Persistence of Underrepresented Minorities in STEM Fields: Are Summer Bridge Programs Sufficient?
- 1Department of Educational Leadership and Policy Studies, University of Houston, Houston, TX, United States
- 2Department of Biology and Biochemistry, University of Houston, Houston, TX, United States
Disparities in undergraduate STEM degree completion across different racial/ethnic groups have been a topic of increasing national concern. This study investigates the long-term outcomes of a STEM intervention program designed to increase the academic preparation, achievement and persistence of under-represented minority students.In particular, this study examines the extent to which participation in a STEM intervention program can impact the long-term persistence and graduation of first-time in college under-represented minority students. Using discrete-time competing risks analysis, results demonstrated that participants of the intervention program had a lower probability of drop out and higher probability of persisting in a STEM field of study compared to non-participants of the program. Additionally, descriptive results demonstrated that participants of the STEM intervention program had higher rates of graduation in any field compared to non-participants of the program, while program participation was not a significant predictor of six-year graduation. Findings highlight the importance of early academic preparation in Calculus and total credit accumulation to student success outcomes of URM students enrolled in STEM fields. Recommendations from this study focus on early intervention efforts, particularly in the areas of mathematics, that ensure URM students are adequately prepared with the skills needed to succeed in a STEM field of study.
Introduction
For years, disparities in STEM degree completion has been a topic of increasing concern among policy makers, educational leaders, and the scientific community at large. On a global scale, disparities in STEM educational achievement is a persistent and pressing issue, causing a myriad of education reform and intervention efforts to combat achievement gaps (Clark, 2014). Internationally, disparities between student performance in STEM by socio-economic status, gender, and race/ethnicity are demonstrated through several measures such as persistence and graduation rates, drop-out rates, and GPA (Clark, 2014; Heilbronner, 2014). According to the 2012 international PISA assessment, disparities in STEM achievement exist in every country in the world, with larger disparities apparent across socio-economic status (Marginson et al., 2013). In the United States a growing population of minority students and increasing racial disparities in STEM degree attainment have raised much concern over the country’s ability to maintain its prominence in the fields of technology and scientific innovations (Holdern & Lander, 2010; National Academies of Science, Engineering and Medicine, 2019). The failure to build an adequately trained, diverse STEM workforce in the U.S. that mirrors its shifting demographics will undoubtedly have critical implications on the economic and scientific development of the country. (Espinosa, 2011; Foltz et al., 2014).
In the United States under-represented minority (URM) students are defined as students from Black, American Indian, Alaskan Native, Hawaiian Native, Mexican American, or Mainland Puerto Rican backgrounds (Association of American Medical Colleges, 2003). There is a robust body of evidence indicating that URM students are more likely to come from low socio-economic backgrounds and endure financial troubles during college or university (Cullinane and Leewater, 2009; Estrada et al., 2016). For undergraduate students pursuing STEM fields, research studies indicate that under-represented minority students are equally as likely to enter STEM fields as their white peers. However, wide gaps exist in the persistence rates of minority students completing these fields (Eagan et al., 2013; Lane, 2016). Recent data by the National Center of Education Statistics indicate that in the 2018/2019 academic year, 59% of Bachelor degrees in Science, Technology, Engineering, and Mathematics were awarded to white students, while only 15% were awarded to Hispanic students, and 9% to African American students (NCES, 2018). These percentages fail to reflect the growing minority population in the United States, where the Hispanic and Black population make up 18.5 and 13.4% of the nation’s demographic, respectively (U.S. Census Bureau, 2019). Contributing to these wide disparities in educational achievement is the inadequate academic preparation and limited financial resources available to many URM students (Lichtenberger and George-Jackson, 2013).
Several national institutions have embarked on initiatives designed to combat the disparities in STEM educational achievement among URM students. One of these initiatives is STEM intervention programs, that focus on increasing the academic preparation of URM students in their respective fields of study (Carpi et al., 2017; Estrada et al., 2014). Studies indicate that participation in STEM intervention programs can significantly influence persistence among URM students pursuing STEM fields (Jackson and Winfield, 2014; Lee and Harmon, 2013; May and Chubin, 2003). Certain charactecteristics of STEM intervention programs are particularly helpful to increasing persistence among URM student populations, including the focus on enhancing academic and social integration among minority students (Astin and Astin, 1992; Pascarella and Terenzini, 1991; Pascarella et al., 2011). Such features not only increase the chances of minority student persistence in STEM fields, but also aid in the development of their science identity, a critical component to the motivation and persistence of URM students in STEM fields of study (Carlone and Johnson, 2007; Espinosa, 2011).
Literature Review
The following sections illustrates how certain features of STEM intervention programs, particularly those of the program examined in the present study, could collectively contribute to the persistence of URM students in STEM fields. In line with previous studies surrounding STEM intervention program outcomes on URM students, such programs target disadvantaged students from low socio-economic backgrounds without the adequate academic preparation or economic means to successfully pursue a degree in a STEM field (Aulk et al., 2018; Rask, 2010). Prior research posits that, rather than a single defining feature of intervention programs that singularly affect student persistence, it is the contributing effect of several program elements discussed below that collectively promote URM persistence in STEM (Lane, 2016). To that end, it is important to disentangle ascribed (socio-demographic) and attained characteristics through intervention efforts in the discussion of URM persistence in STEM (Hu and Wolniak, 2010).
Factors Influencing Minority STEM Persistence
Student persistence in STEM is affected by several aspects related to their pre-college academic preparation, race and ethnicity (Saw et al., 2018; Shaw and Barbuti, 2010). Importance of rigorous course taking in mathematics and science has a positive impact on STEM degree attainment (Sadler et al., 2014). High school academic preparation, specifically in math, can increase students’ odds in STEM persistence (Adelman, 1999; Aulck et al., 2017; Chen, 2013). For example, Aulck et al. (2017) used maximum likelihood models to examine factors that predict student persistence in STEM. One of their study’s most notable findings was the weight of success in gatekeeper math courses to student persistence in STEM, particularly during the first year of study (Aulck et al., 2017). The significance of math courses as a predictor of success in STEM has been echoed in other studies (e.g. Chen, 2013; Rask, 2010; Sadler et al., 2014; Whalen and Shelley, 2010). In a report for the National Center for Education Statistics (NCES), Chen (2013) examined a nationally representative sample of undergraduate students to uncover factors related to STEM attrition and graduation. He found that students who persisted in STEM are more likely to have taken calculus in their first year of college compared to those who fail to persist or drop out (Chen, 2013).
Furthermore, a student’s precollege academic background plays a vital role in determining their persistence in a STEM field of study (Acton, 2015; Crisp et al., 2009). Acton (2015) conducted a survival analysis model analyzing the factors that influence time to graduation among undergraduate students enrolled in STEM fields. Results of the proportional hazards model indicated that higher math SAT scores increased students’ hazard of persisting in STEM by 0.3%, while taking calculus in high school increased a student’s hazard of graduating by 177.2% (Acton, 2015). Pre-college academic preparation is particularly crucial among disadvantaged URM populations for whom many did not receive the adequate high school training in math or STEM foundational courses that would aptly prepare them for college-level work (Chang et al., 2014; Riegle-Crumb et al., 2019; Lisberg and Woods, 2018). The achievement gaps that are already apparent prior to URM students’ matriculation into college could not only impact their persistence in STEM fields, but also plays a part in negatively impacting their mindset and motivation as they begin their STEM majors (Riegle-Crumb et al., 2019; Lisberg and Woods, 2018). To that effect, one of the key characteristics of STEM intervention programs is academic preparation in mathematics. Summer bridge programs often offer students intensive instruction in foundational math and science courses to give academically under-prepared students a boost prior to beginning their STEM degree (Moreno and Muller, 1999; Duncan and Dick, 2000). Drawing upon Treisman’s (1992) Mathematics’ Workshop Model, the collaborative learning approaches used in mathematics courses are particularly helpful in improving the academic preparation of URM students. In addition, STEM intervention programs continue to strengthen students’ academic skills in math as they move along their degree plan through supplemental instruction, workshops and faculty mentoring (Lee and Harmon, 2013; Estrada et al., 2016).
