- 1School of Mental Health, Wenzhou Medical University, Wenzhou, China
- 2School of Public Health and Management, Wenzhou Medical University, Wenzhou, China
- 3Center for Health Behaviours Research, Faculty of Medicine, JC School of Public Health and Primary Care, The Chinese University of Hong Kong, Hong Kong, Hong Kong SAR, China
- 4Renji College, Wenzhou Medical University, Wenzhou, China
- 5Wenzhou Medical University, Wenzhou, China
- 6The Affiliated Kangning Hospital, Wenzhou Medical University, Wenzhou, China
- 7Key Laboratory of Alzheimer's Disease of Zhejiang (P)rovince, Institute of Aging, Wenzhou Medical University, Wenzhou, China
Background and Aims: This three-wave longitudinal study investigated the effects of stressful life events on problematic smartphone use and the mediating roles of mental health problems (i.e., depressive symptoms, poor sleep quality, and suicidal ideation) in Chinese undergraduate students.
Methods: A total of 197 undergraduate students completed the three-wave surveys. Their severity of stressful life events, mental health problems, and problematic smartphone use were assessed.
Results: Regression analyses revealed that stressful life events at T1 was significantly associated with problematic smartphone use at T3. Mediation analyses showed that mental health problems (i.e., depressive symptoms, poor sleep quality, and suicidal ideation) at T2 fully mediated the association between stressful life events at T1 and problematic smartphone use at T3 (B = 0.042, 0.034, and 0.022, respectively).
Conclusions: The present study revealed that stressful life events and mental health problems (i.e., depressive symptoms, poor sleep quality, and suicidal ideation) are predictors of problematic smartphone use in Chinese college students.
Introduction
Mobile media use has become a necessity of life around the world. Mobile phones expedite communication without restrictions due to physical contiguity or spatial fixation and empower users to perform a variety of online activities, like online e-meeting, online gaming, and other online services (1–3). In the past decade, the frequency of smartphone use among young people in Asia and Europe has increased dramatically (4). For example, as of July 2020, there were about 932 million smartphone users in China, of which 23.7 percent were students (5). College students are generally highly motivated to use smartphones and update them quickly, and they are also the fastest adopters and users of new applications and new programs (6). Recent researches show that college students generally own smartphones (7, 8), excessive use of a smartphone can lead to problematic smartphone use (9, 10).
Problematic smartphone use (PSU) refers to the excessive use of the smartphone or smartphone addiction in daily life, accompanied by dysfunction and symptoms similar to substance use disorder (11). PSU severity is associated with a wide variety of indicators, such as stress (10, 12, 13), symptoms of depression (12, 14, 15), poor sleep quality (15, 16), and suicidal ideation (17). However, most of this research is based on cross-sectional studies, with only a few longitudinal studies examining risk or protective factors predicting PSU symptoms over time. Given the negative consequences of PSU, it is crucial to explore the possible psychological mechanisms of PSU symptoms utilizing longitudinal designs.
Stressful life events refer to the things that compel people to make changes in their ongoing life patterns (18), such as study pressure, and interpersonal tension (19). Stress has been well-documented as a risk element in the development of addiction and the vulnerability to relapse (10, 12, 20), and stressful life events have also been considered important factors potentially contributing to the development of PSU (13). Some authors conceptualize PSU as dysfunctional coping with everyday life (10, 13). Smartphone use has become a convenient and popular means for stress relief, entertainment, and social connection in young people (8). Empirical studies revealed that stressful life events were positively correlated with problematic or addictive online behaviors, such as online gaming addiction, Internet addiction, and problematic smartphone use (13, 21, 22). Longitudinal studies have also demonstrated that problematic smartphone use can be predicted by stressful life events (13, 23).
Undergraduate students are at an important transitional stage and may face various new challenges, such as adjustment to college life, poverty, academic pressures, part-time work, and identity changes (24–26). These stressors might increase the risk of stress-induced mental health problems in undergraduate students, such as depression (27), poor sleep quality (28, 29), and suicidal ideation (30). There is good evidence that one of the most important risk factors for mental health problems is stressful life events (31, 32).
According to the Diathesis Stress Model of Depression, depression may be the result of the combined effect of stress and individual susceptibility to diathesis (33). Several studies have confirmed that there was a positive correlation between stressful life events and depression (34, 35), stressful life events is a risk factor for depression (36), and have a direct impact on individuals' depressive symptoms (37, 38). Longitudinal findings have also revealed that stressful life events can predict depression symptoms over time (13, 39).
Stressful life events may also reduce poor sleep quality. Sterling and Eyer (40) put forward the concept of the “unsteady state,” whereby an organism's stress response is the process of reaching a steady-state again. However, in the case of severe and prolonged stress, this process will gradually become disordered and collapse, leading to an “unsteady state.” Stress triggers the body's physical and mental responses, and these regulatory effects can affect sleep quality. Previous studies have demonstrated the positive association between stressful life events and poor sleep quality, including both cross-sectional studies (41) and longitudinal studies (28, 29).
Suicidal ideation refers to thoughts about suicide and serious self-injury behaviors; it is the pre-clinical stage of suicide and can predict suicide behaviors (42). The stress theory of suicide proposes that long-term stress or coping failure can also cause suicidal ideation or behavior; stressful life events are the most common stressors of suicidal ideation (43–45). People with suicidal ideation have often experienced major stressful, negative, or traumatic sexual events (46), especially in adolescents (44). Longitudinal research has also shown that stressful life events significantly predicted suicidal ideation (30).
Nowadays, the smartphone's small size and portability enable persons to constantly access online (and offline) content, potentially causing overuse (47). Individuals who experience affective mental health problems (such as depression, poor sleep quality, and suicidal ideation) are more likely to increase smartphone use in daily life (48, 49). Therefore, mental health problems are considered as the influential risk factors for PSU (11, 49, 50). Associations between mental health problems and PSU have been evaluated through several studies. For instance, a systematic review and meta-analysis has found that mental health problems were significantly linked to PSU (51). Some studies have also suggested that PSU can be predicted by depression (11, 14), sleep problems (50), and suicidal ideation (49).
Theoretical Framework
The Interaction of Person-Affect-Cognition-Execution (I-PACE) model (52) presents a comprehensive theoretical framework that attempts to explain the mechanism of Internet-related overuse. According to the I-PACE model, predisposing characteristics are important for PSU, such as personality, mental health (e.g., anxiety and depression), genetics, and biology in general; Cognitive and affective consequences are important influences, such as coping styles, executive impairment, mood dysregulation, and internet-related cognitive bias. The I-PACE model assumes that these cognitive and affective consequences involve responses to predisposing characteristics and can lead to healthy enjoyment through technology or excessive use, including PSU (9). Empirical studies suggest that mental health problems such as depressive symptoms, sleep quality and suicidal ideation are products of stressful life events (28–30), and they can lead to PSU (11, 49, 50). Based on the I-PACE model (52) and existing literature, mental health problems (i.e., depressive symptoms, sleep quality, and suicidal ideation) may play mediating roles in the association between stressful life events and PSU.
