accumulate in small spaces when the temperature and humidity are high in the region. Moreover, higher relative humidity enhances hygroscopic growth and multiphase reactions of aerosols and inhibits the diffusivity of air pollutants. Therefore, temperature and humidity tend to be positively correlated with PM2.5 concentration. Zhang et al. (2021) analyzed the effects of two winter precipitation events on PM2.5 in Beijing in 2015 and 2016, respectively. Based on the simulation results, they found that precipitation had a significant effect on PM2.5 removal when atmospheric dispersion conditions were favorable. Yang et al. (2022a) took sunlight into account when studying the effect of PM2.5 exposure on vitamin D status of pregnant women. They found that PM2.5 exposure had a significant effect on vitamin D deficiency under weather conditions with short daylight hours. After further analysis, they concluded that the ability of PM2.5 to absorb solar radiation causes a negative correlation between sunlight duration and PM2.5 concentration. In addition, factors such as the level of environmental regulation by the government can also influence PM2.5 concentration (Yu et al., 2021).
2.2 Policy background of ambient air quality standards in China
Similar to other countries, the environmental policies adopted by the Chinese government began with legal constraints based on administrative orders (Yao et al., 2022). Early legal regulations often had a significant environmental improvement effect. However, as productivity developed and production demand increased, many companies began to bypass environmental regulations for production violations, which led to a sharp decline in environmental quality (Gangwar et al., 2019; Li and Lu, 2021). To continuously improve the environment, promote government regulation, and strengthen public oversight, China has begun to focus on the role of environmental information disclosure in pollution control and to meet public demand for environmental information disclosure (Tian et al., 2016). Ambient Air Quality Standard promulgated in 2012 is a policy product of this background. This standard requires that air quality data must be released to the public in a uniform, real-time, and comprehensive manner (Bai et al., 2021). In addition, the government will announce in four batches the monitoring stations set up in the corresponding cities nationwide for the establishment of the ambient air quality network. In May 2012, the government announced the first phase of 496 monitoring stations. The first batch of cities included 74 prefecture-level cities in key regions such as Beijing, Tianjin, Hebei, Yangtze River Delta and Pearl River Delta, as well as municipalities directly under the central government and provincial capitals. The second batch of 388 monitoring stations was announced in March 2013, which included 87 prefecture-level cities such as key national environmental protection cities and model cities. The third batch, consisting of 117 other prefecture-level cities, was announced in May 2014 and contained 552 monitoring sites. In January 2015, the government announced the full rollout of the ambient air quality standards, which contain a total of 1,436 monitoring sites in 338 prefecture-level cities and above nationwide. The spatial distribution of cities implemented the new AAQS are shown in Figure 1. The announcement of ambient air quality standards has facilitated public participation in air quality monitoring, while enriching the government’s means of combating air pollution and strengthening the environmental awareness of enterprises (Bai et al., 2021). As the disclosure of environmental information raises the concern of the whole society about air quality, this may change the air pollution situation especially PM2.5 concentration.
2.3 Research hypothesis
The effective disclosure of environmental information means an increase in environmental awareness throughout society. Once the government, enterprises, and the public start to pay attention to air quality levels, they will take appropriate measures to avoid the intensification of air pollutant concentrations. In addition, the AAQS announcement process is temporally divided into four batches over a 3-year period, and the spatial distribution of monitoring sites in different batches shows a clustering of key areas to a diffusion of non-key areas. Therefore, when the AAQS monitoring points in one region are published, their suppressive effect on air pollutants may have a spatial spillover effect, thus promoting the reduction of air pollutant concentrations in neighboring areas (Wang et al., 2022a). Based on the above analysis, this paper proposes the following Hypothesis 1.
H1:. Environmental information disclosure will reduce local air pollution and reduce air pollution in surrounding areas through spillover effects.Environmental information disclosure can directly affect the concentration of pollutants in the air and may also inhibit air pollution through other pathways. On the one hand, environmental information disclosure enhances public environmental concerns and increases public awareness of environmental protection, which in turn reduces air pollution. For example, Li et al. (2022) explored the effect of public environmental concern on air pollution using air pollution data and Baidu index for prefecture-level cities in China from 2013 to 2018. They found that public environmental concern raised public dissatisfaction and public health risk concern. When concern rises, the pressure on the government starts to become greater, which ultimately promotes government measures to reduce air pollution. Ding et al. (2022) conducted a quasi-natural experiment on pollution information transparency in Chinese cities in 2008 based on the Pollution Information Transparency Index (PITI) to investigate the effect of PITI disclosure on corporate green innovation. They found that environmental information disclosure increased green patenting activities of highly polluting firms. In addition, environmental information disclosure may promote industrial upgrading. Since the tertiary sector possesses lower pollution levels than the secondary sector, once the share of the tertiary sector increases, it can effectively reduce air pollution. Liu et al. (2021b) assessed the impact of national environmental disclosure programs on regional industrial structure upgrading in 2003 and 2017. They found that environmental pollution information disclosure significantly upgraded local industrial structure and promoted ecological transformation at the city level, thereby reducing air pollution. Based on the above analysis, this paper proposes the following Hypothesis 2.
H2:. Environmental information disclosure mainly reduces air pollution by enhancing public environmental concerns, green innovation, and industrial upgrading.The effect of environmental information disclosure on air pollution reduction may also be influenced by the level of government environmental governance. It has been generally accepted in the literature that increased government environmental regulation significantly reduces regional air pollution (Ouyang et al., 2019; Wang et al., 2021c; Chen et al., 2022). Environmental information disclosure enhances public and governmental concern about air pollution issues. For regions with higher levels of environmental regulation, governments respond to public concerns by treating air pollution with more stringent standards (Wang et al., 2021c). However, for regions with lower levels of environmental regulation, environmental concerns cannot enhance the government’s pollution control in the short term, so its effect on reducing air pollution is limited. Therefore, this paper proposes the following Hypothesis 3.
H3:. Increased government environmental regulation will enhance the effect of environmental information disclosure on air pollution reduction.
3 Methods and data
3.1 Data
This paper selects the panel data of 269 prefecture-level cities from 2006 to 2017 as research sample, including 3,228 observations. The sample selection period covers 6 years before and after the implementation of AAQS, which is sufficient to assess the treatment effects of the policy. The data of PM2.5 concentration comes from China’s air quality online monitoring and analysis platform (https://www.aqistudy.cn/). The data of city-level control variables come from Chinese City Statistics Database (CCSD) in Chinese Research Data Services (CNRDS) Platform (https://www.cnrds.com/Home/Index#/FinanceDatabase/DB/CCSD). In this paper, cities with missing samples are excluded to maintain a balanced panel dataset to meet the estimation assumption of spatial panel model.
