Why comparing matters – on case comparisons in organic chemistry
- Institute of Chemistry Education, Justus-Liebig-University Giessen, Giessen, Germany
When working with domain-specific representations such as structural molecular representations and reaction mechanisms, learners need to be engaged in multiple cognitive operations, from attending to relevant areas of representations, linking implicit information to structural features, and making meaningful connections between information and reaction processes. For these processes, appropriate instruction, such as a clever task design, becomes a crucial factor for successful learning. Chemistry learning, and especially organic chemistry, merely addressed meaningful task design in classes, often using more reproduction-oriented predict-the-product tasks. In recent years, rethinking task design has become a major focus for instructional design in chemistry education research. Thus, this perspective aims to illustrate the theoretical underpinning of comparing cases from different perspectives, such as the structure-mapping theory, the cognitive load theory, and the variation theory, and outlines, based on the cognitive theory of multimedia learning, how instructors can support their students. Variations of this task design in the chemistry classroom and recommendations for teaching with case comparisons based on current state-of-the-art evidence from research studies in chemistry education research are provided.
1 Introduction
As educators in chemistry, we would unanimously agree that understanding the relationship between the Lewis structure representations of organic molecules and their chemical properties, the molecular architecture, as named by Laszlo (2002), is essential for explaining or predicting chemical behavior. When learning chemistry, students, thus, encounter various ways of representing structures and processes (i.e., electron-pushing formalism) and must connect this to chemical and physical characteristics and energetic considerations (Goodwin, 2010). As a chemical entity has both a visible structural representation and an underlying conceptual aspect, difficulties in linking these two aspects can lead to a superficial understanding. Studies consistently show that students often focus on surface features or patterns when estimating the reactivity of molecules, overlooking functional or more abstract relational similarities (cf. Cooper et al., 2013; Anzovino and Bretz, 2016; Talanquer, 2017). They tend to equate visual similarity with chemical similarity, potentially missing out on understanding how different structural environments can lead to property changes, i.e., changes in chemical reactivity (Bhattacharyya, 2014; Graulich et al., 2019).
One may now ask, why comparing and contrasting should be an important part of learning in chemistry. The act of comparing is inherent to the discipline because it allows us to understand the properties of substances by comparing their behavior in different conditions (Goodwin, 2008). Chemists often compare different substances to identify similarities and differences of chemical and physical properties. In chemical synthesis, making small changes in functional groups at a target catalyst, for example, allows us to determine which ones are most effective at promoting specific chemical reactions (Afagh and Yudin, 2010). By comparing the behavior of chemical systems, chemists can gain a deeper understanding of the underlying principles of chemical processes to monitor and control chemical reactions or refine computational models. Comparing either experimental, machine learning or computational data allows us to estimate the magnitude of effects (Keith et al., 2021). Comparing, for instance, kinetic data of reactions helps determine the magnitude of reaction speed, for instance, influenced by changes of electronic substituent effects (Trabert and Schween, 2018). In some cases, we have this data at hand in terms of empirical properties, such as electronegativity or pKa values, but in other cases, in which we do not have access to these data, chemists often express qualitatively the properties of a functional group or molecule, e.g., this leaving group or nucleophile is good, or this structure is stable (Popova and Bretz, 2018). However, to estimate what “good” means requires answering the question “Good, compared to what” and essentially answering the question “why is it better?.” This is an inherently comparative process that requires knowledge about implicit properties, electron distribution, strength of effects, and energetic considerations. Purposeful case comparisons may engage learners in meaningful sense-making about organic reactions. This assumption is further supported by studies in psychology that have highlighted the educational value of using case comparisons to assist students in grasping new concepts (Schwartz and Bransford, 1998; Gentner et al., 2003). In particular, Gentner et al. (2003) found that comparing two cases simultaneously was more effective for learning than studying five single cases in sequence. By comparing and contrasting different cases, students learn to discern both common and distinctive characteristics that help differentiate and understand key concepts or phenomena. As the instruction continues, such comparisons offer a chance for learners to develop inferences and justifications for the specific features. A meta-analysis by Alfieri et al. (2013) has shown that this method significantly enhances learning. This perspective outlines the theoretical underpinning of case comparisons and highlights how instruction in chemistry can profit from well-designed and orchestrated cases.
2 Why should we learn with case comparisons? Theoretical underpinning
2.1 What does structure mapping theory tell us about comparing?
Learning by comparing cases can be rationalized from a cognitive psychology perspective because it taps into several important cognitive processes, essential for learning and problem-solving. When comparing cases, a learner is engaged in a process called analogical reasoning, which involves finding similarities and differences between cases and using those similarities and differences to make inferences and draw conclusions. This analogical reasoning is a fundamental cognitive process that allows transfer knowledge and skills from one domain to another, or from one context to another (Gick and Holyoak, 1983). The structure mapping theory by Gentner (1989) and Gentner and Markman (1997) explains how this analogical reasoning works. When we compare two situations, objects, or reactions, we look for shared relationships. These relationships could either be similarities in surface features or relational features, such as causal or functional ones. Surface features are always visible features and details of a situation or object and, thus, are easy to discern. While relational structures refer to the abstract relationships between features and implicit information conveyed, they can, but do not necessarily share surface similarities. Comparing a set of correspondences between the surface or relational features of two cases leads to a structural alignment, i.e., discerning the information that two cases share. According to the structure mapping theory, the more shared relational features there are between two situations, the stronger the analogy, the easier to transfer our knowledge about one situation to reason about the other. For example, knowing that an electronegativity difference is needed to make a carbon-heteroatom bond polar, we can use that knowledge to infer that other carbon-heteroatom bonds might be polar as well, when there is a difference in electronegativity, even if the functional group looks different. However, attending to the relational similarity between cases is modulated by expertise. With increasing expertise, we can make use of abstract schemas and use them to categorize tasks based on implicit, conceptual aspects, whereas novice chemistry learners tend to focus on more explicit concrete features (Graulich et al., 2019; Lapierre and Flynn, 2020).
2.2 Cognitive load – the gatekeeper for accessibility
The Cognitive Load Theory (CLT) (Sweller and Chandler, 1994; Kalyuga et al., 1998) offers substantial insights into the use of case comparisons in learning chemistry, emphasizing how instructional design can manage cognitive resources to enhance learning (Paas et al., 2003). The CLT acknowledges the structure or extraneous load of a task (extraneous cognitive load), as well as the cognitive affordances that come with the content (intrinsic cognitive load) and the cognitive effort that a learner needs to activate for learning (germane cognitive load). When we compare cases, we activate our working memory system. However, the use of working memory and the associated capacity is limited, which is why sufficient available capacity must be accessible for effective learning or application of knowledge (Baddeley, 2010). CLT describes that learning is associated with cognitive load and that learning can be simplified or be more challenging depending on the circumstances. Intrinsic cognitive load is related to the difficulty or complexity of the learning material. Sweller (2003) focuses here on element interactivity. In concrete terms, this means that different elements must be processed simultaneously in the working memory during learning. This can happen sequentially, which causes a lower intrinsic cognitive load, or simultaneously, which results in an increased intrinsic cognitive load. If the elements are processed one after the other, e.g., in learning with single cases, this usually leads to memorization; if they are processed simultaneously, e.g., by comparing cases, links are created, which generates understanding but is also more demanding for the working memory (Sweller, 2010). The more prior knowledge learners have, the more links already exist and the lower the intrinsic cognitive load, even when processing elements simultaneously (Paas and Sweller, 2014). Two assumptions support the use of case comparison in light of the intrinsic cognitive load. On the one hand, as our working memory is limited in capacity, comparing cases instead of single cases helps us to be able to attend easily to differences and similarities and neglect other possibly irrelevant features of a situation or object (Schwartz and Bransford, 1998). Simultaneous processing of multiple and maybe irrelevant aspects can be challenging for learners; thus, the extraneous and intrinsic load can be reduced if cases help learners to focus on a reduced number of relevant aspects, as the one variable that needs to be compared can be focused on. This allows us to save capacity in our working memory. Furthermore, studying multiple cases allows learners to see how the same underlying principles apply to different contexts. This can help learners develop a deeper understanding of those principles and how they relate, which makes it easier to build conceptual chunks instead of memorizing single features (Schwartz and Bransford, 1998; Alfieri et al., 2013; Roelle and Berthold, 2015). Studying a single case in isolation may not give learners enough context or variation to understand the underlying principles involved fully (Alfieri et al., 2013). However, using case comparisons does not, per se, remediate mediocre ways of teaching. If the cases are not fully understood and the learner struggles to determine the relevant aspects, comparing cases might increase the intrinsic cognitive load compared to a single case, especially when multiple variables are involved (Schwartz and Bransford, 1998).
