Policy significance statement
The study provides insights on the role of trust, perceived benefits, and perceived risk as determinants of university students’ intention to share location data during health emergencies. Results enhance policy makers’ ability to understand, predict, and proactively act to influence the social acceptance of services that exploits location data to manage emergency situations. Based on the empirical analysis, suggestions are made for policy makers. These include strengthening communication strategies aimed at increasing the perceived benefits deriving from sharing personal location data; implementing organizational strategies that could contribute to decrease university students’ perception of risk; leverage on the involvement of third-party trustworthy organizations.
1. Introduction
The coronavirus disease-2019 (COVID-19) pandemic, spreading around the world since late 2019, has caused many States to put in place unprecedented containment measures, in an attempt to contain the spread of the virus. Among the different measures adopted, several countries have developed contact tracing apps, on the wave of positive results obtained in Singapore and South Korea (Whitelaw et al., Reference Whitelaw, Mamas, Topol and Van Spall2020). Simulations indicate that contact tracing apps could prevent up to 80% of the virus’ transmissions in case the majority of the population would download them (Kretzschmar et al., Reference Kretzschmar, Rozhnova, Martin, van Boven, van de Wijgert and Marc2020). However, contrary to expectations, the apps’ penetration rates with respect to the total population ranged from 0.04% in Mexico to 80% in Singapore, with a standard deviation of 20,6 percentage points and mean of 16.85%. These results are quite unsatisfactory since only 34% of the countries registered have reached the threshold of 20% at which simulations showed the first positive impacts on the reduction of transmissions (Armbruster and Brandeau, Reference Armbruster and Brandeau2007). Contact tracing app can be designed according to two major technical configurations: GPS and Bluetooth. While the first type collects location data, the second one leverages on encrypted tokens to track proximity between individuals (Urbaczewski and Lee, Reference Urbaczewski and Lee2020). Although for contact tracing purpose the two technical configurations are equivalent, the usage of mobility data (i.e., GPS) supports the investigation of spatial heterogeneity in the spread of virus (Desai et al., Reference Desai, Kraemer, Bhatia, Cori, Nouvellet, Herringer, Cohn, Carrion, Brownstein, Madoff and Lassmann2019; Budd et al., Reference Budd, Miller, Manning, Lampos, Zhuang, Edelstein, Rees, Emery, Stevens, Keegan, Short, Pillay, Manley, Cox, Heymann, Johnson and McKendry2020), hence providing relevant insights for policy makers for designing tailored and effective interventions (Cori et al., Reference Cori, Donnelly, Dorigatti, Ferguson, Cori, Donnelly, Dorigatti, Ferguson, Fraser, Garske, Jombart, Nedjati-Gilani, Nouvellet, Riley, Van, Mills and Blake2017).
Understanding which factors influence data-sharing initiatives is a research area that has drawn the attention of researchers in the last 50 years (Culnan, Reference Culnan1995), leaving three major development phases.
The determinants of individuals’ intention to disclose personal information have been investigated using the general privacy theories developed by Altman (Reference Altman1975), Westin (Reference Westin1967), and Margulis (Reference Margulis1977, Reference Margulis2003). Smith et al. (Reference Smith, Dinev and Xu2011) provided an overarching macro framework describing the relationship between privacy concern and other constructs as a result of a literature review of privacy related research including 320 privacy articles and 128 book sections. Referred to as the Antecedents → Privacy Concerns → Outcomes (APCO) model, the model links citizens’ privacy concerns in disclosing personal information with its antecedents and outcomes. Specifically, synthetizing previous literature on the topic, Smith identifies the privacy experience and awareness, the personality and demographic differences and the culture as antecedent factors influencing the privacy concern, while the trust level, the privacy calculus, the behavioral reaction, and the regulations are considered outcomes.
A second wave of attention to the topic began in the second decade of the 2000s with the widespread use of digital technologies. Understanding which factors (both personal and contextual) have direct or indirect influence on the intention to share data, has become a relevant research topic for public and private organizations (Chopdar et al., Reference Chopdar, Korfiatis, Sivakumar and Lytras2018). Indeed, demographic variables such as age and education directly influence the willingness to share personal data through digital apps. Among other findings, this body of research suggests that individuals with higher education tend to make more balanced judgments regarding public management, exhibit greater trust in public institutions than their counterparts, and, as a result, may be more inclined to share data with these institutions (Tenopir et al., Reference Tenopir, Dalton, Allard, Frame, Pjesivac, Birch, Pollock and Dorsett2015). In addition to personal factors, this research has also confirmed that contextual elements influence trust levels in public institutions (Newton, Reference Newton, Zmerli and van der Meer2017) and consequently the propension to share data with them (e.g., higher freedom of expression decreases the trust level in public institutions (Yakovlev and Gilson, Reference Yakovlev and Gilson2015).
Lastly, because of its world-wide impact and the widespread use of contact tracing apps, the pandemic has prompted a new wave of studies linking the adoption of these apps with the motivations behind it in terms of willingness to share data during an outbreak condition. These studies are either focused on specific geographies, population sub-group or even cross-countries comparisons. For instance, the role of demographic characteristics on the acceptance of digital surveillance technologies have been confirmed by recent studies with a focus on COVID-19 outbreak, with younger generations showing greater acceptance of contact tracing apps compared to elderly generations (Altmann et al., Reference Altmann, Milsom, Zillessen, Blasone, Gerdon, Bach, Kreuter, Nosenzo, Toussaert and Abeler2020; Kostka and Habich-Sobiegalla, Reference Kostka and Habich-Sobiegalla2020; Garrett et al., Reference Garrett, Wang, White, Hsieh, Strong, Lee, Lewandowsky, Dennis and Yang2021; Lederer et al., Reference Lederer, Hoban, Lipson, Zhou and Eisenberg2021; von Wyl et al., Reference von Wyl, Höglinger, Sieber, Kaufmann, Moser, Serra-Burriel, Ballouz, Menges, Frei and Puhan2021). However, the ineffectiveness of the tracing apps in limiting the spread of COVID-19 have been investigated mainly by looking at one single factor such incentives (Munzert et al., Reference Munzert, Selb, Gohdes, Stoetzer and Lowe2021), technical factors (Trang et al., Reference Trang, Trenz, Weiger, Tarafdar and Cheung2020) or privacy concern (Bengio et al., Reference Bengio, Ippolito, Janda, Jarvie, Prud’homme, Rousseau, Sharma and Yu2021). Furthermore, authors investigated the adoption of contact tracing apps developed to contain COVID-19 diffusion (Altmann et al., Reference Altmann, Milsom, Zillessen, Blasone, Gerdon, Bach, Kreuter, Nosenzo, Toussaert and Abeler2020; Moon, Reference Moon2020; Oldeweme et al., Reference Oldeweme, Märtins, Westmattelmann and Schewe2021; von Wyl et al., Reference von Wyl, Höglinger, Sieber, Kaufmann, Moser, Serra-Burriel, Ballouz, Menges, Frei and Puhan2021; Walrave et al., Reference Walrave, Waeterloos and Ponnet2021), rather than the willingness of individuals to share their personal data. Answering the call for additional empirical research studies for testing and validating the relationships put forward by other authors, this research focuses on the links between trust and privacy benefits and privacy risks, whose trade-off evaluation has been named as “privacy calculus”, with the intention to disclose location data. Using as reference the Theory of Reasoned Action (TRA) exploring the relationship between the actual behavior of individuals and the intention to perform that specific behavior (Fishbein and Ajzen, Reference Fishbein and Ajzen1975), the model assume that behavioral reaction is comparable to the intention of citizens in disclosing their personal information with other parties.
