Sex-specific changes in autosomal methylation rate in ageing common terns
- 1Behavioural Genomics, Max Planck Institute for Evolutionary Biology, Plön, Germany
- 2Institute of Avian Research, Wilhelmshaven, Germany
- 3Berlin Center for Genomics in Biodiversity Research, Berlin, Germany
- 4Wellcome Genome Campus, Wellcome Sanger Institute, Cambridge, United Kingdom
- 5The Rockefeller University, New York, NY, United States
- 6Department of Evolutionary Genetics, Leibniz Institute for Zoo and Wildlife Research (IZW), Berlin, Germany
- 7Howard Hughes Medical Institute, Chevy Chase, MD, United States
Senescence, an age-related decline in survival and/or reproductive performance, occurs in species across the tree of life. Molecular mechanisms underlying this within-individual phenomenon are still largely unknown, but DNA methylation changes with age are among the candidates. Using a longitudinal approach, we investigated age-specific changes in autosomal methylation of common terns, relatively long-lived migratory seabirds known to show senescence. We collected blood at 1-, 3- and/or 4-year intervals, extracted DNA from the erythrocytes and estimated autosomal DNA methylation by mapping Reduced Representative Bisulfite Sequencing reads to a de novo assembled reference genome. We found autosomal methylation levels to decrease with age within females, but not males, and no evidence for selective (dis)appearance of birds of either sex in relation to their methylation level. Moreover, although we found positions in the genome to consistently vary in their methylation levels, individuals did not show such strong consistent variance. These results pave the way for studies at the level of genome features or specific positions, which should elucidate the functional consequences of the patterns observed, and how they translate to the ageing phenotype.
1. Introduction
Senescence is a within-individual decline in survival probability (actuarial senescence) and/or reproductive performance (reproductive senescence) with age. Although the rate and shape of the decline vary both among and within species, this detrimental process occurs in most species across the tree of life (Shefferson et al., 2017). It is hypothesized to have evolved because unavoidable environmentally-driven mortality reduces the strength of selection against poor performance with age (Fisher, 1930; Medawar, 1952; Williams, 1957; Hamilton, 1966). From a genetic point of view, this so-called ‘selective shadow’ could allow for the accumulation of late-acting deleterious mutations over evolutionary time (mutation accumulation, Medawar, 1952), or for selection favouring alleles with beneficial effects early, but detrimental effects late in life (antagonistic pleiotropy, Williams, 1957). Moreover, early-life investment of limited resources in reproduction over that in perfect somatic maintenance and repair would also be evolutionarily beneficial, such that senescence could be a ‘best-of-a-bad-job’ consequence of accumulated, unrepaired damage (disposable soma, Kirkwood, 1977).
Although the classical theories of mutation accumulation and antagonistic pleiotropy assume a purely sequence-level genetic basis to senescence, the disposable soma theory does not. Given its firm foundation in life history theory, resource acquisition and allocation (sensu van Noordwijk and de Jong, 1986) are important facets to consider. Both are known to show phenotypic plasticity in response to environmental variation (e.g., Erikstad et al., 1998; Descamps et al., 2016), and experimental manipulation of one of these facets without adjustment of the other is known to affect rates of senescence (e.g., Boonekamp et al., 2014). As such, part of the senescence process is also expected to be underpinned by more flexible, regulatory processes (Wilson et al., 2008). In line with this, numerous studies across taxa have shown that senescence is underpinned by both genetic and epigenetic processes (Sen et al., 2016; Melzer et al., 2020).
Epigenetic processes are those that affect the regulation of gene expression (Holliday, 2006). This regulation is complex and encompasses several mechanisms, a major one of which is DNA methylation, the addition of a methyl-group to the fifth carbon site of a cytosine in a CpG (5’-C-phosphate-G-3’) dinucleotide context (Jaenisch and Bird, 2003; Miranda and Jones, 2007; Suzuki and Bird, 2008). Such DNA methylation could lead to transcriptional silencing of genes and repetitive elements, as DNA methylation acts as a ligand for methyl-binding domains, which in turn are targets for further chromatin-modifying complexes such as histone deacetylase complexes or chromatin remodeling complexes (Jaenisch and Bird, 2003; Suzuki and Bird, 2008; Brenet et al., 2011; Miller and Grant, 2013). DNA methylation of gene bodies, however, can lead to increased gene expression (Suzuki and Bird, 2008) or be involved in alternative splicing (Lev Maor et al., 2015). Age-specific DNA methylation has been described for many model species (e.g., Maegawa et al., 2010; Hannum et al., 2013; Tharakan et al., 2020), and the methylation status of specific CpGs has been shown to be a powerful predictor of both chronological and biological age, i.e., to function as an epigenetic clock (Bocklandt et al., 2011). Although age-specific methylation therefore seems the norm, at least in model organisms, the direction of methylation changes with age is harder to predict: both non-directional changes (representing epigenetic drift, Fraga et al., 2005; Tan et al., 2016), and global directional changes (representing global hyper- and hypomethylation, Zampieri et al., 2015; Ciccarone et al., 2018; but see Unnikrishnan et al., 2019) have been reported.
Research on senescence, including its epigenetic underpinning, has so far mostly focused on humans or model organisms kept under controlled laboratory conditions. Extending the taxonomic range and incorporating field studies is crucial to understand the evolutionary ecology of senescence (Monaghan et al., 2008). For this extension, birds are an especially interesting taxon. They have longer life spans relative to their body size than mammals (e.g., Lindstedt and Calder, 1976), and various populations of birds with vastly different life histories have been studied over several decades, such that many basic insights into their senescence patterns can now be obtained (Bouwhuis and Vedder, 2017). Bird genomes are rather compact, show high levels of synteny across species (Zhang et al., 2014), and, similar to those of other vertebrates, are globally methylated (Suzuki and Bird, 2008; Li et al., 2011; Sepers et al., 2019). With respect to age-specific DNA methylation in birds, we are aware of the existence of an epigenetic clock for short-tailed shearwaters (Ardenna tenuirostris; De Paoli Iseppi et al., 2019), and of findings of early-life within-individual increases in global methylation in both great tit (Parus major; Watson et al., 2019) and zebra finch (Taeniopygia guttata; Sheldon et al., 2020) nestlings. However, we are not aware of any study reporting age-specific DNA methylation patterns in avian adulthood, or in avian late-life specifically.