3.2 Econometric model
This paper uses the DID model to assess the impact of environmental information disclosure, i.e., the implementation of ambient air quality standards (AAQS), on air pollution. In addition, local air pollution will affect the air pollution in surrounding areas, and the air pollution of the previous year will also affect the air pollution level of the current year (Wang et al., 2022b; Yang et al., 2022b). Therefore, this paper incorporates spatial factors and dynamic effects based on the traditional DID model, and employees the dynamic spatial DID model to assess the impact of AAQS on air pollution. The econometric model is specified:
where
where
3.3 Variables
The dependent variable in this study was air pollution. Since the main environmental monitoring index disclosed after the implementation of AAQS is PM2.5 concentration, this paper selects the logarithmic value of PM2.5 concentration in prefecture-level cities as a proxy variable to measure air pollution. The higher the PM2.5 concentration, the more serious the air pollution is. The independent variable in this paper is the implementation of AAQS. According to Eq. 2, the sample after the implementation of AAQS takes the value of 1, and the sample before the implementation of AAQS takes the value of 0. Meanwhile, this paper selects a series of control variables adding into the model to assess the impact of AAQS on air pollution more reliably. The control variables selected in this paper include economic development (lnrgdp), the total population (lnpop), the number of buses (lnbus), the industrial structure (is), temperature (tem), humidity (hum), precipitation (pre) and sunshine duration (Sun) (Wu et al., 2020; Han and Miao, 2022). The measurement and descriptive statistics of each variable are shown in Table 1.
4 Results
4.1 Spatial autocorrelation test
The spatial distribution characteristics of key variables need to be analyzed before using spatial econometric model. This section analyzes the spatial distribution and spatial correlation of urban PM2.5 concentration in China. Figure 2 reports the spatial distribution of urban PM2.5 concentration. It can be seen that the air pollution is most severe in northern China and decreases gradually from its center to the periphery. This indicates that there is a clear spatial correlation of urban PM2.5 concentrations in China. The scatter plot of Moran’s I index can reflect the spatial correlation of urban PM2.5 concentration more visually. Figure 3 shows the scatter plots of PM2.5 concentration in each city in 2006, 2011 and 2017. In these three plots, the horizontal axis denotes the standardized PM2.5 concentration and the vertical axis denotes the spatial lagged values. According to the scatter plots, the coefficients of the main fitted lines are significantly larger than zero, which indicates a positive correlation between air pollution in neighboring cities. Table 2 shows the specific results of the Moran index of urban PM2.5 concentration. The Moran’s I index is significantly positive at the 1% level during the period from 2006 to 2017. The values of Moran’s I index range between 0 and 1. This indicates that urban PM2.5 concentration have a strong spatial correlation. Thus, spatial factors should be considered in the estimation model.
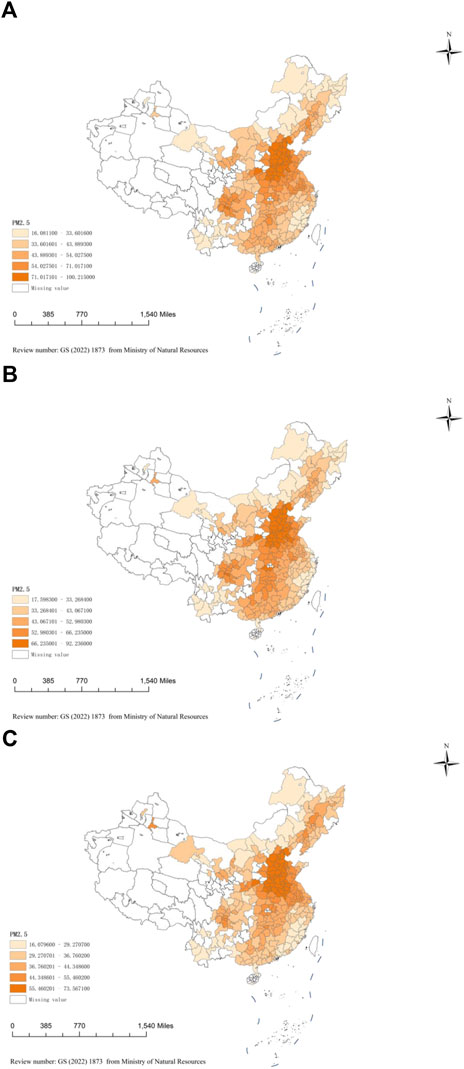
FIGURE 2. Spatial distribution of PM2.5 concentration in China. (A) denotes the maps in 2006, (B) denotes the maps in 2011, and (C) denotes the maps in 2017.
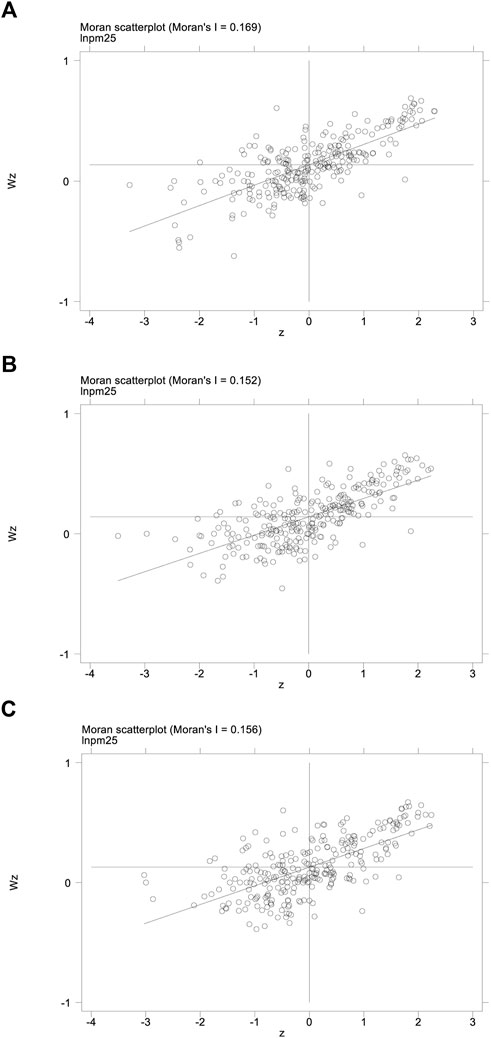
FIGURE 3. Moran scatterplot of urban PM2.5 concentration. (A) denotes the Moran scatterplot in 2006, (B) denotes the Moran scatterplot in 2011, and (C) denotes the Moran scatterplot in 2017.
4.2 Impact of environmental information disclosure on air pollution
According to the hypothesis one in Section 2.3, this paper concludes that environmental information disclosure will reduce air pollution. We adopt a dynamic spatial DID model to estimate the effect of AAQS on urban PM2.5 concentration to test hypothesis 1. The following Table 3 reports the results. Column (1) reports the estimation results of the fixed effects model, while column (2), column (3) and column (4) report the estimation results of SEM, SLM and SDM, respectively.
The coefficient of AAQS in the estimation results based on the FE model is −0.015 (p < 0.01), which passes the 1% significance level test. After considering dynamic effects and spatial lag effects in the FE model, the regression coefficient of AAQS in the SLM estimation results is −0.023 (p < 0.01), which is also significant at the 1% level. After incorporating spatial error effects based on the FE model, the coefficient of AAQS in SEM is −0.037 (p < 0.01). Finally, this paper uses SDM to consider dynamic effects, spatial error effects and spatial lag effects simultaneously. The results show that the coefficient of AAQS is −0.020 (p < 0.01), which passes the 1% significance level test, the results coincide with Zhang et al. (2022c). This indicates that the implementation of the AAQS reduced urban PM2.5 concentration by 2%, i.e., environmental information disclosure significantly reduced air pollution.