The aim of this research is to analyze the influence of trust in the organization collecting data, the positive trade-off between the perceived privacy benefit and the perceived privacy risk on university students’ intention to share personal location data. The research empirically tests the hypothesis put forward in the APCO model on determinants of personal data sharing (Smith et al., Reference Smith, Dinev and Xu2011), focusing on the factors that may bring Italian university students to share their location data with public institutions during health emergency. Different elements render this subpopulation group particularly relevant for analysis within the scope of this study. Younger individuals are often early adopters of digital technologies and show greater willingness to embrace digital surveillance technologies (Altmann et al., Reference Altmann, Milsom, Zillessen, Blasone, Gerdon, Bach, Kreuter, Nosenzo, Toussaert and Abeler2020; Kostka and Habich-Sobiegalla, Reference Kostka and Habich-Sobiegalla2020; von Wyl et al., Reference von Wyl, Höglinger, Sieber, Kaufmann, Moser, Serra-Burriel, Ballouz, Menges, Frei and Puhan2021). Understanding their motivations is key to understanding future behaviors of the broader population. Additionally, the higher engagement in social activities together with the frequent absence of the symptoms and the consequent lower perceived vulnerability made younger generations a substantial source of COVID-19 transmission (Garg et al., Reference Garg, Kim, Whitaker, O’Halloran, Cummings, Holstein, Prill, Chai, Kirley, Alden, Kawasaki, Yousey-Hindes, Niccolai, Anderson, Openo, Weigel, Monroe, Ryan, Henderson, Kim, Como-Sabetti, Lynfield, Sosin, Torres, Muse, Bennett, Billing, Sutton, West, Schaffner, Talbot, Aquino, George, Budd, Brammer, Langley, Hall and Fry2020; Liao et al., Reference Liao, Fan, Chen, Wu, Xu, Guo, Li, Zhang, Wu, Mou, Song, Li, Wu, Zhang, Guo, Liu, Lv, Xu and Lang2020). Furthermore, having been Italy significantly affected by COVID-19, the enforced extensive lockdowns during the initial waves, saw university students among those whose life was mostly disrupted, including the shift from in-person to e-learning (Lederer et al., Reference Lederer, Hoban, Lipson, Zhou and Eisenberg2021; Maatuk et al., Reference Maatuk, Elberkawi, Aljawarneh, Rashaideh and Alharbi2022) and its consequent psychological repercussions (Berman et al., Reference Berman, Bendtsen, Molander, Lindfors, Lindner, Granlund, Topooco, Engström and Andersson2022).
To guide our research study, we have formulated the following research questions:
RQ1. To what extent is trust in public institutions related to university students’ intention to share their location data with them?
RQ2. To what extent are the perceived benefit and perceived risk of sharing location data related to university students’ intention to share their location data with public institutions?
The remainder of this paper consists of five sections. Following Section 1, Section 2 describes the overarching theory and provides the theoretical framework developed based on the State of the art on the determinants of individuals’ intention to share personal data. In Section 3, we introduce the methodology adopted for empirically testing the theoretical framework and describe the peculiarities of the Italian context. In Section 4, results of the study are presented and discussed. Finally, in Section 5 main results are summarized and theoretical and practical implications are discussed.
2. Research Model and Hypotheses Development
Throughout the last decades, the analysis of the attitude and the behavior of individuals towards the disclosure of personal information has drawn the attention of scholars from several disciplines ranging from marketing to information science and organizational behavior. In 2011 Smith et al. offered an overall macro framework defining the link between privacy concerns and other constructs. The model is based on the systematic literature review of 408 different sources which brought to the identification of three main research streams, focusing on the conceptualization of information privacy, the relationship between information privacy and other constructs, and the contextual nature of these relationships. Authors connected the three streams and synthetized their findings in the APCO model. The model relates the privacy concerns of people over the disclosure of personal information to its antecedents and outcomes (Figure 1).

Figure 1. Antecedents privacy concerns outcomes model (Smith et al., Reference Smith, Dinev and Xu2011).
The model illustrates the relationship between a number of antecedents and the privacy concerns. This latter is the result of personal characteristics of individuals and past experiences influencing the evaluation of potential risks resulting from the information disclosure (Xu et al., Reference Xu, Dinev, Smith and Hart2008). High privacy concerns can be the result of negative past experiences where the individual was subject to personal information abuses (Smith et al., Reference Smith, Milberg and Burke1996). The awareness on the information collected by the organizations and on the procedures implemented for safeguarding privacy are also related to the privacy concerns. Individuals’ characteristics including personality, demographic and cultural differences can influence the privacy concerns. With respect to differences in personality, authors investigated the impact of introverted and extroverted personality (Lu et al., Reference Lu, Tan and Hui2004), the incidence of independence (Xu, Reference Xu2007) and the influence of the big-five personality traits (Bansal et al., Reference Bansal, Mariam and Gefen2010). Socio-demographic characteristics, such as the gender, the age or the income also affect the privacy concerns of individuals. Researchers also demonstrated that women (Sheehan, Reference Sheehan1999), elderly people and wealthy people are more concerned with privacy if compared to other demographic groups (Culnan, Reference Culnan1995). Lastly, according to the APCO model, cultural aspects characterizing the society have also an impact on the privacy concerns. The concept of privacy can be, indeed, shaped by cultural beliefs (Dinev et al., Reference Dinev, Bellotto, Hart, Russo and Serra2006).
On the right side of the framework, the model treats the privacy concerns as an independent variable, hence structuring and analyzing the relationship between privacy concerns and other outcomes. The primary outcome investigated by authors in the field of information privacy is the behavioral reactions of individuals in connection with the willingness of disclosing information. Consistently with the TRA (Fishbein and Ajzen, Reference Fishbein and Ajzen1975), actual behavioral reaction is considered by authors as comparable of intentions. Hence, by measuring the intention of individuals’ towards sharing their personal information authors can investigate the actual behaviors of citizens. In addition to privacy concern, authors investigated the impact of other constructs on the willingness to share personal information, including trust and privacy calculus.
