- 1Division of Endocrinology and Metabolism, Department of Medicine, Samsung Medical Center, Sungkyunkwan University School of Medicine, Seoul, Republic of Korea
- 2Department of Clinical Research Design and Evaluation, Samsung Advanced Institute for Health Sciences and Technology, Sungkyunkwan University, Seoul, Republic of Korea
Aim: We explored the effectiveness of continuous glucose monitoring for 1 year on glycated A1c reduction in adults with type 1 diabetes mellitus.
Methods: We included type 1 diabetes mellitus adults who were either new continuous glucose monitoring users (N = 155) or non-users who were under standard care (N = 384). Glycated A1c was measured at baseline and 3, 6, 9, and 12 months. Individuals with (N = 155) or without continuous glucose monitoring use (N = 310) were matched 1:2 by propensity score. We used the linear mixed models to identify the quantitative reduction in repeated measures of glycated A1c.
Results: The change in glycated A1c from baseline to 12 months was −0.5% ± 1.0% for the continuous glucose monitoring user group (N = 155, P < 0.001) and −0.01% ± 1.0% for the non-user group (N = 310, P = 0.816), with a significant difference between the two groups (P = 0.003). Changes in glycated A1c were significant at 3, 6, 9, and 12 months compared with those at baseline in patients using continuous glucose monitoring (P < 0.001), and the changes differed significantly between the groups (P < 0.001). A linear mixed model showed an adjusted treatment group difference in mean reduction in glycated A1c of −0.11% (95% confidence interval, −0.16 to −0.06) each three months. In the continuous glucose monitoring user group, those who achieved more than 70% of time in range significantly increased from 3 months (37.4%) to 12 months (48.2%) (P < 0.001).
Conclusion: In this longitudinal study of type 1 diabetes mellitus adults, the use of continuous glucose monitoring for 1 year showed a significant reduction in glycated A1c in real-world practice.
Introduction
Hemoglobin A1c (HbA1c) has been the gold standard marker for assessing glycemic status and predicting diabetes complications, but it provides limited information that cannot reflect glycemic variability and the presence of severe hypoglycemia or hyperglycemia (1, 2). Continuous glucose monitoring (CGM) demonstrates a continuous measurement of glucose levels over time and detects glucose variations with CGM metrics (3). The main CGM metrics including time in range (TIR), time below range (TBR), and time above range (TAR) inform more personalized glycemic profiles compared with HbA1c (4). Studies have demonstrated that the use of CGM improved glycemic control in patients with type 1 diabetes mellitus (T1DM) using daily insulin injection (5–8). In various diabetes guidelines, the use of CGM is suggested as a standard treatment for the management of T1DM (9, 10). In addition, the use of CGM in T1DM is continuously expanding due to recent improvements in the accuracy and period of use of the sensor and the expansion of the national reimbursement policy (11). However, to the best of our knowledge, longitudinal studies determining the effectiveness of CGM on glycemic control in adults with T1DM under routine clinical care are limited. In the current study, we therefore evaluated the association between CGM use for 1 year and glycemic control among patients with T1DM in a longitudinal real-world setting. In addition, we investigated longitudinal changes of CGM-derived metrics in T1DM patients who used CGM for 1 year.
Materials and methods
Study population and study design
This propensity-matched, single-center, 1-year longitudinal study was conducted at Samsung Medical Center (SMC) in the Republic of Korea. In the CGM user cohort, patients with diabetes mellitus (DM) who visited the outpatient clinic of the Endocrinology Department of SMC between January 2019 and April 2022 and consented to the study requirements to initiate CGM use were prospectively enrolled (N = 595, Figure 1). The use of CGM was recommended by the physicians and included those who consented to its use. Among them, those who applied CGM for at least 70% of the study period (N = 329) and those who continued to visit the outpatient clinic for more than 1 year (N = 171) were included. Also, patients with type 2 diabetes mellitus (T2DM) who had ICD-10 (International Classification of Diseases 10th Revision) code of E11–14 (N = 15) and missing data for covariates (N = 1) were excluded. Finally, a total of 155 people were analyzed as CGM users. They continued to use CGM for 1 year, and HbA1c levels were measured at baseline and 3, 6, 9, and 12 months. CGM data were collected during regular clinical visits each three months for the 12-month study period.