In contrast to the intrinsic cognitive load, the extraneous cognitive load is about how learning materials are designed (Sweller, 2010). The more superfluous or irrelevant information learners are presented with, the greater the possibility that they will not be able to distinguish between relevant and irrelevant information and will be distracted, which increases extraneous cognitive load. To minimize extraneous cognitive load for learners, it is therefore advisable to use design principles such as Mayer’s, which are evidence-based and conducive to learning (Mayer, 2021). In relation to case comparisons, this means, for example, that in addition to reducing irrelevant information, the relevant information can be emphasized, e.g., by highlighting techniques (Rodemer et al., 2022).
The germane cognitive load describes the load that relates directly to learning as an activity and is considered productive (Paas and Sweller, 2014). The more a learner can focus on the learning itself, the more effectively links can be created. The germane cognitive load thus relates to the intrinsic cognitive load. Currently, there is an assumption “that germane cognitive load has a redistributive function from extraneous to intrinsic aspects of the task rather than imposing a load in its own right” (Sweller et al., 2019, p. 264). The lower the extraneous cognitive load is kept, the more space is given to the intrinsic cognitive load, which in turn results in an increased germane cognitive load (which is positive). However, this only becomes important with complex learning material, as the intrinsic cognitive load only becomes noticeable here. The simpler a task is, the lower the intrinsic cognitive load and the lower the germane cognitive load (Paas and Sweller, 2014). In relation to case comparisons, this means that the way in which the learning material is designed should be well considered so that there is more space for the germane cognitive load. Complex tasks can be chosen, whereby the complexity must match the prior knowledge and the capacity of the working memory to be able to generate effective learning and links (Sweller, 1994).
Overall, comparing cases as a task design can offload the working memory and engage multiple cognitive processes that are essential for learning and problem-solving when they match the capability of the learners (Roelle and Berthold, 2015).
2.3 Variation theory – instructional design principles
While Cognitive Load Theory (CLT) focuses on the capacity of working memory and how instructional design can be optimized to avoid cognitive overload, Variation theory is a learning theory that emphasizes the importance of variation in the design of instructional materials and activities and places emphasis on the importance of experiencing variations in the learning material to understand and discern the critical aspects of the content. While CLT is more about managing the quantity and complexity of information, Variation Theory is about the quality and structure of learning experiences. According to this theory, learners need to experience variations in the material they are studying in order to fully understand the underlying concepts, i.e., to abstract the relational connections beside surface similarities. Variation theory is based on the work of Swedish researcher Ference Marton and his colleagues, who developed the theory in the 1970s and 1980s (Marton, 1981). Marton (1981) was interested in understanding how students develop their understanding of complex concepts, and he observed that learners often struggle to transfer knowledge from one context to another.
Lo and Marton (2011) proposed that the key to understanding complex concepts is to focus on the variations in the material. They argued that learners need to experience different examples of a concept in order to fully understand it and develop a flexible understanding that can be applied to new contexts, advocating for a deep understanding of the subject matter instead of surface-level memorization.
Variation Theory of Learning helps further to support the use of case comparisons in chemistry education, as it emphasizes the importance of discerning critical features of a concept being taught. Using case comparisons (like different chemical reactions) helps students notice and understand the essential characteristics of each case; for example, contrasting an acid–base reaction with a redox reaction can help students understand the unique features of each type of reaction. Second, Variation Theory suggests that exposure to a range of examples, prototypical and non-prototypical examples, can help students see beyond single examples and support the ability to discriminate between different entities and recognize the significance of these differences. Certain elements become more salient to the viewer through variation, while other elements are kept invariant (Lo and Marton, 2011; Bussey et al., 2013), which allows learners to notice critical features more quickly (Bussey et al., 2013). Using case comparisons helps in achieving this by requiring students to apply principles to different scenarios, thereby promoting a deeper understanding of the underlying concepts (Roelle and Berthold, 2015; Bego et al., 2023). By focusing on these variations, variation theory aims to help learners develop a more nuanced and flexible understanding of the concept they are studying, which can be applied to new situations and contexts. The theory highlights the importance of experiencing variations in the material being studied in order to develop a flexible understanding that can be applied to new situations.
3 How good are students in comparing chemical reactions?
Multiple studies in chemistry education in the last decades documented that students when either not taught or not prompted appropriately to compare meaningfully, show a more surface-level-oriented comparison behavior when categorizing molecules or reactions. Moreover, by comparing two or more structures just because of their similar surface features, learners may overlook their properties (Talanquer, 2008; DeFever et al., 2015). Considering implicit properties and underlying processes of a reaction mechanism is crucial for higher modes of reasoning (Weinrich and Sevian, 2017) and leads to greater success when solving novel mechanistic problems (Grove et al., 2012). Stains and Talanquer (2007, 2008) compared the behaviors of undergraduate and graduate students while engaged in classifying different chemical representations and analyzed how often surface and deep-level attributes were used in the classification tasks. They determined that graduate students used more implicit information from the representations given than explicit ones for their classification. The most common approach used by undergraduates was a single attribute decision-making process. In the domain of organic chemistry, Domin et al. (2008) investigated the behavior of undergraduate students and experts while engaged in categorizing different cyclic or acyclic a-chloro derivatives of aldehydes and ketones. Consistent with Stains and Talanquer’s findings, they found that students primarily categorized these compounds dichotomously by choosing a single surface-level attribute, such as aldehyde/ketone, cyclic/acyclic, or halogenated/non-halogenated. In Stains and Talanquer’s study, experts tended to build similar categories as novices, also focusing on functional groups, but made the decision based on more implicit considerations, such as reactivity of the functional group toward the addition of nucleophiles. This increased focus on functional similarity, i.e., focusing on nucleophilicity/electrophilicity as well as reactivity of reactants, has been as well observed in various studies using card sorting activities (Graulich and Bhattacharyya, 2017; Galloway et al., 2018). It seems as if experts or advanced students in organic chemistry are able to generate more abstract schemas and store implicit information about molecules and reactions in bigger chunks, mirroring chemical reactivity patterns. Regarding investigating the development of expertise, a study revealed that successfully categorizing organic chemistry reaction cards is, with a large effect, correlated with the students’ academic performance (r = 0.62). Moreover, the findings that academic performance is correlated with the successful online categorization were confirmed over the years (Lapierre et al., 2022). In a study from Graulich et al. (2019), learners were prompted to identify, for example, which two out of three nucleophiles would react similarly in a given substitution reaction. Thereby, the explicit properties of the given reactants matched or did match with the correct solutions. The findings revealed that students experienced greater challenges with items in which the structural representations of the correct answer did not share explicit similarity. Therefore, it might be helpful from time to time to use molecules or reactions with similar explicit surface features that are not undergoing similar reaction pathways or reactions that seem to be similar on the surface but undergo different pathways (Graulich and Schween, 2018). This could ideally induce cognitive dissonance in learners and challenge their strong focus on surface similarity. As a result, learners are required to use implicit properties to get to a proper solution and might be open to new explanatory concepts. Moreover, studies revealed that learners experience difficulties in activating the same concept knowledge in different contexts; thus, using a variety of molecules to introduce nucleophilicity might help students not to look only for negative charges and may help learners broaden their concept knowledge (Anzovino and Bretz, 2015; Popova and Bretz, 2018).