When studying age-specific trait expression, many studies, including most of those producing epigenetic clocks or otherwise studying age-specific differences in DNA methylation patterns, use cross-sectional samples and analysis tools. Patterns revealed by cross-sectional approaches, however, are the result of a combination of within- and among-individual processes. If we aim for understanding the within-individual process of senescence, we need to account for the effect of compositional changes of a population, for example when birds with a certain level of DNA methylation are more likely to die and selectively disappear from the study population (e.g., Vaupel and Yashin, 1985; Forslund and Part, 1995). Mixed-effect models applied to (partly) longitudinal data to specifically test whether within-individual patterns and population-level patterns are the same, or differ, are a powerful analytical tool to do so (van de Pol and Wright, 2009). Across taxa, efforts have increasingly been made to validate and complement findings from cross-sectional analyses of senescence through longitudinal studies (Nussey et al., 2008; Bouwhuis and Vedder, 2017; Gaillard et al., 2017), and this methodological turn is also reflected in studies of human DNA methylation (Bollati et al., 2009; Tan et al., 2016). Longitudinal studies of DNA methylation and ageing in model species and natural populations are, however, rare (but see Lemaître et al., 2021) and needed (Bell et al., 2019).
Here, we report on a longitudinal study of autosomal methylation levels in the common tern (Sterna hirundo). Common terns are long-lived migratory seabirds whose patterns of senescence have been the topic of various studies. Although breeders of both sexes show little sign of reproductive senescence - they breed earlier in the year and fledge more offspring as they grow older (Nisbet et al., 2002; Zhang et al., 2015c; Nisbet et al., 2020) - breeding and survival probabilities are known to decline with age (Zhang et al., 2015b; Vedder et al., 2021b). In addition, there is evidence for sex-specific transgenerational senescence, with daughters of older mothers and sons of older fathers suffering from reduced lifetime reproductive success (Bouwhuis et al., 2015). Studies aiming to identify a molecular basis for these within- and transgenerational effects have so far focused on telomere dynamics, and found that: (i) telomeres shorten with age (Bichet et al., 2020); (ii) telomere length is genetically correlated with lifespan (Vedder et al., 2021a); and (iii) paternal age is negatively correlated with offspring telomere length (Bouwhuis et al., 2018). The explanatory power of telomere length, however, is very low for all of these patterns (e.g., 1.1% of phenotypic variation in lifespan is explained by additive genetic variation in telomere length, Vedder et al., 2021a), such that additional mechanisms are expected. To evaluate whether age-specific changes in global DNA methylation could be such a mechanism, we (i) sequenced and de novo assembled a high-quality chromosome-scale reference genome and (ii) used it to compare within-individual age-specific changes in DNA methylation at shared sites across the genome. Although rates of within-generational senescence do not differ between the sexes (Zhang et al., 2015a), transgenerational effects are known to be sex-specific (Bouwhuis et al., 2015), such that we also considered sex-specificity of any patterns in autosomal methylation status.
2. Materials and methods
2.1. Study population
The data we present were collected as part of a long-term individual-based study of a mono-specific common tern colony located on six artificial concrete islands at the Banter See (53°30’40” N, 08°06’20” E) in Wilhelmshaven, Germany. Fledglings from this colony have been marked with metal rings since 1984, subcutaneously injected with transponders since 1992 (Becker and Wendeln, 1997) and molecularly sexed since 1996 (Becker and Wink, 2003). This marking and sexing, combined with placement of antennae on elevated platforms on the edges of the colony site and around each nest during incubation (shared between both parents), allows for a well-described family structure of all known-sex and known-age philopatric birds (e.g., Moiron et al., 2020).
2.2. Reference genome
To create the chromosome-scale reference genome, blood of one adult female common tern was collected in 100% EtOH and stored at −80°C. 30μg of High Molecular Weight DNA (HMW DNA) was isolated from the whole blood sample using an agarose plug protocol of the Bionano Prep Blood and Cell Culture DNA Isolation Kit (cat no. RE-130-10) modified for avian nucleated erythrocytes. Lysates were embedded into agarose plugs, followed by Proteinase K and RNase A treatments and 1X TE drop dialysis purification. Four sequence datasets were generated following the VGP 1.5 pipeline (Rhie et al., 2021): 67.91x Pacific Biosciences (Pacbio) continuous long reads (CLR); 698.35x Bionano Genomics optical maps; 169.30x 10X Genomics linked-reads; and 79.62x Arima Genomics Hi-C Illumina reads.
To create the Pacbio data, DNA was sheared using a 26G blunt end needle (Pacbio protocol PN 101-181-000 Version 05) to approximately ~40kb fragment length. We used 10μg of this fragmented DNA to generate a large-insert Pacbio library using the Pacific Biosciences Express Template Prep Kit v1.0 (#101-357-000). The library was then size selected (>15 kb) using the BluePippin system (Sage Science). The resulting PacBio Library was sequenced on 10 PacBio 1M v3 smrtcells (#101-531-000) on a Sequel instrument with the sequencing kit 3.0 (#101-427-500) and a 10 h movie with 2 h pre-extension time. Unfragmented HMW DNA was used to generate a linked-read library on the 10X Genomics Chromium (Genome Library Kit and Gel Bead Kit v2 PN-120258, Genome Chip Kit v2 PN-120257, i7 Multiplex Kit PN-120262). We sequenced this 10X library on an Illumina Novaseq S4 150 bp PE lane. uHMW DNA was labeled for Bionano Genomics optical mapping using the Bionano Prep Direct Label and Stain (DLS) Protocol (30206E) and 1 flow cell was run on the Saphyr instrument. Hi-C libraries were generated with the Arima Genomics v1.0 2-enzyme protocol (P/N: A510008), according to the manufacturer’s protocol and sequenced on Illumina HiSeq X.