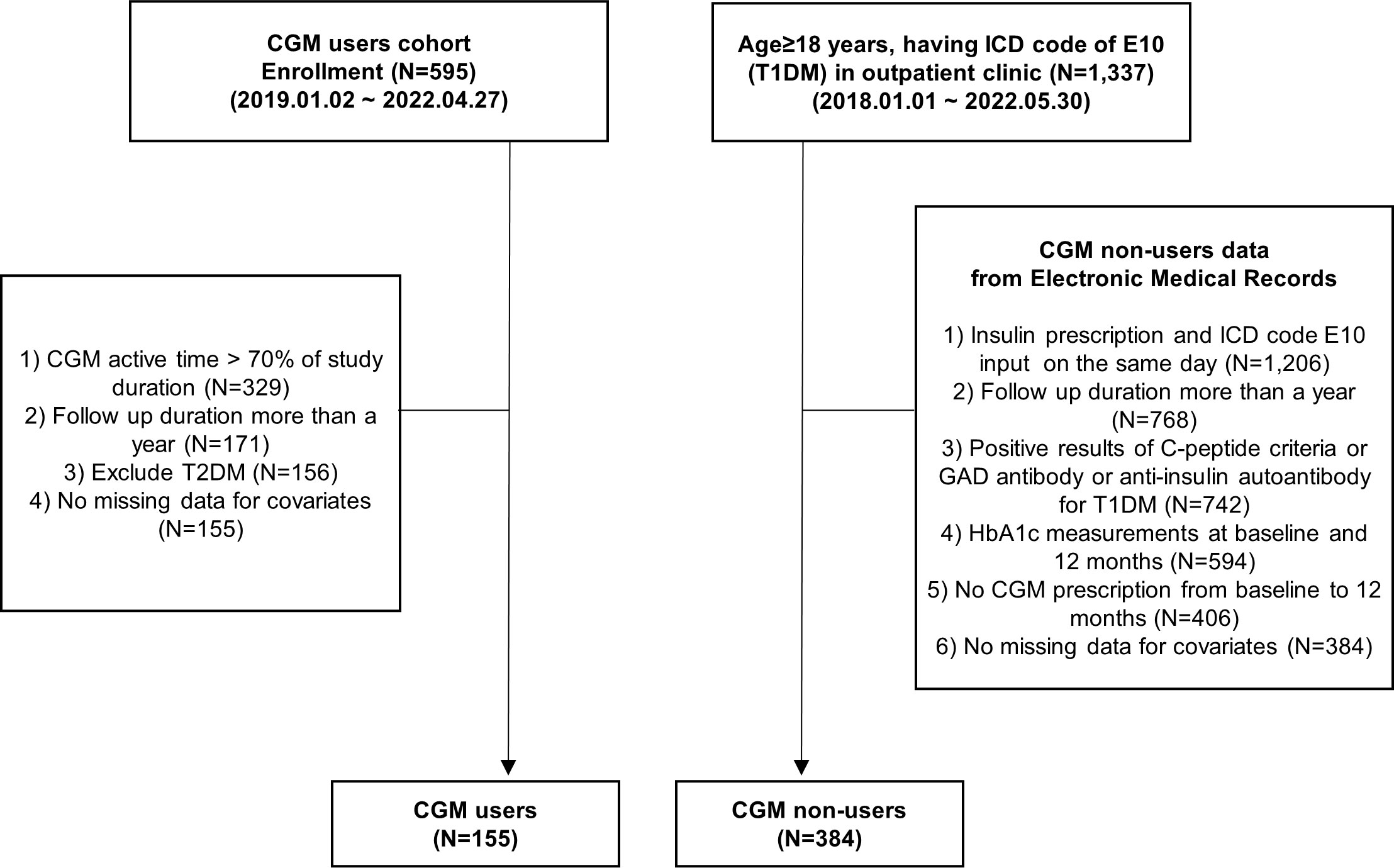
Figure 1 Flowchart. CGM, continuous glucose monitoring; GAD, glutamic acid decarboxylase; HbA1c, hemoglobin A1c; ICD, International Classification of Diseases, T1DM, type 1 diabetes mellitus; T2DM, type 2 diabetes mellitus.
To compare the efficacy of CGM use on glycemic control between CGM users and non-users, data from CGM non-users were also collected retrospectively from the Clinical Data Warehouse (CDW) of SMC electronic medical records (EMR) between January 2018 and May 2022. Among CGM non-users, those who visited the outpatient clinic of the Endocrinology Department and Pediatrics of SMC and those who were 18 years of age or older and who had an E10 ICD code and did not use CGM (N = 1,337) were included. Among them, we included T1DM patients who were at least on insulin prescription for T1DM on the same day as E10 ICD code diagnosis; tested positive for GAD II antibody, anti-insulin autoantibody, or C-peptide criteria (fasting C-peptide <0.6 ng/ml or post-stimulated C-peptide <1.8 ng/ml); and had more than a year of outpatient visits with their HbA1c measured at baseline and 12 months (N = 594).
We excluded people who had a CGM prescription in the follow-up duration of 1 year (N = 188) and missing variables for covariates (N = 22). Finally, we analyzed 155 T1DM CGM users and 384 T1DM CGM non-users, respectively (Figure 1).
Clinical and laboratory measurements
The index dates of the CGM user and non-user groups are the date of first use of CGM and the date when insulin and E10 diagnosis were first prescribed on the same day in the study period, respectively. We collected demographic information of the patients, including age, sex, duration of diabetes, total daily dose of insulin use, anthropology measurements of body weight and height, and laboratory values such as HbA1c, estimated glomerular filtration rate (eGFR), alanine aminotransferase (ALT), aspartate aminotransferase (AST), total cholesterol, high-density lipoprotein (HDL) cholesterol, low-density lipoprotein (LDL) cholesterol, and triglyceride (TG) at baseline. Body mass index (BMI) was calculated as body weight (kg) divided by height (m) squared. Blood samples were collected after an overnight fast. HbA1c level as the main outcome of this study was determined by high-performance liquid chromatography on an HLC-723G8 automated glycohemoglobin analyzer (TOSOH, Yokkaichi, Japan). We classified patients with type 1 diabetes by insulin regimen, such as multiple daily injection with rapid-acting analogs and long-acting analogs, mixpen analogs, and continuous subcutaneous insulin infusion.
CGM parameters
The CGM systems used were real-time Dexcom G5 and G6 (Dexcom, San Diego, CA, USA), Guardian Connect with Enlite Sensor (Medtronic, Northridge, CA, USA), and first-generation FreeStyle Libre System (Abbott Diabetes Care, Witney, UK). We assessed the percentage of time CGM was active during the study period and defined CGM users as those who applied CGM for more than 70% of the study period. The CGM metrics were calculated with percent time in range (TIR) of 70–180 mg/dl, level 2 hyperglycemia of time above range (TAR) >250 mg/dl, level 1 + 2 hyperglycemia of TAR >180 mg/dl, level 1 + 2 hypoglycemia of time below range (TBR) <70 mg/dl, and level 2 hypoglycemia of TBR <54 mg/dl (11). Also, the glucose management indicator (GMI), mean glucose, and glycemic variability such as the coefficient of variation (CV) were assessed (12). The CGM metrics were calculated from the previous 90 days at the 3- and 12-month time points, respectively. In the subgroup analyses of CGM metrics, data from 139 patients were analyzed, excluding individuals who switched type of CGM or changed accounts (N = 2) or did not have CGM data for two continuous weeks at 3 and 12 months, respectively (N = 14).