4 Designing and orchestrating cases
Case comparisons have been widely used as a task design across natural sciences and mathematics to foster students’ ability to derive implicit features and weigh multiple arguments when reasoning. In their meta-analysis, Alfieri et al. (2013) found that case comparisons led to a higher number of identified variables than single cases (d = 0.60, 95% CI[0.47, 0.72]). Appropriately designed case comparisons offer the possibility to support learners to see how the same underlying principles apply to different chemical systems or to what extent reactions might occur differently (Graulich and Schween, 2018). This offers a chance to foster a deeper understanding of those principles and help students abstract from the explicit and sometimes misleading features of structural representations. Case comparisons seem to be more effective at the beginning rather than the end of an instructional topic, as it can prepare students to be sensitive to important features that need to be properly considered or to key features that must be transferred to new cases (Schwartz and Bransford, 1998; Schwartz et al., 2011).
When learners compare different chemical reactions that involve similar reactants and products but occur under different conditions, learners can experience how changes in conditions can affect the reaction rate and yield and relate this observation to the principles of thermodynamics and kinetics (Pölloth et al., 2022). Moreover, by comparing different cases, learners are forced to consider multiple influential factors and have to evaluate the similarities and differences. This can help them develop their ability to recognize patterns, make connections, and draw conclusions, which are essential skills in scientific inquiry and research (Alfieri et al., 2013). Figure 1 illustrates the differences between tasks based on single cases, contrasting cases with one variable and contrasting cases with two (or more) variables. When comparing a simple single case (Figure 1, upper part), the prompt is often only answered superficially, for example in stating as to whether reactions take place from a thermodynamic point of view. But when another case is added, such as changing the leaving group, this could be considered the simplest format of a case comparison, as only one variable of two displayed reactions is changed (Figure 1, middle part). This requires univariate reasoning and a strong focus on how the leaving group, in this case, the bromide or the chloride ion, is influencing the kinetic outcome of the reaction. Case comparisons can be adapted to more complex ones by changing a second variable, for example, several substituents or positions. The lower part of Figure 1 illustrates a case comparison that requires multivariate reasoning, as not only the leaving group (bromide or chloride-ion) but also the nature of the substrate (e.g., carbonyl vs. double bond) influences the reaction kinetic. Thus, learners have to weigh multiple arguments and justify their decisions based on the strength of implicit properties, in this case, mesomeric and inductive effects (Lieber and Graulich, 2022; Watts et al., 2023).
Case comparisons have been widely used in chemistry education studies, but the way in which these case comparisons were used differed (e.g., Bodé et al., 2019; Lieber and Graulich, 2022; Kranz et al., 2023). Figure 2 illustrates three different possibilities for using contrasting cases in argumentation processes. In the simplest case, an argument is divided into three parts: a claim, evidence and reasoning (evidence and reasoning can be combined as justification) (McNeill and Krajcik, 2012). One possibility for a task design involving case comparisons is that students compare two reactions at the beginning of the task to reason deeply about which reaction will proceed more likely. Thereby, the justification process can take place first and is guided by scaffolding which leads to a claim (Kranz et al., 2023) (see Figure 2, first example). Moreover, after comparing two reaction mechanisms at the beginning, it is also possible that learners first make a claim and justify their claim afterwards (Bodé et al., 2019; Deng and Flynn, 2021) (see Figure 2, second example). Besides comparing reactions at the beginning, it is also possible to build arguments on single reaction products of a reaction but contrast the reaction products at the end of the task. Thereby, students first claim if the respective reaction product is plausible or implausible, which is each justified with evidence and reasoning and compare the plausibilities of the reaction products in the end (see Figure 2, third example). This can lead to a revision of students’ claims of most plausible reaction products toward a correct claim by weighing key concepts when contrasting them (Lieber et al., 2022; Lieber and Graulich, 2022). These studies indicate that the use of case comparison, at the beginning or at the end, has a beneficial effect for building arguments.
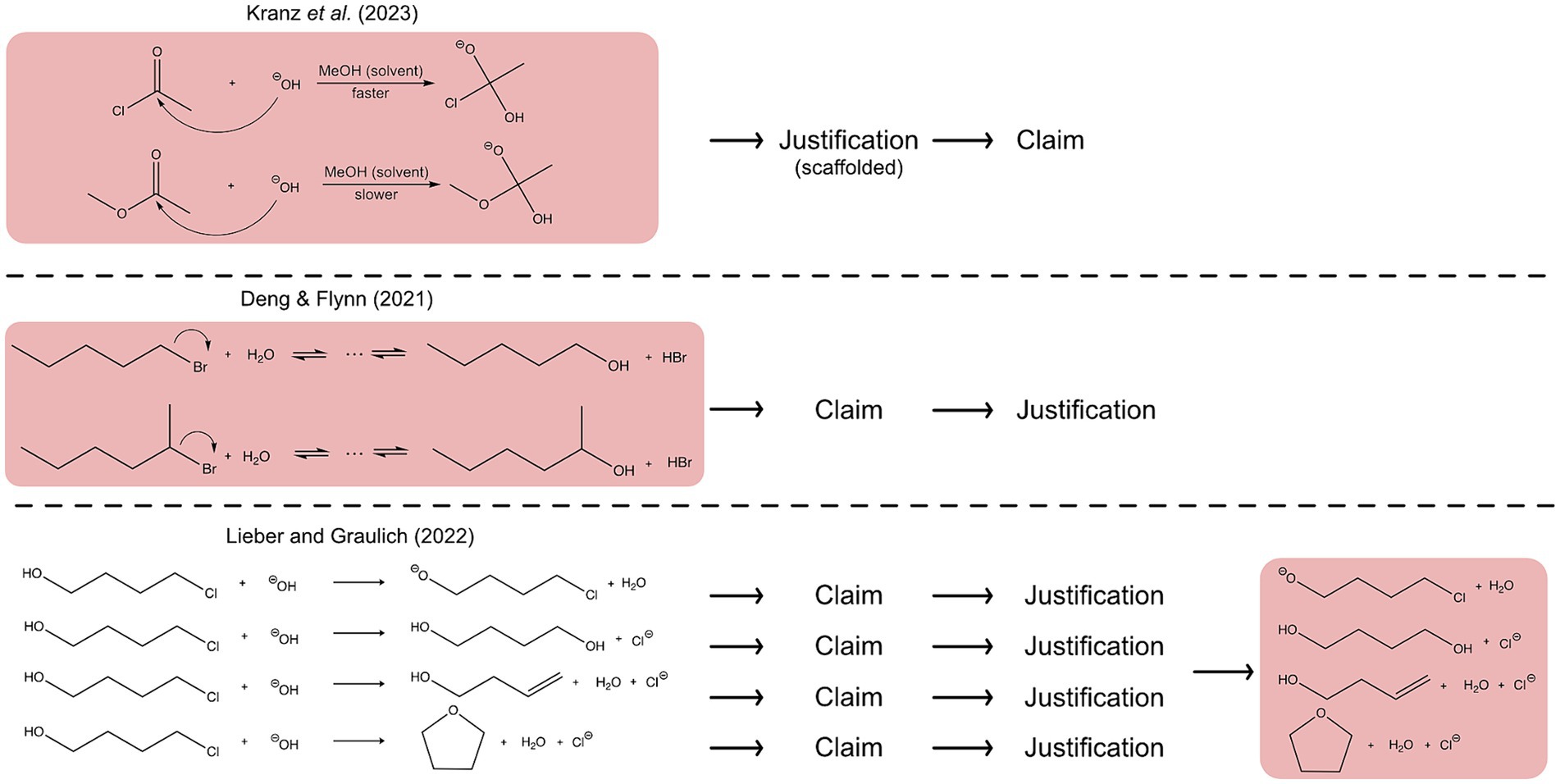
Figure 2. Illustration of different possibilities for the use of case comparisons in argumentation and reasoning processes. The red background highlights when the case comparison is used during the process.