The detailed results for SDM are presented in Table 4, SDM further reported the direct, indirect, and total effects of AAQS on PM2.5 concentration. The direct effect is −0.030 (p < 0.01), which indicates that AAQS implementation resulted in a 3% reduction in local PM2.5 concentration. The indirect effect is −0.033 (p < 0.1), which indicates that AAQS implementation leads to a 3.3% reduction in PM2.5 concentration in other areas. The total effect is −0.063 (p < 0.01), which indicates that AAQS implementation leads to an average reduction of 6.3% in PM2.5 concentration in all cities nationwide.
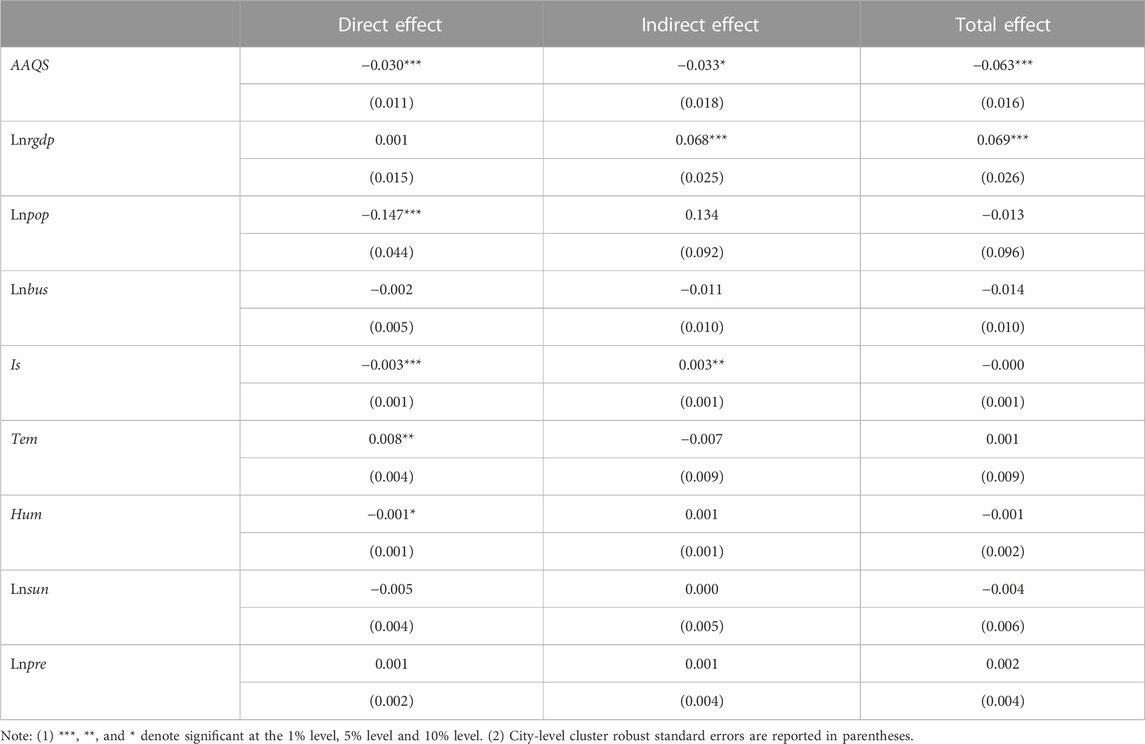
TABLE 4. Direct, indirect, and total effects of SDM in Table 3.
The literature has generally supported that environmental information disclosure significantly reduces air pollution. For example, the study of Bai et al. (2021) showed that AAQS led to significant reductions in PM10 and NO2 concentration in Tianjin from 2014 to 2017. The study of Zhong et al. (2021) also hold that the implementation of PITI resulted in a 0.959% reduction in urban SO2 emissions in China, resulting in an effective reduction in air pollution. However, previous studies do not assess the impact of environmental information disclosure on PM2.5 concentration, and further explore the spillover effect of environmental information disclosure. Our findings suggest that environmental information disclosure not only reduces local air pollution, but also has a significant spillover effect, i.e., reduces air pollution in surrounding areas. This suggests that environmental information disclosure is beneficial to different cities in the region for pollution control. Cities in the region can better improve air quality through cooperation and supervision.
4.3 Parallel trend test
The dynamic spatial DID model estimation is predicated on meeting the parallel trend assumption that there is no significant difference in PM2.5 concentration between the treated group and control group samples before and after AAQS implementation. Figure 4 reports the results of the parallel trend test. None of the regression coefficients passed the significance test prior to the implementation of the AAQS. This indicates that there was no significant difference between the control and experimental groups prior to the implementation of the policy. The hypothesis of a parallel trend was satisfied. In addition, the AAQS had the most significant effect on air pollution reduction in the first year after its implementation. However, that effect was not significant in the second and third years. By the fourth year of AAQS implementation its effect on air pollution gradually stabilized and was significantly negative. This indicates that the effect of AAQS on air pollution reduction is only significant in the short term.
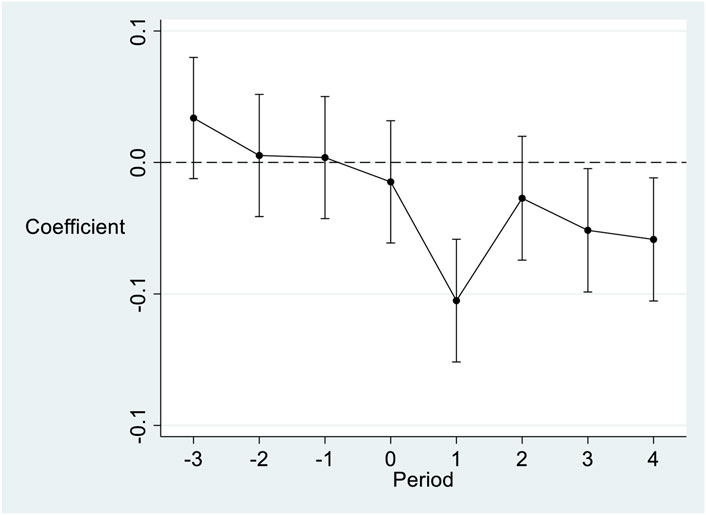
FIGURE 4. Results of Parallel Trend Test. The X-axis denotes the window period for AAQS implementation. The Y-axis represents the regression coefficient of AAQS implementation. The year before AAQS is implemented as the base period.