The Role of Peer and Faculty Mentorship on Minority Student Success in STEM
Literature examining racial persistence gaps in STEM fields underscores the role of peer and faculty mentorship as key factors to URM student success (Holland et al., 2012; Leggon, 2009). For URM student populations, peer and faculty mentorship can help students feel better integrated with their academic community, and help students develop resiliency, coping and time management skills (Mondisa and McComb, 2015). For instance, Hurtado et al. (2007) examined factors that predicted minority student adjustment in STEM through their first year of study using regression analysis. Their study found that informal peer groups can facilitate student’s transition into their STEM field of study, helps promote camaraderie among participants of the program and increases sense of belonging of students as they progress through their majors (Hurtado et al., 2007). Collective findings from prior research studies also point to the importance of faculty mentorship on improving academic performance of minority students in STEM courses, enhancing students’ sense of academic and social integration within their academic community (Kendricks et al., 2013; Wilson et al., 2010). Kendricks et al. (2013) investigated the impact of faculty mentoring conducted as part of the Benjamin Banneker Scholar Program (BBSP), a STEM intervention program designed to increase the retention and academic success of URM students enrolled in STEM fields. Their study found that faculty mentoring was continually the factor with the strongest impact on minority students’ academic performance in STEM. Additionally, findings from student survey data revealed that many faculty mentor roles exceeded the realms of academic assistance in coursework. The faculty mentor, for many minority students, represented a source of social and cultural support, provided guidance during financial and family hardships, and offered valuable internship opportunities and career guidance for students. Considering the integral role of science identity in establishing a foundation for minority student success in STEM, faculty and peer mentoring also represent two crucial components central to fostering minority students’ academic integration and identification with the science community (Carlone and Johnson, 2007; Holland et al., 2012; Kendricks et al., 2013).
Characteristics of STEM Intervention Programs
STEM intervention programs, in recent years, have been used to combat the disparities in STEM degree completion among URM student populations. Research suggests that one of the most meaningful ways in which STEM intervention programs can increase minority student success is through building their science identity (Carlone and Johnson, 2007; Chang et al., 2014; Schultz et al., 2011; Estrada et al., 2018). This aspect of intervention programs is particularly relevant to minority student populations who tend to experience less belonging to their academic communities as compared to white students (Hausmann et al., 2007). Along those lines, STEM intervention programs that focus on building URM students’ sense of identification with being scientists, and promote students’ sense of integration within the scientific community are more likely to have successful outcomes in terms of STEM persistence and graduation (Foltz et al., 2014; Estrada et al., 2018). Program features such as collaborative learning activities, strong faculty mentorship and advising collectively play a role in promoting minority students’ sense of integration with their academic environment, as well as the motivation and confidence to pursue their academic and career goals (Carlone and Johnson, 2007; Ghee et al., 2016).
Evidence shows that STEM intervention programs provide numerous benefits in promoting students’ confidence and communication skills, as well as building student’s skill-set and knowledge to succeed in different STEM career pathways (Barlow and Villarejo, 2004; Ghee et al., 2016). Studies have shown the positive gains associated with participation in STEM intervention programs (Fecheimer et al., 2011; Ghee et al., 2016). For instance, Ghee et al. (2016) examined the aspects of a STEM summer enrichment program that were particularly related to persistence and success in a STEM field of study. Their results demonstrated that mentorship, collaborative study groups, and research preparation activities incorporated in the summer program increased students’ research skills and self-efficacy. Financial aid also represents a major barrier towards degree completion and persistence among minority students. Intervention programs that offer financial aid to students provide relief for students by removing financial pressures, increasing student motivation to persist through their STEM degree (Eagan et al., 2010).
Purpose of the Study
Given the importance of STEM intervention programs to URM persistence in STEM fields of study, and the national imperative to diversify and adequately prepare students for a successful STEM career, this quantitative study examines the extent to which participation in the University of Houston’s SEP Summer Bridge Program has an influence on student persistence and dropout rates among URM student populations. In particular, the study addresses the following research questions:
1) To what extent does participation in an undergraduate STEM intervention program have an association with six-year graduation rates, relative to their non-participating peers?
2) To what extent does participation in an undergraduate STEM intervention program Summer Bridge Program have an association with student dropout and persistence patterns over time, relative to their non-participating peers?
Findings from this study can contribute to the growing body of literature related to the persistence of minority students in STEM fields. More importantly, it can pinpoint specific areas where minority students are more likely to drop out along their educational trajectory. These results, therefore, could be extremely useful to institutional leaders and educational researchers in identifying and providing support to URM students during the time when they need it most. In addition, results from this analysis could provide valuable recommendations for building and improving STEM intervention programs in ways that could better equip URM students for a successful STEM career.
Materials and Methods
Program Components
Summer Bridge Program: Students recruited into the SEP program initially begin a nine-week summer bridge program that focuses on strengthening student knowledge in basic STEM courses. The typical daily schedule lasts from 9 am to 3 pm, and incorporates faculty led instruction, along with peer mentorship and collaborative hands-on problem-solving sessions. Students are given a foundational pre-calculus course during the first two weeks of the program, followed by freshman calculus and chemistry courses. Additionally, students also receive training on time management and building their study skills as part of the program. The focused, hands-on approach implemented throughout the summer bridge program ensures summer bridge students are adequately prepared for their first fall semester in their respective STEM disciples. Participants of the summer bridge program receive financial assistance to cover the cost of program participation.
Scholar Enrichment Program: Summer bridge students transition to become participants in the Scholar Enrichment Program (SEP) at the start of each fall semester. SEP students benefit from several features designed to support student success and persistence in STEM, including scholarship aid, small peer-led learning groups, and collaborative learning groups. The SEP program also promotes network building between students and STEM alumni by hosting several career seminars and social events throughout the academic year. To also strengthen the relationship between students and their SEP community, participants in the summer program are also encouraged and recruited to work as tutors, peer facilitators and mentors as they progress through their degree.
Data Source
This quantitative study examines transcript records from the 2013 and 2014 SEP summer bridge freshman cohort (n = 102), and a matched group of non-SEP freshman students from the same cohort (n = 1,459). The selection of years was made to ensure that the six-year graduation time frame could be captured in our analysis, while also providing a timely and current representation of URM graduation and STEM retention rates across SEP and non-SEP participants. The matched group of students was restricted to students enrolled in STEM fields of study from the three major colleges of Natural Sciences and Mathematics, Technology, or Engineering. Student transcript records used in this analysis offered in-depth insight into the academic progress of students along their STEM educational trajectory. Specifically, data analyzed in this study included a breadth of indicators regarding student demographics, by semester GPA, course taking patterns, and graduation data. Such detailed student information allowed this study to track students across time and analyze patterns of persistence and drop-out among SEP summer bridge participants and their non-SEP counterparts.