4.1 CPOE cycle – embedding case comparisons in inquiry processes
One way to combine the use of case comparisons with lab work is to embed these case comparisons in the CPOE cycle (Graulich and Schween, 2018), an adapted form of the Predict-Observe-Explain cycle (White and Gunstone, 2014) with an added “Compare” step. The cycle is based on learners first receiving a case comparison where they need to compare two given reactions (C), to predict (P) by generating a hypothesis which of the two reactions, for example, is faster than the other. This hypothesis can then be tested experimentally. By experimentally testing the hypotheses that have arisen from the case comparison, the outcome of the reactions is observed (O). Once the data has been analyzed, the final step takes place, in which conclusions are drawn about the previously formulated hypothesis based on the experimental results (E). Figure 3 illustrates the theoretical CPOE cycle by giving concrete examples how each step can look like, which is described in more detail in the following section.
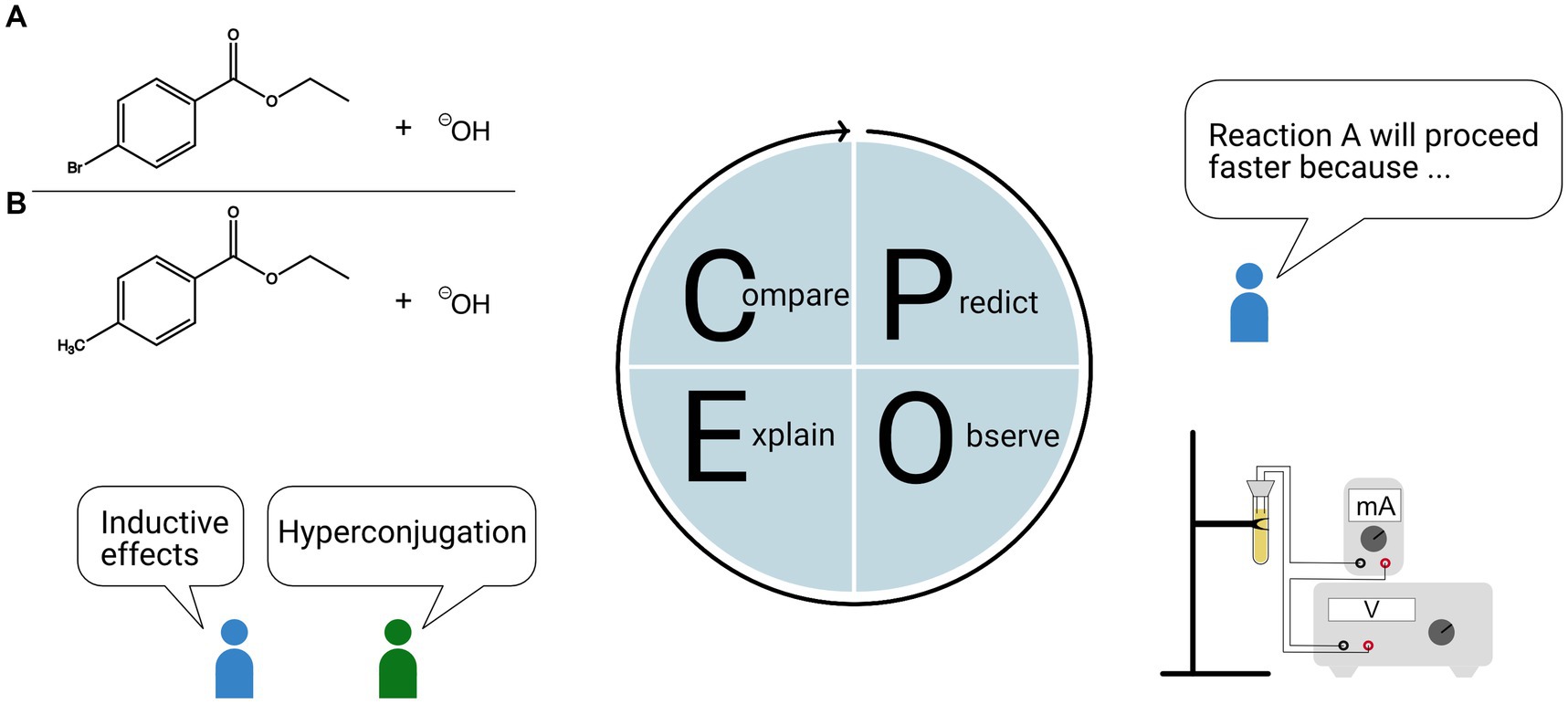
Figure 3. Embedding case comparisons in the CPOE cycle as illustrated with an example from Trabert and Schween (2020).
As the name suggests, however, this may not be a linear process with a defined end but a cycle that can be repeated based on new case comparisons. In this way, learners not only become familiar with scientific principles through independent experience, but the targeted choice of contrasting cases and experiments also enables a specific promotion of chemical concepts.
Schween’s group has developed numerous experiments that make intermediate stages “visible,” for example, based on conductivity measurements (cf. Trabert et al., 2023 for an overview). In each case, two or more reactions are compared with each other and learners are prompted to estimate the reaction with the higher reaction rate. Their work resulted in experimental case comparisons on electrophilic substitution on aromatic compounds, in which the sigma complexes were determined by conductivity measurements (Vorwerk et al., 2015), on the stability of carbenium ions, which makes intermediates directly and indirectly visible through color gradients as well as conductivity measurements (Schmitt et al., 2013), on the competition of primary and secondary haloalkanes in SN2 reactions (Schmitt et al., 2018), as well as on electronic substituent effects in alkaline ester hydrolysis (Trabert and Schween, 2018). All these experiments can be used in a CPOE cycle. Figure 3 illustrates the linkage of Trabert and Schween’s (2020) case comparisons of an alkaline ester hydrolysis, which is focused on inductive effects and their experimental design to the CPOE cycle. Thereby, students first receive contrasting cases of ester hydrolysis, which differ in their substituents on the phenyl group (Figure 3, compare) Based on these two reactions, students have to predict which of the reactions proceed faster including a justification (Figure 3, predict). Students test their hypothesis afterwards in the laboratory with conductivity measurements (Figure 3, observe). Based on their observations, students are encouraged to explain the phenomenon and refer to their hypothesis (Figure 3, explain). When the shown cycle is used in teaching and learning, learners can transfer their knowledge of inductive effects into a second cycle. Therefore, learners can apply their knowledge of inductive effect on new reactions, which focus on the position of substituents. Thereby, learners complete the CPOE cycle a second time by comparing the position of substituents on aromatic compounds, predicting the reaction rate, observing the hypothesis by conducting experiments, and explaining the position dependency of inductive effects. The key aim of these experimental case comparisons is to engage learners in reflection about reaction rate, slowly increasing the sophistication of chemical concepts such as electronic effects that is not only supported by the experimental investigations but can also be advanced to other reactions and contexts. Those cases used in the lab and discussed in lecture might serve as a bridge between these two traditional course formats in organic chemistry.