The role of trust has drawn the attention of several scholars examining the direct impact of the construct on the behavioral reaction (Milne and Boza, Reference Milne and Boza1999; Schoenbachler and Gordon, Reference Schoenbachler and Gordon2002) or its mediating role of the privacy concerns (Metzger, Reference Metzger2004; Xu et al., Reference Xu, Teo and Bernard2005). Researchers agree on the role of trust as an important determinant of individual data sharing, recognizing trust in service providers as a facilitator for data sharing as citizens are more willing to share their tracking data with trustworthy and transparent service providers (Aloudat et al., Reference Aloudat, Michael, Chen and Al-Debei2014). More recently, in confirmation of this relationship, studies highlighted that higher citizens’ trust in governments could have positively affected the willingness of citizens in sharing their tracking data for supporting public institutions in managing the COVID-19 pandemic (Leins and Culnane, Reference Leins and Culnane2020).
Other research studies investigated the role of privacy calculus, defined as the tradeoff between costs and benefits, as a determinant of individual’s behavioral reactions. Several works suggested that, as for economic evaluation, individuals assess the risks and the benefits of sharing personal information, accordingly making a choice (Milne and Gordon, Reference Milne and Gordon1993; Culnan Reference Culnan1995; Chellappa and Sin, Reference Chellappa and Sin2005; Dinev et al., Reference Dinev, Bellotto, Hart, Russo and Serra2006; Hui et al., Reference Hui, Tan and Goh2006; Hann et al., Reference Hann, Hui, Lee and Png2008; Xu et al., Reference Dinev, Xu and Smith2009). Smith et al., defined privacy risks as “the degree to which an individual believes that a high potential for loss is associated with the release of personal information.” The consequence of such loss includes organizational opportunistic behavior, such as insider disclosure (Rindfleisch, Reference Rindfleisch1997), unauthorized access and theft, and the provision of personal data to third parties (Budnitz, Reference Budnitz1998; Preston, Reference Preston2004; Wald, Reference Wald2004). The assessment of the risk covers the likelihood of the consequences and the perceived severity (Peter and Tarpey, Reference Peter and Tarpey1975). According to Smith et al., the intention of citizens to provide data is further facilitated by the benefits they perceive from the service enabled by the data sharing. A recent study shows that benefits might be more influential than citizens’ perceived risks in determining their intention to share tracking data (Cho et al., Reference Cho, Ippolito and Yu2020).
As the risk associated to the sharing of personal information may affect the individual’s emotion and perceptions (Moon, Reference Moon2020), the evaluation of the risk associated with the disclosing of personal information is subjective. Thus, we will refer to the risk as perceived risks.
The same consideration holds true for the benefits individuals perceive they may derive from sharing their data, indeed “individuals are assumed to behave in ways that they believe will result in the most favorable net level of outcomes” (Stone and Stone, Reference Stone and Stone1990, p. 363). Despite literature often refers to this concept as privacy benefits; since in our model the privacy is not treated as linked with the privacy concerns, but rather as a determinant of the behavioral reaction, we decided to refer to the concepts of benefits and risks as perceived benefits and perceived risks. The prefix perceived was added to emphasize its character as a perception rather than an actual event.
Bearing in mind the relationships identified by authors and summarized by Smith et al., in their APCO model, we test the influence of trust in public institutions and the perceived benefits and perceived risks on the willingness of university students to share personal tracking data. It is worth to mention that in this study, we used the term location data and tracking data interchangeably. Following the APCO model, the definition of target population and the use of control variables enable the minimization of the influence of privacy concerns on behavioral reactions, trust and perceived risks.
The research is focused on a particularly relevant population subset: Italian university students. In general, it is significant to study sub-population groups (e.g., young adults, elderly, disabled), to ensure their representativeness in the scientific and political debate (Naudé and Vinuesa, Reference Naudé and Vinuesa2021) and support a better-informed policy decision making.
Several compelling factors render this subpopulation group particularly relevant for examination within the scope of this study.
Recent studies on COVID-19 have highlighted the connection between age and data-sharing behaviors. Previous research in various contexts, such as health (Yamin et al., Reference Yamin, Emani, Williams, Lipsitz, Karson, Wald and Bates2011; Ghafur et al., Reference Ghafur, Van Dael, Leis, Darzi and Sheikh2020) and research (Tenopir et al., Reference Tenopir, Rice, Allard, Baird, Borycz, Christian, Grant, Olendorf and Sandusky2020), has demonstrated differences in data sharing behaviors among subpopulations, emphasizing how demographics shape the intention and actual behavior of sharing. The demographics of a population also significantly affect the acceptance of contact tracing apps during the COVID-19 pandemic, with younger individuals displaying greater willingness to adopt digital surveillance technologies compared to their elderly counterparts (Altmann et al., Reference Altmann, Milsom, Zillessen, Blasone, Gerdon, Bach, Kreuter, Nosenzo, Toussaert and Abeler2020; Kostka et al., Reference Kostka and Habich-Sobiegalla2020; Garrett et al., Reference Garrett, Wang, White, Hsieh, Strong, Lee, Lewandowsky, Dennis and Yang2021; Lederer et al., Reference Lederer, Hoban, Lipson, Zhou and Eisenberg2021; von Wyl et al., Reference von Wyl, Höglinger, Sieber, Kaufmann, Moser, Serra-Burriel, Ballouz, Menges, Frei and Puhan2021). Often regarded as early adopters, comprehending the drivers of location data sharing within this subgroup is crucial for understanding the future behavior of the broader population.
Additionally, the role of “superspreaders” of young generation was proved by recent study conducted during COVID-19 outbreak (Laxminarayan et al., Reference Laxminarayan, Wahl, Dudala, Gopal, Mohan, Neelima, Reddy, Radhakrishnan and Lewnard2020). Younger individuals, engaging more frequently in social activities and often asymptomatic, have emerged as significant sources of COVID-19 transmission (Garg et al., Reference Garg, Kim, Whitaker, O’Halloran, Cummings, Holstein, Prill, Chai, Kirley, Alden, Kawasaki, Yousey-Hindes, Niccolai, Anderson, Openo, Weigel, Monroe, Ryan, Henderson, Kim, Como-Sabetti, Lynfield, Sosin, Torres, Muse, Bennett, Billing, Sutton, West, Schaffner, Talbot, Aquino, George, Budd, Brammer, Langley, Hall and Fry2020; Liao et al., Reference Liao, Fan, Chen, Wu, Xu, Guo, Li, Zhang, Wu, Mou, Song, Li, Wu, Zhang, Guo, Liu, Lv, Xu and Lang2020). Furthermore, the incidence of COVID-19 has disproportionately increased among individuals aged 0–39 years as the pandemic has progressed, resulting in a shift in the age distribution of cases, with a higher proportion now occurring among younger demographics (Malmgren et al., Reference Malmgren, Guo and Kaplan2020).