Data Analysis
Propensity Score Analysis
To control for selection bias, the first phase of analysis included creating a propensity-score matched sample of students that will match SEP summer bridge students with similar STEM undergraduate students using a selection of baseline covariates (Ghazzawi et al., 2020; Rosenbaum & Rubin, 1983). To that effect, the use of propensity score analysis created treatment and control groups that were statistically similar in baseline characteristics, allowing the effect of program participation to be more accurately captured (Ashford et al., 2016). The first step of the propensity score model included the calculation of a propensity score, which is probability of group assignment (SEP or non-SEP). An examination of areas of common support was conducted to observe the balance of covariates among treatment and control groups. This was followed by a 1:1 nearest neighbor matching method within a predetermined caliper, enabling SEP students to be matched with their non-SEP peers with similar propensity scores. Selection of covariates was informed by research studies linking student socio-demographic and pre-college characteristics to persistence in STEM fields (Hurtado et al., 2007; Murphy et al., 2010; Ashford et al., 2016; Ghazzawi et al., 2020) These covariates included race, gender, age, and standardized math test scores. Descriptions and coding of baseline covariates are presented in Table 1.
A propensity score matched sample of an equal number of SEP summer bridge students, and matched non-SEP students was created using 1:1 nearest neighbor matching within caliper (n = 194). Approximately 34% of the matched sample consisted of black students, 43% Hispanic, 10% White, and 13% Asian. Table 2 presents descriptive statives of baseline covariates across pre and post-matched samples of treatment and control groups. Prior to the matching, the average math SAT score of non-SEP students was higher than those of SEP students. As observed in Table 2, differences in post-matched average math SAT scores across treatment and control groups were reduced. In addition, the matched sample contained a more balanced distribution of students from different ethnicities across treatment and control groups.
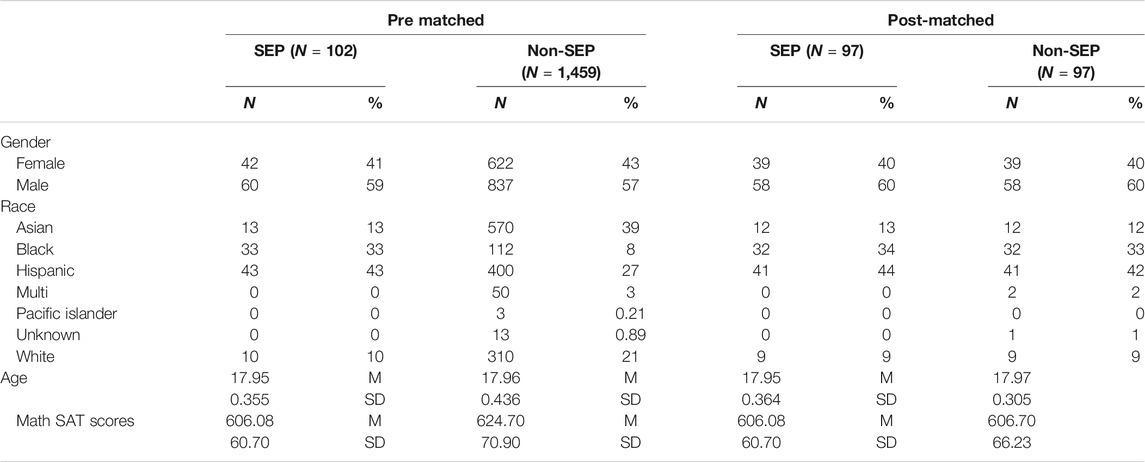
TABLE 2. Descriptive Statistics- Full Sample of SEP Bridge and Non-SEP Bridge Students. By Dina Ghazzawi, Donna Pattison, and Catherine Horn.
Impact of Program Participation on Six-Year Graduation
Following the propensity score matching procedure, a logistic regression analysis was conducted on the resulting matched dataset of SEP and non-SEP students to examine the impact of program participation on six-year graduation rates (in both STEM and non-STEM fields) after controlling for the baseline characteristics of race and pre-college academic performance. Guided by supporting literature on the importance of the Calculus course taking patterns on student graduation rates, a categorical variable indicating the time in which students took Calculus I was incorporated into the analysis as an independent variable (Chen, 2013). In addition, the academic progress variables of final cumulative GPA and total credits accumulated were added into the model to assess their effect on graduation rates.
Discrete-Time Survival Analysis
Following this procedure, the second phase of the analysis involved discrete-time survival analysis. Discrete survival analysis was chosen as an appropriate analytic method due to its unique contributions to the field of education research where events occur in distinct periods of time. In addition, survival analysis examines the time to event occurrence, using predictors that can be both time-varying and time-invariant, lending flexibility and depth to models. Finally, inherent in survival analysis models is the concept that the effect of a predictor on the probability of event occurrence can change over time (Singer and Willet, 1993). Given the unique characteristics that are directly applicable to the research questions in this study, discrete survival analysis was chosen as the most appropriate methodological approach.
Guided by Singer and Willet (2003), the dataset was constructed as a person-period dataset, where each record represented a particular semester in which a student was enrolled in a STEM field of study. Students were in one of two states, the treatment group (TREAT = 1), which included students enrolled in the SEP summer bridge program, and those in the control group (TREAT = 0). Students were tracked from their first entering semester in the program (freshman year) where time = 0. The data was right-censored, meaning that not all students graduated within the time frame captured in the data.
Discrete-time survival analysis was employed in two ways in this study. Firstly, to examine the survival and hazard estimates of persistence in a STEM field across the matched sample of SEP and non-SEP students. Secondly, competing risk discrete-time event history analysis was conducted to examine the differences in the probability of dropping out of a STEM field of study between participants of the SEP summer bridge program and those not enrolled in the program. The use of competing risk analysis was necessary in examining drop out behavior among students given the probability of persistence in a STEM field, as both events are mutually exclusive as students who persist in a STEM field are no longer at risk of dropping out and vice versa. The use of competing risks analysis, rather than traditional logistic regression models, enabled the study to account for timing of student dropout, and was not bound by the statistical assumptions of standard logistic regression models. To that effect, this study utilizes a competing risks model to analyze time to dropout as a function of program participation and academic factors, while simultaneously taking into account the competing risk of persisting in STEM.
Dependent Variables
To examine the impact of program participation on six-year graduation across SEP summer bridge participants and their non-SEP peers, the dependent variable used was dichotomous, coded 0 if a student did not graduate in any field (both STEM and non-STEM) and 1 if a student graduated in a given semester.
To examine the impact of program participation on drop out, with persistence in STEM as a competing event, the dependent variable used was a categorical variable with three values, measuring the probability staying enrolled in university regardless of field, coded as 0; dropping out, coded as 1, and finally persisting in a STEM field of study, coded as 2. Power calculations were conducted to ensure the sufficiency of observations and sample size for discrete-time survival analysis. Results showed that a sample size of 72 yielded 80% power, ensuring that our sample size was adequate for proceeding with the analysis.
Independent Variables
The first stage of the analysis matched SEP students with similar non-SEP students according to baseline characteristics of race, age, and SAT Math scores that reduce the likelihood of bias and enable program participation effects to be adequately captured (Haeger and Fresquez, 2016). Given the literature on the importance of academic progress variables, such as cumulative GPA, Calculus course taking patterns and credit accumulation on the persistence and graduation of students in a STEM field, these variables were included in the logistic regression and competing risks model (Acton, 2015; Chen, 2013; Hurtado et al., 2010). To account for the over-estimation of effects involved with non-standardized continuous variables, the variable of Cumulative GPA was mean centered supported by research studies that suggest using standardizing approaches or categorizing of continuous predictors to overcome the problem of the over-estimation of odds ratios (e.g., Ottenbacher et al., 2004) studies suggest that statistical significance or insignificance remains regardless of the method used (Nick and Campbell, 2007). Summary statistics of Cumulative GPA yielded a mean of 2.82 and standard deviation of 0.81.