5 Supporting students to learn meaningfully with case comparisons
When engaged in comparing, meaningful problem-solving requires attending to the relevant features of a representation, as well as linking the necessary implicit information to it (Mason et al., 2019). This may not be an intuitive process for students, as the connection between the feature of a carbonyl group (e.g., C=O) and its electron distribution has to be learned. The first visual selection process when looking at a structure is guided by learners’ perception of saliency, their individual framing of what a given task entails, as well as their prior knowledge and the cognitive resources that a learner is able to activate (Bodé et al., 2019). Just comparing is not a one-size-fits-all solution, especially when implicit or functional information is more important than superficial features and might not result in the intended deeper reasoning about critical features (Bhattacharyya, 2023). For beginners, it might thus be necessary to be supported in attending to the relevant aspects, in order to decrease the extraneous and intrinsic load. The Cognitive Theory of Multimedia Learning (CTML) by Mayer (2021) allows informed instructional design to support students in these aspects. The key assumption of the CTML is that human cognition proceeds by two channels, a visual and a verbal channel, that need to be optimally synchronized in learning. It is thus beneficial to present information both visually, which we typically do with structural representations and verbally (e.g., written or spoken explanations), to engage both channels. Both channels have limited capacity, meaning that learners can only process a limited amount of information at a time. In the context of case comparisons, it is important not to overwhelm students with too much information at once and to guide their attention to the relevant aspect in the visual and verbal channel (Rodemer et al., 2020; Eckhard et al., 2022). Thus, both theories, the CLT as well as the CTML, support the same instructional design principles: guiding students visually and conceptually through a task, to make a task accessible for actual learning.
5.1 Visual attention guidance
Guiding learners to attend to the relevant features, i.e., important functional groups involved in a reaction, can be achieved by multiple means, such as simply signaling or highlighting the relevant areas of the representation [i.e., signaling principle as described by Mayer (2021)], e.g., by zooming in or out, spotlights, coloring, added on-screen text or symbols. Others used experts’ eye gaze as a model for the learner, as used in the context of medicine (Jarodzka et al., 2012; Gegenfurtner et al., 2017), whereas transferring this idea to learning organic reaction mechanisms has not yet been convincing (Graulich et al., 2022). By “signaling” (highlighting key structural features in a static or dynamic fashion) students can focus on these key features of the representation and reduce their attentional focus to the rest of the structure, thus, reducing their extraneous cognitive load, if they are not attending to everything all at once (Richter et al., 2016; Schneider et al., 2018). It can also allow us to model a certain sequence of comparing by highlighting, for example, a starting point of comparison and then the sequential decoding process. Although attending to the relevant features is a key step. Implicit chemical properties cannot be read out of the functional group but need to be linked to it. When the attention of the learner is on the relevant features of a representation, the respective implicit information needs to be added, either in terms of verbal or written information. This is in line with the dual channel assumption of the CTML, providing highlighting for the visual features and chemical information for the verbal channel, as well as presenting it at the same time, i.e., the contiguity principle (Mayer and Fiorella, 2014). Some instructors might intuitively use highlighting techniques by pointing toward the representational features on the blackboard and explaining simultaneously or by adding conceptual information, such as pKa or partial charges on the board. Redirecting a learner’s attention to the relevant aspects, thus, can be complex, as decisions have to be made that cannot just be guided by the salience of a functional group, and conceptual information needs to be linked to make a purposeful selection.
In a quantitative study, we tested if a highlighting technique actually supports students to attend to relevant areas of organic chemistry case comparisons and solve them more successfully. Thus, we created tutorial videos with case comparisons and used a dynamic moving dot highlighting representational features, which was synchronized with the information given as a verbal explanation in parallel (Rodemer et al., 2020; Eckhard et al., 2022). The study could document that all students in the study were profiting from the given verbal explanation, but especially low performing students profited from the highlighting. Following students while watching the videos with highlighting with the help of eye-tracking could show that the attention to relevant areas is focused over the entire time of the video, and the perceived extraneous cognitive load is decreased (Rodemer et al., 2022). These overall results illustrated that beginners need more support in decoding the molecular structures that we use in organic chemistry, and guiding their attention is key for a decreased extraneous cognitive load. Besides using eye-tracking as an analytical lens to track students’ attention, using it in instruction might help students understand their own viewing behavior. In an eye-tracking study conducted by Hansen et al. (2019), they investigated how students view and critique different animations of redox reactions and precipitation reactions. After their reasoning process, students received visual feedback on their own viewing behavior. Hansen et al. (2019) revealed that viewing this feedback helped the students to be critical about their own viewing behavior and to deepen the critique regarding the animations shown.
5.2 Conceptual guidance
Further breaking down the reasoning process with case comparisons into manageable parts can help students process the information more effectively (Belland, 2017). A simple nucleophilic substitution, taught in an introductory organic chemistry course, for instance, requires the consideration of three main influential factors, i.e., leaving group ability, nucleophilicity, substrate effects, and the cause-effect relationships that determine the reactivity in this type of mechanism. Thus, a lot needs to be considered by the learners. Using case comparison can have positive effects on students’ engagement with the conceptual knowledge, as it shifts the focus onto implicit and influential factors of the organic reaction mechanism (Watts et al., 2021). However, if we expect students to reason in a particular way, i.e., building cause-effect relationships, and connect different concepts and properties, we need to be explicit how students should integrate these multiple pieces of knowledge. Developing mastery requires explicit learning of how to create those mechanistic explanations (Cooper, 2015). Thus, supporting students in solving case comparisons should acknowledge the complexity and reasoning steps required and ideally make these steps transparent through a scaffold (Caspari et al., 2018; Kranz et al., 2023). Scaffolding is a known technique widely used as an instruction in science education (cf. Lin et al., 2012; Wilson and Devereux, 2014) and helps students to slow down the decision-making process and gives students the opportunity to activate necessary conceptual and procedural knowledge (Rittle-Johnson and Star, 2007; Rittle-Johnson and Star, 2009; Shemwell et al., 2015; Chin et al., 2016). A scaffold for the case comparisons illustrated therein thus can guide the learner through the different considerations necessary to make a claim about the outcome of a case: (1) describing the chemical changes in the given cases; (2) explicitly stating the overall goal of comparison (task prompt); (3) naming the similarities and differences; (4) stating the role of the influential factors (i.e., implicit properties); (5) explaining and contrasting the influences of the implicit properties; (6) stating how the transition state is affected to refer to the energetic account and (7) making a final claim about the reactivity of both reactions (Bernholt et al., 2023).