From a contextual perspective, as socioeconomic and political elements affect the level of trust, perceived privacy benefits, and risks, studying local/national contexts is also significant to inform national policies (Ghafur et al., Reference Ghafur, Van Dael, Leis, Darzi and Sheikh2020; Naudé and Vinuesa, Reference Naudé and Vinuesa2021). Italy was among the most affected countries during the first COVID-19 wave with full lock down in place for several weeks during both the first and the second wave. Italian university students constitute therefore an interesting population for our study since they were particularly impacted in their daily routine, having to move from in-person to e-learning (Lederer et al., Reference Lederer, Hoban, Lipson, Zhou and Eisenberg2021; Berman et al., Reference Berman, Bendtsen, Molander, Lindfors, Lindner, Granlund, Topooco, Engström and Andersson2022; Maatuk et al., Reference Maatuk, Elberkawi, Aljawarneh, Rashaideh and Alharbi2022).
On these premises, we put forward the following hypothesis:
HP1: University students’ trust in public institutions positively influences their intention to share personal tracking data with them.
HP2: The benefits that university students perceive in sharing tracking data with public institutions are positively related to their intention to share data with them.
HP3: The risks that university students perceive in sharing tracking data with public institutions are negatively related to university students’ intention to share their data with them.
Large body of empirical literature investigated the relationships between the perceived benefits, the perceived risks and the trust suggesting that mediation effects can be observed when analyzing the influence of benefits, risk and trust on behaviors. Thus, the rest of the research hypothesis were developed on the basis of existing empirical evidence, still formulated consistently with the APCO theory.
A considerable body of literature supported the mediating role of perceived risk and perceived benefits in the relationship between trust and behavior. The relationship between trust and perceived risk was suggested in 1999 by Jarvenpaa et al. According to the authors, the level of trust affects the level of perceived risk which, in turn, influence the intention of individuals (Jarvenpaa et al., Reference Jarvenpaa, Tractinsky and Saarinen1999). Sztompka suggested that trust can nullify the perception of risks, hence considering the lack of trust as a dichotomous variable activating the perception of risk (Sztompka, Reference Sztompka1999). Other authors provided evidence of the mediating role of perceived risk in the relationship between trust and behavior in the field of ecommerce (Stewart, Reference Stewart1999; Ratnasingam and Klein, Reference Ratnasingam and Klein2001).
Moreover, the level of trust can positively influence the perceived benefits by reducing the uncertainty associated to the behavior (Ratnasingam and Klein, Reference Ratnasingam and Klein2001; Aloudat et al., Reference Aloudat, Michael, Chen and Al-Debei2013). Hence, it is posited that the level of university students’ trust in public institutions negatively influences the risk that university students perceived in sharing tracking data and positively influences the benefit university students perceive in sharing tracking data. The following hypothesis are then put forward:
HP4: University students’ trust in public institutions negatively influences the perceived risks that university students perceive in sharing tracking data
HP5: University students’ trust in public institutions positively influences the perceived benefits that university students perceive in sharing tracking data
Finally, previous studies posited a relationship between the perceived risk and the perceived benefits (Aloudat et al., Reference Aloudat, Michael, Chen and Al-Debei2013). In their study, Aloudat et al. define the role of perceived risk for the acceptance and the usage of e-services as the individual loss and the perception of negative consequences arising from the usage of the service (Pavlou and Gefen, Reference Pavlou and Gefen2004; Van der Heijden et al., Reference Van der Heijden, Ogertschnig and Van Der Gaast2005). The perceived risk can hence negatively impact on the individual perception of the benefits perceived in sharing tracking data. The following hypothesis is proposed:
HP6: The risks that university students perceive in sharing tracking data with public institutions are negatively related to the benefits that university students perceive in sharing tracking data
All the hypothesis investigated and the different constructs are shown in Figure 2.
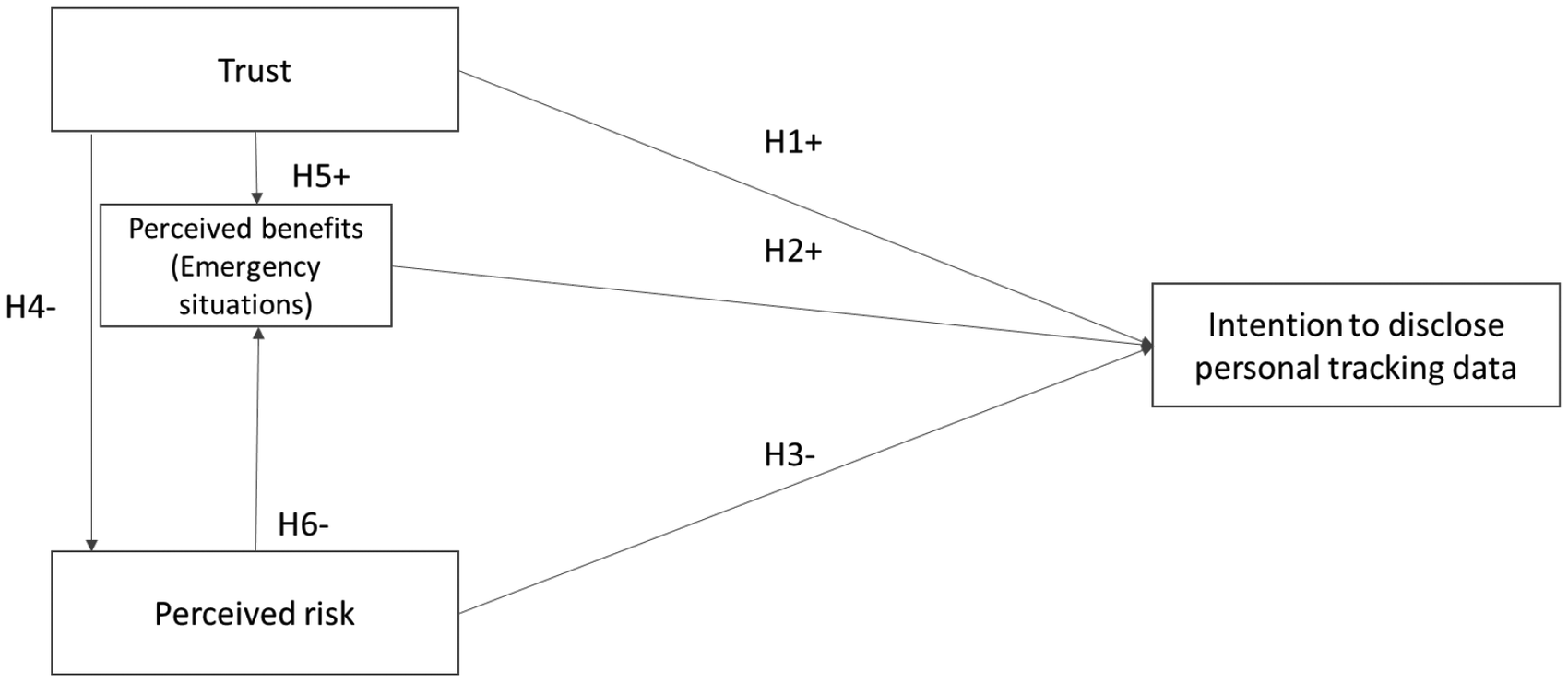
Figure 2. Research framework.