4.4 Further analysis
4.4.1 Mechanisms of environmental information disclosure reducing air pollution
According to the hypothesis 2, this paper argues that environmental information disclosure affects air pollution mainly through three mechanisms, which are promoting public environmental concern, green innovation, and industrial upgrading. In this section, the following mediating effects model is constructed to test each of these three mechanisms:
where Mit denotes the mediating variable, including public environmental concern, green innovation, and industrial upgrading. The data of public environmental concern are from Baidu search index. Green innovation is measured by the logarithm of the total number of green invention patents and green applicable patents of city i in year t. Industrial upgrading is measured by the ratio of tertiary sector output to secondary sector output of city i in year t. The results of the mechanism test are shown in Table 5.
According to the regressions in Table 5, the regression coefficients of AAQS in column (1), column (3) and column (5) are 1.300 (p < 0.01), 1.435 (p < 0.01) and 0.066 (p < 0.01), which all pass the 1% significance level test. This indicates that environmental information disclosure can significantly promote public environmental concern, green innovation, and industrial upgrading. In addition, the coefficients of epc, green_inn and iu in column (2), column (4) and column (6) are −0.009 (p < 0.01), −0.019 (p < 0.01) and −0.038 (p < 0.05), which all pass the 5% significance level test. Therefore, the implementation of AAQS will not only directly reduce PM2.5 concentration, but also indirectly reduce PM2.5 concentration by enhancing epc, green_inn and iu. Some of the literature emphasizes the importance of environmental information disclosure but does not discuss in depth the mechanisms by which it affects pollution control (Henry and Gordon, 2003; Tu et al., 2020), they highlight the change of public behavior regarding to the environmental information and argue that the public will be more concerned, but the consequences and the mechanism are ignored. Numerous studies have shown that public environmental concern, green innovation and industrial upgrading can effectively reduce air pollution (Liu et al., 2021b; Ding et al., 2022; Li et al., 2022). This section explains the mechanisms of environmental information disclosure to reduce air pollution from the perspective of three mechanisms. The results hold that environmental information disclosure will reduce air pollution by promoting public environmental concern, green innovation, and industrial upgrading i.e., the results of the study indicate support for hypothesis 2.
4.4.2 The moderating effect of government environmental regulation
To test hypothesis 3, i.e., whether government environmental regulation enhances the effect of environmental information disclosure on the reduction of air pollution, the following model is constructed:
where Egit denotes level of government environmental regulation of city i in year t. Egit×AAQSit denotes the interaction term between environmental information disclosure and government environmental regulation. Egit is measured by environmental word frequency. According to the study of Chen et al. (2018), this paper manually collected the text of government work reports from 2006 to 2017 from each prefecture-level city and extracted the reports and processed them by word separation. In this paper, the number of total words and the number of environment-related words in the government reports of each prefecture-level city were counted separately, and the local level of environmental regulation was measured by the proportion of the number of occurrences of environment protection-related words to the total number of words. The words related to the environment include environmental protection, green, pollution, emission reduction, etc. The test results of the above model are shown in Table 6.
According to the results in Table 6, the coefficient of AAQS in column (1) is −0.025 (p < 0.01) and coefficient of Eg in column (2) is −0.016 (p < 0.01), which both pass the 1% significance level test. This suggests that both environmental information disclosure and government environmental regulation can significantly reduce air pollution, and we can further test the moderating effect of government environmental regulation. The coefficient of Eg×AAQS is −0.016 (p < 0.01), which passes the 1% significance level test. The results hold that increased government environmental regulation significantly enhances the effect of environmental information disclosure on reducing air pollution, which is consistent with hypothesis 3. Although previous literature has also emphasized the importance of government environmental regulation in reducing air pollution, it has ignored its moderating effect on environmental information disclosure (Feng et al., 2020; Chen et al., 2022). As a complement to formal environmental regulation, environmental information disclosure and government environmental regulation have complementary effects (Ouyang et al., 2019; Wang et al., 2021a). The findings of this paper suggest that government environmental regulation has a positive moderating effect. Therefore, while environmental information disclosure should be continuously promoted, government environmental regulation should also be enhanced to reduce air pollution more effectively.
4.5 Heterogeneity analysis
There are huge differences in the level of economic development between regions in China, with the eastern region being higher than the central and western regions (Yu et al., 2021). Regions with higher levels of economic development have higher levels of education and complete their industrial upgrading earlier (Zhang et al., 2022a). The effect of environmental information disclosure on reducing air pollution may be stronger than that of regions with lower levels of economic development. Therefore, it is necessary to analyze the differences in the effects of AAQS implementation on air pollution in different regions. In addition, coal-fired heating is one of the important factors affecting urban PM2.5 concentration (Yu et al., 2021). Therefore, a distinction should also be made between heating and non-heating areas when exploring the effect of AAQS implementation on urban PM2.5 concentration. According to the study of Yu et al. (2021), the heating area is north of Qinling and Huaihe River, and the non-heating area is south of Qinling and Huaihe River. In this paper, we further investigate the differences in the effects of AAQS implementation on air pollution between heating and non-heating area. Finally, cities with higher administrative ranks have richer educational resources and innovative technologies, and their marginal effects on air pollution may be diminishing (Yu et al., 2021; Ding et al., 2022). As a result, the impact of AAQS may differ across different cities. Therefore, differences among cities with different administrative ranks should be considered when assessing the impact of AAQS implementation on PM2.5 concentration. The results of heterogeneity analysis are shown in Table 7.
According to the results in Table 7, the regression coefficient of AAQS×Central in column (1) is 0.023, which passes the 1% significance level test. The effect of AAQS on air pollution in the central region is significantly lower than that in the eastern region, with a reduction effect of only 0.1%. The regression coefficient of AAQS×Western is −0.009, which does not pass the 10% significance level test, indicating that there is no significant difference between the effects of AAQS on air pollution in the eastern and western regions. The coefficient of AAQS×Heating in column (2) is 0.035, which passes the 1% significance level test. This indicates that AAQS can significantly reduce PM2.5 concentration in non-heating areas by 4.4%. For heating zones, the effect of AAQS implementation on PM2.5 concentration reduction is only 0.9%. In column (3), the coefficient of AAQS×Rank is 0.006, which fails to pass the 10% significance level test. This indicates that the effect of AAQS on air pollution does not differ significantly among cities at different administrative levels.
Our findings suggest that the effect of environmental disclosure on air pollution differs significantly across economic development levels and heating and non-heating zones. This is more consistent with the study of Yu et al. (2021), whose findings suggest that the impact of coal-to-gas policy on air pollution differs significantly between the eastern, central and western and the heating and non-heating areas. This paper argues that the main factors that lead to differences in the impact of environmental information disclosure in different regions are influenced by education level and technology level. Higher education levels can lead to stronger public environmental concerns, thus amplifying the effect of environmental information disclosure on air pollution reduction (Gu et al., 2021). Unlike Liu et al. (2021b), our results does not show statistical difference in terms of heterogeneity in administrative rank of cities. Although the EID is not directly environmental regulation in China, however, as the concentration of PM2.5 is relatively high, the government may directly intervene and force related firms to reduce their pollutant emission since the data is public. Meanwhile, the public is also informed, they may also pressure local governments in improving air quality. As a result, the impact of EID is statistically indifferent for cities with different administrative rank.
