Abstract
The aim of this study is to predict the delivered dose distribution [Ddelivered(x, y)] with the use of a fluence-to-dose network (FDNet) to conduct patient-specific intensity-modulated radiation therapy (IMRT) quality assurance (pQA). The architecture of the FDNet was based on a convolutional neural network. Forty-four IMRT clinical cases of planned dose distributions for pQA [Dplanned(x, y)] and dynamic multileaf collimator (MLC) log files (Dynalog files) were collected. Using the Dynalog files, the expected fluence stack [Fexpected(x, y, t)] and the actual fluence stack [Factual(x, y, t)] were created from the expected and the actual machine parameters, respectively. The actual fluence stack, which was reconstructed from the partial information of the Dynalog file, corresponded to the control points of the Digital Imaging and Communications in Medicine radiation treatment plan and was denoted as [Factual(x, y, tpartial)]. The entire dataset was split into 11 subsets for the k-fold averaging cross-validation (k = 11). Ten (out of the 11) folds were used to train 10 candidate optimal FDNet models, and an ultimate FDNet was determined by averaging the parameters of the optimal models. The pQA was performed using the test data of the remaining fold with the ultimate FDNet. The dose distributions predicted using Factual(x, y, t) [Dpredicted(Factual(x, y, t))] and Factual(x, y, tpartial) [Dpredicted(Factual(x, y, tpartial))] were acquired. To evaluate the predicted pQA results, we conducted dosimetry using EBT3 films and an ion-chamber array detector (MatriXX). These dose distributions were compared with the Dplanned(x, y) by using a gamma analysis. The average gamma passing rates were determined based on the 3%/3 mm gamma criterion and were, respectively, equal to 98.49%, 97.21%, 97.23%, and 98.03%, for the Dpredicted(Factual(x, y, t)), Dpredicted(Factual(x, y, tpartial)), EBT3 film, and MatriXX. According to this study, the feasibility of the dose prediction method using the FDNet with complete Dynalog information was verified for the pQA. The respective differences of the average gamma passing rates for the Dpredicted(Factual(x, y, t)), and Dpredicted(Factual(x, y, tpartial)) were equal, respectively, to 1.28% and 2.88% according to the 3%/3 mm and the 2%/2 mm gamma criteria.
Similar content being viewed by others
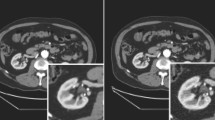
References
A. C. Hartford et al., Int. J. Radiat. Oncol. Biol. Phys. 73, 9 (2009).
J. M. Moran et al., Med. Phys. 38, 5067 (2011).
X. Y. Cedric, C. J. Amies and M. Svatos, Med. Phys. 35, 5233 (2008).
G. A. Ezzell et al., Med. Phys. 30, 2089 (2003).
Intensity Modulated Radiation Therapy Collaborative Working Group, Int. J. Radiat. Oncol. Biol. Phys. 51, 880 (2001).
M. S. Huq et al., Med. Phys. 43, 4209 (2016).
V. Gregoire and T. R. Mackie, Cancer Radiother. 15, 555 (2011).
S. Park et al., J. Korean Phys. Soc. 69, 248 (2016).
A. M. Gueli et al., Radiat. Meas. 72, 44 (2015).
H. S. Jeong et al., NET 42, 670 (2010).
R. Dreindl, D. Georg and M. Stock, Z. Med. Phys. 24, 153 (2014).
E. Y. Marroquin et al., J. Appl. Clin. Med. Phys. 17, 466 (2016).
V. C. Borca et al., J. Appl. Clin. Med. Phys. 14, 158 (2013).
H. Jung et al., J. Korean Phy. Soc. 65, 1829 (2014).
Y. Onishi et al., J. Korean Phys. Soc. 67, 89 (2015).
G. Yan et al., Med. Phys. 37, 108 (2010).
Q. L. Li et al., Chin. J. Cancer 29, 617 (2010).
P. A. Jursinic, Med. Phys. 36, 2165 (2009).
L. D. Wolfsberger et al., J. Appl. Clin. Med. Phys. 11, 241 (2010).
Y. Shimohigashi et al., J. Appl. Clin. Med. Phys. 13, 3856 (2012).
D. Rangaraj et al., Pract. Radiat. Oncol. 3, 80 (2013).
C. E. Agnew, D. M. Irvine and C. K. McGarry, J. Appl. Clin. Med. Phys. 15, 4994 (2014).
M. D. Kumar, N. Thirumavalavan, D. V. Krishna and M. Babaiah, J. Med. Phys. 31, 36 (2006).
Y. Katsuta et al., Phys. Med. 32, 701 (2016).
E. Schreibmann, A. Dhabaan, E. Elder and T. Fox, Med. Phys. 36, 4530 (2009).
D. L. Defoor et al., J. Appl. Clin. Med. Phys. 16, 206 (2015).
J. W. Lee et al., J. Korean Phys. Soc. 55, 1649 (2009).
Y. Jung and J. Hu, J. Nonparam. Stat. 27, 167 (2015).
A. L. McNiven, M. B. Sharpe and T. G. Purdie, Med. Phys. 37, 505 (2010).
Varian User Manual, Dynalog File Viewer Reference Guide (2003).
G. Xu, H. Z. Wu and Y. Q. Shi, in Proceedings of the 4th ACM Workshop on Information Hiding and Multimedia Security (ACM, 2016, Vigo, Spain), p. 103.
A. Krizhevsky, I. Sutskever and G. E. Hinton, in Advances in Neural Information Processing Systems (2012), p. 1097.
K. He, X. Zhang, S. Ren and J. Sun, in Proceedings of the IEEE Conference on Computer Vision and Pattern Recognition (2016, Nevada, USA), p. 770.
S. Ioffe and C. Szegedy, arXiv preprint arXiv:1502.03167 (2015).
M. Rezaei, H. Yang and C. Meinel, in International Conference on Neural Information Processing (Springer, 2017, Guangzhou, China), p. 798.
D. P. Kingma and J. Ba, arXiv preprint arXiv:1412.6980 (2014).
V. Nair and G. E. Hinton, in Proceedings of the 27th International Conference on Machine Learning (ICML- 10) (2010, Haifa, Israel), p. 807.
D. A. Low, W. B. Harms, S. Mutic and J. A. Purdy, Med. Phys. 25, 656 (1998).
A. Agnew et al., Phys. Med. Biol. 59, N49 (2014).
W. Luo et al., Med. Phys. 33, 2557 (2006).
P. Zygmanski, J. H. Kung, S. B. Jiang and L. Chin, Med. Phys. 30, 2736 (2003).
J. F. Calvo-Ortega et al., J. Appl. Clin. Med. Phys. 15, 4665 (2014).
J. Qian, L. Xing, W. Liu and G. Luxton, Phys. Med. Biol. 56, 4827 (2011).
Acknowledgments
This work was supported by the National Research Foundation of Korea funded by the Ministry of Science, ICT & Future Planning (MSIP) (2017R1C1B2011257 and 2013M2A2A7043507).
Author information
Authors and Affiliations
Corresponding authors
Rights and permissions
About this article
Cite this article
Cheon, W., Kim, S.J., Hwang, UJ. et al. Feasibility Study of the Fluence-to-Dose Network (FDNet) for Patient-Specific IMRT Quality Assurance. J. Korean Phys. Soc. 75, 724–734 (2019). https://doi.org/10.3938/jkps.75.724
Received:
Revised:
Accepted:
Published:
Issue Date:
DOI: https://doi.org/10.3938/jkps.75.724