The resulting four data types were processed using the VGP v1.5 pipeline (Rhie et al., 2021), which includes: assembling Pacbio contigs using FALCON v2018.31.08-03.06; FALCON-Unzip v6.0.0.47841; purging false haplotype duplications with purge_haplotigs v1.0.3+ 1.Nov. 2018; scaffolding with 10X with scaff10x v4.1.0; scaffolding with Bionano Solve DLS v3.2.1; scaffolding with Hi-C data with Salsa HiC v2.2; filling in gaps and polishing for base call accuracy with CLR and Arrow smrtanalysis v6.0.0.47841; and polishing with Illumina short reads with longranger align v2.2.2; and freebayes v1.3.1. The resulting assembly was then manually curated to fix any errors, using gEVAL and Hi-C short read linked-read mapping profiles as described in Howe et al. (2021). BUSCO v4.1.4 with the bird lineage dataset (aves_odb10) was used to assess assembly completeness. The reference genome was submitted to NCBI as GCA_009819605.1, as part of the Vertebrate Genomes Project (VGP).1 Reference genomes consisting of short-read assemblies (e.g., using Illumina reads) exhibit GC bias, where GC-rich regions such as promoters could be incorrectly assembled or even missing (Kim et al., 2021). Our reference genome, however, has GC-rich promoter regions due to the use of Pacbio long reads (Kim et al., 2021; Rhie et al., 2021).
2.3. Blood sampling and methylation sequencing
Blood of breeding common terns was sampled in the years 2013, 2014 and 2017 using larval stages of the bloodsucking bug Dipetalogaster maximus. Within 9-14 days of clutch completion of each focal bird in each year, bugs were placed into dummy eggs with holes and placed in the nests (Becker et al., 2006; Arnold et al., 2008; Bichet et al., 2019). After 20–30 min of incubation by the focal bird, “bug eggs” were collected and the focal birds’ blood, sucked by the bug, was removed from the bugs’ abdomen using a syringe. Upon collection, whole blood was stored in EDTA buffer (2%) in a fridge (3–7°C) for up to 3 weeks, before the red blood cells were transferred to glycerol buffer (40%) and frozen at −80°C. Each bug was used only once to prevent cross-contamination of blood samples.
In total, we obtained 74 samples from 34 individuals making up 17 breeding pairs: 3 breeding pairs (i.e., 6 individuals) were sampled in all 3 years (i.e., with intervals of 1, 3 and 4 years), 14 breeding pairs (i.e., 28 individuals) in two of the 3 years (with a 1-year interval for 4 breeding pairs, a 3-year interval for 9 breeding pairs and a 4-year interval for 1 breeding pair; see Supplementary Table S1 for details). The age of the sampled females ranged between 4 and 20 years, that of males between 3 and 19 years.
Genomic DNA was extracted from each sample and libraries for RRBS were generated as described in Klughammer et al. (2015), following standard steps such as MspI digestion, end-fill-in, A-tailing and size selection. This included the enrichment of the libraries with Pfu-Turbo Cx Hotstart DNA polymerase to allow the assessment of the bisulfite conversion rate. After clean-up and quality control, libraries were sequenced with an Illumina HiSeq 4000 (50 bp SE).
2.4. Methylation data selection
Across all 74 samples, individuals were sequenced with an average of 51,482,011 (range: 24,942,071–82,760,425) reads (Supplementary Tables S1, S2). After conversion of unmapped bam files to fastq with SamToFastq.jar from picard v1.118, the quality of the sequencing reads was checked using Fastqc v0.11.5 (Andrews, 2010) and Multiqc v1.8 (Ewels et al., 2016). Spiked-in sequences were used to estimate over- and underconversion using RefFreeDMA (Klughammer et al., 2015). Trimgalore v0.3.3 (implemented in RefFreeDMA; Krueger et al., 2021) was used to remove adaptors to guarantee good mapping and to remove low quality bases (≥20) as well as short read fragments (≥16 bp).
We used Bismark v0.22.3 (Krueger and Andrews, 2011) to prepare and index the reference genome for subsequent mapping of the bisulfite-converted reads using Bowtie 2 v2.4.1 (Langmead and Salzberg, 2012), allowing for one mismatch (score_min L, 0, −0.20). Methylation extraction was conducted with Bismark Extractor v0.22.3 (Krueger and Andrews, 2011) with the ‘ignore’ option to remove unmethylated cytosines introduced during the end-repair step. We combined data from the cytosines of both strands at each CpG site with a custom-made python script. The R-package Methylkit v.1.16.1 in R v.4.0 (Akalin et al., 2012; R Core Team, 2021) and its function methRead were used to load and analyse the methylation calls.