Statistical analysis
Propensity score (PS) matching used to adjust for measured confounding factors was conducted at a 1:2 ratio by using a nearest-neighbor algorithm, and age, sex, and baseline HbA1c were included as covariates. The comparability of covariates between CGM users and non-users was evaluated by standardized mean difference (SMD). An SMD value below 0.1 means the covariates are balanced between groups (13). We calculated the mean and standard deviation of the changes in the HbA1c value of each subsequent observation from the baseline value, and we used a paired t-test to determine if the changes were statistically significant. The significance of the difference in the changes in HbA1c levels between the CGM users and non-users was also examined using a two-sample t-test.
We used repeated-measures analysis in a linear mixed model (LMM) for longitudinal data, which allows the inclusion of all available data and deals with the missing data. The model showed random intercepts and random slopes with adjustment for confounding factors to identify the quantitative reduction in HbA1c between CGM users and non-users (14). To account for possible baseline confounding factors, we fitted three models with varying degrees of adjustment. Model 1 was a crude model. Model 2 was adjusted for age, sex, baseline HbA1c, and BMI. According to a previous study identifying the significant association between HbA1c and lipid levels, model 3 further was adjusted for the continuous value of eGFR, total cholesterol, HDL cholesterol, LDL cholesterol, TG, duration of diabetes, total daily dose of insulin use, and insulin regimen (15). Multiple linear regression analysis was conducted to identify the predictive marker for a better reduction in HbA1c from baseline to 12 months in baseline variables among individuals with CGM use (N = 155). In the sensitivity analysis, we retrospectively included all CGM users with T1DM during the same study period. We collected all variables mentioned above, as well as follow-up HbA1c values at baseline and 3, 6, 9, and 12 months. We also fitted the adjusted LMM to explore the association between the use of CGM and HbA1c. In the analysis for metrics of CGM, continuous variables were presented as mean ± SD or numbers (%), and categorical variables were expressed as ratios of percentages. Continuous variables were compared using paired t-test and χ2 test between 3 and 12 months. Comparisons of CGM metrics measured from 3 to 12 months were calculated using the linear mixed-effect analysis for continuous variables and the Cochran–Armitage trend test for categorical variables. P-values <0.05 were considered statistically significant, and analyses were performed using SPSS software ver. 27.0 (IBM, Armonk, NY, USA) and R Studio version 1.4.1103 software.
Results
Baseline characteristics of the study population
The baseline characteristics of the study population are presented in Table 1. Before PS matching, 155 and 384 patients were classified as CGM users and non-users, respectively. After 1:2 PS matching, 155 CGM users and 310 CGM non-users were finally analyzed. The SMD of matching variables including age, sex, and baseline HbA1c was below 0.1 and comparable between the two groups. The mean age of the study population was 43.4 ± 15.7 years, and 46% were men. The mean duration of diabetes and the total daily dose of insulin of the participants were 12.8 ± 9.3 years and 43.6 ± 24.2 units, respectively. The mean baseline BMI was 23 ± 3.5 kg/m2, and the mean baseline HbA1c level was 7.5% ± 1.2% in both groups.
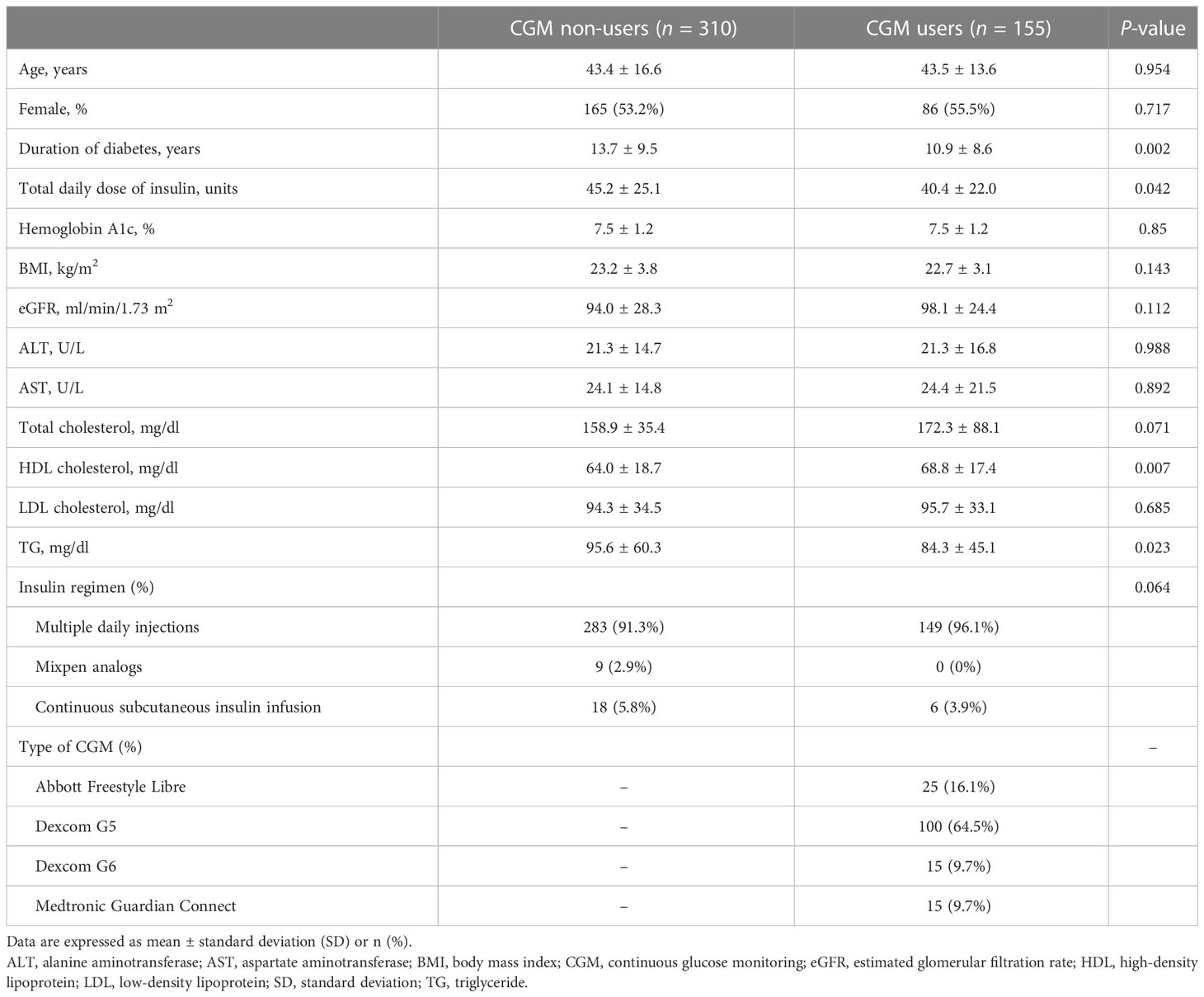
Table 1 Baseline characteristics of the study participants after propensity score matching (n = 465).