Results
Descriptive Statistics
For the 97 students in the control sample of non-SEP summer bridge students, 55 students graduated within the study time period (approximately 57%). For the 97 students in the SEP-summer bridge program, 66 students (68%) graduated within the study time period.
Table 4 presents the total percentage of students graduating in any field, dropping out or persisting in a STEM field of study. Chi-square tests of significance showed no significant differences between the proportion of students graduated, persisting, or dropping out of their respective STEM majors across treatment and control groups.

TABLE 4. Descriptive Statistics: Graduation, STEM Persistence, and Drop-out. By Dina Ghazzawi, Donna Pattison, and Catherine Horn.
Logistic Regression Predicting Six-Year Graduation
Table 5 presents the results of the logistic regression model predicting six-year graduation rates across SEP and non-SEP students. The pseudo-R squared indicated that the variables in the model explained 71% of the variation in graduation. The model indicates that participation in the SEP summer bridge program was not a significant predictor of six-year graduation after controlling for baseline characteristics. On the other hand, taking Calculus I in the first year of university was a significant predictor of graduation in six years. Students who took Calculus I in their first year of college were 1.68 times more likely to graduate in six years compared to students who did not take Calculus I at all.
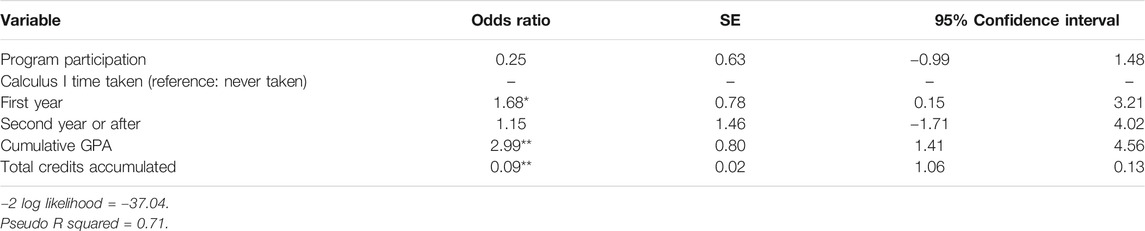
TABLE 5. Logistic Regression Examining Graduation in Six-years. By Dina Ghazzawi, Donna Pattison, and Catherine Horn.
Additionally, after centering, final cumulative GPA was a strong significant predictor of six-year graduation. Students were 2.99 times more likely to graduate with each point increase in final cumulative GPA. Finally, total credits accumulated was also a significant predictor of six-year graduation, raising student odds of graduating by 1.10 times with higher accumulated credits.
Discrete-Time Survival Analysis
Table 6 illustrates the Life Table showing the hazard and survival functions of students persisting in a STEM field of study within the 6-years study period. Table 4 indicates that non-SEP students have a greater hazard or chance of persisting in STEM between their 9th and 10th semester (48.8%), compared to SEP summer bridge students who have a greater chance of persisting in STEM by the end of their study period (between semesters 12 and 13). The chances of students persisting in STEM increased as students remained enrolled in the program, for both SEP and non-SEP students. For instance, between their 6th and 7th semesters of study, the 66 non-SEP students that remained enrolled in their STEM field of study had approximately 1.5% hazard, or chance of persisting. By the end of the study period (12th semester), their chances of persisting increased to approximately 33%. SEP students had approximately a 4% chance of persisting in STEM between their 6th and 7th semesters, which increased to 66.7% by the end of the study period.
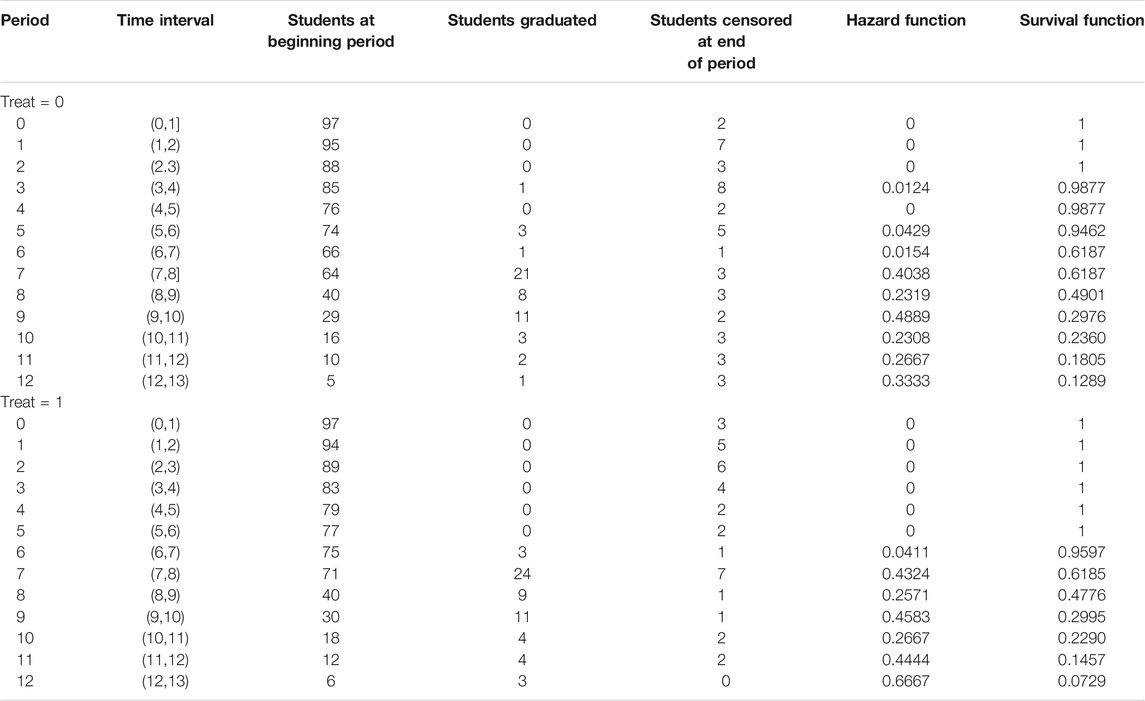
TABLE 6. Life Table Summarizing Time to Graduation in a STEM field across Treatment and Control Groups. By Dina Ghazzawi, Donna Pattison, and Catherine Horn.
Competing Risks Analysis
To examine drop out behavior among students given the probability of persistence in a STEM field, a competing risks discrete-time analysis was conducted as both events are mutually exclusive as students who persist in a STEM field are no longer at risk of dropping out and vice versa. Figure 1 displays the cumulative incidence function indicating the probability of drop-out prior to the end of the study time-frame (12 semesters), across treatment (SEP) and control (Non-SEP groups). The cumulative incidence function indicates that the probability of drop-out among both treatment and control groups is low during the first five semesters of study. At the 10th semester, the probability of drop-out for students in the SEP summer bridge program is 5%, compared to 8% for non-SEP students. The graph also indicates a reduced incidence of drop-out among SEP students compared to non-SEP students due to the reduced risk among SEP students and an increased risk of persistence (the competing event). By the 12th semester of study, non-SEP students had a 12% probability of drop out, whereas SEP students had a 7.5% chance of dropping out prior to the 12th semester. With time, there is an increase in the disparity of cumulative incidence between SEP and non-SEP students.