Methylation calls were filtered to those with a minimum coverage of 10 reads per CpG position. CpGs with a coverage >99.9th percentile most likely result from PCR bias and were removed. These two steps were performed using the filterByCoverage function in Methylkit. Across all 74 samples, there were on average 5,536,718 CpG positions before, and 661,447 CpG positions after, filtering for >10x and <99.9th percentile coverage (Supplementary Tables S1, S2). Methylation call distributions between samples were normalized using the normalizeCoverage function implemented in Methylkit to reduce the potential bias of systematic oversampling in some samples. We then merged CpG positions of the different samples using the unite function to make sure positions were sequenced in at least 70% of the samples (within and across the sexes) to facilitate a longitudinal analysis approach. Following Meng et al. (2010) and Sziráki et al. (2018), sites that showed little or no variation were removed as well, applying a threshold of a standard deviation <0.15. This resulted in 927,490 observations of 15,700 positions, distributed across all autosomal chromosomes (Supplementary Figure S1), which we used to assess autosomal methylation rates by using the number of positions read as our denominator and the number of cases in which the position was methylated as our dependent variable (see Statistical analyses).
2.5. Statistical analyses
To identify and partition sources of variation in autosomal methylation rates, we used the R package glmmTMB (Brooks et al., 2017) to run a generalized linear mixed model (GLMM) with the BFGS algorithm as an optimizer, using the number of methylated and unmethylated Cs (represented by the sequenced Cs and Ts) for each position as our dependent variable, assuming a binomial error distribution (Lea et al., 2017). As fixed effects we added ‘sex’ (as a two-level class variable using males as the reference category) and age (as a covariate). With respect to the latter, each individual’s age was partitioned into an ‘average age’ and ‘delta age’ component following van de Pol and Wright (2009). Average age was calculated as the average of all ages at which we assessed a bird’s autosomal methylation rate, while delta age was calculated as the difference between the bird’s actual age and its average age (i.e., delta age = age − average age). When adding both age variables as covariates, average age represents the among-individual, and delta age the within-individual age effect (van de Pol and Wright, 2009). If the among- and within-individual age effects were to differ, this would indicate that the effect of age among individuals cannot be explained by changes within individuals, thereby revealing age-specific selective (dis)appearance of individuals with certain levels of methylation (van de Pol and Wright, 2009). We additionally included the interaction between sex and delta age in our model to test for sex-differences in the within-individual age trajectory of methylation. Random effects included were ‘bird identity’ (1–34), genomic ‘position identity’ (1–15,700 scored as the base-pair-number on our scaffold) and an ‘observation’-level random effect (1–927,490 scored at the lowest level of observation, i.e., one value for each genomic position for each individual). The latter was added to (successfully) account for overdispersion detected by the R package performance (Lüdecke et al., 2021), whereas the first two were included to account for pseudoreplication caused by repeated sampling of individuals and genomic positions, respectively. Although we could have theoretically added breeding pair identity as a random effect, doing so would only add information when pairs regularly break up and reform among sampling events. Our common tern pairs all stayed together, such that we would not be able to disentangle the effects from pair and bird identify effects.
Because we found a significant interaction between sex and delta age (see Results), we also split our data set in one for males and one for females and ran sex-specific analyses including ‘average age’ and ‘delta’ age as fixed effects and ‘bird identity’, genomic ‘position identity’ and ‘observation’ as random effects. Moreover, by including positions that were sequenced in at least 70% of the samples, we facilitated a longitudinal analysis approach and a comparison between males and females, but excluded CpGs from the sex chromosome. To also assess age-specificity in methylation rate of the W chromosome, we also ran the sex-specific model for females for the 103 CpG positions we could assess there by including positions that were sequenced in at least 70% of the female samples.
Model evaluation and summary of parameter estimates and statistics were conducted using the R package parameters (Lüdecke et al., 2020). Results were plotted using the R-package sjplot (Lüdecke, 2018).
3. Results
3.1. Reference genome
The reference genome was generated using 68x PacBio sequencing reads, Bionano Genomics optical maps, 10X Genomics linked-reads and Arima Hi-C reads. This allowed us to scaffold the assembly to chromosome-level (Rhie et al., 2021) and we successfully assigned 99.3% of the assembled sequence to 25 identified autosomes, two sex chromosomes and the mitochondrial genome, leaving only 95 scaffolds unlocalised. The total length of the primary haplotype assembly was 1.23 Gbp with a contig N50 of 22.0 Mb and a scaffold N50 of 85.5 Mb. The assembly included 96.0% complete single-copy and 0.4% duplicated orthologs according to the BUSCO analysis. Only 1.3% of the gene models were fragmented, and 2.3% missing (n = 8,338 genes). This represents a high-quality assembly, surpassing the aspired VGP contiguity metrics ~20-fold (Rhie et al., 2021).
3.2. Age-specific global methylation
Across the 74 samples, 29,044,662 RRBS reads (~ 55.6% mapping efficiency) could be uniquely mapped to the reference genome (Supplementary Tables S1, S2). The mean bisulfite conversion rate was 99.2% (Supplementary Tables S1, S2). 15,700 autosomal CpG positions occurred in at least 70% of samples within and across the sexes, and across these positions methylation rates ranged from 0 to 1 in both males and females (Supplementary Figure S2). The average predicted methylation levels per sample ranged from 0.437 to 0.752 (mean: 0.562) in females and from 0.424 to 0.777 (mean: 0.627) in males (Figure 1).