Changes in HbA1c
Changes in HbA1c from baseline to each follow-up in CGM users and non-users are described in Figure 2 and Table 2. The changes in HbA1c from baseline to 12 months were −0.5% ± 1.0% for the CGM user group (P < 0.001) and −0.01% ± 1.0% for the CGM non-user group (P = 0.816) with significant difference between the two groups (P = 0.003). The levels of HbA1c were significantly decreased at 3 months compared with baseline by −0.4% ± 0.8% in the CGM user group (P < 0.001) with significant between-group differences (P < 0.001). In all follow-up study periods, CGM users showed significant improvement in HbA1c levels from baseline and the changes differed significantly between the two groups.
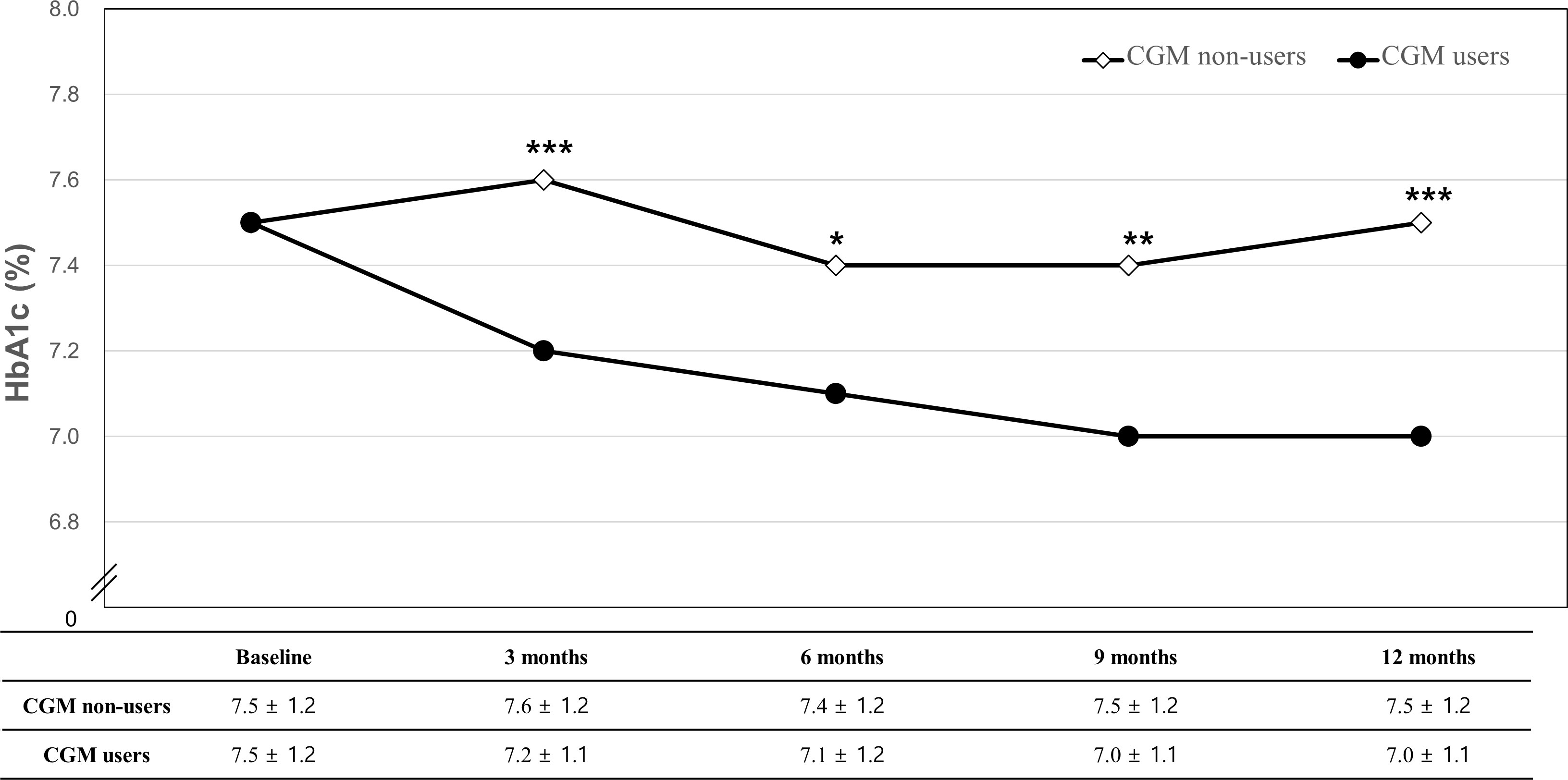
Figure 2 Changes in HbA1c from baseline to 12 months. *P-value for the mean difference between CGM non-users and users (*P < 0.05, **P < 0.01, ***P < 0.001).