The Present Study
The three-wave longitudinal surveys of Chinese undergraduates were used in this study. We hypothesized that stressful life events at T1 would positively predict PSU at T3 (Hypothesis 1). Figure 1 presents Hypotheses 1. Furthermore, we hypothesized that depressive symptoms at T2 would mediate the positive relationships between stressful life events at T1 and PSU at T3 (Hypothesis 2a), and also proposes sleep quality at T2 would mediate the relationship between stressful life events at T1 and PSU at T3 (Hypothesis 2b), as well as suicidal ideation at T2 would mediate the relationships between stressful life events at T1 and PSU at T3 (Hypothesis 2c). Figure 2 presents Hypotheses 2a, 2b, and 2c.
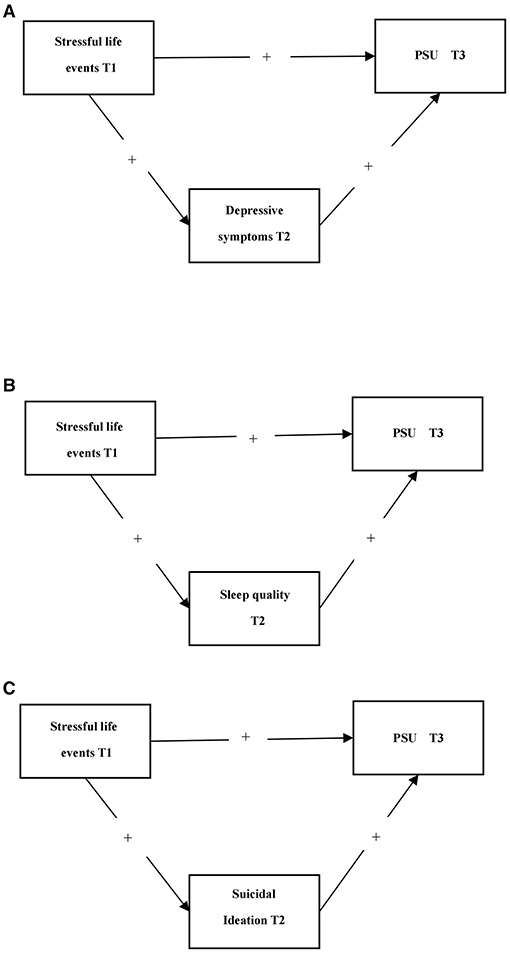
Figure 2. Visualization of Hypothesis 2: (A) Hypothesis 2a; (B) Hypothesis 2b; and (C) Hypothesis 2c.
Materials and Methods
Participants
Undergraduate students from Wenzhou Medical University in Wenzhou city, Zhejiang Province, China were the study samples. The inclusion criteria of this study were as follows: (1) willingness to participate in the baseline and follow-up surveys; and (2) having used a smartphone on an everyday basis in the past year. The study invited 219 students in four majors (i.e., Forensic Medicine, Stomatology, Anesthesia Medicine, and Traditional Chinese Medicine) to participate in the questionnaire surveys. A total of 212, 205, and 197 students completed the survey at baseline (T1; response rate: 96.8%), T2, and T3, respectively; 197 students completed all three waves of the survey. Of these 197 participants, 58.9% were female, 52.3% were from urban areas, 48.5% lived in the one-child family, and 58.6% spent more than 4 h a day on smartphones.
Materials
Adolescent Self-Rating Life Events Checklist
The 27-item Adolescent Self-Rating Life Events Checklist (19) was used to evaluate the intensity and frequency of stressful life events in undergraduate students in the past year. It is divided into six dimensions: learning pressure (e.g., heavy study burden), interpersonal relationship (e.g., discriminated against or ignored), punishment (e.g., criticized or punished), loss (e.g., friends and relatives died), health adaptation (e.g., being away from family for a long time), and other (e.g., a bad relationship or failure in love). Participants were told to recall whether such events had occurred during the preceding 12 months of their lives. If they answered “no, the score was 0; when answering “yes,” they were required to assess the impact of the stressful life event from 1 (not at all) to 5 (very much), with higher sum scores (0–135) representing more experiences of stressful life events. The good reliability and validity of this scale have been shown in previous studies (53, 54), as well as in the current study (Cronbach's α T1 = 0.91, α T2 = 0.94, α T3 = 0.94).
Mobile Phone Addiction Index Scale
The Mobile Phone Addiction Index Scale (55) contains 17 questions, get adapted to measure the PSU. Eight items of Young's Internet Addiction Diagnosis Questionnaire (56) were adapted to form eight items (i.e., items 3, 5, 6, 7, 8, 10, 15, and 16) of this scale. An example item is “When you are feeling down, you play with your smartphone to improve your mood.” Items are rated on a Likert scale ranging from 1 (never) to 5 (always), with higher sum scores representing higher levels of excessive smartphone use. The scale has been found to have good reliability and validity and to be suitable for assessing the excessive use of smartphones in Chinese populations (57). Reliability in the current study was good (Cronbach's α T1 = 0.85, α T2 = 0.88, α T3 = 0.91).
Center for Epidemiologic Studies Depression Scale
Depressive symptoms were assessed using the Chinese version of the 20-item Center for Epidemiologic Studies Depression (CESD) Scale (58) at T1, T2, and T3. The scale assesses the current severity of depressive symptoms, emphasis is placed on the emotional component of depression (59). The CESD can be used to indicate possible depressive symptoms, and its outcome score is significantly associated with clinical assessment results (59, 60). Furthermore, possible depressive symptoms has been found to predict depression diagnosis (61). Over the past 7 days, participants scored on a 4-point scale from 0 (little or none) to 3 (almost all of all) on how often they experienced symptoms such as restless sleep and feeling lonely. Reliability in the current sample was good (Cronbach's α T1 = 0.90, α T2 = 0.92, α T3 = 0.89).
Pittsburgh Sleep Quality Index
The 18-item Pittsburgh Sleep Quality Index revised by Liu (62) was used to evaluate the sleep quality of the subjects for nearly 1 month. The scale measures the following seven factors, each factor is scored on a scale from 0 to 3, and the total score of all seven factors is the total score of the scale. The higher the score, the poorer the quality of sleep. An example item is “Have you had enough energy to do anything in the past month?” Because this scale has good reliability and validity, it is used to measure the sleep quality of Chinese populations (63). Reliability in this current study was acceptable (Cronbach's α T1 = 0.68, α T2 = 0.71, α T3 = 0.69).