4.6 Robustness
4.6.1 Placebo test
Since the implementation of AAQS may also affect urban PM2.5 concentration that do not make environmental information disclosures, this may reduce the reliability of the estimation results. Therefore, this paper adopts Monte Carlo simulation to conduct a placebo test, and the results are shown in Figure 5. This paper randomly selected samples from the control group multiple times as the treated group. Then, this paper uses the DID model for re-estimation and extracts the estimated parameters. The analysis results in this paper are reliable if the estimated parameters are normally distributed and have a mean value of 0. The estimated coefficient distributions and kernel density curves after 500 random samples are given in Figure 5. As expected from the placebo test, the estimated coefficients show a normal distribution with a mean around 0. This indicates that the reduction in PM2.5 concentration in the treated group came from the implementation of the AAQS.
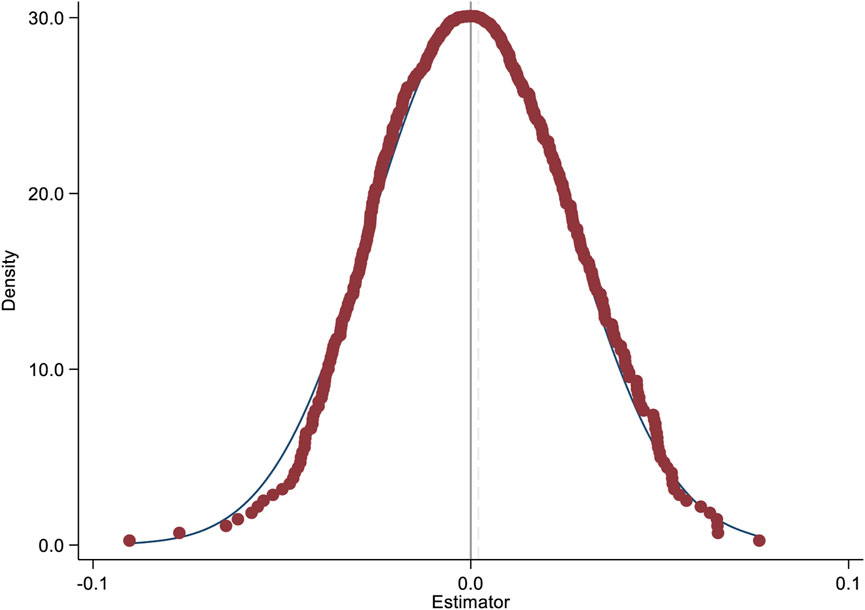
FIGURE 5. Results of placebo test. Treated group is randomly drawn 500 times in the control group by Monte Carlo simulation and DID regression was performed. Plot the obtained regression coefficients as a distribution graph. This figure reports the results of PM2.5 concentration of cities without environmental information disclosures as a dependent variable, presenting a normal distribution with an average value of 0.
4.6.2 Re-estimation based on PSM-DID
To ensure that the sample selection bias does not affect the reliability of the conclusions in this paper, we use the difference-in-difference model after propensity score matching (PSM-DID) for re-estimation. The results of PSM-DID are shown in the following Table 8.
According to the regression results in Table 8 above, the regression coefficients of AAQS in columns (2) and (4) were −0.014 (p < 0.05) and −0.022 (p < 0.01), respectively, both of which passed the 5% significance level test. The PSM-DID based on nearest neighbor matching with kernel density matching obtained the same conclusion as Table 3. This indicates that environmental information disclosure can have a reduction in air pollution, i.e., the results of the previous analysis are robust.
4.6.3 Re-estimation excluding other policy disturbances
To exclude the interference of other contemporaneous policies on the analysis results, this paper further controls for the policy shocks of Pollution Information Transparency Index (PITI), Low Carbon City Pilot (LCCP), Carbon Emission Trading Pilot (CETP) and Air Pollution Prevention and Control Program (APCP) in the model (Feng et al., 2019; Cui et al., 2021; Zhang et al., 2022a). The results of the effects of AAQS on urban PM2.5 concentration after adding the above four policy shocks are shown in Table 9.
According to the results in Table 9, the regression coefficients of AAQS in columns (1) to (4) are −0.024 (p < 0.01), −0.022 (p < 0.01), −0.023 (p < 0.01) and −0.023 (p < 0.01), respectively, all of which pass the 1% significance level test. This indicates that the effect of AAQS on the reduction of urban PM2.5 concentration remains robust after considering the contemporaneous policies. Therefore, environmental information disclosure can effectively reduce air pollution.
5 Conclusion and discussion
5.1 Conclusion
Through a dynamic spatial DID model, this paper uses AAQS as a quasi-natural experiment to assess the impact of environmental information disclosure on air pollution. Through empirical analysis, we obtain the following three conclusions. First, implementation of the AAQS resulted in a 3% reduction in local PM2.5 concentration and a 3.3% reduction in PM2.5 concentration in surrounding areas. The total reduction effect of the AAQS on PM2.5 concentration in the sample cities was 6.3%. Our findings suggest that environmental information disclosure not only reduces local air pollution, but also has significant spillover effects. Second, this paper further explores the mechanism by which environmental information disclosure affects air pollution. Our findings suggest that environmental information disclosure reduces air pollution by enhancing public environmental concerns, green innovation, and industrial upgrading. In addition, we examine the externalities of government environmental regulation using a mediating effects model. The findings hold that the increase in government environmental regulation enhances the effect of environmental information disclosure on air pollution reduction. Third, the results of the heterogeneity analysis show that the effect of environmental information disclosure on air pollution varies across regions. In terms of economic development level, the reduction effect of environmental information disclosure on air pollution is strongest in the more developed eastern region. The effect of environmental information disclosure on air pollution in the central region is significantly lower than that in the eastern region. In addition, the reduction effect of environmental information disclosure on air pollution in non-heating areas is much higher than that in heating areas. We also performed a series of robustness tests to ensure the reliability of the findings, including placebo tests, re-estimation based on PSM-DID, and the re-estimation excluding contemporaneous policy interference. The robustness tests yielded consistent conclusions.
This paper contributes to current literature in the following aspects. First, we investigate the impact of EID on air quality with a quasi-natural experiment in China, the implementation of the new Ambient Air Quality Standards helps us better identify the causal inference. And the spatial DID model is employed to identify the spatial spillover effect. Second, we further investigate the impact mechanism between EID and air quality which has rarely been explored in current studies. Three channels are investigated: public environmental concern, green innovation, and industrial upgrading. Finally, the heterogeneity of cities is considered, including location, heating approach, and the administrative rank.