The average difference in decreasing HbA1c each three months in patients with and without CGM at baseline
The LMM showed that the overall average values in decreasing HbA1c each three months in patients with and without CGM were −0.01% [95% confidence interval (95% CI), −0.04 to 0.02; P = 0.61] and −0.12% (95% CI, −0.16 to −0.08; P < 0.001), respectively (Table 3). The treatment group difference in mean reduction in HbA1c each three months was −0.11% (95% CI, −0.16 to −0.06; P < 0.001) after adjustment for age, sex, baseline HbA1c, BMI, eGFR, total cholesterol, HDL cholesterol, LDL cholesterol, TG, and insulin regimen (model 3).
Sensitivity analysis
In the sensitivity analysis, the overall average values each three months of reduction in HbA1c in patients with and without CGM were −0.02% (95% CI, −0.05 to 0.004) and −0.12% (95% CI, −0.14 to −0.09), respectively (Supplementary Table 1). The adjusted treatment group difference in mean reduction in HbA1c each three months in model 3 was −0.09% (95% CI, −0.13 to −0.05).
Analysis of baseline characteristics associated with better HbA1c reduction by CGM use
To identify the predictive marker of CGM use on HbA1c reduction from baseline to 12 months, multiple linear regression was conducted in individuals with CGM use (Supplementary Table 2). After adjustment for age, sex, BMI, eGFR, total cholesterol, HDL, LDL, TG, duration of diabetes, total daily dose of insulin, and insulin regimen, higher baseline HbA1c was a predictive marker for a better HbA1c reduction glycemic control following CGM use [β (95% CI) = −0.433 (−0.545, −0.321), P < 0.001].
Analysis of CGM-derived metrics
In the analysis of CGM-derived metrics, CGM users (N = 139) having ambulatory glucose profile (AGP) data at 3 and 12 months were included. Table 4 shows the difference in CGM-derived metrics at 3 and 12 months and the proportions of participants who achieved the CGM metrics goals (TIR > 70%, TBR < 70 mg/dl < 4%, TBR < 54 mg/dl < 1%, TAR > 180 mg/dl < 25%, TAR > 250 mg/dl < 5%, CV ≤ 36%, GMI ≤ 7%) in accordance with the international consensus recommendations.
The variables of TIR, TAR >180 mg/dl, TAR >250 mg/dl, and CV at 12 months showed significant improvements compared with the first 3 months (P = 0.002, P = 0.021, P = 0.032, and P = 0.014, respectively). The proportions of individuals with TIR >70% at 3 and 12 months were 37.4% and 48.2%, respectively (P < 0.001). The proportions of individuals who achieved target goals of TBR <54 mg/dl, TAR >180 mg/dl, TAR >250 mg/dl, CV, and GMI values at 12 months were significantly increased compared with the first 3 months (P < 0.001).
Discussion
In this real-world propensity-matched cohort comparison study with T1DM patients under routine clinical practice, CGM use over 1 year showed a significant reduction in HbA1c level of 0.5% from baseline compared with PS-matched CGM non-users with a significant difference between the two groups (P = 0.003). In addition, a longitudinal LMM showed that the main effect of CGM on HbA1c change was significant in CGM users compared with non-users after adjusting for multivariable confounders. Furthermore, in the analysis of CGM-derived metrics in the CGM user group, we demonstrated a significant improvement in TIR (P = 0.002) in the last 3 at 12 months compared with the first 3 months, and the proportion of those who achieved target goals of TIR >70%, TBR <54 mg/dl <1%, TAR >180 mg/dl <25%, TAR >250 mg/dl <5%, CV ≤36%, and GMI ≤7% was higher over the last 3 and 12 months than the first 3 months (all P < 0.001).
These results are in line with previous studies (5, 16–18). A previous randomized clinical trial (RCT) from the United States reported that the use of CGM (N = 105) compared with usual care (N = 53) resulted in a greater decrease in HbA1c levels over the course of 24 weeks in T1DM patients who required multiple daily insulin injections (5). The adjusted treatment group difference in mean change in HbA1c level from baseline was −0.6% (95% CI, −0.8% to −0.3%; P < 0.001). Also, one meta-analysis of RCTs comparing CGM to self-monitoring of blood glucose (SMBG) in patients with T1DM reported a significantly lower HbA1c at the endpoint with a duration of at least 12 weeks (−0.2%, 95% CI, −0.3% to −0.1%) (17). Recently, one retrospective study reported a statistical decrease in HbA1c using real-time CGM compared with non-initiators (19). This trial of real-time CGM initiators (N = 3,806) vs. non-initiators (N = 37,947) who received care in Northern California (2014–2019) for insulin-treated diabetes reported a decrease in mean HbA1c from 8.2% to 7.8% in the CGM group and 8.3% to 8.2% in the control group over 12 months (difference-in-differences estimate, −0.4%; 95% CI, −0.5% to −0.3%; P < 0.001). Also, hypoglycemia rates increased among non-initiators from 1.9% to 2.3% while falling in CGM initiators from 5.1% to 3.0% (difference-in-differences estimate, −2.7%; 95% CI, −4.4% to −1.1%; P = 0.001). In our study, we prospectively enrolled Asians, particularly Korean T1DM patients who were CGM users, and used strict inclusion criteria of T1DM using not only diagnostic codes but also C-peptide levels, autoantibodies, and consistent insulin use. We further conducted a LMM analysis to show that the effect of CGM use on HbA1c change was independently significant in CGM users compared with non-users after adjusting for multivariable confounders.