Self-Rating Idea of Suicide Scale
The 26-item Self-Rating Idea of Suicide Scale was used to evaluate the suicidal ideation of undergraduate students (64). The 26 questions are used to assess the four following factors: despair (12 questions), optimism (4 questions), sleep (4 questions), and concealment (5 questions). Each item is scored as “yes” or “no”; For positive items, the score is positive, with a “yes” score of 1 and a “no” score 0, whereas for negative items, the score is reversed, with a “no” score 1 and a “yes” score 0. An example item is “I often feel pessimistic and disappointed.” The scale has good reliability and validity and is suitable for measuring suicidal ideation in the Chinese population (65). Individuals with high levels of suicidal ideation scored high. If the masking factor (sometimes I also gossip about other people) score was ≥4, which indicates that the subject was unwilling, to tell the truth, the data were removed from the study. Reliability in this study was acceptable (Cronbach's α T1 = 0.67, α T2 = 0.72, α T3 = 0.71).
Procedure
The three-wave surveys were conducted at the end of each semester (December 2018/June 2019/January 2020) in the first year and the second year of college. All surveys were conducted in classroom settings. A well-trained and experienced research assistant explained to participants that their participation was voluntary, and refusal to participate would not result in any negative consequences. Data confidentiality was guaranteed and only the researchers could access the data. Student IDs were collected for data matching. Researchers were not able to access students' names or other identifying information. Participants who completed the study were paid 60 RMB (9.27 dollars).
Statistical Analyses
Statistical analyses were conducted with the Statistical Package for the Social Sciences 24 (SPSS, New York, NY, USA) and the PROCESS macro version 3.3 (www. processmacro.org/index.html) which the Process macro from SPSS. All variables of interest were normally distributed; the skewness and kurtosis of stressful life events, PSU and mental health problems (i.e., depressive symptoms, sleep quality, and suicidal ideation), fell within the acceptable range [i.e., skewness < |2.0| and kurtosis < |7.0|; (66)]. First, repeated-measures analyses of variance (within-subject ANOVAs) were applied to assess changes of the studied variables between T1, T2, and T3. Then, the correlations between all variables were investigated using zero-order bivariate correlations. Next, two-step hierarchical regression analyses (95% confidence intervals [CIs]) were performed to test the associations between independent variables and dependent variables. In the regression model, sex was included as a control variable in Step 1. In Step 2, stressful life events at T1 was entered as an independent variable, and PSU at T3 were entered as dependent variables (see Hypotheses 1). Finally, the mediation effects of mental health problems (i.e., depressive symptoms were tested using bootstrapping analyses (5,000 resamples) via Process (model 4; see Hypotheses 2a, 2b, and 2c) and 95% CIs are reported. Effect sizes (PM = the ratio of the indirect effect to the total effect) are also reported (67). Given the multiple testing, p-values were corrected by applying the Holm correction [level of significance: p < 0.05; (68)]. A priori power analyses (G*Power program, version 3.1) indicated that a sample size of at least N = 86 was required for valid results [Power >0.80, α = 0.05; (69)].
Results
We compared the characteristics of those who were followed up (n = 197) vs. those who were missing in the first and third follow-up surveys (n = 15). The two groups did not differ in socio-demographic characteristics (sex, age, and major) or the levels of the independent and dependent variables (p > 0.05).
As shown in Table 1, the levels of stressful life events, PSU, sleep quality, and suicidal ideation were significantly different. Namely, stressful life events and PSU were significantly lower at T2 and T3 than that at T1 (T2/T3 < T1) (F = 10.176, p < 0.05; F = 8.062, p < 0.001). Sleep quality and suicidal ideation were significantly lower at T1 and T2 than that at T3 (T1/T2 < T3) (F = 88.370, p < 0.001; F = 296.026, p < 0.001). The levels of depressive symptoms at T1, T2, and T3 were not significantly different (F = 0.063, p = 0.802).
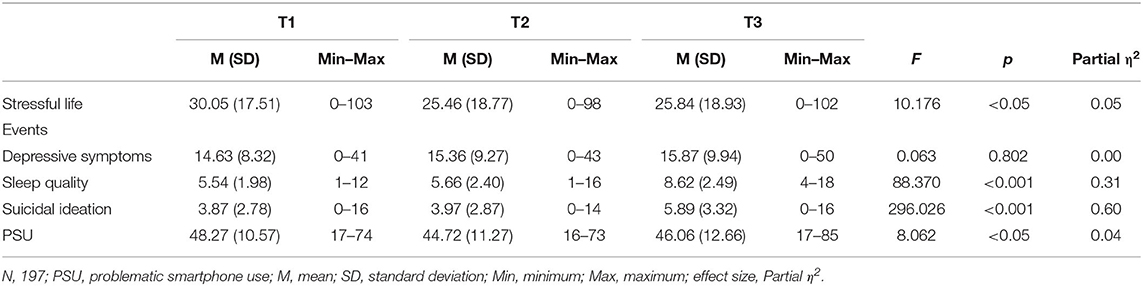
Table 1. Mean, SD, minimum, maximum, and repeated-measures ANOVAs (T1, T2, and T3) of all variables.
Table 2 shows the correlations between the investigated variables at T1, T2, and T3. Stressful life events at T1 were significantly and positively associated with depressive symptoms, sleep quality, and suicidal ideation at T2, as well as PSU at T3.
Regression analyses (Table 3) revealed that stressful life events at T1 was a significant predictor of the PSU at T3. These analyses revealed a predictive variance of 2.8% for PSU at T3.
Figure 3 shows the results of the mediation analyses. In the first model (Figure 3A), the total effect was significant (c: B = 0.168, p < 0.05), but the direct effect (c′: B = 0.126, p > 0.05) was not significant, which indicates that the depressive symptoms at T2 fully mediated the association between stressful life events at T1 and PSU at T3. The indirect effect (ab) was also significant (B = 0.042, 95% CI = [0.014, 0.098]; PM = 0.250); in the second model (Figure 3B), the total effect was significant (c: B = 0.168, p < 0.05), but the direct effect was not significant (c′: B = 0.134, p >0.05). This indicates that the sleep quality at T2 fully mediated the association between stressful life events at T1 and PSU at T3. The indirect effect (ab) was significant (B = 0.034, 95% CI = [0.003, 0.086]; PM = 0.202); In the third model (Figure 3C), the total effect was significant (c: B = 0.168, p < 0.05), but the direct effect was not significant (c′: B = 0.101, p > 0.05), which indicates that suicidal ideation at T2 fully mediated the association between stressful life events at T1 and PSU at T3. The indirect effect (ab) was significant (B = 0.067, 95% CI = [0.022, 0.124]; PM = 0.399).