5.2 Discussion
Based on the findings of the study, this paper proposes the following three recommendations. First, government should further develop the scope and quality of environmental quality information disclosure. Findings of this paper show that environmental information disclosure can significantly reduce urban air pollution. Therefore, the state should speed up the introduction of mandatory environmental information disclosure rules for wastewater, waste gas, and other areas in various environmental protection areas where technical conditions and cost inputs allow. It is also important to disclose environmental quality indicators that have a greater impact on residents’ health and social welfare to the society and to improve the quality of environmental information data disclosure. These measures can effectively promote public participation in regional pollution prevention and control and enhance the construction of a shared governance system for environmental governance. Second, the government should expand the impact of environmental information disclosure by enhancing public awareness of environmental protection, encouraging enterprises to engage in green innovation, and promoting the clustering of high-tech industries. Enterprises should make full use of the preferential policies for green development, improve their R&D capabilities, and accelerate the pace of green transformation. Only by turning the pressure of pollution reduction into the motivation of upgrading, the internal upgrading from polluting production to clean production can be completed. The local government should provide more support for the green transformation of enterprises and provide necessary support during the transition period. Finally, the government should construct a cross-regional synergistic system for pollution prevention and control. The findings of this paper suggest that there is a spatial spillover effect of environmental information disclosure on air pollution. Long-term sustainable environmental protection policies must be able to mobilize regional initiatives for green development. An ecological compensation system based on the effectiveness of pollution prevention and control can enable regions with different levels of economic development to continuously improve the quality of the regional ecological environment. Cities in the region should provide timely feedback on the process and results of environmental pollution events based on the regional environmental quality information platform.
This paper highlights the important role of environmental information disclosure in improving air quality. However, there are still some limitations in this paper. Our data only contains city-level sample, not firm-level sample. However, firms are the entities of air pollution, thus, the conclusion will be more precise if firm-specific data is employed. Moreover, the impact of EID should differ across different industries, existing data does not provide this difference. As a result, for future research, one can complete the dataset with firm-level samples, which will precisely identify the firm- and industry-specific heterogeneity.
Data availability statement
Publicly available datasets were analyzed in this study. This data can be found here: Chinese City Statistics Database (CCSD) in Chinese Research Data Services (CNRDS) Platform (https://www.cnrds.com/Home/Index#/FinanceDatabase/DB/CCSD).
Author contributions
LX, HL contributed to conception and design of the study. ZW organized the database. XZ performed the statistical analysis. CY wrote the first draft of the manuscript.
Funding
LX acknowledges the financial supports sponsored by the National Social Science Foundation of China (Grant No. 20CJY040) and the Scientific Research Foundation of Chongqing University of Technology.
Conflict of interest
The authors declare that the research was conducted in the absence of any commercial or financial relationships that could be construed as a potential conflict of interest.
Publisher’s note
All claims expressed in this article are solely those of the authors and do not necessarily represent those of their affiliated organizations, or those of the publisher, the editors and the reviewers. Any product that may be evaluated in this article, or claim that may be made by its manufacturer, is not guaranteed or endorsed by the publisher.
References
Akbarzadeh, M. A., Khaheshi, I., Sharifi, A., Yousefi, N., Naderian, M., Namazi, M. H., et al. (2018). The association between exposure to air pollutants including PM10, PM2.5, ozone, carbon monoxide, sulfur dioxide, and nitrogen dioxide concentration and the relative risk of developing stemi: A case-crossover design. Environ. Res. 161, 299–303. doi:10.1016/j.envres.2017.11.020
Bai, Y., Ni, Y., and Zeng, Q. (2021). Impact of ambient air quality standards revision on the exposure-response of air pollution in Tianjin, China. Environ. Res. 198, 111269. doi:10.1016/j.envres.2021.111269
Bao, R., and Liu, T. (2022). How does government attention matter in air pollution control? Evidence from government annual reports. Resour. Conserv. Recycl. 185, 106435. doi:10.1016/j.resconrec.2022.106435
Brauer, M., Casadei, B., Harrington, R. A., Kovacs, R., Sliwa, K., Brauer, M., et al. (2021). Taking a stand against air pollution—the impact on cardiovascular disease. J. Am. Coll. Cardiol. 77, 1684–1688. doi:10.1016/j.jacc.2020.12.003
Brulle, R., Turner, L., Carmichael, J., and Jenkins, J. (2007). Measuring social movement organization populations: A comprehensive census of U.S. Environmental movement organizations. Mobilization Int. Q. 12, 255–270. doi:10.17813/maiq.12.3.j08421508773764m
Bu, X., Xie, Z., Liu, J., Wei, L., Wang, X., Chen, M., et al. (2021). Global PM2.5-attributable health burden from 1990 to 2017: Estimates from the Global Burden of disease study 2017. Environ. Res. 197, 111123. doi:10.1016/j.envres.2021.111123
Che, W., Zhang, Y., Lin, C., Fung, Y. H., Fung, J. C. H., and Lau, A. K. H. (2023). Impacts of pollution heterogeneity on population exposure in dense urban areas using ultra-fine resolution air quality data. J. Environ. Sci. 125, 513–523. doi:10.1016/j.jes.2022.02.041
Chen, Z., Kahn, M. E., Liu, Y., and Wang, Z. (2018). The consequences of spatially differentiated water pollution regulation in China. J. Environ. Econ. Manag. 88, 468–485. doi:10.1016/j.jeem.2018.01.010
Chen, Y., Yao, Z., and Zhong, K. (2022). Do environmental regulations of carbon emissions and air pollution foster green technology innovation: Evidence from China’s prefecture-level cities. J. Clean. Prod. 350, 131537. doi:10.1016/j.jclepro.2022.131537
Cui, J., Wang, C., Zhang, J., and Zheng, Y. (2021). The effectiveness of China’s regional carbon market pilots in reducing firm emissions. Proc. Natl. Acad. Sci. 118, e2109912118. doi:10.1073/pnas.2109912118
Ding, J., Lu, Z., and Yu, C.-H. (2022). Environmental information disclosure and firms’ green innovation: Evidence from China. Int. Rev. Econ. Finance 81, 147–159. doi:10.1016/j.iref.2022.05.007
Duan, J., Mao, S., Xie, P., Lang, J., Li, A., Tong, J., et al. (2022). Key emergency response technologies for abrupt air pollution accidents in China. J. Environ. Sci. 123, 235–254. doi:10.1016/j.jes.2022.03.030
Feng, Y., Ning, M., Lei, Y., Sun, Y., Liu, W., and Wang, J. (2019). Defending blue sky in China: Effectiveness of the “air pollution prevention and control action plan” on air quality improvements from 2013 to 2017. J. Environ. Manage. 252, 109603. doi:10.1016/j.jenvman.2019.109603
Feng, T., Du, H., Lin, Z., and Zuo, J. (2020). Spatial spillover effects of environmental regulations on air pollution: Evidence from urban agglomerations in China. J. Environ. Manage. 272, 110998. doi:10.1016/j.jenvman.2020.110998
Gan, T., Bambrick, H., Tong, S., and Hu, W. (2022). Air pollution and liver cancer: A systematic review. J. Environ. Sci. 126, 817–826. doi:10.1016/j.jes.2022.05.037
Gangwar, C., Choudhari, R., Chauhan, A., Kumar, A., Singh, A., and Tripathi, A. (2019). Assessment of air pollution caused by illegal e-waste burning to evaluate the human health risk. Environ. Int. 125, 191–199. doi:10.1016/j.envint.2018.11.051
Gu, Y., Ho, K.-C., Yan, C., and Gozgor, G. (2021). Public environmental concern, CEO turnover, and green investment: Evidence from a quasi-natural experiment in China. Energy Econ. 100, 105379. doi:10.1016/j.eneco.2021.105379
Han, S., and Miao, C. (2022). Does a polycentric spatial structure help to reduce industry emissions? Int. J. Environ. Res. Public. Health 19, 8167. doi:10.3390/ijerph19138167
Hanley, N., Shogren, J. F., and White, B. (2016). Environmental economics: In theory and practice. Macmillan International Higher Education.