Various studies already documented the positive effect of using scaffolding with case comparisons on students’ reasoning. In prior studies, we used a scaffold grid, represented by a worksheet with empty boxes, which visually connects the structural differences, changes, and cause-effect relations (Caspari et al., 2018). By utilizing this grid, students can systematically relate each structural difference to each ongoing change, verbalizing the influence of the structural difference on the change. We compared how students are reasoning through contrasting cases with and without a scaffold and could observe that students’ reasoning is more guided and includes the consideration of more implicit properties and influential effects when solving a contrasting case with a scaffold (Caspari et al., 2018). This structured approach helps students avoid jumping to the final answer without considering the underlying reasons. A mixed-methods study could confirm that especially students with a low prior knowledge profited from working with a scaffold and had a higher learning gain, whereas it does also not harm those with higher prior knowledge (Kranz et al., 2023). Lieber et al. (2022) advanced a scaffold further by acknowledging students’ individual needs when arguing about alternative reaction pathways. Those adaptive scaffolds could show that more individualized instruction when using different cases in organic chemistry might be a new avenue to improve teaching.
6 Conclusion
Comparing the outcome of organic reactions, the strength of nucleophiles, or the reaction rate is at the core of organic chemistry. Through asking comparative questions, we gain insight into reaction processes and reactivity patterns, which allow us to predict and explain novel ones. Learning a collection of seemingly unrelated reactions, or even name reactions in organic chemistry, as often the practice in organic chemistry classes, does not allow learners or make it more difficult to understand and derive the underlying principles that govern reactions. Structure mapping theory tells us, that our cognitive structure is barely made to extract with ease a conceptual similarity just by looking at reactions. An explicit surface similarity will always be more salient for an inexperienced learner. The limited capacity of our working memory additionally affects how much effort we can put into learning and understanding. Purposefully comparing and reasoning through case comparisons can help regain the focus on conceptual understanding in organic chemistry but has not yet been fully explored in instructional design as well as assessments. Multiple studies have documented the potential of using case comparisons compared to more traditional task formats, characterized the type of reasoning that can be elicited from learners, and integrated case comparisons into laboratory experiments. We illustrated therein how, based on various theories of cognition and instruction, comparing can serve as a valuable process for selecting attention, limiting the extraneous cognitive load as well as focusing on implicit and explicit properties and cause-effect relationships. This process of comparing can further be supported, following the principles of the Cognitive Theory of Multimedia Learning, by highlighting relevant features of representations through cueing techniques or providing scaffolding by sequentially guiding students through solving a case comparison. This perspective was meant to consolidate the current state of the art around the use of case comparison to provide instructors with a theory-informed basis for changing their practice and exploring comparing.
Author contributions
NG: Conceptualization, Funding acquisition, Visualization, Writing – original draft, Writing – review & editing. LL: Conceptualization, Visualization, Writing – original draft, Writing – review & editing.
Funding
The author(s) declare financial support was received for the research, authorship, and/or publication of this article. NG would like to thank the German Research Foundation DFG (Deutsche Forschungsgemeinschaft) for funding (project number: 446349713).
Conflict of interest
The authors declare that the research was conducted in the absence of any commercial or financial relationships that could be construed as a potential conflict of interest.
Publisher’s note
All claims expressed in this article are solely those of the authors and do not necessarily represent those of their affiliated organizations, or those of the publisher, the editors and the reviewers. Any product that may be evaluated in this article, or claim that may be made by its manufacturer, is not guaranteed or endorsed by the publisher.
References
Afagh, N. A., and Yudin, A. K. (2010). Chemoselectivity and the curious reactivity preferences of functional groups. Angew. Chem. Int. Ed. 49, 262–310. doi: 10.1002/anie.200901317
Alfieri, L., Nokes-Malach, T. J., and Schunn, C. D. (2013). Learning through case comparisons: a Meta-analytic review. Educ. Psychol. 48, 87–113. doi: 10.1080/00461520.2013.775712
Anzovino, M. E., and Bretz, S. L. (2015). Organic chemistry students' ideas about nucleophiles and electrophiles: the role of charges and mechanisms. Chem. Educ. Res. Pract. 16, 797–810. doi: 10.1039/C5RP00113G
Anzovino, M. E., and Bretz, S. L. (2016). Organic chemistry students' fragmented ideas about the structure and function of nucleophiles and electrophiles: a concept map analysis. Chem. Educ. Res. Pract. 17, 1019–1029. doi: 10.1039/C6RP00111D
Bego, C. R., Chastain, R. J., and DeCaro, M. S. (2023). Designing novel activities before instruction: use of contrasting cases and a rich dataset. Br. J. Educ. Psychol. 93, 299–317. doi: 10.1111/bjep.12555
Belland, B. R., (2017), In instructional scaffolding in STEM education: strategies and efficacy evidence, Cham: Springer International Publishing.
Bernholt, S., Eckhard, J., Rodemer, M., Langner, A., Asmussen, G., and Graulich, N. (2023). In digital learning and teaching in chemistry The Royal Society of Chemistry.
Bhattacharyya, G. (2014). Trials and tribulations: student approaches and difficulties with proposing mechanisms using the electron-pushing formalism. Chem. Educ. Res. Pract. 15, 594–609. doi: 10.1039/C3RP00127J
Bhattacharyya, G. (2023) “Assessment of assessment in organic chemistry - Review and analysis of predominant problem types related to reactions and mechanisms”, in Student reasoning in organic chemistry. eds. N. Graulich and G. Shultz (Cambridge (UK): Roy. Soc. Chemistry), 269–284.