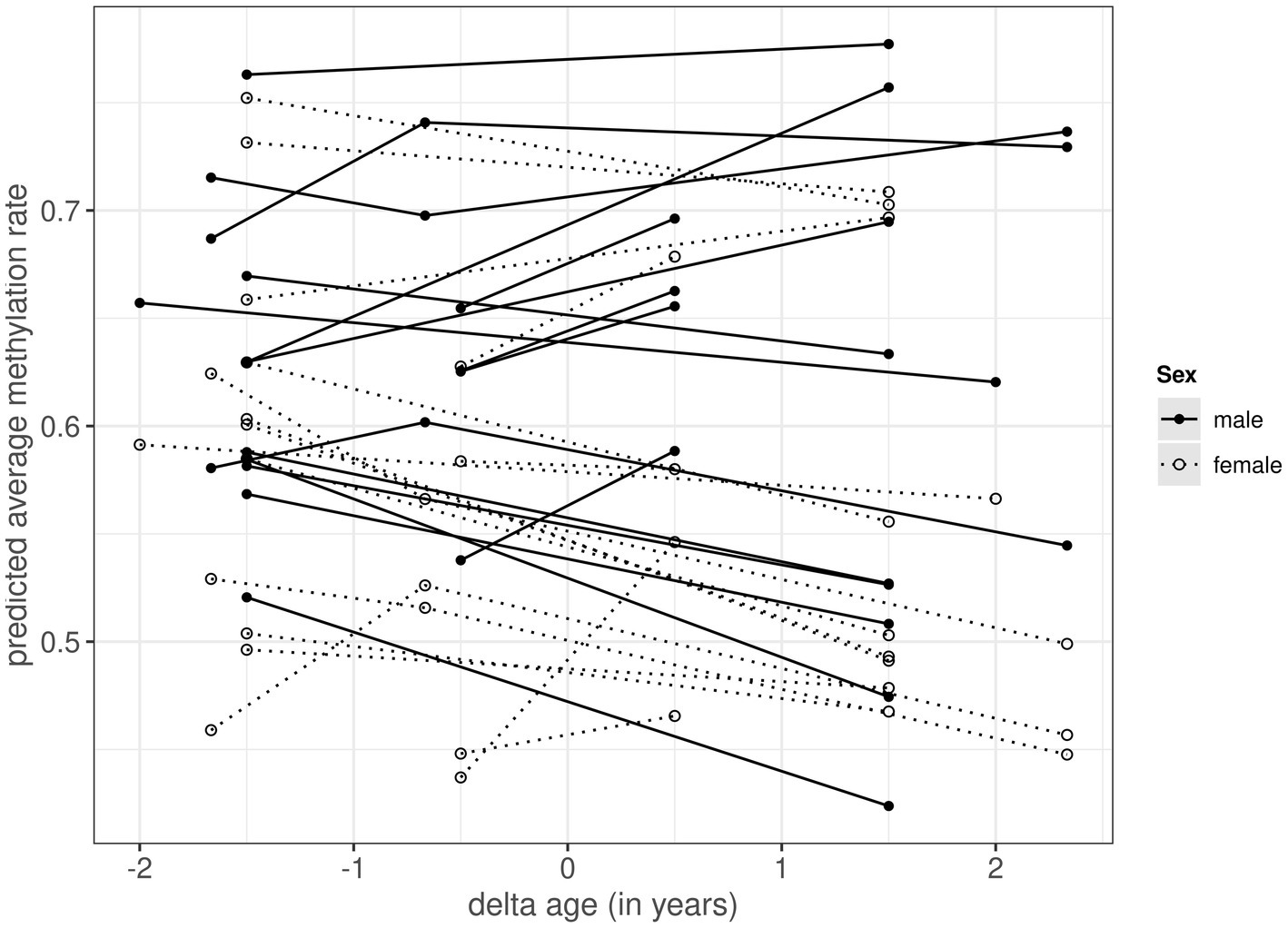
Figure 1. Within-individual changes in predicted average autosomal methylation rate for 17 male (black circles with solid lines) and 17 female (white circles with dotted lines) common terns sampled at 1, 3 and/or 4 year intervals. The x-axis, termed delta age, represents the within-individual difference in age (in years) in relation to the average age at sampling (at negative values the individual was younger than its average age at sampling, at positive values it was older). The predicted methylation rate on the y-axis is extracted from the model presented in Table 1, and based on 15,700 autosomal CpG positions.
When analyzing sources of variation in methylation rates across the 15,700 autosomal CpG positions, we found a significant main effect of sex, as well as a significant interaction between sex and delta age (Table 1). The main effect of sex showed males to have higher methylation rates than females, the interaction term indicated that methylation rates showed different age-specificity in males and females. Subsequent sex-specific analyses showed that methylation rates declined with age within individual females, but did not change with age within individual males (Figure 1; Supplementary Tables S3, S4). In the main model (as well as in the sex-specific models, Supplementary Tables S3, S4), the effect of average age was non-significant and the credible intervals of the average and delta age components strongly overlapped (Table 1), suggesting no selective (dis)appearance of individuals based on their autosomal methylation rates.
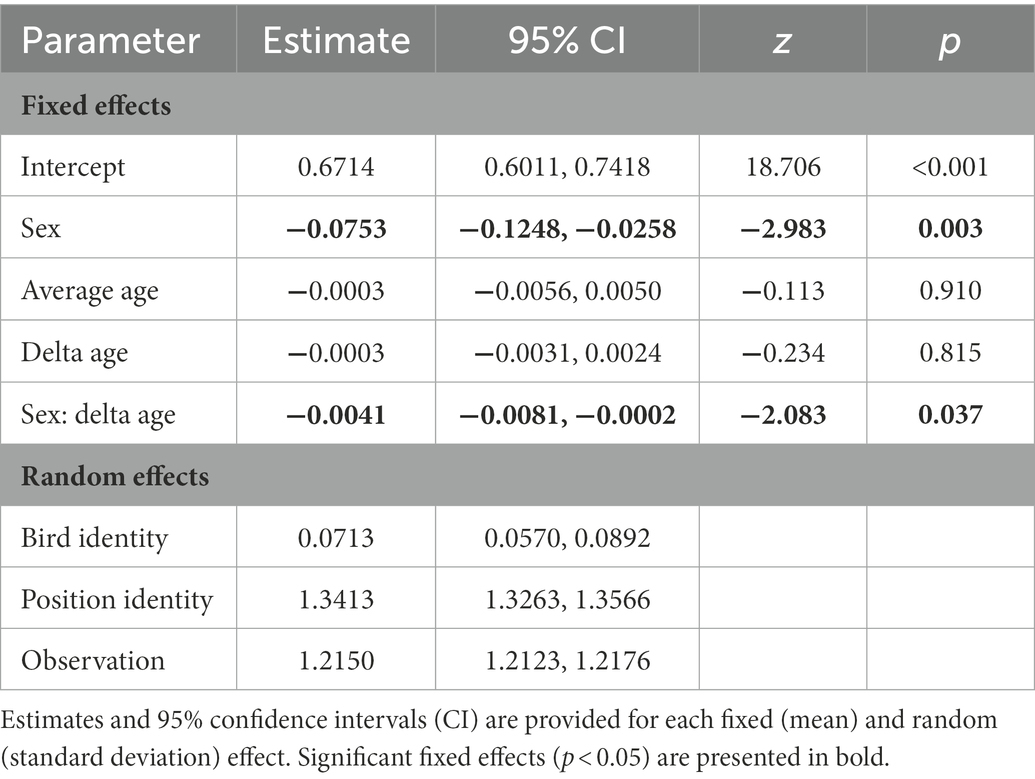
Table 1. Results from a generalised linear mixed model with a binomial error distribution testing whether variation in autosomal methylation level is explained by sex (males as a reference category) and the between- (average age) and within-individual (delta age) components of age.