3. Methodology
3.1 Instrument development
The survey questionnaire was designed based on the privacy literature, with a focus on tracking data. Following our research aims, the main constructs measured concern trust, perceived benefits, perceived risks, and intentional reaction to disclose personal information. When possible, the measures have been derived by investigating privacy literature about tracking data sharing with the service provider. Constructs from the measurement scale used in prior studies were adapted to fit the public institutions as the service provider. More precisely, in the questionnaire we refer to the Ministry of Health as an example of public institutions. Since measures for perceived benefits related to the contact tracing purpose were not already available, ad hoc scales were developed, based on the literature findings.
Literature treated the concept of trust as a multi-dimensional construct. In particular, Davis and Schoorman (1995) proposed that trust consists of three distinct components that comprise the overall trustworthiness: ability, benevolence, and integrity (Mayer et al., Reference Mayer, Davis and Schoorman1995). Ability refers to the set of skills a trustee has in managing the data within a specific domain. Benevolence refers to the perceived willingness of a trustee to pursue the trustor’s good aside profit. Integrity refers to an organization’s adherence to predefined rules, acceptable to both trustee and trustor (Mayer et al., Reference Mayer, Davis and Schoorman1995).
Moreover, literature considers perceived benefits a multi-dimensional construct where dimensions are defined according to the contextual setting of the study. As the focus of the research study is the willingness of sharing tracking data with public institutions in the emergency context, benefit involves the provision of services preventing the spread of infectious disease (Aloudat et al. Reference Aloudat, Michael, Chen and Al-Debei2014) through the analysis of mobility dynamics on spatial and temporal scale (i.e., outbreaks) (Aloudat et al., Reference Aloudat, Michael and Abbas2009, Reference Aloudat, Michael, Chen and Al-Debei2014; Desai et al., Reference Desai, Kraemer, Bhatia, Cori, Nouvellet, Herringer, Cohn, Carrion, Brownstein, Madoff and Lassmann2019).
Literature treats perceived risk as a single-dimensional construct that measures the potential loss of control over personal information (Dinev et al., Reference Dinev, Xu and Smith2009). Different sources of organizational opportunistic behavior are identified, including insider disclosure or unauthorized access and theft (Thomas Rindfleisch, Reference Rindfleisch1997), and selling personal data to, or sharing information with third parties or financial institutions (Budnitz, Reference Budnitz1998).
According to privacy literature, the intention of sharing tracking data with service providers is a mono-dimensional concept and it is measured with three scales expressing the likelihood of data disclosure (Venkatesh et al., Reference Venkatesh, Morris, Davis and Davis2003). The scales for measuring the constructs and the studies from which the scales were derived can be found in Supplementary Tables S5 and S6.
Previous research studies have emphasized the influence of various factors on the relationship between trust, perceived benefits, perceived risks, and the willingness to share personal data. To address issues of internal validity and rule out alternative explanations, it is essential to control for these factors (Schmitt and Klimoski, Reference Schmitt and Klimoski1991) or manipulate the sample nature to ensure uniformity across participants (Keppel, Reference Keppel1991). The former, an experimental design choice, ensures consistency among individuals in the sample, while the latter, a statistical choice, isolates the effect of these factors from other relationships of interest in the statistical analysis.
As detailed in Section 3.2, we selected a target population with fixed privacy concerns antecedents, mitigating demographic differences and culture/climate through sample selection. This approach ensures that participants share similar characteristics, providing meaningful insights into the role of trust, perceived benefits, and perceived risks in data sharing within this specific population segment.
Additionally, we used control variables to account for other antecedents, namely privacy awareness, privacy experience, and personality differences. Controlling for these factors helps isolate the impact of the relationships of interest and addresses potential confounding effects. Previous research has shown that negative past privacy experiences can increase privacy concerns, influencing data sharing behavior (Smith et al., Reference Smith, Milberg and Burke1996). The awareness of individuals about the information collected by organizations and their usage, along with the existence of privacy safeguarding procedures, also impact privacy concerns. Moreover, authors have highlighted the role of personality in shaping privacy concerns (Lu et al., Reference Lu, Tan and Hui2004; Bansal et al., Reference Bansal, Mariam and Gefen2010). Despite these factors potentially correlating with the willingness to share personal data, we consider them as biasing factors that need controlling to ensure the validity of our results.
Furthermore, to check for results consistency, we formed a secondary analysis excluding control variables from our model. The consistency of results from this secondary analysis demonstrated that the presence of control variables did not lead to misleading findings (Becker, Reference Becker2005).
According to Bansal et al. (Reference Bansal, Mariam and Gefen2010), Goldberg’s Big-Five factors (Goldberg, Reference Goldberg1990, Reference Goldberg1992) of personality, that is, Extroversion, Agreeableness, Emotional Instability, Consciousness, and Intellect, were used to assess the personality differences of the participants. Bansal’s study demonstrated a low influence of “Agreeableness” and “Intellect” and no influence from “Extroversion” and “Conscientiousness” on the risk and benefit calculus to share data. Being four out of five personality dimensions not influential for the risk and benefits calculus, we treated Emotional Stability as the only influential factor and therefore as a proxy of the “Personality differences” reported by the APCO model. The items used for measuring control variables and the respective studies can be found in Supplementary Table S7.
All the items defined in Supplementary Tables S5–S7, are measured with a 7-point Likert scale (1: strongly disagree, 7: strongly agree). Indeed, according to authors higher reliability and stronger correlations with t-test have been experienced when using a 7 points scale (Lewis, Reference Lewis1993; Colman et al., Reference Colman, Norris and Preston1997). Furthermore, as most of the empirical studies on privacy adopt a 7 points scale, it would be possible to compare results and reliability with studies using the same scales.
Following the development phase, the questionnaire was tested in order to validate the research instrument, hence strengthening the confirmatory empirical results (Straub, Reference Straub1989). First, the time required to complete the survey was calculated by carrying out an observational study with two students with limited/no knowledge on the topic of information sharing. Second, a pilot of the questionnaire was sent to ten students to test the questionnaire before its large-scale deployment. The feedbacks about problems and difficulties when answering received in this phase were used to update the questionnaire with minor wording amendments.
3.2 Sampling, data collection, and research context
Following the APCO model, the target population has been selected with fixed privacy concerns antecedents, that is, demographic differences, culture/climate, to minimize the influence of privacy concerns on trust and perceived risks. Therefore, the target population of this study is represented by emotionally stable Italian university students with low experience and awareness in privacy.
To discriminate the target population, Italian undergraduate students enrolled in Italian universities have been selected. The discrimination of the target population is further supported by the use of the control variables privacy awareness, privacy experience, emotional instability.
Participants were selected through a multi-stage process. Initially, a random sample of 1100 potential participants were drawn from a relevant population. The data collection lasted one month and was performed through one wave: the questionnaire was distributed electronically via mail to around 1100 undergraduate students. 306 surveys were completed and submitted, yielding a 27,8% response rate. The final sample size consisted of 245 individuals who met the inclusion criteria (i.e., full responses) and provided informed consent for participation.