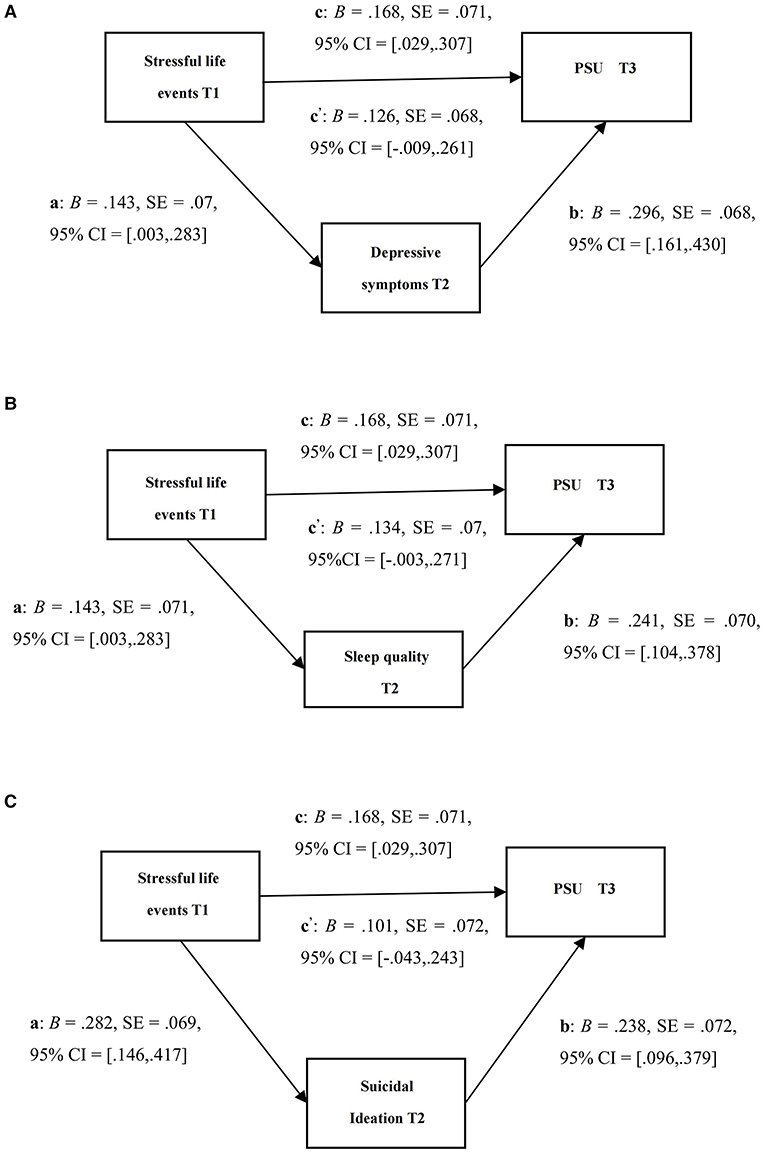
Figure 3. (A) The mediation model including stressful life events at T1, depressive system at T2, and PSU at T3; (B) The mediation model including stressful life events at T1, sleep quality at T2, and PSU at T3; (C) The mediation model including stressful life events at T1, suicidal ideation at T2, and PSU at T3. Note c: total effect, c′: direct effect; b: standardized regression coefficient, SE: standard error, CI: confidence interval.
Discussion
The present three-wave longitudinal research was designed to examine the possible mediating roles of mental health problems (i.e., depressive symptoms, sleep quality, and suicidal ideation) at T2 in the linkage between stressful life events at T1 and PSU at T3 in a sample of Chinese undergraduate students. The current study is the first longitudinal study to highlight the significant mediating roles of mental health problems as a potential underlying mechanism that can explain the association between stressful life events and PSU. The results support our hypotheses.
We found that the levels of depressive symptoms at T1, T2, and T3 were not significantly different, while the levels of stressful life events, PSU, sleep quality, and suicidal ideation significantly changed over time. Exactly, the levels of stressful life events and PSU at T2 and T3 were significantly lower than that at T1, while the levels of sleep quality and suicidal ideation at T1 and T2 were significantly lower than that at T3. It is plausible that as students adjusted to college life and gained more access to different coping resources over time, they experienced fewer new stressful life events or were less likely to consider some events as stressful, and relied less on smartphones to cope with stress (70). Sleep quality decreased and suicidal ideation increased over time, which may be because of the pressures associated with graduation, job hunting, and lifestyle change as graduation approaches (71–73).
We found that stressful life events at T1 significantly predicted PSU at T3. Thus, Hypotheses 1 was supported. This finding corroborates the results of both cross-sectional (21, 74) and longitudinal studies (13, 23). Furthermore, our result is consistent with the I-PACE model (52). Stress is a hazardous trigger for problematic smartphone usage because it may reduce positive affect and impulse control and increase withdrawal in actual life, leading to passive stress coping, such as spending a great amount of time online to avoid real-life stress (75). Studying in universities, especially in the medical education environment is perceived to be stressful, and students may experience stressful events such as psychosocial and academic stress (76). In college students, the strong connection between stressful life events and problematic smartphone usage suggests that decreased stressful life events may decrease the risk of problematic smartphone usage. Smartphone addiction is not established in the study, but given the frequency of PSU amongst young people and its significant association with symptoms of common mental disorders (51), the potential causative factors of PSU require urgent further exploration.
The direct association between stressful life events at T1 and PSU at T3 was not significant when the mediator depressive symptoms were inserted into the linkage, revealing that depressive symptoms fully mediated this relationship. The result is consistent with the Diathesis Stress Model of Depression (33) and the I-PACE model (52), and corroborates the prior research (13, 77, 78). This finding suggests that stressful life events might exacerbate the level of depressive symptoms (34), and in turn increase PSU levels (14, 79). One possible reason is that stressful life events experienced by college students may lead to depressive symptoms (34), and individuals with depressive symptoms may rely on surfing the internet to alleviate their negative emotions (14). Furthermore, we found that sleep quality at T2 fully mediated the association between stressful life events at T1 and PSU at T3, which is consistent with “unsteady state” (40) and expanded the I-PACE model (52). That is, individuals who experience stressful life events are more likely to unable to fall asleep on time, their sleep problems are more serious (29, 41), such as lack of sleep and have difficulty in sleeping. In turn, people who have sleep problems are more likely to use mobile phones to kill time or seek to satisfy sleep problems caused by the tired, anxious, and daytime dysfunction of negative feelings, making individuals more willing to focus on mobile phones and increasing the time spent using mobile phones, thus leading to PSU (50). Suicidal ideation at T2 fully mediated the association between stressful life events at T1 and PSU at T3, which is consistent with the stress theory of suicide (44) and enriched the I-PACE model (52). That is, stressful life events may lead to suicidal ideation (30), and the anonymity of the Internet and the diversity of information can help them seek social support to alleviate or eliminate suicidal ideation (80), but misuse or overuse of smartphone may lead to PSU.