When assessing the age-specificity of methylation rates in the 103 CpG positions of the female W chromosome, neither average nor delta age was significant (Supplementary Table S5).
When comparing the random effects in the main model, most variance was explained by position identity (Table 1), showing that genomic positions vary in their average rate of methylation. Bird identity, on the other hand, explained variation in methylation rates to a much lesser extent (Table 1), such that there is little evidence for consistent variation in methylation among individuals.
4. Discussion
DNA methylation patterns at CpG sites are increasingly used as biomarkers, so-called epigenetic clocks, to predict both chronological and biological age across species and taxa (e.g., Lu et al., 2021). How DNA methylation rate changes within individuals and whether it can explain phenotypic senescence patterns, however, is still largely unknown (Bell et al., 2019). Here, we used blood samples from common terns collected at 1-, 3- and/or 4-year intervals and a longitudinal analysis approach to investigate whether autosomal methylation rates changed with age within individual birds, and whether any change differed between males and females sharing environments and broods. Based on our selection of 15,700 positions, we found female genomes to be generally less methylated than those of males, and to also become even less methylated as these females aged, whereas there was no such age-specific decline of autosomal methylation rate in males. Moreover, we found the estimates for the within- and among-individual components of age to be similar, such that there was no indication for selective (dis)appearance of individuals based on their methylation rate. Finally, we provide evidence for positions in the genome to consistently vary in their methylation rates, whereas evidence for consistent variation among individuals was considerably less.
Our finding of female common terns showing a decrease in methylation rate as they aged fits with findings of global hypomethylation in older compared to younger mammals (Zampieri et al., 2015; Ciccarone et al., 2018; Unnikrishnan et al., 2019). Such methylation loss is thought to partly originate from demethylation of large regions of repetitive sequences, CpG-poor promoters or large hypomethylated blocks of "open sea" regions outside the CpG islands (Bollati et al., 2009; Heyn et al., 2012; Yuan et al., 2015). Whether these changes affect chromatin configuration and thus genome (in)stability, and whether changes in promoter methylation interact with histone modifications and transcription factors to alter expression remains to be investigated (Zampieri et al., 2015; Ciccarone et al., 2018).
Male terns, in contrast, showed no signs of decreased methylation as they grew older. Although many studies developing epigenetic clocks have assumed age-related changes to be similar across the sexes and used mixed-sex datasets to obtain them [e.g., Horvath et al., 2021; Raj et al., 2021), others have found sex-differences in these clocks (e.g., in some human ethnicities (Horvath et al., 2016), baboons (Anderson et al., 2021) or elephants (Prado et al., 2021)]. Moreover, a rare partly longitudinal study in a wild population of roe deer also showed sex-specific epigenetic clock regions, with an accelerated ageing signal in males, which are known to undergo stronger survival senescence in this species (Lemaître et al., 2021). Combined with our findings, this suggests that tests for sex-specificity of methylation should best be the norm.
Interestingly, male and female terns from our study population do not differ in the onset or rate of senescence in survival or breeding probabilities (Zhang et al., 2015b; Vedder et al., 2021b), nor in their average lifespan (7.4 years for both males and females, Bouwhuis et al., 2015), such that sex-specificity in the ageing process is only found in how parental age affects the quality of the offspring that recruit back into the population [with maternal age negatively affecting the reproductive performance of daughters and paternal age negatively affecting survival of sons (Bouwhuis et al., 2015)]. As such, we did not necessarily expect sex differences in the age-specificity of the birds’ autosomal methylation level. The fact that we were able to observe them, raises the question of which site-specific methylation patterns drive the pattern observed on the global level. As mentioned above, global loss is thought to originate from the demethylation of specific regions: repetitive sequences, CpG-poor promoters or large hypomethylated blocks of "open sea" regions outside the CpG islands (Bollati et al., 2009; Heyn et al., 2012; Yuan et al., 2015). CpG island promoters, on the other hand, have been found to show age-specific increases in methylation (Heyn et al., 2012; Day et al., 2013). As such, global demethylation may perhaps be compensated for by such increases in males, but not females. In combination with the fact that we found genomic positions to consistently differ in methylation levels, this stresses the need for moving from the level of global autosomal methylation assessment that we and others (e.g., Watson et al., 2019; Sheldon et al., 2020) have started with, to fully annotating the (common tern) genome and studying patterns across genomic features and focal sites.