Bodé, N. E., Deng, J. M., and Flynn, A. B. (2019). Getting past the rules and to the WHY: causal mechanistic arguments when judging the plausibility of organic reaction mechanisms. J. Chem. Educ. 96, 1068–1082. doi: 10.1021/acs.jchemed.8b00719
Bussey, T. J., Orgill, M., and Crippen, K. J. (2013). Variation theory: a theory of learning and a useful theoretical framework for chemical education research. Chem. Educ. Res. Pract. 14, 9–22. doi: 10.1039/C2RP20145C
Caspari, I., Kranz, D., and Graulich, N. (2018). Resolving the complexity of organic chemistry students' reasoning through the lens of a mechanistic framework. Chem. Educ. Res. Pract. 19, 1117–1141. doi: 10.1039/C8RP00131F
Chin, D. B., Chi, M., and Schwartz, D. L. (2016). A comparison of two methods of active learning in physics: inventing a general solution versus compare and contrast. Instr. Sci. 44, 177–195. doi: 10.1007/s11251-016-9374-0
Cooper, M. M., Corley, L. M., and Underwood, S. M. (2013). An investigation of college chemistry students' understanding of structure-property relationships. J. Res. Sci. Teach. 50, 699–721. doi: 10.1002/tea.21093
DeFever, R. S., Bruce, H., and Bhattacharyya, G. (2015). Mental Rolodexing: senior chemistry Majors' understanding of chemical and physical properties. J. Chem. Educ. 92, 415–426. doi: 10.1021/ed500360g
Deng, J. M., and Flynn, A. B. (2021). Reasoning, granularity, and comparisons in students’ arguments on two organic chemistry items. Chem. Educ. Res. Pract. 22, 749–771. doi: 10.1039/D0RP00320D
Domin, D. S., Al-Masum, M., and Mensah, J. (2008). Students' categorizations of organic compounds. Chem. Educ. Res. Pract. 9, 114–121. doi: 10.1039/B806226A
Eckhard, J., Rodemer, M., Bernholt, S., and Graulich, N. (2022). What do University students truly learn when watching tutorial videos in organic chemistry? An exploratory study focusing on mechanistic reasoning. J. Chem. Educ. 99, 2231–2244. doi: 10.1021/acs.jchemed.2c00076
Galloway, K. R., Leung, M. W., and Flynn, A. B. (2018). A comparison of how undergraduates, graduate students, and professors organize organic chemistry reactions. J. Chem. Educ. 95, 355–365. doi: 10.1021/acs.jchemed.7b00743
Gegenfurtner, A., Lehtinen, E., Jarodzka, H., and Säljö, R. (2017). Effects of eye movement modeling examples on adaptive expertise in medical image diagnosis. Comput. Educ. 113, 212–225. doi: 10.1016/j.compedu.2017.06.001
Gentner, D., Loewenstein, J., and Thompson, L. (2003). Learning and transfer: a general role for analogical encoding. J. Educ. Psychol. 95, 393–408. doi: 10.1037/0022-0663.95.2.393
Gentner, D., and Markman, A. B. (1997). Structure mapping in analogy and similarity. Am. Psychol. 52, 45–56. doi: 10.1037/0003-066X.52.1.45
Gick, M. L., and Holyoak, K. J. (1983). Schema induction and analogical transfer. Cogn. Psychol. 15, 1–38. doi: 10.1016/0010-0285(83)90002-6
Goodwin, W. (2008). Structural formulas and explanation in organic chemistry. Found. Chem. 10, 117–127. doi: 10.1007/s10698-007-9033-2
Goodwin, W. (2010). How do structural formulas embody the theory of organic chemistry? Br. Soc. Philos. Sci. 61, 621–633. doi: 10.1093/bjps/axp052
Graulich, N., and Bhattacharyya, G. (2017). Investigating students' similarity judgments in organic chemistry. Chem. Educ. Res. Pract. 18, 774–784. doi: 10.1039/C7RP00055C
Graulich, N., Hedtrich, S., and Harzenetter, R. (2019). Explicit versus implicit similarity - exploring relational conceptual understanding in organic chemistry. Chem. Educ. Res. Pract. 20, 924–936. doi: 10.1039/C9RP00054B
Graulich, N., Rodemer, M., Eckhard, J., and Bernholt, S., (2022), Eye-Tracking in der Mathematik- und Naturwissenschaftsdidaktik: Forschung und Praxis, Berlin, Heidelberg: Springer Berlin Heidelberg.
Graulich, N., and Schween, M. (2018). Concept-oriented task design: making purposeful case comparisons in organic chemistry. J. Chem. Educ. 95, 376–383. doi: 10.1021/acs.jchemed.7b00672
Grove, N. P., Cooper, M. M., and Cox, E. L. (2012). Does mechanistic thinking improve student success in organic chemistry? J. Chem. Educ. 89, 850–853. doi: 10.1021/ed200394d
Hansen, S., Hu, B., Riedlova, D., Kelly, R., Akaygun, S., and Villalta-Cerdas, A. (2019). Critical consumption of chemistry visuals: eye tracking structured variation and visual feedback of redox and precipitation reactions. Chem. Educ. Res. Pract. 20, 837–850. doi: 10.1039/C9RP00015A
Jarodzka, H., Balslev, T., Holmqvist, K., Nyström, M., Scheiter, K., Gerjets, P., et al. (2012). Conveying clinical reasoning based on visual observation via eye-movement modelling examples. Instr. Sci. 40, 813–827. doi: 10.1007/s11251-012-9218-5
Kalyuga, S., Chandler, P., and Sweller, J. (1998). Levels of expertise and instructional design. Hum. Factors 40, 1–17. doi: 10.1518/001872098779480587
Keith, J. A., Vassilev-Galindo, V., Cheng, B., Chmiela, S., Gastegger, M., Müller, K.-R., et al. (2021). Combining machine learning and computational chemistry for predictive insights into chemical systems. Chem. Rev. 121, 9816–9872. doi: 10.1021/acs.chemrev.1c00107
Kranz, D., Schween, M., and Graulich, N. (2023). Patterns of reasoning - exploring the interplay of students' work with a scaffold and their conceptual knowledge in organic chemistry. Chem. Educ. Res. Pract. 24, 453–477. doi: 10.1039/D2RP00132B
Lapierre, K. R., and Flynn, A. B. (2020). An online categorization task to investigate changes in students' interpretations of organic chemistry reactions. J. Res. Sci. Teach. 57, 87–111. doi: 10.1002/tea.21586
Lapierre, K. R., Streja, N., and Flynn, A. B. (2022). Investigating the role of multiple categorization tasks in a curriculum designed around mechanistic patterns and principles. Chem. Educ. Res. Pract. 23, 545–559. doi: 10.1039/D1RP00267H
Laszlo, P. (2002). Describing reactivity with structural formulas, or when push comes to shove. Chem. Educ. Res. Pract. 3, 113–118. doi: 10.1039/B2RP90009B
Lieber, L., and Graulich, N. (2022). Investigating Students' argumentation when judging the plausibility of alternative reaction pathways in organic chemistry. Chem. Educ. Res. Pract. 23, 38–54. doi: 10.1039/D1RP00145K
Lieber, L., Ibraj, K., Caspari-Gnann, I., and Graulich, N. (2022). Closing the gap of organic chemistry Students' performance with an adaptive scaffold for argumentation patterns. Chem. Educ. Res. Pract. 23, 811–828. doi: 10.1039/D2RP00016D
Lin, T.-C., Hsu, Y.-S., Lin, S.-S., Changlai, M.-L., Yang, K.-Y., and Lai, T.-L. (2012). A review of empirical evidence on scaffolding for science education. Int. J. Sci. Math. Educ. 10, 437–455. doi: 10.1007/s10763-011-9322-z
Lo, M. L., and Marton, F. (2011). Towards a science of the art of teaching: using variation theory as a guiding principle of pedagogical design. Int. J. Lesson Learn. Stud. 1, 7–22. doi: 10.1108/20468251211179678
Marton, F. (1981). Phenomenography—describing conceptions of the world around us. Instr. Sci. 10, 177–200. doi: 10.1007/BF00132516
Mason, B., Rau, M. A., and Nowak, R. (2019). Cognitive task analysis for implicit knowledge about visual representations with similarity learning methods. Cogn. Sci. 43:e12744. doi: 10.1111/cogs.12744
Mayer, R. E., and Fiorella, L., (2014). The Cambridge handbook of multimedia learning, New York: Cambridge University Press.
McNeill, K. L., and Krajcik, J., (2012), Book study facilitator’s guide: Supporting grade 5–8 students in constructing explanations in science: the claim, evidence and reasoning framework for talk and writing, New York: Pearson Allyn & Bacon.
Paas, F., Renkl, A., and Sweller, J. (2003). Cognitive load theory and instructional design: recent developments. Educ. Psychol. 38, 1–4. doi: 10.1207/S15326985EP3801_1
Paas, F., and Sweller, J., (2014). “Implications of Cognitive Load Theory for Multimedia Learning”, in The Cambridge handbook of multimedia learning, 2nd Edition. Ed. R. Mayer (New York: Cambridge University Press), 27–42.