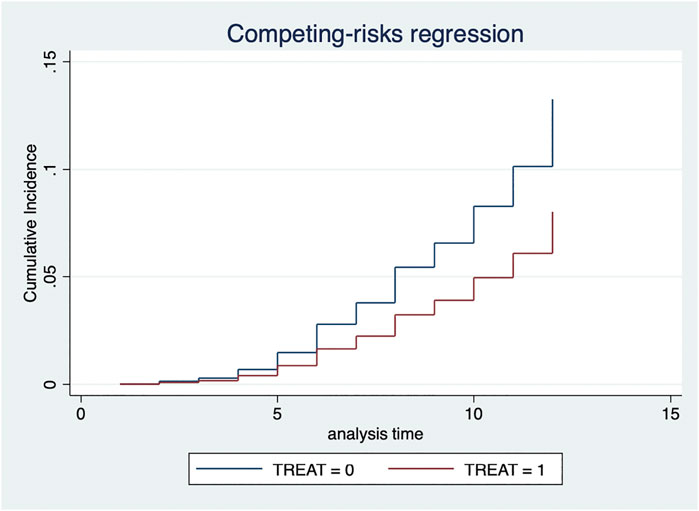
FIGURE 1. Cumulative Incidence Functions Across Treatment and Control Groups. By Dina Ghazzawi, Donna Pattison, and Catherine Horn.
Table 7 presents the results of the competing-risks regression for the cumulative incidence of dropping out during the 12 study semesters of study, using the variables of program participation, time in which Calculus I was taken, cumulative GPA, and total credit hours accumulated. Persistence in a STEM field was treated as a competing event. When taking into account the odds of persisting in a STEM field of study, the results of the competing-risks regression model indicate that SEP summer bridge students are less likely to drop out and more likely to persist in STEM. This is evident from the significant sub-hazard ratios of dropout for SEP summer bridge students that are 54.1% of those ratios for non-SEP summer bridge students (p < 0.05). Additionally, the total number of credits accumulated was associated with a reduced incidence of drop out among students, after controlling for program participation effects. Students with a higher number of accumulated total credits were less likely to drop out and had an increased likelihood to persist in their respective STEM field of study (p < 0.005). Final cumulative GPA and timing of Calculus I course taking were not significantly related to drop-out among students in STEM fields.
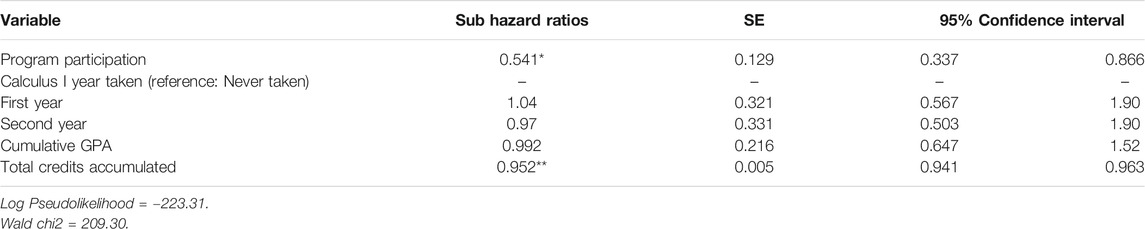
TABLE 7. Competing-Risks Analysis: Program Participation. By Dina Ghazzawi, Donna Pattison, and Catherine Horn.
Discussion
The preliminary results of this study demonstrate the effectiveness of STEM intervention programs at increasing graduation rates among participants. In summary, the results of the study demonstrate that a higher percentage of SEP participants (68%) graduated in any field compared to non-SEP participants (57%). Findings also showed that taking Calculus I in the first year of college, as well as the total number of credits accumulated, were significant predictors of six-year graduation. In addition, descriptive results indicated that a lower drop-out rate and higher persistence rate of SEP summer bridge participants, compared to their non-SEP peers. These findings support previous studies that found higher rates of persistence and graduation among participants of STEM intervention programs (Estrada et al., 2016; Lee and Harmon, 2013). Furthermore, these results provide an unbiased, robust reflection of program effects on student success measures, as propensity score matching accounts for baseline covariates that could influence results (Haeger and Fresquez, 2016).
Results of the logistic regression analysis indicated that, after accounting for pre-college and socio-demographic variables, program participation did not significantly impact six-year graduation. However, results do demonstrate that taking Calculus I in the first year was a strong predictor of six-year graduation. Students who took Calculus I in their first year of college were 5.36 times more likely to graduate compared to those students who never take Calculus. The importance of math, and the timing of course taking in particular, is well-documented in the literature (Chen et al., 2013; Sadler, 2014; Acton, 2015; Aulck et al., 2017). One of the requirements of the SEP summer bridge program is Calculus I in the first year, as well as giving students ample preparation in mathematics during their nine-week summer bridge program. The academic preparation in math provided by the SEP summer bridge program gives greater context to the results of this study. Despite program participation not being a significant predictor of six-year graduation, the strong academic preparation in math that characterizes the SEP summer bridge program is a strong predictor of graduation. This aspect of the results provides evidence of one of the most beneficial features of the program that significantly impacts student success.
Results of the competing risks discrete-time analysis indicated that SEP summer bridge students had a lower cumulative incidence rate of drop-out compared to non-SEP students, and a higher risk of persisting in STEM, supporting prior findings (Ghee et al., 2016; Fechheimer et al., 2011). These findings contribute to a growing body of literature concerning the significant role of STEM intervention programs in reducing drop-out and increasing persistence among URM populations in several ways. Firstly, the robust and flexible implementation of competing risks regression enabled the study to capture the risk of dropout across SEP and non-SEP summer bridge students while simultaneously taking account the risk of persisting in STEM. Secondly, our results indicate that, across time, the disparity in dropout rates between SEP summer bridge and non-SEP students increases. Several studies support the benefits of collaborative learning approaches, particularly through mentorship and course-based practice, on increasing the retention, academic achievement, motivation and engagement of students in STEM fields (Lewis, 2011; Shields et al., 2012; Smith et al., 2014). Furthermore, there is strong evidence that the collaborative approaches used by STEM intervention programs such as SEP are particularly helpful in reducing achievement gaps for low-income URM students, due to the contributing effect of academic support, faculty mentorship, and sense of belonging and community offered through this mode of learning (Eddy and Hogan, 2014; Loui and Robbins, 2008; Reisel et al., 2014). These aspects of academic support are particularly beneficial in promoting URM student persistence in STEM according to collective research students (Carlone and Johnson, 2007; Estrada et al., 2018; Ghee et al., 2016).
The number of total credit hours accumulated was also significantly associated with a lower risk of dropout and a higher risk of persistence, supporting prior studies that indicate the significance of credit hours to student retention in STEM fields (Mau, 2016). The number of total credit hours is also indicative of the time spent in the program of study, demonstrating that as students’ progress through their respective STEM majors they accumulate more credit hours and are at a higher risk of persisting in STEM. Since SEP summer bridge students are at a lower risk of dropping out and persist at higher rates, increasing total credit hours is a crucial component of persistence.
Despite the strong evidence demonstrating achievement gaps across race in STEM degree persistence, few studies address ways to overcome this persistent problem (Mutegi, 2013). Findings from this study present a longitudinal view of the outcomes associated with participation in an intervention programs, contributing to the extant literature by addressing the key elements that could significantly increase URM students’ odds of persisting in their STEM degree. Results support continuous calls for early intervention, particularly in the areas of mathematics, which are in line with previous studies highlighting the integral role of advancing URM students’ academic preparation in math in increasing STEM degree persistence (Chang et al., 2014; Lisberg and Woods, 2018; Riegle-Crumb et al., 2019).
Limitations
Findings from this study are limited to the outcomes of a single STEM intervention from one institution, therefore results may not apply to students from different geographic areas or different types of programs. Furthermore, although discrete-time survival analysis, as well as competing risks analysis, is a robust methodological technique for tracking students’ educational trajectories, it does not take into account students who may have dropped out and another university.