In terms of response to treatment, HbA1c changes were evaluated, and a greater reduction in HbA1c in patients with higher HbA1c at baseline has been revealed in a previous meta-analysis (20). The weighted R2 value assessing the association between baseline HbA1c and absolute change in HbA1c was 0.485 (P < 0.001). As the American Diabetes Association recommends a general goal for glycemic control of HbA1c <7% for T1DM patients, it is especially notable that the HbA1c level at baseline was 7.5% in our study, which is closer to the target level. Moreover, we also found that among CGM users, the proportions of individuals with TIR >70% at 3 and 12 months were 37.4% (52 of 139) and 48.2% (67 of 139), respectively (P < 0.001). The correlation between TIR and chronic complications in T1DM was suggested by several studies including the Diabetes Control and Complications Trial (DCCT), as well as between TIR and HbA1c (21–23). One study using the DCCT dataset evaluated the association of TIR from the seven-point glycemic profiles with the development of DM complications (24). For every 10% lowering of TIR, the hazard rate of development of retinopathy progression was increased by 64% (95% CI, 51% to 78%), and the rate of microalbuminuria development was increased by 40% (95% CI, 25% to 56%). Yapanis and colleagues analyzed 34 publications, mostly cross-sectional studies, including a total of 20,852 participants, and presented the association between CGM-derived measures and diabetes-related complications (25). Higher TIR was related to a low risk of retinopathy, albuminuria, and abnormal carotid intima–media thickness. Also, glycemic variability such as standard deviation of blood glucose levels (SD) and mean amplitude of glycemic excursions (MAGE) was associated with peripheral neuropathy. Our study found that the CV at 12 months (34.9% ± 6.1%) showed a significant decrease compared with that at the first 3 months (35.8% ± 5.9%) (P = 0.014). These data also support that glycemic variability is lowered when using a personal CGM in a longitudinal real-world setting (26, 27). In this line, using a personal CGM is associated with a better HbA1c level and CGM-derived metrics of glycemic variability, and further longitudinal studies are required to confirm its relationship with diabetes-related complications in patients with T1DM.
In previous randomized trials of adults with T1DM, CGM use reduced the number of hypoglycemic events (28–30). The SILVER study supported the beneficial long-term effects of CGM on HbA1c and hypoglycemia in people with T1DM. In this RCT, 107 patients with T1DM continued to use CGM over 1 year, and the HbA1c showed a decrease of 0.4% (95% CI, 0.2% to 0.5%). The time spent in hypoglycemia (<54 and <72 mg/dl) decreased from 2.1% to 0.6% (P < 0.001) and from 5.4% to 2.9% (P < 0.001), respectively (31). Because lowering HbA1c may be associated with an increased risk of hypoglycemia in T1DM, it is important to lower the average blood glucose levels while minimizing hypoglycemia. This was demonstrated in our real-world study by improvements in HbA1c, TIR, and TBR when using CGM over 1 year. Although not all CGM-derived metrics improved statistically, after the use of CGM for 1 year, there was a significant increase in those who reached target goals of the CGM core metrics such as TIR, TBR, TAR, CV, and GMI according to the 2019 consensus statement (32).
The strength of this study is its prospective analysis of CGM use in an observational study in a real-world setting to determine if CGM use promotes the reduction of HbA1c level in T1DM patients. Also, with follow-up of CGM users, we continuously collected CGM-derived metrics data over 1 year in a clinical setting. Also, a LMM was used to consider missing data and examine the trend after adjustment for multivariable confounders. Furthermore, sensitivity analysis was performed to identify the robustness of our findings for the longitudinal effectiveness of CGM use on lowering HbA1c in comparison with those prospectively enrolled. This analysis included all T1DM patients who used CGM based on a retrospective review of EMR. We also further investigated a predictive marker of CGM use on HbA1c reduction from baseline to 12 months in patients with CGM use and found that patients with higher baseline HbA1c had a greater HbA1c reduction after 12 months of CGM use.
However, there are some limitations to this study. First, this was based on Korean individuals at a single center, which may limit the ability to generalize our results to other settings. Second, CGM users may be a group of motivated individuals and that may cause a selection bias, and applying CGM itself may have affected the behavioral change of the patients and lifestyle modification.
Also, CGM users were enrolled prospectively and may have influenced the results, whereas the non-CGM users were reviewed retrospectively. Third, the different types of CGM may have different results in the CGM metrics. Further long-term follow-up trials on the use of CGM in patients with T1DM and analyses of real-world data are needed.
Conclusions
In conclusion, our longitudinal study of real-world data from T1DM patients over 12 months demonstrated significant improvements in HbA1c among CGM users compared with non-users.
Data availability statement
The original contributions presented in the study are included in the article/Supplementary Materials, further inquiries can be directed to the corresponding authors.
Ethics statement
The studies involving human participants were reviewed and approved by the Institutional Review Board (IRB) of Samsung Medical Center (approval no. SMC 2018-17-132, 2022-07-110). All patients who were classified as CGM users provided informed, signed consent to participate in this study. An informed consent exemption for non-CGM users was granted by the IRB because all data provided by the CDW of SMC to researchers were de-identified. Written informed consent for participation was not required for this study in accordance with the national legislation and the institutional requirements.
Author contributions
JK conceived this study. GK and JK contributed to the design of the study. SC and SK conducted the data collection and analysis. SC and SK interpreted the results and wrote the main manuscript text. Y-BL, S-MJ, KH, GK and JK edited the manuscript and contributed to the discussion. All authors contributed to the article and approved the submitted version.
Conflict of interest
The authors declare that the research was conducted in the absence of any commercial or financial relationships that could be construed as a potential conflict of interest.
Publisher’s note
All claims expressed in this article are solely those of the authors and do not necessarily represent those of their affiliated organizations, or those of the publisher, the editors and the reviewers. Any product that may be evaluated in this article, or claim that may be made by its manufacturer, is not guaranteed or endorsed by the publisher.
Supplementary material
The Supplementary Material for this article can be found online at: https://www.frontiersin.org/articles/10.3389/fendo.2023.1165471/full#supplementary-material
Supplementary Figure 1 | Flow chart of sensitivity analysis. CGM, continuous glucose monitoring; GAD, glutamic acid decarboxylase; HbA1c, glycated hemoglobin; ICD, International Classification of Diseases; T1DM, Type 1 diabetes mellitus; T2DM, Type 2 diabetes mellitus.