Pölloth, B., Häfner, M., and Schwarzer, S. (2022). At the same time or one after the other?–exploring reaction paths of nucleophilic substitution reactions using historic insights and experiments. Chemkon 29, 77–83. doi: 10.1002/ckon.202100060
Popova, M., and Bretz, S. L. (2018). Organic chemistry Students' understandings of what makes a good leaving group. J. Chem. Educ. 95, 1094–1101. doi: 10.1021/acs.jchemed.8b00198
Richter, J., Scheiter, K., and Eitel, A. (2016). Signaling text-picture relations in multimedia learning: a comprehensive meta-analysis. Educ. Res. Rev. 17, 19–36. doi: 10.1016/j.edurev.2015.12.003
Rittle-Johnson, B., and Star, J. R. (2007). Does comparing solution methods facilitate conceptual and procedural knowledge? An experimental study on learning to solve equations. J. Educ. Psychol. 99, 561–574. doi: 10.1037/0022-0663.99.3.561
Rittle-Johnson, B., and Star, J. R. (2009). Compared with what? The effects of different comparisons on conceptual knowledge and procedural flexibility for equation solving. J. Chem. Educ. 101:529. doi: 10.1037/a0014224
Rodemer, M., Eckhard, J., Graulich, N., and Bernholt, S. (2020). Decoding case comparisons in organic chemistry: eye-tracking Students' visual behavior. J. Chem. Educ. 97, 3530–3539. doi: 10.1021/acs.jchemed.0c00418
Rodemer, M., Lindner, M. A., Eckhard, J., Graulich, N., and Bernholt, S. (2022). Dynamic signals in instructional videos support students to navigate through complex representations: an eye-tracking study. Appl. Cogn. Psychol. 36, 852–863. doi: 10.1002/acp.3973
Roelle, J., and Berthold, K. (2015). Effects of comparing contrasting cases on learning from subsequent explanations. Cogn. Instr. 33, 199–225. doi: 10.1080/07370008.2015.1063636
Schmitt, C., Bender, M., Trabert, A., and Schween, M. (2018). What's the effect of steric hindrance? Experimental comparison of reaction rates of primary and secondary alkyl halides in competing SN2 reactions. Chemkon 25, 231–237. doi: 10.1002/ckon.201800012
Schmitt, C., Wißner, O., and Schween, M. (2013). Carbenium ions as reactive intermediates – an (experimental) access to a deeper understanding of organic reactions. Chemkon 20, 59–65. doi: 10.1002/ckon.201310195
Schneider, S., Beege, M., Nebel, S., and Rey, G. D. (2018). A meta-analysis of how signaling affects learning with media. Educ. Res. Rev. 23, 1–24. doi: 10.1016/j.edurev.2017.11.001
Schwartz, D. L., and Bransford, J. D. (1998). A time for telling. Cogn. Instr. 16, 475–5223. doi: 10.1207/s1532690xci1604_4
Schwartz, D. L., Chase, C. C., Oppezzo, M. A., and Chin, D. B. (2011). Practicing versus inventing with contrasting cases: the effects of telling first on learning and transfer. J. Educ. Psychol. 103, 759–775. doi: 10.1037/a0025140
Shemwell, J. T., Chase, C. C., and Schwartz, D. L. (2015). Seeking the general explanation: a test of inductive activities for learning and transfer. J. Res. Sci. Teach. 52, 58–83. doi: 10.1002/tea.21185
Stains, M., and Talanquer, V. (2007). Classification of chemical substances using particulate representations of matter: an analysis of student thinking. Int. J. Sci. Educ. 29, 643–661. doi: 10.1080/09500690600931129
Stains, M., and Talanquer, V. (2008). Classification of chemical reactions: stages of expertise. J. Res. Sci. Teach. 45, 771–793. doi: 10.1002/tea.20221
Sweller, J. (1994). Cognitive load theory, learning difficulty, and instructional design. Learn. Instr. 4, 295–312. doi: 10.1016/0959-4752(94)90003-5
Sweller, J., (2003), In psychology of learning and motivation: Advances in research and theory, San Diego, USA: Elsevier Science, pp. 215–266.
Sweller, J. (2010). Element interactivity and intrinsic, extraneous, and germane cognitive load. Educ. Psychol. Rev. 22, 123–138. doi: 10.1007/s10648-010-9128-5
Sweller, J., and Chandler, P. (1994). Why some material is difficult to learn. Cogn. Instr. 12, 185–233. doi: 10.1207/s1532690xci1203_1
Sweller, J., van Merriënboer, J. J., and Paas, F. (2019). Cognitive architecture and instructional design: 20 years later. Educ. Psychol. Rev. 31, 261–292. doi: 10.1007/s10648-019-09465-5
Talanquer, V. (2008). Students' predictions about the sensory properties of chemical compounds: additive versus emergent frameworks. Sci. Educ. 92, 96–114. doi: 10.1002/sce.20235
Talanquer, V. (2017). Concept inventories: predicting the wrong answer may boost performance. J. Chem. Educ. 94, 1805–1810. doi: 10.1021/acs.jchemed.7b00427
Trabert, A., Schmitt, C., and Schween, M. (2023). “Building bridges between tasks and flasks - Design of a coherent experiment-supported learning environment for deep reasoning in organic chemistry”, in Student reasoning in organic chemistry. Eds. N. Graulich and G. Shultz (Cambridge (UK): Roy. Soc. Chemistry), 248–266.
Trabert, A., and Schween, M. (2018). How do electronic substituent effects work?-design of a concept-based approach applying inventing with contrasting cases to the example of alkaline ester hydrolysis. Chemkon 25, 334–342. doi: 10.1002/ckon.201800010
Trabert, A., and Schween, M. (2020). How do electronic substituent effects work?–additional contrasting cases for a differentiated inquiry illustrated by the example of alkaline ester hydrolysis. Chemkon 27, 22–33. doi: 10.1002/ckon.201800076
Vorwerk, N., Schmitt, C., and Schween, M. (2015). Understanding electrophilic aromatic substitutions– sigma-complexes as (experimental) key structures. Chemkon 22, 59–68. doi: 10.1002/ckon.201410237
Watts, F. M., Dood, A. J., Shultz, G. V., and Rodriguez, J.-M. G. (2023). Comparing student and generative artificial intelligence Chatbot responses to organic chemistry writing-to-learn assignments. J. Chem. Educ. 100, 3806–3817. doi: 10.1021/acs.jchemed.3c00664
Watts, F. M., Zaimi, I., Kranz, D., Graulich, N., and Shultz, G. V. (2021). Investigating students' reasoning over time for case comparisons of acyl transfer reaction mechanisms. Chem. Educ. Res. Pract. 22, 364–381. doi: 10.1039/D0RP00298D
Weinrich, M. L., and Sevian, H. (2017). Capturing students’ abstraction while solving organic reaction mechanism problems across a semester. Chem. Educ. Res. Pract. 18, 169–190. doi: 10.1039/C6RP00120C
Keywords: case comparisons, chemistry education, support, guidance, instruction
Citation: Graulich N and Lieber L (2024) Why comparing matters – on case comparisons in organic chemistry. Front. Educ. 9:1374793. doi: 10.3389/feduc.2024.1374793
Edited by:
Michael Wentzel, Augsburg University, United StatesReviewed by:
Dušica D. Rodic, University of Novi Sad, SerbiaPatrick Willoughby, Ripon College, United States
Copyright © 2024 Graulich and Lieber. This is an open-access article distributed under the terms of the Creative Commons Attribution License (CC BY). The use, distribution or reproduction in other forums is permitted, provided the original author(s) and the copyright owner(s) are credited and that the original publication in this journal is cited, in accordance with accepted academic practice. No use, distribution or reproduction is permitted which does not comply with these terms.
*Correspondence: Nicole Graulich, Nicole.Graulich@dc.jlug.de