Conclusion
Recommendations from this study underscore the importance of early STEM intervention practices, particularly academic preparation in the areas of mathematics, that enable minority student populations to be equipped with the necessary knowledge and tools to succeed in their STEM field of study. Given the strong empirical evidence supporting the significance of early academic preparation in math to minority student persistence in STEM, earlier interventions TO recruit rather than ARE and prepare URM students to enter and succeed in the STEM workforce is vital (Mau, 2016). Findings from this study underscore the importance of early preparation in math as one of the most significant factors in increasing graduation rates, emphasizing the role of mathematics preparation as an essential basis for establishing minority students’ science identity and integration into their scientific community (Carlone and Johnson, 2007; Estrada et al., 2018). These findings support prior literature that highlight the importance of early intervention efforts at laying the groundwork for URM students to develop the academic skills, resiliency, and motivation to succeed in an increasingly challenging academic environment (Lisberg & Woods, 2017; Ballen et al., 2020; Yeager et al., 2016). Given these findings, further work is needed to disentangle the role of specific intervention efforts such as faculty and peer advising, and collaborative learning relative to the early exposure of key academic content on its own to uncover in which ways they precisely influence student success factors. Such information could provide valuable recommendations to program administrators on how to improve and modify program components in ways that are particularly helpful to URM populations and address their specific challenges. Specifically, future work might explore the relative amount of influence varying degrees of exposure to elements of a comprehensive intervention may have in order to make increasingly informed resource investment decisions toward outcomes of interest.
Furthermore, our results provide a nuanced perspective on progress towards degree completion that involves placing more focus on continuity rather than degree completion within a specific timeframe. For URM student populations with significant financial barriers and family responsibilities, finishing a degree plan within a classic four or six-year time-frame may not be achievable. What our results suggest is that continuity for these students, along with the right intervention and academic support, is important and leads to higher persistence rates in STEM and lower drop-out rates among URM students. Ultimately, these findings point to the need for more nuanced work in how we think of and define persistence for student populations. While this study does take into account graduation within a pre-defined six-year period, our results indicate that total credit hours accumulated was strongly associated with a lower rate of drop-out and a higher rate of persistence among students. Future work needs to go beyond the standard 4- and 6-years guidelines and examine persistence and completion out to the 8-years mark. These studies need to be also be tied to surveys documenting students financial and family situations in tandem with their persistence and completion. Setting realistic, achievable goals balanced with work/life issues would be a better service to students than defining “success” with a 4- or 6-years expiration date. Along those lines, future work should further examine the role of intervention efforts at increasing continuity and progress towards degree completion in a way that defines persistence in its varying degrees as it applies to URM populations.
Data Availability Statement
The raw data supporting the conclusions of this article will be made available by the authors, without undue reservation.
Ethics Statement
Findings from this study were part of an approved Institutional Review Board project.
University of Houston’s Summer Bridge Scholar Enrichment Program.
The University of Houston’s Summer Bridge Scholar Enrichment Program was established in 2000 through funding from the NSF Louis Stokes Alliance for Minority Participation. Primarily focused on increasing the participation of URM students in STEM fields of study, the program pays special attention to the recruitment of low-performing URM students though targeted communication with local high schools to determine eligible candidates that would benefit most from the program’s features. The program’s development was guided by Uri Treisman’s (1992) Mathematics Workshop Model, and centers on using a collaborative and supportive peer learning process promote minority students’ skills in mathematics and STEM foundational courses. The Mathematics Workshop Model is founded upon theories of URM students’ sense of belonging and academic integration and emphasizes the importance of faculty mentoring and collaborative learning techniques for URM student success in STEM fields of study.1
Author Contributions
DG conducted the analyses for this manuscript and prepared the first draft. DP and CH made significant intellectual contributions to the development and editing of the manuscript. DP was involved in SEP operations during 2014 and 2015 and currently oversees the program. All authors approved the final version of the manuscript for publication.
Funding
The research was funded by the National Science Foundation (NSF) Louis Stokes Alliance for Minority Participation. The NSF # for the award dated, 9/1/19–2/28/24, is HRD-1911310(HRD# 000177721) The NSF # for the award dated, 9/1/14–2/28/21, is HRD-1407736(HRD# G107891) The NSF # for the award dated, 9/1/09–8/31/15, is HRD-0903948(HRD# G097982).
Conflict of Interest
The authors declare that the research was conducted in the absence of any commercial or financial relationships that could be construed as a potential conflict of interest.
Footnotes
1For more information on how Treisman’s (1992) model guided the development of the UH summer bridge program, please refer to: Ghazzawi et al., 2020, the previous paper in this series which discusses the impact of program participation on the graduation of URM students in STEM fields.
References
Acton, R. K. (2015). “Characteristics of STEM success: A Survival Analysis Model of Factors Influencing Time to Graduation Among Undergraduate STEM Majors,” . Business and Economics Honors Papers. Paper 1 (Ursinus College).
Adelman, C. (1999). Answers in the Toolbox: Academic Intensity, Attendance Patterns, and Bachelor’s Degree Attainment. U.S. Department of Education. Washington, DC: Office of Educational Research and Improvement. https://files.eric.ed.gov/fulltext/ED431363.pdf.
Ashford, S. N., Lanehart, R. E., KersaintKersaint, G. K., Lee, R. S., and Kromrey, J. D. (2016). STEM Pathways: Examining Persistence in Rigorous Math and Science Course Taking. J. Sci. Educ. Technol. 25 (6), 961–975. doi:10.1007/s10956-016-9654-0
Association of American Medical Colleges (2003). Underrepresented in Medicine Definition. AAMC. https://www.aamc.org/initiatives/urm/.
Astin, A. W., and Astin, H. S. (1992). “Undergraduate Science Education: The Impact Of Different College Environments on the Educational Pipeline in the Sciences,”. Final Report to the National Science Foundation (Grant Number SPA-8955365) (Los Angeles: The Higher Education Research Institute, University of California). https://files.eric.ed.gov/fulltext/ED362404.pdf.
Aulck, L., Aras, R., Li, L., L’Heureux, C., Lu, P., and West, J. (2017). “STEM-ming the Tide: Predicting STEM Attrition Using Student Transcript Data,” in Proceedings of Machine Learning for Education Workshop. Editors S. Matwin, S. Yu, and F. Farooq. https://arxiv.org/pdf/1708.09344.pdf.
Ballen, C. J., Salehi, S., and Sehoya, C. (2020). Variation in Incoming Academic Preparation: Consequences for Minority and First-Generation Students. Front. Edu. 5, 552364. doi:10.3389/feduc.2020.552364
Barlow, A. E. L., and Villarejo, M. (2004). Making a Difference for Minorities: Evaluation of an Educational Enrichment Program. J. Res. Sci. Teach. 41 (9), 861–881. doi:10.1002/tea.20029
Carlone, H. B., and Johnson., A. (2007). Understanding the Science Experiences of Successful Women of Color: Science Identity as an Analytic Lens. J. Res. Sci. Teach. 44 (8), 1187–1218. doi:10.1002/tea.20237
Carpi, A., Ronan, D. M., Falconer, H. M., Lents, N. H., and Lents, N. H. (2017). Cultivating Minority Scientists: Undergraduate Research Increases Self-Efficacy and Career Ambitions for Underrepresented Students in STEM. J. Res. Sci. Teach. 54 (2), 169–194. doi:10.1002/tea.21341
Chang, M. J., Sharkness, J., Hurtado, S., and Newman, C. B. (2014). What Matters in College for Retaining Aspiring Scientists and Engineers from Underrepresented Racial Groups. J. Res. Sci. Teach. 51 (5), 555–580. doi:10.1002/tea.21146
Chen, X. (2013). “STEM Attrition: College Students' Paths into and Out of STEM Fields,” Statistical Analysis Report. NCES 2014-001 (National Center for Education Statistics). https://files.eric.ed.gov/fulltext/ED544470.pdf
Clark, J. V. (2014). “The Road to Excellence: Promoting Access and Equity to Close the Achievement gap Internationally,” in Closing the Achievement gap from an International Perspective (Dordrecht: Springer), 307–315. doi:10.1007/978-94-007-4357-1_15
Crisp, G., Nora, A., and Taggart, A. (2009). Student Characteristics, Pre-college, College, and Environmental Factors as Predictors of Majoring in and Earning a STEM Degree: An Analysis of Students Attending a Hispanic Serving Institution. Am. Educ. Res. J. 46 (4), 924–942. doi:10.3102/0002831209349460
Cullinane, J., and Leewater, L. H. (2009). Diversifying the STEM Pipeline: The Model Replication Institutions Program. Washington, DC: Institute for Higher Education Policy.