Abbreviations
AGP, ambulatory glucose profile; ALT, alanine aminotransferase; AST, aspartate aminotransferase; BMI, body mass index; CDW, clinical data warehouse; CGM, continuous glucose monitoring; CI, confidence interval; CV, coefficient of variation; DCCT, Diabetes Control and Complications Trial; DM, diabetes mellitus; eGFR, estimated glomerular filtration rate; EMR, electronic medical records; GAD, glutamic acid decarboxylase; GMI, glucose management indicator; HbA1c, hemoglobin A1c; HDL, high-density lipoprotein; ICD-10, International Classification of Diseases 10th revision; IRB, Institutional Review Board; IU, international unit; LDL, low-density lipoprotein; LMM, linear mixed model; MAGE, mean amplitude of glycemic excursions; PS, propensity score; RCT, randomized clinical trial; SD, standard deviation; SMBG, self-monitoring of blood glucose; SMC, Samsung Medical Center; SMD, standardized mean difference; T1DM, type 1 diabetes mellitus; T2DM, type 2 diabetes mellitus; TAR, time above range; TBR, time below range; TG, triglyceride; TIR, time in range.
References
1. Wright LA, Hirsch IB. Metrics beyond hemoglobin A1C in diabetes management: time in range, hypoglycemia, and other parameters. Diabetes Technol Ther (2017) 19(S2):S16–s26. doi: 10.1089/dia.2017.0029
2. Kilpatrick ES, Rigby AS, Goode K, Atkin SL. Relating mean blood glucose and glucose variability to the risk of multiple episodes of hypoglycaemia in type 1 diabetes. Diabetologia (2007) 50(12):2553–61. doi: 10.1007/s00125-007-0820-z
3. Nathan DM, Cleary PA, Backlund JY, Genuth SM, Lachin JM, Orchard TJ, et al. Intensive diabetes treatment and cardiovascular disease in patients with type 1 diabetes. New Engl J Med (2005) 353(25):2643–53. doi: 10.1056/NEJMoa052187
4. Chehregosha H, Khamseh ME, Malek M, Hosseinpanah F, Ismail-Beigi F. A view beyond HbA1c: role of continuous glucose monitoring. diabetes therapy : research, treatment and education of diabetes and related disorders. Diabetes ther (2019) 10(3):853–63. doi: 10.1007/s13300-019-0619-1
5. Beck RW, Riddlesworth T, Ruedy K, Ahmann A, Bergenstal R, Haller S, et al. Effect of continuous glucose monitoring on glycemic control in adults with type 1 diabetes using insulin injections: the DIAMOND randomized clinical trial. Jama (2017) 317(4):371–8. doi: 10.1001/jama.2016.19975
6. Bolinder J, Antuna R, Geelhoed-Duijvestijn P, Kröger J, Weitgasser R. Novel glucose-sensing technology and hypoglycaemia in type 1 diabetes: a multicentre, non-masked, randomised controlled trial. Lancet (London England) (2016) 388(10057):2254–63. doi: 10.1016/S0140-6736(16)31535-5
7. van Beers CA, DeVries JH, Kleijer SJ, Smits MM, Geelhoed-Duijvestijn PH, Kramer MH, et al. Continuous glucose monitoring for patients with type 1 diabetes and impaired awareness of hypoglycaemia (IN CONTROL): a randomised, open-label, crossover trial. Lancet Diabetes Endocrinol (2016) 4(11):893–902. doi: 10.1016/S2213-8587(16)30193-0
8. Weiss R, Garg SK, Bode BW, Bailey TS, Ahmann AJ, Schultz KA, et al. Hypoglycemia reduction and changes in hemoglobin A1c in the ASPIRE in-home study. Diabetes Technol Ther (2015) 17(8):542–7. doi: 10.1089/dia.2014.0306
9. Holt RIG, DeVries JH, Hess-Fischl A, Hirsch IB, Kirkman MS, Klupa T, et al. The management of type 1 diabetes in adults. a consensus report by the American diabetes association (ADA) and the European association for the study of diabetes (EASD). Diabetes Care (2021) 44(11):2589–625. doi: 10.1007/s00125-021-05568-3
10. Hur KY, Moon MK, Park JS, Kim SK, Lee SH, Yun JS, et al. Clinical practice guidelines for diabetes mellitus of the Korean diabetes association. Diabetes Metab J (2021) 45(4):461–81. doi: 10.4093/dmj.2021.0156
11. Yoo JH, Kim JH. Time in range from continuous glucose monitoring: a novel metric for glycemic control. Diabetes Metab J (2020) 44(6):828–39. doi: 10.4093/dmj.2020.0257
12. Draznin B, Aroda VR, Bakris G, Benson G, Brown FM, Freeman R, et al. 6. glycemic targets: standards of medical care in diabetes-2022. Diabetes Care (2022) 45(Suppl 1):S83–s96. doi: 10.2337/dc22-S006
13. Austin PC. Balance diagnostics for comparing the distribution of baseline covariates between treatment groups in propensity-score matched samples. Stat Med (2009) 28(25):3083–107. doi: 10.1002/sim.3697
14. Sinn DH, Kang D, Chang Y, Ryu S, Gu S, Kim H, et al. Non-alcoholic fatty liver disease and progression of coronary artery calcium score: a retrospective cohort study. Gut (2017) 66(2):323–9. doi: 10.1136/gutjnl-2016-311854
15. Borg R, Kuenen JC, Carstensen B, Zheng H, Nathan DM, Heine RJ, et al. HbA1 (c) and mean blood glucose show stronger associations with cardiovascular disease risk factors than do postprandial glycaemia or glucose variability in persons with diabetes: the A1C-derived average glucose (ADAG) study. Diabetologia (2011) 54(1):69–72. doi: 10.1007/s00125-010-1918-2
16. Haviland N, Walsh J, Roberts R, Bailey TS. Update on clinical utility of continuous glucose monitoring in type 1 diabetes. Curr Diabetes Rep (2016) 16(11):115. doi: 10.1007/s11892-016-0808-5