Besides finding a sex-specific overall level and within-individual change in autosomal methylation level with age, and significant among-position consistency in methylation level, we found little evidence for consistent among-individual levels of autosomal methylation across years, or for selective (dis)appearance of birds in relation to their methylation level. This suggests that birds differentially change their autosomal methylation from year to year, i.e., show different gaps between epigenetic and chronological age each year, with these changes or gaps perhaps reflecting their individual-specific condition or environment, but not relating to their breeding status (breeding versus non-breeding) in the study population or their local survival. Implementing a random regression analytical framework is data-hungry and not possible with our current dataset, but linking among-year changes in e.g., body mass, other measures of physiology or age-specific reproductive performance seems a promising research avenue, especially when taking the analyses to a site-specific level, such that distinct genotype-methylation-phenotype correlations can be identified.
The strength of our study lies in its longitudinal sampling and analytical approach, which allows us to characterise within-individual changes, rather than infer them from cross-sectional data. At the same time, however, this sampling approach may come with some limitations. Non-destructive, longitudinal sampling in natural populations often relies on using blood as the focal tissue, but how DNA methylation of (in our case) erythrocytes translates to phenotypes mostly remains an open question that needs addressing (Husby, 2020), ideally in experimental study systems. Moreover, because we used RRBS, a high-throughput and low-cost method, to assess methylation, our findings may mostly pertain to methylation of high density CpG regions (Smith et al., 2009; Gu et al., 2011). As such, we may have focally answered the question of what happens at CpG islands during ageing (Beck et al., 2021), something which annotation of the common tern genome will be able to tell. Keeping this in mind, however, our study has provided evidence for a sex-specific overall level and within-individual change in autosomal methylation with age and has shown that CpG positions in the genome vary consistently with respect to their methylation levels, such that future work, changeing the perspective from genome-wide average estimates to the specific genome feature or base pair level, can elucidate whether, where and how much methylation might affect ageing males and females, as such establishing a longitudinal epigenetic clock.
Data availability statement
The dataset and bioinformatic code presented in this study can be found at Zenodo (10.5281/zenodo.7533890 and 10.5281/zenodo.7493357).
Ethics statement
The study was performed under licenses of the city of Wilhelmshaven and the Lower Saxony State Office for Consumer Protection and Food Safety, Germany.
Author contributions
SB collected the blood samples and life history data on the terns. SB and ML designed the study. ML organized sequencing of the samples. BM analyzed the methylation data and performed the statistical analyses with the help of MM. The reference genome was generated as part of the Vertebrate Genomes Project, coordinated by EJ. BH and JM were responsible for sample processing and generated the genome sequence data. CC, GF, and MU-S assembled the genome under coordination of OF. WC, JW, and KH curated the final reference genome. BM and SB wrote the manuscript with input from ML, MM, and EJ. All authors contributed to the manuscript and approved the submitted version.
Funding
This study was supported by the Max Planck Society through a MPRG grant (MFFALIMN0001 to ML), a sequencing grant (PSLIMN6000 to ML and SB), and the “Norddeutscher Wissenschaftspreis 2018” (to BM, SB and ML).
Acknowledgments
We are indebted to Peter H. Becker for setting up the long-term individual-based common tern study at the Banter See and to Götz Wagenknecht and the many scientists, students and field assistants who collected the data across the years. We express our gratitude to Johanna Klughammer, Amelie Nemc and Christoph Bock for their advice on sequencing strategy and for performing the RRBS sequencing. We also thank Michaela Schwarz for help with DNA extraction, Kristian Ullrich for his support in solving bioinformatics hurdles, Melanie J. Heckwolf, Robin Cristofari and our epigenetic journal club for inspiring discussions on DNA methylation and 2 reviewers for constructive comments that helped us improve our paper.
Conflict of interest
The authors declare that the research was conducted in the absence of any commercial or financial relationships that could be construed as a potential conflict of interest.
Publisher’s note
All claims expressed in this article are solely those of the authors and do not necessarily represent those of their affiliated organizations, or those of the publisher, the editors and the reviewers. Any product that may be evaluated in this article, or claim that may be made by its manufacturer, is not guaranteed or endorsed by the publisher.
Supplementary material
The Supplementary material for this article can be found online at: https://www.frontiersin.org/articles/10.3389/fevo.2023.982443/full#supplementary-material
Footnotes
References
Akalin, A., Kormaksson, M., Li, S., Garrett-Bakelman, F. E., Figueroa, M. E., Melnick, A., et al. (2012). methylKit: a comprehensive R package for the analysis of genome-wide DNA methylation profiles. Genome Biol. 13:R87. doi: 10.1186/gb-2012-13-10-r87
Anderson, J. A., Johnston, R. A., Lea, A. J., Campos, F. A., Voyles, T. N., Akinyi, M. Y., et al. (2021). High social status males experience accelerated epigenetic aging in wild baboons. eLife 10:66128. doi: 10.7554/eLife.66128
Andrews, S. (2010). A Quality Control Tool for High Throughput Sequence Data. Available at: http://www.bioinformatics.babraham.ac.uk/projects/fastqc (Accessed July 27, 2018).