The survey informing this research was conducted between November and December 2020, while Italy was experiencing the second intense wave of COVID-19 and six months after the contact tracing app Immuni was released.
Italy has been the first European country to be severely affected by COVID-19. The first cluster of the virus was identified in Northern Italy on February 21, 2020, and it spread to other regions within a few weeks.
The task to develop a contact tracking application was entrusted by the Italian State, through a “rapid call”, to a private corporation operating in the app-development sector. The revelation that the work was assigned to a private corporation sparked an extensive public discussion on the correctness of the choice, on data privacy and data ownership rights. The debate risen a widespread alarm about privacy infringement although no personal data are recorded and all data rights are recognized to the Italian State. The effect of the public debate on the efficacy of the app, both in terms of functionality and market penetration, has not been evaluated, although it is likely that it has been (e.g., choosing a Bluetooth proximity tracking system instead of a GPS based position tracking). At the beginning of June 2020 the app Immuni, based on casual codes assignment to each IP and Bluetooth proximity recognition was released. The app was downloaded by 19.8 percent of the Italian population. The penetration rate varied greatly throughout the regions of Italy, ranging from 30.1% in Valle D’Aosta to 12.5% in Campania.
Representativeness of participants compared to the overall population was checked through a comparison between the demographic characteristics of respondents and the entire population of university students in Italy.
Table 1 presents a comparison between the demographic characteristics (age and gender) of the respondents and the entire population of students enrolled at universities in Italy, based on data from the Ministero dell’Università e della Ricerca MIUR. The age distribution of the respondents closely resembles the distribution of the total population. Although there were slightly fewer respondents in the age groups of 15–19 and 26–30, the majority of respondents fall within the 19–26 age range. Notably, 96.5% of the sampled individuals were from the largest represented age group, indicating a good representation of the sample compared to the entire population.
Table 1. Age and gender distribution of selected sample and overall population (frequency and percentage)

Regarding gender distribution, the sample aligns well with the overall population of university students in Italy. The sample is almost evenly balanced in terms of female and male respondents, with 49% and 51%, respectively, which is consistent with the gender distribution in the entire student population (44% male students and 56% female students).
In conclusion, the demographic characteristics of the respondents in the study closely mirror those of the overall population of university students in Italy, indicating a good level of representativeness and reliability of the sample for analysis and drawing meaningful conclusions, at least when examining demographic characteristics.
4. Data Analysis and Results
Partial least squares (PLS), a second-generation causal modeling statical technique, was used for the analysis of data. Providing the possibility of simultaneously testing the measurement model and the structural model, PLS enables a complete analysis of interrelationship between variables in the model. In order to ensure reliability of measurements and discriminant validity of the constructs we first assessed the quality of the measurement model checking whether requirements or individual item reliability, internal consistency and discriminant validity were fulfilled. Following the assessment of the measurement model, the research hypothesis as well as the overall quality of the model were tested using structural modeling.
4.1. Measurement model
First, individual item reliability, internal consistency and discriminant validity of the developed scales were analyzed to assess the measurement model (Barclay et al., Reference Barclay, Thompson and Higgins1995). Content validity was already addressed in the questionnaire development, as measures were based on previous research studies in order to ensure validity of results.
To evaluate the reliability of single items and confirm the relations among the observed variables and the latent constructs, that is, trust, perceived benefits, perceived risks, intentional reaction, a confirmatory factor analysis (CFA) has been performed (Table 2). Hair et al. (Reference Hair, Ringle and Sarstedt2011) recommended accepting items with a loading factor equal or higher than 0.5. Loading the single items on their respective constructs, all the loadings are considerably above 0.5, except for one of the trust items. The relatively low reliability of this item is due to the fact that trust is defined by three different factors, that is, trust ability, trust benevolence, trust integrity, each one described by one item. Given the loading factors of the other two items, it was deemed acceptable. Hence, reliability of the items included in the model was validated, and the measurement model adopted is consistent with our understanding of the nature of the constructs.
Table 2. Loading and cross loadings of the constructs and their items. Bolded numbers are the factor loadings, otherwise cross-loading

Discriminant validity refers to the degree to which measures of different constructs are distinct (Campbell and Fiske, Reference Campbell and Fiske1959). The assessment of discriminant validity can be verified ensuring that the items loaded more highly on the intended constructs than any other constructs and that each item correlate more highly with other items measuring the same constructs than with items measuring other constructs (Chin, Reference Chin1998). First, the CFA showed that cross loadings were lower than the loadings of each construct (Table 3). Second, the analysis of the square root of the average variance extracted (AVE) between a construct and its items revealed that all constructs share more variance with their indicators than with other constructs (Fornell and Larcker, Reference Fornell and Larcker1981). Table 3 shows on the diagonal the square roots of the AVEs demonstrating that these values are all higher than the correlations. Hence, the requirement of discriminant validity for the items of the study are met.
Table 3. Internal consistency and discriminant validity of constructs

The reliability of the developed scales has been tested for their internal consistency by analyzing the covariance among all the items used to measure the same construct and the Cronbach’s alpha. These two indicators enable to assess how well the items used for one construct correlate or move together (Straub and Gefen, Reference Straub and Gefen2004). Cronbach’s alpha and composite reliability are two indicators that measures internal reliability among the items used for one construct, but composite reliability is considered more rigorous in the context of structural equation modeling (Chin, Reference Chin1998). The overall Cronbach’s alpha is 0.73, while composite reliability of single items are shown in Table 3. All the measures of composite reliability are close to the recommended minimum value of 0.60 (Fornell and Larcker, Reference Fornell and Larcker1981).
4.2. Data analysis
Following the assessment of the validity of the measures, the developed hypothesis were tested analyzing the sign and significance of the path coefficients in the structural model. The structural model was evaluated conducting the statistical tests at 5% level of significance and including the control variables (i.e., privacy awareness, privacy experience, emotional instability in our study). Control variables had no statistically significant effect on the endogenous construct of the study. In order to assess the predictive validity of the model we evaluated the explanatory power of the model and the significance of the relationship that were hypothesized. The explanatory power of the overall model is estimated on the basis of the amount of variance explained in the dependent variable of the study, that is, the intention to disclose personal location data. The structural model is shown in Figure 3.

Figure 3. Structural model.
The structural model explained 46.2 percent of the variance in intention to disclose personal tracking data. As shown in Figure 3, the university student’s trust in public institutions (b = 0.319; p = 0.007), the perceived benefits (b = 0.496; p = 0.000) and the perceived risk (b = -0.452; p = 0.000) are significant predictor of the intention to disclose personal tracking data, thus supporting H1, H2 and H3. However, the analysis of the goodness of fit of the model revealed that the perceived benefits and perceived risk can explain more than 35% of the variance of the dependent variable (35.3% and 43%, respectively), while university student’s trust in public institutions was able to explain only 16% of the variance of intention to disclose personal tracking data.