Duncan, H., and Dick, T. (2000). Collaborative Workshops and Student Academic Performance in Introductory College Mathematics Courses: A Study of a Treisman Model Math excel Program. Sch. Sci. Math. 100 (7), 365–373. doi:10.1111/j.1949-8594.2000.tb18178.x
Eagan, , Hurtado, S., Chang, M. J., Garcia, G. A., Herrera, F. A., Garibay, J. C., et al. (2013). Making a Difference in Science Education. Am. Educ. Res. J. 50 (4), 683–713. doi:10.3102/0002831213482038
Eagan, K., Hurtado, S., and Chang, M. (2010). “What Matters in STEM: Institutional Contexts that Influence STEM Bachelor’s Degree Completion Rates,” . Paper presented at the annual meeting of the Association for the Study of Higher Education, Indianapolis, IN.
Eddy, S. L., and Hogan, K. A. (2014). Getting under the Hood: How and for Whom Does Increasing Course Structure Work? Lse 13 (3), 453–468. doi:10.1187/cbe.14-03-0050
Espinosa, L. (2011). Pipelines and Pathways: Women of Color in Undergraduate STEM Majors and the College Experiences that Contribute to Persistence. Harv. Educ. Rev. 81 (2), 209–241. doi:10.17763/haer.81.2.92315ww157656k3u
Estrada, M., Burnett, M., Campbell, A. G., Campbell, P. B., Denetclaw, W. F., Gutiérrez, C. G., et al. (2016). Improving Underrepresented Minority Student Persistence in STEM. Lse 15, es5. doi:10.1187/cbe.16-01-0038
Estrada, M., Hernandez, P. R., and Schultz, P. W. (2018). A Longitudinal Study of How Quality Mentorship and Research Experience Integrate Underrepresented Minorities into STEM Careers. Lse 17 (1), ar9. doi:10.1187/cbe.17-04-0066
Estrada, M. (2014). “Ingredients for Improving the Culture of STEM Degree Attainment with Co-curricular Supports for Underrepresented Minority Students,”. National Academies of Sciences White Paper. https://sites.nationalacademies.org/cs/groups/dbassesite/documents/webpage/dbasse_088832.pdf.
Fechheimer, M., Webber, K., and Kleiber, P. B. (2011). How Well Do Undergraduate Research Programs Promote Engagement and Success of Students? Lse 10 (2), 156–163. doi:10.1187/cbe.10-10-0130
Foltz, L. G., Gannon, S., and Kirschmann, S. L. (2014). Factors that Contribute to the Persistence of Minority Students in STEM Fields. Plann. Higher Edu. 42 (4), 1–13. EBSCOhost.
Ghazzawi, D., Patisson, D., Horn, C. L., Brown, B., and Hardy, J. (2020). Impact of an Intensive Multi-Disciplinary STEM Enrichment Program on the Long-Term Impact of Underrepresented Minority Student Success. Manuscript submitted for publication.
Ghee, M., Keels, M., Collins, D., Neal-Spence, C., and Baker, E. (2016). Fine-tuning Summer Research Programs to Promote Underrepresented Students' Persistence in the STEM Pathway. Lse 15 (3), ar28. doi:10.1187/cbe.16-01-0046
Haeger, H., and Fresquez, C. (2016). Mentoring for Inclusion: The Impact of Mentoring on Undergraduate Researchers in the Sciences. Lse 15 (3), ar36–9. doi:10.1187/cbe.16-01-0016
Hausmann, L. R. M., Schofield, J. W., and Woods, R. L. (2007). Sense of Belonging as a Predictor of Intentions to Persist Among African American and White First-Year College Students. Res. High Educ. 48 (7), 803–839. doi:10.1007/s11162-007-9052-9
Heilbronner, N. (2014). International STEM Achievement: Not a Zero-Sum Game. Glob. Edu. Rev. 1 (4), 7–14.
Holland, J. M., Major, D. A., and Orvis, K. A. (2012). Understanding How Peer Mentoring and Capitalization Link STEM Students to Their Majors. Career Dev. Q. 60 (4), 343–354. doi:10.1002/j.2161-0045.2012.00026.x
Hu, S., and Wolniak, G. C. (2010). Initial Evidence on the Influence of College Student Engagement on Early Career Earnings. Res. High Educ. 51 (8), 750–766. doi:10.1007/s11162-010-9176-1
Hurtado, S., Han, J. C., Sáenz, V. B., Espinosa, L. L., Cabrera, N. L., and Cerna, O. S. (2007). Predicting Transition and Adjustment to College: Biomedical and Behavioral Science Aspirants' and Minority Students' First Year of College. Res. High Educ. 48 (7), 841–887. doi:10.1007/s11162-007-9051-x
Jackson, K. M., and Winfield, L. L. (2014). Realigning the Crooked Room: Spelman Claims a Space for African American Women in STEM. Peer Rev. 16 (2), 9–12.
Kendricks, K., Nedunuri, K. V., and Arment, A. R. (2013). Minority Student Perceptions of the Impact of Mentoring to Enhance Academic Performance in STEM Disciplines. J. STEM Educ. Innov. Res. 14 (2), 38–46.
Lane, T. B. (2016). Beyond Academic and Social Integration: Understanding the Impact of a STEM Enrichment Program on the Retention and Degree Attainment of Underrepresented Students. Lse 15 (3), ar39. doi:10.1187/cbe.16-01-0070
Lee, D. M., and Harmon, K. (2013). The Meyerhoff Scholars Program: Changing Minds, Transforming a Campus. Metropolitan Universities 24 (2), 55–70.
Leggon, C. B. (2009). African American Males in Academic Science and Engineering. Divers. Higher Edu. 7, 209–222. doi:10.1108/S1479-3644(2009)0000007013
Lewis, S. E. (2011). Retention and Reform: An Evaluation of Peer-Led Team Learning. J. Chem. Educ. 88 (6), 703–707. doi:10.1021/ed100689m
Lichtenberger, E., and George-Jackson, C. (2013). Predicting High School Students' Interest in Majoring in a STEM Field: Insight into High School Students' Postsecondary Plans. J. Career Tech. Edu. 28 (1), 19–38. doi:10.21061/jcte.v28i1.571
Lisberg, A., and Woods, B. (2018). Mentorship, Mindset and Learning Strategies: An Integrative Approach to Increasing Underrepresented Minority Student Retention in a STEM Undergraduate Program. J. STEM Edu. 19 (3), 14–20.
Loui, M. C., and Robbins, B. A. (2008). “Work-in-progress-assessment of Peer-Led Team Learning in an Engineering Course for Freshmen,” in 2008 38th Annual Frontiers in Education Conference (IEEE), F1F–F7. doi:10.1109/fie.2008.4720315
Marginson, S., Tytler, R., Freeman, B., and Roberts, K. (2013). “STEM: Country Comparisons: International Comparisons of Science, Technology, Engineering and Mathematics (STEM) Education,”. Final report.