In general, the results suggests that mental health problems (i.e., depressive symptoms, sleep quality, and suicidal ideation) are important mediators in the relationship between stressful life events and PSU. Previous studies have shown that different types of stressful life events have different effects on mental health problems. For example, some studies have found that interpersonal stress and academic stress are associated with depressive symptoms, sleep quality, suicidal ideation, and PSU (81–83); another study showed that punishment significantly predicted depressive symptoms, but have no effects on poor sleep quality and suicidal ideation (84). Future research should examine the effects of specific types of stressful life events on different mental health problems.
Implications
The results of this study have some important implications. First, these findings show that stressful life events may influence mental health problems in undergraduate students. Undergraduate students are faced with academic, lifestyle, and interpersonal pressures; thus, reducing the frequency and severity of stressful life events faced by undergraduate students early on could help to reduce future mental health problems (27, 29, 30, 34, 39). Second, by establishing mediation models, our findings can help practitioners understand the longitudinal relationship between stressful life events and PSU, and could aid the development of potential interventions. For example, implementing methods to enhance stress relaxation and adaptive stress coping skills, and increasing offline social support could help to reduce mental health problems and enhance sleep quality in undergraduate students, thereby reducing the negative effects of problematic smartphone use.
Limitations and Further Research
The limitations of this study are as follows. First, it may not be conducive to the generality of the results of this study due to the convenience of sampling, and future studies could use random sampling methods to obtain more objective results. Second, the self-reported measures may be biased by self-evaluation, and further research could collect data from multiple resources (e.g., peers, parents, and teachers) to validate the present findings. Third, PSU and suicidal ideation were not assessed using diagnostic tools, future studies could test our models with clinical samples (such as smartphone addiction or Internet addiction). Fourth, different stressful life events may have different influences on undergraduate students' mental health problems. Future research should investigate the influences of different sorts of stressful life events on undergraduate students' mental health problems. Fifth, the reliabilities of the Pittsburgh Sleep Quality Index (0.68, 0.71, and 0.69) and the Self-Rating Idea of Suicide Scale (0.67, 0.72, and 0.71) were relatively low. Sixth, SEM was not used in this study to test the longitudinal relationships, which should be considered in future studies. Finally, measurement invariance is not taken into account when comparing mean values, which needs to be taken into account in future studies.
Conclusion
The present study revealed that stressful life events and mental health problems (i.e., depressive symptoms, sleep quality, and suicidal ideation) are risk factors for PSU, and that mental health problems (i.e., depressive symptoms, sleep quality, and suicidal ideation) at T2 mediated the relationship between stressful life events at T1 and PSU at T3.
Data Availability Statement
The datasets in the study are available from the corresponding author on reasonable request. Requests to access these datasets should be directed to zghcnu@wmu.edu.cn.
Ethics Statement
The studies involving human participants were reviewed and approved by the Ethics Committee of the Wenzhou Medical University. The patients/participants provided their written informed consent to participate in this study.
Author Contributions
XY and GZ prepared the study concept and design. CZ wrote the main manuscript text, analyzed the data, and edited the draft. HX, XL, XT, and ND conducted investigation and data curation. YL, DX, and GZ provided funds to conduct the study. All authors had full access to all data in the study, and take responsibility for the integrity of the data and the accuracy of the data analysis. All authors have approved the final version of the manuscript.
Funding
This research was supported by the Social Science Foundation of China (BIA170166).
Conflict of Interest
The authors declare that the research was conducted in the absence of any commercial or financial relationships that could be construed as a potential conflict of interest.
Publisher's Note
All claims expressed in this article are solely those of the authors and do not necessarily represent those of their affiliated organizations, or those of the publisher, the editors and the reviewers. Any product that may be evaluated in this article, or claim that may be made by its manufacturer, is not guaranteed or endorsed by the publisher.
References
1. Parnes P. Secure inclusion of phones into online e-meetings. In: Marshall A, Agoulmine N, editors. Management of Multimedia Networks and Services, Proceedings. Berlin: Springer (2003). p. 396–408.
2. Botta F, Moat HS, Preis T. Quantifying crowd size with mobile phone and Twitter data. R Soc Open Sci. (2015) 2:150162. doi: 10.1098/rsos.150162
3. Hong W, Liu R, Ding Y, Zhen R, Jiang R, Fu X. Autonomy need dissatisfaction in daily life and problematic mobile phone use: the mediating roles of boredom proneness and mobile phone gaming. Int J Environ Res Public Health. (2020) 17:5305. doi: 10.3390/ijerph17155305
4. Billieux J, Maurage P, Lopez-Fernandez O, Kuss DJ, Griffiths MD. Can disordered mobile phone use be considered a behavioral addiction? An update on current evidence and a comprehensive model for future research. Curr Addict Rep. (2015) 2:156–62. doi: 10.1007/s40429-015-0054-y
6. Qudah MFA, Albursan IS, Bakhiet SFA, Hassan EMAH, Alfnan AA, Aljomaa SS, et al. Smartphone addiction and its relationship with cyberbullying among university students. Int J Ment Health Addict. (2019) 17:628–43. doi: 10.1007/s11469-018-0013-7
7. Jun SK. The emotional factors influencing mobile phone dependency of college students and gender difference. J Fish Mar Sci Educ. (2019) 31:1193–200. doi: 10.13000/JFMSE.2019.8.31.4.1193
8. Zhang G, Yang X, Tu X, Ding N, Lau JTF. Prospective relationships between mobile phone dependence and mental health status among Chinese undergraduate students with college adjustment as a mediator. J Affect Disord. (2020) 260:498–505. doi: 10.1016/j.jad.2019.09.047
9. Elhai JD, Yang H, Fang J, Bai X, Hall BJ. Depression and anxiety symptoms are related to problematic smartphone use severity in Chinese young adults: fear of missing out as a mediator. Addict Behav. (2020) 101:105962. doi: 10.1016/j.addbeh.2019.04.020
10. Zhao C, Xu H, Lai X, Yang X, Tu X, Ding N, et al. Effects of online social support and perceived social support on the relationship between perceived stress and problematic smartphone usage among Chinese undergraduates. Psychol Res Behav Manag. (2021) 14:529–39. doi: 10.2147/prbm.S302551
11. Elhai JD, Levine JC, Hall BJ. The relationship between anxiety symptom severity and problematic smartphone use: a review of the literature and conceptual frameworks. J Anxiety Disord. (2019) 62:45–52. doi: 10.1016/j.janxdis.2018.11.005
12. Long J, Liu TQ, Liao YH, Qi C, He HY, Chen SB, et al. Prevalence and correlates of problematic smartphone use in a large random sample of Chinese undergraduates. BMC Psychiatry. (2016) 16:408. doi: 10.1186/s12888-016-1083-3
13. Xie JM, Zimmerman AD, Rost H, Yin X, Wang J. Stressful life events and problematic smartphone usage among Chinese boarding-school adolescents: a moderated mediation model of peer support and depressive symptoms. J Addict Res Theor. (2020) 28:493–500. doi: 10.1080/16066359.2019.1692824
14. Yuan G, Elhai JD, Hall BJ. The influence of depressive symptoms and fear of missing out on severity of problematic smartphone use and Internet gaming disorder among Chinese young adults: a three-wave mediation model. Addict Behav. (2021) 112:106648. doi: 10.1016/j.addbeh.2020.106648
15. Li M, Lu L. The influence of mobile phone addiction on left-behind middle school students' sleep quality: the mediator role of loneliness. Revista Argentina de Clinica Psicologica. (2017) 26:71. doi: 10.24205/03276716.2017.1006
16. Mohammadbeigi A, Absari R, Valizadeh F, Saadati M, Sharifimoghadam S, Ahmadi A, et al. Sleep quality in medical students; the impact of over-use of mobile cell-phone and social networks. J Res Health Sci. (2016) 16:46–50.