Hao, X., Hu, X., Liu, T., Wang, C., and Wang, L. (2022). Estimating urban PM2.5 concentration: An analysis on the nonlinear effects of explanatory variables based on gradient boosted regression tree. Urban Clim. 44, 101172. doi:10.1016/j.uclim.2022.101172
Hassan, A., and Ibrahim, E. (2012). Corporate environmental information disclosure: Factors influencing companies’ success in attaining environmental awards. Corp. Soc. Responsib. Environ. Manag. 19, 32–46. doi:10.1002/csr.278
Henry, G. T., and Gordon, C. S. (2003). Driving less for better air: Impacts of a public information campaign. J. Pol. Anal. Manag. 22 (1), 45–63. doi:10.1002/pam.10095
Hopke, P. K., and Hill, E. L. (2021). Health and charge benefits from decreasing PM2.5 concentrations in New York State: Effects of changing compositions. Atmos. Pollut. Res. 12, 47–53. doi:10.1016/j.apr.2021.01.018
Ji, X., Yao, Y., and Long, X. (2018). What causes PM2.5 pollution? Cross-economy empirical analysis from socioeconomic perspective. Energy Policy 119, 458–472. doi:10.1016/j.enpol.2018.04.040
Jin, H., Chen, X., Zhong, R., and Liu, M. (2022). Influence and prediction of PM2.5 through multiple environmental variables in China. Sci. Total Environ. 849, 157910. doi:10.1016/j.scitotenv.2022.157910
Li, H., and Lu, J. (2020). Can regional integration control transboundary water pollution? A test from the Yangtze River economic belt. Environ. Sci. Pollut. Res. 27, 28288–28305. doi:10.1007/s11356-020-09205-1
Li, H., and Lu, J. (2021). Can stable environmental protection officials’ tenure reduce illegal emissions? Socioecon. Plann. Sci. 78, 101055. doi:10.1016/j.seps.2021.101055
Li, Y., Zhu, K., and Wang, S. (2020). Polycentric and dispersed population distribution increases PM2.5 concentrations: Evidence from 286 Chinese cities, 2001–2016. J. Clean. Prod. 248, 119202. doi:10.1016/j.jclepro.2019.119202
Li, X., Hu, Z., Cao, J., and Xu, X. (2022). The impact of environmental accountability on air pollution: A public attention perspective. Energy Policy 161, 112733. doi:10.1016/j.enpol.2021.112733
Lin, Y., Huang, R., and Yao, X. (2021). Air pollution and environmental information disclosure: An empirical study based on heavy polluting industries. J. Clean. Prod. 278, 124313. doi:10.1016/j.jclepro.2020.124313
Lin, T., Qian, W., Wang, H., and Feng, Y. (2022). Air pollution and workplace choice: Evidence from China. Int. J. Environ. Res. Public. Health 19, 8732. doi:10.3390/ijerph19148732
Liu, G., Dong, X., Kong, Z., and Dong, K. (2021a). Does national air quality monitoring reduce local air pollution? The case of PM2.5 for China. J. Environ. Manage. 296, 113232. doi:10.1016/j.jenvman.2021.113232
Liu, S., Liu, C., and Yang, M. (2021b). The effects of national environmental information disclosure program on the upgradation of regional industrial structure: Evidence from 286 prefecture-level cities in China. Struct. Change Econ. Dyn. 58, 552–561. doi:10.1016/j.strueco.2021.07.006
Liu, G., Li, Y., Zhou, J., Xu, J., and Yang, B. (2022a). PM2.5 deregulated microRNA and inflammatory microenvironment in lung injury. Environ. Toxicol. Pharmacol. 91, 103832. doi:10.1016/j.etap.2022.103832
Liu, J., Ruan, Z., Gao, X., Yuan, Y., and Dong, S. (2022b). Quantifying contribution of weather patterns to PM2.5 concentrations based on spatial effects and health risk assessment. Sustain. Cities Soc. 83, 103980. doi:10.1016/j.scs.2022.103980
Liu, X., and Anbumozhi, V. (2009). Determinant factors of corporate environmental information disclosure: An empirical study of Chinese listed companies. J. Clean. Prod. 17, 593–600. doi:10.1016/j.jclepro.2008.10.001
Ouyang, X., Shao, Q., Zhu, X., He, Q., Xiang, C., and Wei, G. (2019). Environmental regulation, economic growth and air pollution: Panel threshold analysis for OECD countries. Sci. Total Environ. 657, 234–241. doi:10.1016/j.scitotenv.2018.12.056
Renard, J.-B., Surcin, J., Annesi-Maesano, I., Delaunay, G., Poincelet, E., and Dixsaut, G. (2022). Relation between PM2.5 pollution and covid-19 mortality in western europe for the 2020–2022 period. Sci. Total Environ. 848, 157579. doi:10.1016/j.scitotenv.2022.157579
Tian, X.-L., Guo, Q.-G., Han, C., and Ahmad, N. (2016). Different extent of environmental information disclosure across Chinese cities: Contributing factors and correlation with local pollution. Glob. Environ. Change 39, 244–257. doi:10.1016/j.gloenvcha.2016.05.014
Tu, M., Zhang, B., Xu, J., and Lu, F. (2020). Mass media, information and demand for environmental quality: Evidence from the “under the dome”. J. Dev. Econ. 143, 102402. doi:10.1016/j.jdeveco.2019.102402
Varotsos, C. (2013). The grand challenges to air pollution. Front. Environ. Sci. 1. doi:10.3389/fenvs.2013
Wang, J., Zhang, L., Niu, X., and Liu, Z. (2020). Effects of PM2.5 on health and economic loss: Evidence from Beijing-Tianjin-Hebei region of China. J. Clean. Prod. 257, 120605. doi:10.1016/j.jclepro.2020.120605
Wang, K.-L., Xu, R.-Y., Zhang, F.-Q., Miao, Z., and Peng, G. (2021a). Spatiotemporal heterogeneity and driving factors of PM2.5 reduction efficiency: An empirical analysis of three urban agglomerations in the Yangtze River Economic Belt, China. Ecol. Indic. 132, 108308. doi:10.1016/j.ecolind.2021.108308
Wang, R., Huang, J., Zhang, L., Xia, Y., Xu, X., and Nong, T. (2021b). Assessments of air pollution control effectiveness based on a sharp regression discontinuity design —evidence from China’s environmental big data. Front. Environ. Sci. 9, 724716. doi:10.3389/fenvs.2021.724716
Wang, T., Peng, J., and Wu, L. (2021c). Heterogeneous effects of environmental regulation on air pollution: Evidence from China’s prefecture-level cities. Environ. Sci. Pollut. Res. 28, 25782–25797. doi:10.1007/s11356-021-12434-7