As hypothesized, the role of trust on the perceived benefits and the perceived risk were confirmed by the results of the analysis. University student’s trust in public institutions, indeed, positively influence the perception of benefits of sharing tracking data (b = 0.245; p = 0.016; level of significance 10%) and negatively influence the perceived risk (b = –0.526; p = 0.000) in sharing personal tracking data. Hence, H4 and H5 were validated by the empirical analysis. The influence of perceived risk in disclosing personal tracking data on the perceived benefits deriving from sharing personal tracking data was also confirmed by the analysis (b = –0.199; p = 0.000), thus validating H6.
In order gather further insights on the interdependence between the investigated variable, we conducted post hoc analysis to investigate the potential mediating effect of perceived benefits and perceived risk in the relationship between trust, perceived risk and the intention of sharing personal tracking data. Mediation effect refers to the influence of one variable in mediating the relationship between the independent variable and the dependent variable. The investigation of mediation effects is therefore useful to estimate the importance of the direct impact that the independent variable has on the dependent variable and the importance of the indirect impact the same independent variable has by impacting another variable.
Following the procedure proposed by Baron and Kenny, we verified that the three conditions were fulfilled (Baron and Kenny, Reference Baron and Kenny1986): (i) the independent variable has a statistically significant effect on the mediator, (ii) the independent variable significantly affect the dependent variable, and (iii) the relationship between the independent and the dependent variable is weaker when the proposed mediator is in the model than when the proposed mediator is not included in the equation.
As the first two conditions has been shown to be proved by testing the developed hypothesis, we postulated that perceived benefit mediates the effect of trust and risk on the dependent variable and that risk mediates the effect of trust on the dependent variable.
Results in Table 4 shows:
-
- the mediating role of the perceived benefits on the relationships between university students trust on public institutions and the intention to share tracking data;
-
- The mediating role of the perceived benefits between the perceived risk and the intention to share tracking data;
-
- the mediating role of perceived risk in the relationship between university students’ trust in public institutions and the intention to share tracking data.
Table 4. Mediating effects

Results show that, as well as directly influencing intention to share tracking data, the perception of benefits deriving from the sharing of personal location data positively mediates the effect of trust on the intention to share personal tracking data (p = 0.000) and negatively mediates the effect of perceived risk on the intention to disclose personal tracking data (p = 0.008). The negative effect of perceived risk on the relationship between the university students’ trust on public institutions and the intention to disclose personal tracking data was also confirmed by the analysis of mediating effects (p = 0.000)
The examination of the variance accounted for (VAF) of the mediating variable revealed that VAF is greater than 20%, hence partial mediation was confirmed in the three investigated relationships (Hair et al., Reference Hair, Ringle and Sarstedt2011).
5. Discussion and Conclusions
The aim of this study was to examine the factors that influence individuals’ willingness to share their personal location data during the COVID-19 pandemic. While numerous studies have explored the factors influencing the adoption of contact tracing apps during this recent health crisis, limited attention has been devoted to understanding individuals’ intentions regarding the sharing of personal location data with public institutions for health emergency management.
Drawing on previous research studies on Privacy Calculus theory and the perspective of the TRA we hypothesized that the university students’ trust in public institutions, the perceived risk and the perceived benefits influence the intention of university students of sharing personal location information with public institutions. The developed hypothesis were empirically tested through a structural model which is able to account for the 46.2% of the variance in the dependent variable, hence proving goodness of fit in terms of explanatory power for the interpretation of results. Providing insights on the determinants of location data sharing with public institutions during the COVID-19 health emergency, the empirical analysis contributes to the privacy calculus theory by confirming the validity of the hypothesis put forward by authors in the context of the outbreak.
Coherently with the results of recent studies investigating determinants of adoption of contact tracing activities (Altmann et al., Reference Altmann, Milsom, Zillessen, Blasone, Gerdon, Bach, Kreuter, Nosenzo, Toussaert and Abeler2020; Oldeweme et al., Reference Oldeweme, Märtins, Westmattelmann and Schewe2021; von Wyl et al., Reference von Wyl, Höglinger, Sieber, Kaufmann, Moser, Serra-Burriel, Ballouz, Menges, Frei and Puhan2021), this study revealed the relevance of trust in public institutions as a factor influencing the willingness of university students to share their personal tracking data. The study adds to the empirical research on the topic insights on the role of the risk perceived in terms of loss of control of their data and their consequences and the perceived benefits of sharing personal location data for managing and controlling the health emergency. Results show that both perceived risk and perceived benefits determine the willingness of university students to share personal tracking data during COVID-19. Notably, the comparison of the explanation power of the three constructs on the dependent variable revealed that perceived benefits and perceived risk explain, respectively, 35.3% and 43% of the variance of the intention to share personal location data, more than trust on institutions which accounts for the 16%. The high level of explanation in the dependent variable variance provides insight on the relevance of the perception of the trade-off between potential risks and benefits when decisions on sharing personal data are made.
Furthermore, by analyzing direct and indirect effects of determinants on the dependent variables it was possible to investigate the mediation effects of factors by evaluating the strengths of these paths. The analysis of the interdependencies between the antecedents of the intention to share personal tracking data revealed that university students trust in public institutions influences the perceived benefits and perceived risks, hence having an indirect effect on the willingness of sharing location data that is mediated by the perception of the benefits and risk associated to the loss of control of personal data. Moreover, the analysis of mediation effects revealed that the perceived benefits deriving from sharing location data during emergency situations mediate the impact of perceived risk on the dependent variable of the study.
In reading the results of the study it is important to acknowledge the influence of cultural and demographic characteristics on them. We focused indeed the study on Italian university students as the target population to achieve consistency within the sample, offering insights into the determinants of location data sharing for this specific sub-population. However, it’s crucial to recognize that these results might not be fully applicable to other sub-population groups due to the potential impact of demographic and cultural differences on data sharing behavior. Demographic attributes like age and education level can indeed significantly influence attitudes and concerns towards data and digital technologies. With regards to our target population, a Special Eurobarometer 518 (2021) conducted in 2021 revealed that younger individuals in Europe tend to expect more advantages than disadvantages from digital tools. The expectation on potential benefits deriving from these tools decreases as the respondents age increases. While age seems not to influence the concern over loss of control of data and consequence such as cyber-attacks or thefts or abuse of personal data; younger generation groups have, however, higher expectations on the improved cybersecurity and safety of digital technologies (Special Eurobarometer 532, 2023). With regards to the education level, concerns about loss of control of data tend to increase with higher level of education. Individuals with higher education are more likely to feel worried about potential cyber-attacks and other forms of data abuse compared to those who have interrupted their studies. This observation underscores the correlation between educational attainment and awareness of potential risks associated with data sharing.