17. Kim HJ, Min JY, Kim HJ, Min KB. Association between psychological and self-assessed health status and smartphone overuse among Korean college students. J Ment Health. (2019) 28:11–6. doi: 10.1080/09638237.2017.1370641
18. Holmes TH, Rahe RH. The social readjustment rating scale. J Psychosom Res. (1967) 11:213–8. doi: 10.1016/0022-3999(67)90010-4
19. Liu X, Ma D, Liu L. Relationship between life events, coping styles and adolescent depression. Chin J Clin Psychol. (1997) 5:166–9.
20. Wei. Influence of Stress on Internet Addiction and its Mechanism. Wuhan: Central China Normal University (2014).
21. Liu Y, Ye B, Yang Q. The influence of stressful life events on college students' learning burnout: a chain-mediated effect analysis. Chin J Clin Psychol. (2019) 27:782–4. doi: 10.16128/j.cnki.1005-3611.2019.04.029
22. Li H, Zou Y, Wang J, Yang X. Role of stressful life events, avoidant coping styles, and neuroticism in online game addiction among college students: a moderated mediation model. Front Psychol. (2016) 7:1794. doi: 10.3389/fpsyg.2016.01794
23. Van Deursen AJ, Bolle CL, Hegner SM, Kommers PA. Modeling habitual and addictive smartphone behavior: the role of smartphone usage types, emotional intelligence, social stress, self-regulation, age, and gender. Comput Hum Behav. (2015) 45:411–20. doi: 10.1016/j.chb.2014.12.039
24. Acharya L, Jin L, Collins W. College life is stressful today - emerging stressors and depressive symptoms in college students. J Am Coll Health. (2018) 66:655–64. doi: 10.1080/07448481.2018.1451869
25. Holliday R, Anderson E, Williams R, Bird J, Matlock A, Ali S, et al. A pilot examination of differences in college adjustment stressors and depression and anxiety symptoms between white, Hispanic and white, non-Hispanic female college students. J Hispanic High Educ. (2016) 15:277–88. doi: 10.1177/1538192715607331
26. Hurst CS, Baranik LE, Daniel F. College student stressors: a review of the qualitative research. Stress Health. (2013) 29:275–85. doi: 10.1002/smi.2465
27. Eisenberg D, Hunt J, Speer N. Mental health in American colleges and universities: variation across student subgroups and across campuses. J Nervous Ment Dis. (2013) 201:60–7. doi: 10.1097/NMD.0b013e31827ab077
28. Pereira D, Elfering A. Social stressors at work, sleep quality and psychosomatic health complaints-a longitudinal ambulatory field study. Stress and Health. (2014) 30:43–52. doi: 10.1002/smi.2494
29. van der Schuur WA, Baumgartner SE, Sumter SR. Social media use, social media stress, and sleep: examining cross-sectional and longitudinal relationships in adolescents. Health Commun. (2019) 34:552–9. doi: 10.1080/10410236.2017.1422101
30. Xu Z, Müller M, Lay B, Oexle N, Drack T, Bleiker M, et al. Involuntary hospitalization, stigma stress and suicidality: a longitudinal study. Soc Psychiatry Psychiatr Epidemiol. (2018) 53:309–12. doi: 10.1007/s00127-018-1489-y
31. Nancy CL, Erika D, Erin O, Daniel R, Gisele C, Michael C, et al. Common stressful life events and difficulties are associated with mental health symptoms and substance use in young adolescents. BMC Psychiatry. (2012) 12:116. doi: 10.1186/1471-244X-12-116
32. Brailovskaia J, Rohmann E, Bierhoff HW, Margraf J, Köllner V. Relationships between addictive Facebook use, depressiveness, insomnia, and positive mental health in an inpatient sample: a German longitudinal study. J Behav Addict. (2019) 8:703–13. doi: 10.1556/2006.8.2019.63
33. Kessler RC. The effects of stressful life events on depression. Annu Rev Psychol. (1997) 48:191–214. doi: 10.1146/annurev.psych.48.1.191
34. Suzuki M, Furihata R, Konno C, Kaneita Y, Ohida T, Uchiyama M. Stressful events and coping strategies associated with symptoms of depression: a Japanese general population survey. J Affect Disord. (2018) 238:482–8. doi: 10.1016/j.jad.2018.06.024
35. Zuo B, Zhang X, Wen F, Zhao Y. The influence of stressful life events on depression among Chinese university students: multiple mediating roles of fatalism and core self-evaluations. J Affect Disord. (2020) 260:84–90. doi: 10.1016/j.jad.2019.08.083
36. Phillips AC, Carroll D, Der G. Negative life events and symptoms of depression and anxiety: stress causation and/or stress generation. Anxiety Stress Coping. (2015) 28:357–71. doi: 10.1080/10615806.2015.1005078
37. Lan T, Jia X, Lin D, Liu X. Stressful life events, depression, and non-suicidal self-injury among Chinese left-behind children: moderating effects of self-esteem. Front Psychiatry. (2019) 10:244. doi: 10.3389/fpsyt.2019.00244
38. Luo Y, Kong F, Niu G, Zhou Z. The influence of stressing events on depression in junior middle school students: the role of internet use motivation and internet use intensity. Psychol Dev Educ. (2017) 33:337–44. doi: 10.16187/j.cnki.issn1001-4918.2017.03.11
39. Yeon EM, Yoon HO, Choi H. Longitudinal relationship among marital conflict, parenting stress, and depression using autoregressive cross-lagged modeling. Korea Assoc Child Care Educ. (2015) 93:195–212. doi: 10.1017/S0954579408000096
40. Sterling P, Eyer J. Allostasis: a new paradigm to explain arousal pathology. In: Fisher JSR, editor. Handbook of Life Stress, Cognition and Health. New York, NY: John Wiley and Sons (1988). p. 629–49.