17. Dicembrini I, Cosentino C, Monami M, Mannucci E, Pala L. Effects of real-time continuous glucose monitoring in type 1 diabetes: a meta-analysis of randomized controlled trials. Acta diabetologica (2021) 58(4):401–10. doi: 10.1007/s00592-020-01589-3
18. Maiorino MI, Signoriello S, Maio A, Chiodini P, Bellastella G, Scappaticcio L, et al. Effects of continuous glucose monitoring on metrics of glycemic control in diabetes: a systematic review with meta-analysis of randomized controlled trials. Diabetes Care (2020) 43(5):1146–56. doi: 10.2337/dc19-1459
19. Karter AJ, Parker MM, Moffet HH, Gilliam LK, Dlott R. Association of real-time continuous glucose monitoring with glycemic control and acute metabolic events among patients with insulin-treated diabetes. Jama (2021) 325(22):2273–84. doi: 10.1001/jama.2021.6530
20. Giugliano D, Maiorino M, Bellastella G, Chiodini P, Esposito K. Relationship of baseline HbA1c, HbA1c change and HbA1c target of < 7% with insulin analogues in type 2 diabetes: a meta-analysis of randomised controlled trials. Int J Clin Pract (2011) 65(5):602–12. doi: 10.1111/j.1742-1241.2010.02619.x
21. Beck RW, Bergenstal RM, Cheng P, Kollman C, Carlson AL, Johnson ML, et al. The relationships between time in range, hyperglycemia metrics, and HbA1c. J Diabetes Sci Technol (2019) 13(4):614–26. doi: 10.1177/1932296818822496
22. Hirsch IB, Welsh JB, Calhoun P, Puhr S, Walker TC, Price DA. Associations between HbA(1c) and continuous glucose monitoring-derived glycaemic variables. Diabetic Med (2019) 36(12):1637–42. doi: 10.1111/dme.14065
23. Vigersky RA, McMahon C. The relationship of hemoglobin A1C to time-in-Range in patients with diabetes. Diabetes Technol Ther (2019) 21(2):81–5. doi: 10.1089/dia.2018.0310
24. Beck RW, Bergenstal RM, Riddlesworth TD, Kollman C, Li Z, Brown AS, et al. Validation of time in range as an outcome measure for diabetes clinical trials. Diabetes Care (2019) 42(3):400–5. doi: 10.2337/dc18-1444
25. Yapanis M, James S, Craig ME, O'Neal D, Ekinci EI. Complications of diabetes and metrics of glycemic management derived from continuous glucose monitoring. J Clin Endocrinol Metab (2022) 107(6):e2221–e36. doi: 10.1210/clinem/dgac034
26. Lind M, Polonsky W, Hirsch IB, Heise T, Bolinder J, Dahlqvist S, et al. Continuous glucose monitoring vs conventional therapy for glycemic control in adults with type 1 diabetes treated with multiple daily insulin injections: the GOLD randomized clinical trial. Jama (2017) 317(4):379–87. doi: 10.1001/jama.2016.19976
27. El-Laboudi AH, Godsland IF, Johnston DG, Oliver NS. Measures of glycemic variability in type 1 diabetes and the effect of real-time continuous glucose monitoring. Diabetes Technol Ther (2016) 18(12):806–12. doi: 10.1089/dia.2016.0146
28. Heinemann L, Freckmann G, Ehrmann D, Faber-Heinemann G, Guerra S, Waldenmaier D, et al. Real-time continuous glucose monitoring in adults with type 1 diabetes and impaired hypoglycaemia awareness or severe hypoglycaemia treated with multiple daily insulin injections (HypoDE): a multicentre, randomised controlled trial. Lancet (London England) (2018) 391(10128):1367–77. doi: 10.1016/S0140-6736(18)30297-6
29. Beck RW, Hirsch IB, Laffel L, Tamborlane WV, Bode BW, Buckingham B, et al. The effect of continuous glucose monitoring in well-controlled type 1 diabetes. Diabetes Care (2009) 32(8):1378–83. doi: 10.2337/dc09-0108
30. Battelino T, Phillip M, Bratina N, Nimri R, Oskarsson P, Bolinder J. Effect of continuous glucose monitoring on hypoglycemia in type 1 diabetes. Diabetes Care (2011) 34(4):795–800. doi: 10.2337/dc10-1989
31. Lind M, Ólafsdóttir AF, Hirsch IB, Bolinder J, Dahlqvist S, Pivodic A, et al. Sustained intensive treatment and long-term effects on HbA(1c) reduction (SILVER study) by CGM in people with type 1 diabetes treated with MDI. Diabetes Care (2021) 44(1):141–9. doi: 10.2337/dc20-1468
Keywords: CGM, CGM metrics, T1DM, HbA1c, longitudinal study
Citation: Cho SH, Kim S, Lee Y-B, Jin S-M, Hur KY, Kim G and Kim JH (2023) Impact of continuous glucose monitoring on glycemic control and its derived metrics in type 1 diabetes: a longitudinal study. Front. Endocrinol. 14:1165471. doi: 10.3389/fendo.2023.1165471
Received: 14 February 2023; Accepted: 02 May 2023;
Published: 15 May 2023.
Edited by:
Bert B. Little, University of Louisville, United StatesReviewed by:
Nam Hoon Kim, Korea University, Republic of KoreaJun Hwa Hong, Eulji University, Republic of Korea
Copyright © 2023 Cho, Kim, Lee, Jin, Hur, Kim and Kim. This is an open-access article distributed under the terms of the Creative Commons Attribution License (CC BY). The use, distribution or reproduction in other forums is permitted, provided the original author(s) and the copyright owner(s) are credited and that the original publication in this journal is cited, in accordance with accepted academic practice. No use, distribution or reproduction is permitted which does not comply with these terms.
*Correspondence: Gyuri Kim, gyuri5.kim@samsung.com; Jae Hyeon Kim, jaehyeonmd.kim@samsung.com
†These authors have contributed equally to this work