Additionally, to well interpret the results, it’s important to consider the impact of the COVID-19 pandemic on the research’s target group. Being university students particularly exposed to the consequences of the pandemic, they might have experienced higher levels of perceived benefits from data sharing compared to other population subgroups or countries. Furthermore, it’s worth noting that higher levels of education have been associated with a higher degree of trust in government management of the COVID-19 situation (Rieger and Wang, Reference Rieger and Wang2022). As university students are positioned at the second highest level of education, this educational background might have positively influenced their trust levels overall.
Cultural characteristics are also critical factors to take into account when interpreting results, since they influence data sharing behavior across countries. Attitudes toward governmental data usage can significantly vary across countries and cultural contexts, impacting individuals’ willingness to share their personal information. For example, in China, a positive attitude toward governmental data use has been observed, whereas US citizens tend to be more reserved about governmental big data analytics. Such country-specific attitudes stem from varying cultural norms, historical factors, and perceptions of data privacy and security (Labrie et al., Reference LaBrie, Steinke, Li and Cazier2018).
Similarly, differences in data sharing behaviors can be observed across European countries. A country analysis has revealed that Italy’s expectations regarding the future importance of digital technologies differ from those of other European nations, with Italy standing out as a country with comparatively lower expectations concerning the advantages offered by digital technologies when compared to other European countries (Eurobarometer 2021). Moreover, as one of the most affected countries during the first wave of the pandemic, Italy endured significant loss of life and implemented stringent lockdown measures. The severity of these measures to limit the virus’s spread could have heightened the perceived benefits of data sharing for public health reasons.
The intricate interplay of factors like age, education, cultural characteristics create a dynamic landscape where the impact of trust, perceived benefits, and perceived risks on data sharing behaviors can be highly context dependent. The relative importance of these determinants could, indeed, be influenced by the specific attitude and concern of the examined population, Therefore, we refrain from making broad generalizations beyond the specific population and circumstances under study. In light of this, further research is paramount to comprehensively explore the attitudes and concerns related to data sharing across different generations and cultural settings.
From a practical perspective, this study has implications for public institutions and governmental agencies in their attempts to incentivize the disclosure on personal tracking data.
As the lack of trust in public institutions such as governments or Ministry of Health negatively impact on the willingness of individual to share their data, policy makers, especially in those countries with limited trust in public institutions, could consider delegating control of data to organizations that are considered as more trustworthy and reliable by citizens (e.g., university, non-profit organizations either local or international). It is worth to highlight the importance of selecting trusted intermediaries that are required to preserve privacy and security of individuals and maximize public value of data (Mulgan and Straub, Reference Mulgan and Straub2019). The involvement of organizations related to for-profit firms or big tech organizations could indeed lower the trust of citizens, hence hindering the success of such initiatives. An example is the Toronto Sidewalk project at the center of controversies for the involvement of Sidewalk Labs, a subsidiary of Google parent company Alphabet, raising concerns over privacy and personal information security (Artyushina, Reference Artyushina2020). The same considerations hold true for younger generations. Indeed, research demonstrated the negative effects of commercial companies’ engagement on the level of trust in data sharing especially (RockHealth, 2018; Ghafur et al., Reference Ghafur, Van Dael, Leis, Darzi and Sheikh2020) subsequently to some major scandals as the one of Cambridge AnalyticaFootnote 1. In this regard therefore we call for commercial companies’ limited involvement and when necessary, to proactively communicate the scope and boundaries of their involvement, especially their rights and access to data.
The innovativeness of contemporary analyzing the three constructs in the pandemic context, revealed that the effect of perceived benefits and perceived risk on the intention to share location data, is higher than trust, This finding invites policy makers to focus their efforts on strengthening communication strategies to show the potential benefits deriving from the sharing of location data, hence increasing the perceived usefulness. On the other side, it invites to put in place actions to reduce the effective and perceived risk connected to data sharing. Actions contributing to increasing trust and reducing perceived risks, could be developed both on the levels of engagement and technology. Including informed citizens representatives in the design and implementation of technological solutions to emergency situations may, for instance, decrease the perceived risk (Aggarwal et al., Reference Aggarwal, Farag, Martin, Ashrafian and Darzi2021). In this regard, the establishment of a trustworthy oversight committee, including citizens representatives, in charge of controlling the service, might be a potential option to reducing the probability that loss of personal data or their misuse occur. To build this shared sense of trust, institutions may act along different lines of action, among them we recommend creating awareness about EU’s initiatives on infrastructures and standards for data sharing (e.g., INSPIRE, GEOSS) and include informed citizens representatives in the design and implementation of data driven applications for emergency situations. On the contrary, the use of decentralized technologies may help in reducing the perceived risks connected to data and privacy violations (Sandvik, Reference Sandvik2020; Naudé and Cameron, Reference Naudé and Cameron2021). For instance, while developing the UK initial contact-tracing app based on a centralized schema, the NHS had to abandon its development and replace it with a decentralized solution (Naudé and Vinuesa, Reference Naudé and Vinuesa2021). Young adults, indeed, extensively use digital resources to access health information (Ghafur et al., Reference Ghafur, Van Dael, Leis, Darzi and Sheikh2020) and trust distributed technologies more than centralized solutions (Tenopir et al., Reference Tenopir, Dalton, Allard, Frame, Pjesivac, Birch, Pollock and Dorsett2015).
5.1. Limitations and future research
The study provides relevant insights on the determinants of location data sharing during COVID-19 health emergency; however, results can be strengthened by further research. First, based on the TRA theory, the model assumes that behavioral reaction is comparable to the intention of university students in disclosing their personal information with other parties, hence assuming that the intention would be translated into actual sharing behavior. Authors encourage the development of further empirical studies finalized to confirm this relation in pandemic contexts. Second, generalizability of results to other geographical contexts and actors (beyond public institutions) should be verified. We also acknowledge the research’s limitation given by the limited representativeness of the university student’s group we have surveyed respect to the whole Italian population, we therefore encourage further replications of the study involving citizens with different cultural, demographic, personality and privacy awareness and experience levels. These studies could confirm or challenge our findings and therefore generate new insights on the topic. Cultural differences and external context factors, including institutional factors and characteristics, may provide greater explanatory power if included in the structural model. Further confirmatory analysis on this will be beneficial to the research field development.
Supplementary material
The supplementary material for this article can be found at https://doi.org/10.1017/dap.2023.42.
Acknowledgments
The authors thank the anonymous referee who provided useful and detailed comments on a previous/earlier version of the manuscript made available for the Data for Policy 2022 Conference.
Author contributions
Conceptualization: F.B., G.A., V.M.U.; Data curation: F.B., V.M.U.; Formal analysis: F.B., V.M.U.; Investigation: F.B., V.M.U.; Methodology: F.B., G.A., V.M.U.; Writing – original draft: F.B., G.A., V.M.U.; Writing – review & editing: F.B., G.A., V.M.U.
Data availability statement
The data that support the findings of this study are available in the Zenodo platform at the following link.
Funding statement
This work received no specific grant from any funding agency, commercial or not-for-profit sectors.
Competing interest
The authors declare none.
Comments
No Comments have been published for this article.