41. Bernert RA, Merrill KA, Braithwaite SR, Van Orden KA, Joiner TEJr. Family life stress and insomnia symptoms in a prospective evaluation of young adults. J Fam Psychol. (2007) 21:58–66. doi: 10.1037/0893-3200.21.1.58
42. Liu X, Tein J, Zhao Z, Sandler ZN. Suicidality and correlates among rural adolescents of China. J Adolesc Health. (2005) 37:443–51. doi: 10.1016/j.jadohealth.2004.08.027
43. Liu X, Kurita H, Uchiyama M, Okawa M, Liu L, Ma D. Life events, locus of control, and behavioral problems among Chinese adolescents. J Clin Psychol. (2000) 56:1565–77. doi: 10.1002/1097-4679(200012)56:12<1565::AID-7>3.0.CO;2-U
44. Xing X, Tao F, Wan Y, Xing C, Qi X, Hao J, et al. Family factors associated with suicide attempts among Chinese adolescent students: a national cross-sectional survey. J Adolesc Health. (2010) 46:592–9. doi: 10.1016/j.jadohealth.2009.12.006
45. Chen C, Yue H, Yang S. The role of stressful life events, self-esteem, and depression in the formation of suicidal ideation. Chin J Clin Psychol. (2010) 18:190–1. doi: 10.16128/j.cnki.1005-3611.2010.02.026
46. Kraaij V, Arensman E, Spinhoven P. Negative life events and depression in elderly persons: a meta-analysis. J Gerontol Ser B. (2002) 57:P87–94. doi: 10.1093/geronb/57.1.P87
47. Elhai JD, Dvorak RD, Levine JC, Hall BJ. Problematic smartphone use: a conceptual overview and systematic review of relations with anxiety and depression psychopathology. J Affect Disord. (2017) 207:251–9. doi: 10.1016/j.jad.2016.08.030
48. Kardefelt-Winther D. Problematizing excessive online gaming and its psychological predictors. Comput Human Behav. (2014) 31:118–22. doi: 10.1016/j.chb.2013.10.017
49. Shen Y, Wang L, Huang C, Guo J, De Leon SA, Lu J, Luo Z, et al. Sex differences in prevalence, risk factors and clinical correlates of internet addiction among chinese college students. J Affect Dis. (2021) 279: 680–6. doi: 10.1016/j.jad.2020.10.054
50. Kang Y, Liu S, Yang L, Xu B, Lin L, Xie L, et al. Testing the bidirectional associations of mobile phone addiction behaviors with mental distress, sleep disturbances, and sleep patterns: a one-year prospective study among Chinese college students. Front Psychiatry. (2020) 11:634. doi: 10.3389/fpsyt.2020.00634
51. Sohn SY, Rees P, Wildridge B, Kalk NJ, Carter B. Prevalence of problematic smartphone usage and associated mental health outcomes amongst children and young people: a systematic review, meta-analysis and GRADE of the evidence. BMC Psychiatry. (2019) 19:356. doi: 10.1186/s12888-019-2350-x
52. Brand M, Young KS, Laier C, Wölfling K, Potenza MN. Integrating psychological and neurobiological considerations regarding the development and maintenance of specific Internet-use disorders: an Interaction of Person-Affect-Cognition-Execution (I-PACE) model. Neurosci Biobehav Rev. (2016) 71:252–66. doi: 10.1016/j.neubiorev.2016.08.033
53. Jin C, Zou H. The relationship between crime and life events, peer positive influence, and problem behavior in general adolescents: dual moderating effects. Chin J Clin Psychol. (2015) 23:88–92. doi: 10.16128/j.cnki.1005-3611.2015.01.020
54. Chen L, Yan Z, Tang W, Yang F, Xie X, He J. Mobile phone addiction levels and negative emotions among Chinese young adults: the mediating role of interpersonal problems. Comput Hum Behav. (2016) 55:856–66. doi: 10.1016/j.chb.2015.10.030
55. Leung. Linking psychological attributes to addiction and improper use of the among adolescents in Hong Kong. J Child Med. (2008) 2:93–113. doi: 10.1080/17482790802078565
56. Young KS. Internet addiction: the emergence of a new clinical disorder. Cyberpsychol Behav. (1998) 1:237–44. doi: 10.1089/cpb.1998.1.237
57. Huang Y, Wu R, Wu J, Yang Q, Zheng S, Wu K. Psychological resilience, self-acceptance, perceived social support and their associations with mental health of incarcerated offenders in China. Asian J Psychiatr. (2020) 52:102166. doi: 10.1016/j.ajp.2020.102166
58. Cheung C-K, Bagley C. Validating an American scale in Hong Kong: the Center for Epidemiological Studies Depression Scale (CES-D). J Psychol. (1998) 132:169–86. doi: 10.1080/00223989809599157
59. Radloff LS. The CES-D scale: a self-report depression scale for research in the general population. Appl Psychol Meas. (1977) 1:385–401. doi: 10.1177/014662167700100306
60. Amtmann D, Kim J, Chung H, Bamer AM, Askew RL, Wu S, et al. Comparing CESD-10, PHQ-9, and PROMIS depression instruments in individuals with multiple sclerosis. Rehabil Psychol. (2014) 59:220–9. doi: 10.1037/a0035919
61. Björgvinsson TJ, Kertz S, Bigda-Peyton JS, McCoy KL, Aderka IM. Psychometric properties of the CES-D-10 in a psychiatric sample. Assessment. (2013) 20:429–436. doi: 10.1177/1073191113481998