Wang, H., Yang, G., Ouyang, X., and Tan, Z. (2022a). Does environmental information disclosure promote the supply of environmental public goods? Evidence based on a dynamic spatial panel durbin model. Environ. Impact Assess. Rev. 93, 106725. doi:10.1016/j.eiar.2021.106725
Wang, Q., Zhu, H., Xu, H., Lu, K., Ban, J., Ma, R., et al. (2022b). The spatiotemporal trends of PM2.5- and O3-related disease burden coincident with the reduction in air pollution in China between 2005 and 2017. Resour. Conserv. Recycl. 176, 105918. doi:10.1016/j.resconrec.2021.105918
Wang, Z., Yang, J., and Jiang, J. (2022c). Urban sprawl and haze pollution: Based on raster data of haze PM2.5 concentrations in 283 cities in mainland China. Front. Environ. Sci. 10, 929558. doi:10.3389/fenvs.2022.929558
Wu, W., Zhang, M., and Ding, Y. (2020). Exploring the effect of economic and environment factors on PM2.5 concentration: A case study of the beijing-tianjin-hebei region. J. Environ. Manage. 268, 110703. doi:10.1016/j.jenvman.2020.110703
Wu, G., Tian, W., Zhang, L., and Yang, H. (2022). The Chinese spring festival impact on air quality in China: A critical review. Int. J. Environ. Res. Public. Health 19, 9074. doi:10.3390/ijerph19159074
Xue, W., Zhang, J., Zhong, C., Li, X., and Wei, J. (2021). Spatiotemporal PM2.5 variations and its response to the industrial structure from 2000 to 2018 in the Beijing-Tianjin-Hebei region. J. Clean. Prod. 279, 123742. doi:10.1016/j.jclepro.2020.123742
Yang, X., Zhang, T., Zhang, Y., Chen, H., and Sang, S. (2021). Global burden of copd attributable to ambient PM2.5 in 204 countries and territories, 1990 to 2019: A systematic analysis for the global burden of disease study 2019. Sci. Total Environ. 796, 148819. doi:10.1016/j.scitotenv.2021.148819
Yang, D., Chen, L., Yang, Y., Shi, J., Huang, Z., Li, M., et al. (2022a). Effect of PM2.5 exposure on vitamin D status among pregnant women: A distributed lag analysis. Ecotoxicol. Environ. Saf. 239, 113642. doi:10.1016/j.ecoenv.2022.113642
Yang, Q., Liu, G., Gonella, F., Chen, Y., Liu, C., Zhao, H., et al. (2022b). Assessing the temporal-spatial dynamic reduction in ecosystem services caused by air pollution: A near-real-time data perspective. Resour. Conserv. Recycl. 180, 106205. doi:10.1016/j.resconrec.2022.106205
Yao, L., Luo, Y., Wang, Y., and Liu, H. (2022). Market response to the hierarchical water environment regulations on heavily polluting firm: Evidence from China. Water Resour. Econ. 39, 100201. doi:10.1016/j.wre.2022.100201
Yu, C., Kang, J., Teng, J., Long, H., and Fu, Y. (2021). Does coal-to-gas policy reduce air pollution? Evidence from a quasi-natural experiment in China. Sci. Total Environ. 773, 144645. doi:10.1016/j.scitotenv.2020.144645
Yu, P., Xu, R., Li, S., Coelho, M. S. Z. S., Saldiva, P. H. N., Sim, M. R., et al. (2022). Associations between long-term exposure to PM2.5 and site-specific cancer mortality: A nationwide study in Brazil between 2010 and 2018. Environ. Pollut. 302, 119070. doi:10.1016/j.envpol.2022.119070
Yuan, J., Shi, L., Li, H., Zhou, J., Zeng, L., Cheng, Y., et al. (2022). The burden of neonatal diseases attributable to ambient PM 2.5 in China from 1990 to 2019. Front. Environ. Sci. 10, 828408. doi:10.3389/fenvs.2022.828408
Zhang, L., Mol, A. P. J., He, G., and Lu, Y. (2010). An implementation assessment of China’s environmental information disclosure decree. J. Environ. Sci. 22, 1649–1656. doi:10.1016/S1001-0742(09)60302-8
Zhang, M., Liu, X., Sun, X., and Wang, W. (2020). The influence of multiple environmental regulations on haze pollution: Evidence from China. Atmos. Pollut. Res. 11, 170–179. doi:10.1016/j.apr.2020.03.008
Zhang, H., Jiang, Q., Wang, J., Li, K., and Wang, F. (2021). Analysis on the impact of two winter precipitation episodes on PM2.5 in Beijing. Environ. Sci. Ecotechnology 5, 100080. doi:10.1016/j.ese.2021.100080
Zhang, H., Xu, T., and Feng, C. (2022a). Does public participation promote environmental efficiency? Evidence from a quasi-natural experiment of environmental information disclosure in China. Energy Econ. 108, 105871. doi:10.1016/j.eneco.2022.105871
Zhang, W., Luo, Q., and Liu, S. (2022b). Is government regulation a push for corporate environmental performance? Evidence from China. Econ. Anal. Policy 74, 105–121. doi:10.1016/j.eap.2022.01.018
Zhang, X., Wang, Y., Zhang, Z., and Long, H. (2022c). How does environmental information disclosure affect public health? Evidence from the new ambient air quality standards. Int. J. Environ. Res. Public Health. 19, 15141. doi:10.3390/ijerph192215141
Keywords: air pollution, environmental information disclosure, ambient air quality standards, dynamic spatial difference-in-difference model, spillover effects
Citation: Xiong L, Long H, Zhang X, Yu C and Wen Z (2023) Can environmental information disclosure reduce air pollution? Evidence from China. Front. Environ. Sci. 11:1126565. doi: 10.3389/fenvs.2023.1126565
Received: 18 December 2022; Accepted: 18 January 2023;
Published: 02 February 2023.
Edited by:
Xiaowei Chuai, Nanjing University, ChinaReviewed by:
Deng Rui, Southwest University of Political Science and Law, ChinaXiaojun Deng, Zhejiang University of Finance and Economics, China
Copyright © 2023 Xiong, Long, Zhang, Yu and Wen. This is an open-access article distributed under the terms of the Creative Commons Attribution License (CC BY). The use, distribution or reproduction in other forums is permitted, provided the original author(s) and the copyright owner(s) are credited and that the original publication in this journal is cited, in accordance with accepted academic practice. No use, distribution or reproduction is permitted which does not comply with these terms.
*Correspondence: Hongyu Long, LONG0071@e.ntu.edu.sg; Xiang Zhang, zhangxiang@cqut.edu.cn; Chenyang Yu, yuchenyang@gzhu.edu.cn