Mau, W. J. (2016). Characteristics of US Students that Pursued a STEM Major and Factors that Predicted Their Persistence in Degree Completion. Universal J. Educ. Res. 4 (6), 1495–1500. doi:10.13189/ujer.2016.040630
May, G. S., and Chubin, D. E. (2003). A Retrospective on Undergraduate Engineering Success for Underrepresented Minority Students. J. Eng. Edu. 92 (1), 27–39. doi:10.1002/j.2168-9830.2003.tb00735.x
Mondisa, J., and McComb, S. A. (2015). Social Community: A Mechanism to Explain the Success of STEM Minority Mentoring Programs. Mentoring Tutoring: Partnership Learn. 23 (2), 149–163. doi:10.1080/13611267.2015.1049018
Moreno, S. E., and Muller, C. (1999). Success and Diversity: The Transition through First-Year Calculus in the university. Am. J. Edu. 108 (1), 30–57. doi:10.1086/444231
Murphy, T. E., Gaughan, M., Hume, R., and Moore, S. G. (2010). College Graduation Rates for Minority Students in a Selective Technical University: Will Participation in a Summer Bridge Program Contribute to Success? Educ. Eval. Pol. Anal. 32 (1), 70–83. doi:10.3102/0162373709360064
Mutegi, J. W. (2013). life's First Need Is for Us to Be Realistic" and Other Reasons for Examining the Sociocultural Construction of Race in the Science Performance of African American Students. J. Res. Sci. Teach. 50 (1), 82–103. doi:10.1002/tea.21065
National Academies of Sciences, Engineering, and Medicine (2019). Increasing Student Success in Developmental Mathematics: Proceedings of a Workshop. Washington, DC: The National Academies Press. doi:10.17226/25547
National Center for Science and Engineering Statistics. (2018). Science and Engineering Degrees, by Race and Ethnicity of Recipients. https://www.nsf.gov/statistics/degreerecipients/.
Nick, T. G., and Campbell, K. M. (2007). Logistic Regression. Top. biostatistics 404, 273–301. doi:10.1007/978-1-59745-530-5_14
Ottenbacher, K. J., Ottenbacher, H. R., Tooth, L., and Ostir, G. V. (2004). A Review of Two Journals Found that Articles Using Multivariable Logistic Regression Frequently Did Not Report Commonly Recommended Assumptions. J. Clin. Epidemiol. 57 (11), 1147–1152. doi:10.1016/j.jclinepi.2003.05.003
Pascarella, E. T., and Terenzini, P. T. (1991). How College Affects Students: Findings and Insights from Twenty Years of Research. San Francisco: Jossey-Bass.
Pascarella, E. T., Salisbury, M. H., and Blaich, C. (2011). Exposure to Effective Instruction and College Student Persistence: A Multi-Institutional Replication and Extension. J. Coll. Student Dev. 52 (1), 4–19. doi:10.1353/csd.2011.0005
Rask, K. (2010). Attrition in STEM fields at a Liberal Arts College: The Importance of Grades and Pre-collegiate Preferences. Econ. Edu. Rev. 29 (6), 892–900. doi:10.1016/j.econedurev.2010.06.013
Reisel, J., Jablonski, M., Munson, E., and Hosseini, H. (2014). Peer-led Team Learning in Mathematics Courses for Freshmen Engineering Students. J. STEM Edu. 15 (2), 7–15.
Riegle-Crumb, C., King, B., and Irizarry, Y. (2019). Does STEM Stand Out? Examining Racial/Ethnic Gaps in Persistence across Postsecondary Fields. Educ. Res. 48 (3), 133–144. doi:10.3102/0013189X19831006
Sadler, P. M., Sonnert, G., Hazari, Z., and Tai, R. (2014). The Role of Advanced High School Coursework in Increasing STEM Career Interest. Sci. Educator 23 (1), 1–13.
Saw, G., Chang, C.-N., and Chan, H.-Y. (2018). Cross-Sectional and Longitudinal Disparities in STEM Career Aspirations at the Intersection of Gender, Race/Ethnicity, and Socioeconomic Status. Educ. Res. 47 (8), 525–531. doi:10.3102/0013189X18787818
Schultz, P. W., Hernandez, P. R., Woodcock, A., Estrada, M., Chance, R. C., Aguilar, M., et al. (2011). Patching the Pipeline. Educ. Eval. Pol. Anal. 33 (1), 95–114. doi:10.3102/0162373710392371
Shaw, E. J., and Barbuti., S. (2010). Patterns of Persistence in Intended College Major with a Focus on STEM Majors. NACADA J. 30 (2), 19–34. doi:10.12930/0271-9517-30.2.19
Shields, S. P., Hogrebe, M. C., Spees, W. M., Handlin, L. B., Noelken, G. P., Riley, J. M., et al. (2012). A Transition Program for Underprepared Students in General Chemistry: Diagnosis, Implementation, and Evaluation. J. Chem. Educ. 89 (8), 995–1000. doi:10.1021/ed100410j
Singer, J. D., and Willett, J. B. (1993). It's about Time: Using Discrete-Time Survival Analysis to Study Duration and the Timing of Events. J. Educ. Stat. 18 (2), 155–195. doi:10.3102/10769986018002155
Singer, J. D., and Willet, J. B. (2003). Applied Longitudinal Data Analysis: Modeling Change and Event Occurrence. New York: Oxford University Press.
Smith, J., Wilson, S. B., Banks, J., Zhu, L., and Varma-Nelson, P. (2014). Replicating Peer-Led Team Learning in Cyberspace: Research, Opportunities, and Challenges. J. Res. Sci. Teach. 51 (6), 714–740. doi:10.1002/tea.21163
Treisman, U. (1992). Studying Students Studying Calculus: A Look at the Lives of Minority Mathematics Students in College. Coll. Math. J. 23 (23), 362–372. doi:10.1080/07468342.1992.11973486
U.S. Census Bureau (2019). Population Estimates. https://www.census.gov/quickfacts/fact/table/US/PST045219.
Whalen, D. F., and Shelley, M. C. (2010). Academic success for STEM and Non-STEM Majors. J. STEM Educ. Innov. Res. 11 (1), 45–60.
Wilson, A. H., Sanner, S., and McAllister, L. E. (2010). An Evaluation Study of a Mentoring Program to Increase the Diversity of the Nursing Workforce. J. Cult. Divers. 17 (4), 144–150. doi:10.21236/ada612587
Keywords: stem education, persistence, underrepresented minorities, intervention, racial differences
Citation: Ghazzawi D, Pattison D and Horn C (2021) Persistence of Underrepresented Minorities in STEM Fields: Are Summer Bridge Programs Sufficient?. Front. Educ. 6:630529. doi: 10.3389/feduc.2021.630529
Received: 17 November 2020; Accepted: 08 June 2021;
Published: 05 July 2021.
Edited by:
Chris Botanga, Chicago State University, United StatesReviewed by:
Hui-Chuan Li, University of Edinburgh, United KingdomFrank Reinhold, University of Education Freiburg, Germany
Copyright © 2021 Ghazzawi, Pattison and Horn. This is an open-access article distributed under the terms of the Creative Commons Attribution License (CC BY). The use, distribution or reproduction in other forums is permitted, provided the original author(s) and the copyright owner(s) are credited and that the original publication in this journal is cited, in accordance with accepted academic practice. No use, distribution or reproduction is permitted which does not comply with these terms.
*Correspondence: Dina Ghazzawi, dalghazzawi@uh.edu