62. Liu X. Reliability and validity of the Pittsburgh Sleep Quality Index. Chin J Psychiatry. (1996) 29:103–7.
63. Lu T, Li Y, Xia P, Zhang G, Wu D. Reliability and validity analysis of Pittsburgh sleep quality index. Chongqing Med. (2014) 43:260–3. doi: 10.3969/j.issn.1671-8348.2014.03.002
64. Xia C, Wang D, Wu S. Preliminary development of self-rating scale for suicidal ideation. J Clin Psychiatry. (2002) 12:100–2. doi: 10.3969/j.issn.1005-3220.2002.02.030
65. Hu Y, Fan F, Dai Y, Cui N, Zhao X. College students' life events and suicidal ideation: the mediating and moderating role of life values. Chin J Clin Psychol. (2016) 24:149–51. doi: 10.16128/j.cnki.1005-3611.2016.01.034
66. Hancock GR, Mueller RO, Stapleton LM. The Reviewer's Guide to Quantitative Methods in the Social Sciences. New York, NY: Routledge (2010).
67. Wen Z, Fan X. Monotonicity of effect sizes: questioning kappa-squared as mediation effect size measure. Psychol Methods. (2015) 20:193–203. doi: 10.1037/met0000029
69. Susanne M, Erdfelder E, Buchner A, Faul F. A short tutorial of GPower. Tutor Quant Methods Psychol. (2007) 3:51–9. doi: 10.20982/tqmp.03.2.p051
70. Wang Z. A study on the Relationship Between Stressful Life Events, Anxiety and Internet Addiction in College Students. Jinzhou: The Yangtze University (2020).
71. Carskadon MA, Sharkey KM, Knopik VS, McGeary JE. Short sleep as an environmental exposure: a preliminary study associating 5-HTTLPR genotype to self-reported sleep duration and depressed mood in first-year university students. Sleep. (2012) 35:791–. doi: 10.5665/sleep.1876
72. Oquendo MA. Subtyping suicidal behavior: a strategy for identifying phenotypes that may more tractably yield molecular underpinnings. J Clin Psychiatry. (2016) 77:813–4. doi: 10.4088/JCP.16f10933
73. Oquendo MA, Sullivan GM, Sudol K, Baca-Garcia E, Stanley BH, Sublette ME, et al. Toward a biosignature for suicide. Am J Psychiatry. (2014) 171:1259–77. doi: 10.1176/appi.ajp.2014.14020194
74. Li D, Zhang W, Li X, Zhou Y, Zhao L, Wang Y. Stressful life events and adolescent Internet addiction: the mediating role of psychological needs satisfaction and the moderating role of coping style. Comput Hum Behav. (2016) 63:408–15. doi: 10.1016/j.chb.2016.05.070
75. Chen B, Liu F, Ding S, Ying X, Wang L, Wen Y. Gender differences in factors associated with smartphone addiction: a cross-sectional study among medical college students. BMC Psychiatry. (2017) 17:341. doi: 10.1186/s12888-017-1503-z
76. Borjalilu S, Mohammadi A, Mojtahedzadeh R. Sources and severity of perceived stress among Iranian medical students. Iran Red Crescent Med J. (2015) 17:e17767. doi: 10.5812/ircmj.17767
77. Zhao F, Zhang Z, Linda B, Wu X, Wang W, Li Y, et al. The association between life events and internet addiction among Chinese vocational school students: the mediating role of depression. Comput Hum Behav. (2017) 70:30–8. doi: 10.1016/j.chb.2016.12.057
78. Xu B. An Investigation on the Correlation Between Mobile Phone Dependence and Sleep Quality, Anxiety and Depression. Guangzhou: Southern Medical University (2019).
79. Elhai JD, Gallinari EF, Rozgonjuk D, Yang H. Depression, anxiety and fear of missing out as correlates of social, non-social and problematic smartphone use. Addict Behav. (2020) 105:106335. doi: 10.1016/j.addbeh.2020.106335
80. Whiteside U, Richards J, Huh D, Hidalgo R, Nordhauser R, Wong AJ, et al. Development and evaluation of a web-based resource for suicidal thoughts: NowMattersNow.org. J Med Internet Res. (2019) 21:e13183. doi: 10.2196/13183
81. Kim HR, Han MA. Associations between problematic smartphone use, unhealthy behaviors, and mental health status in Korean adolescents: based on data from the 13th Korea Youth Risk Behavior Survey (2017). Psychiatry Investig. (2020) 17:1216–25. doi: 10.30773/pi.2020.0007
82. Kim AM, Jeon SW, Cho SJ, Shin YC, Park JH. Comparison of the factors for suicidal ideation and suicide attempt: a comprehensive examination of stress, view of life, mental health, and alcohol use. Asian J Psychiatr. (2021) 65:102844. doi: 10.1016/j.ajp.2021.102844
83. Bahji A, Forth E, Hargreaves T, Harkness K. Genetic markers of the stress generation model: a systematic review. Psychiatry Res. (2021) 304:114139. doi: 10.1016/j.psychres.2021.114139
84. Bordin IA, Handegard BH, Paula CS, Duarte CS, Ronning JA. Home, school, and community violence exposure and emotional and conduct problems among low-income adolescents: the moderating role of age and sex. Soc Psychiatry Psychiatr Epidemiol. (2021). doi: 10.1007/s00127-021-02143-4. [Epub ahead of print].
Keywords: stressful life events, depressive symptoms, sleep quality, suicidal ideation, longitudinal study, problematic smartphone use
Citation: Zhao C, Ding N, Yang X, Xu H, Lai X, Tu X, Lv Y, Xu D and Zhang G (2021) Longitudinal Effects of Stressful Life Events on Problematic Smartphone Use and the Mediating Roles of Mental Health Problems in Chinese Undergraduate Students. Front. Public Health 9:752210. doi: 10.3389/fpubh.2021.752210
Received: 02 August 2021; Accepted: 08 November 2021;
Published: 03 December 2021.
Edited by:
Tingzhong Yang, Zhejiang University, ChinaCopyright © 2021 Zhao, Ding, Yang, Xu, Lai, Tu, Lv, Xu and Zhang. This is an open-access article distributed under the terms of the Creative Commons Attribution License (CC BY). The use, distribution or reproduction in other forums is permitted, provided the original author(s) and the copyright owner(s) are credited and that the original publication in this journal is cited, in accordance with accepted academic practice. No use, distribution or reproduction is permitted which does not comply with these terms.
*Correspondence: Guohua Zhang, zghcnu@wmu.edu.cn; Dongwu Xu, xdw@wmu.edu.cn; Yijun Lv, lyj@wmu.edu.cn
†These authors have contributed equally to this work