Arnold, J. M., Oswald, S. A., Voigt, C. C., Palme, R., Braasch, A., Bauch, C., et al. (2008). Taking the stress out of blood collection: comparison of field blood-sampling techniques for analysis of baseline corticosterone. J. Avian Biol. 39, 588–592. doi: 10.1111/j.0908-8857.2008.04265.x
Beck, D., Ben Maamar, M., and Skinner, M. K. (2021). Genome-wide CpG density and DNA methylation analysis method (MeDIP, RRBS, and WGBS) comparisons. Epigenetics 17, 518–530. doi: 10.1080/15592294.2021.1924970
Becker, P. H., Voigt, C. C., Arnold, J. M., and Nagel, R. (2006). A non-invasive technique to bleed incubating birds without trapping: A blood-sucking bug in a hollow egg. J Ornithol 147, 115–118. doi: 10.1007/s10336-005-0027-3
Becker, P. H., and Wendeln, H. (1997). A new application for transponders in population ecology of the common tern 99, 534–538. doi: 10.2307/1369963
Becker, P. H., and Wink, M. (2003). Influences of sex, sex composition of brood and hatching order on mass growth in common terns Sterna hirundo. Behav Ecol Sociobiol 54, 136–146. doi: 10.1007/s00265-003-0605-4
Bell, C. G., Lowe, R., Adams, P. D., Baccarelli, A. A., Beck, S., Bell, J. T., et al. (2019). DNA methylation aging clocks: challenges and recommendations. Genome Biol. 20, 249–224. doi: 10.1186/s13059-019-1824-y
Bichet, C., Bouwhuis, S., Bauch, C., Verhulst, S., Becker, P. H., and Vedder, O. (2020). Telomere length is repeatable, shortens with age and reproductive success, and predicts remaining lifespan in a long-lived seabird. Mol. Ecol. 29, 429–441. doi: 10.1111/mec.15331
Bichet, C., Vedder, O., Sauer-Gürth, H., Becker, P. H., Wink, M., and Bouwhuis, S. (2019). Contrasting heterozygosity-fitness correlations across life in a long-lived seabird. Mol. Ecol. 28, 671–685. doi: 10.1111/mec.14979
Bocklandt, S., Lin, W., Sehl, M. E., Sánchez, F. J., Sinsheimer, J. S., Horvath, S., et al. (2011). Epigenetic Predictor of Age. PLoS One 6:e14821. doi: 10.1371/journal.pone.0014821
Bollati, V., Schwartz, J., Wright, R., Litonjua, A., Tarantini, L., Suh, H., et al. (2009). Decline in genomic DNA methylation through aging in a cohort of elderly subjects. Mech. Ageing Dev. 130, 234–239. doi: 10.1016/j.mad.2008.12.003
Boonekamp, J. J., Salomons, M., Bouwhuis, S., Dijkstra, C., and Verhulst, S. (2014). Reproductive effort accelerates actuarial senescence in wild birds: an experimental study. Ecol Lett 17, 599–605. doi: 10.1111/ele.12263
Bouwhuis, S., and Vedder, O. (2017). “Avian escape artists?” in Patterns, processes and costs of senescence in wild birds. eds. R. P. Shefferson, O. R. Jones, and R. Salguero-Gómez (Cambridge: Cambridge University Press), 156–174.
Bouwhuis, S., Vedder, O., and Becker, P. H. (2015). Sex-specific pathways of parental age effects on offspring lifetime reproductive success in a long-lived seabird. Evolution 69, 1760–1771. doi: 10.1111/evo.12692
Bouwhuis, S., Verhulst, S., Bauch, C., and Vedder, O. (2018). Reduced telomere length in offspring of old fathers in a long-lived seabird. Biol. Lett. 14:20180213. doi: 10.1098/rsbl.2018.0213
Brenet, F., Moh, M., Funk, P., Feierstein, E., Viale, A. J., Socci, N. D., et al. (2011). DNA methylation of the first exon is tightly linked to transcriptional silencing. PLoS ONE 6:e14524. doi: 10.1371/journal.pone.0014524
Brooks, M. E., Kristensen, K., van Benthem, K. J., Magnusson, A., and Bolker, B. M. (2017). glmmTMB balances speed and flexibility among packages for zero-inflated generalized linear mixed modeling. R J. 9, 378–400. doi: 10.32614/RJ-2017-066
Ciccarone, F., Tagliatesta, S., Caiafa, P., and Zampieri, M. (2018). DNA methylation dynamics in aging: how far are we from understanding the mechanisms? Mech. Ageing Dev. 174, 3–17. doi: 10.1016/j.mad.2017.12.002
Day, K., Waite, L. L., Thalacker-Mercer, A., West, A., Bamman, M. M., Brooks, J. D., et al. (2013). Differential DNA methylation with age displays both common and dynamic features across human tissues that are influenced by CpG landscape. Genome Biol. 14, R102–R119. doi: 10.1186/gb-2013-14-9-r102
De Paoli Iseppi, R., Deagle, B. E., Polanowski, A. M., McMahon, C. R., Dickinson, J. L., Hindell, M. A., et al. (2019). Age estimation in a long-lived seabird (Ardenna tenuirostris) using DNA methylation-based biomarkers. Molecular Ecology Resources 19, 411–425. doi: 10.1111/1755-0998.12981
Descamps, S., Gaillard, J. M., Hamel, S., and Yoccoz, N. G. (2016). When relative allocation depends on total resource acquisition: implication for the analysis of trade-offs. J. Evol. Biol. 29, 1860–1866. doi: 10.1111/jeb.12901
Erikstad, K. E., Fauchald, P., Tveraa, T., and Steen, H. (1998). On the cost of reproduction in long-lived birds: the influence of environmental variability. Ecology 79, 1781–1788. doi: 10.1890/0012-9658(1998)079[1781,OTCORI]2.0.CO;2
Ewels, P., Magnusson, M., Lundin, S., and Käller, M. (2016). MultiQC: summarize analysis results for multiple tools and samples in a single report. Bioinformatics 32, 3047–3048. doi: 10.1093/bioinformatics/btw354
Forslund, P., and Part, T. (1995). Age and reproduction in birds - hypotheses and tests. Trends Ecol. Evol. 10, 374–378. doi: 10.1016/s0169-5347(00)89141-7
Fraga, M. F., Ballestar, E., Paz, M. F., Ropero, S., Setien, F., Ballestar, M. L., et al. (2005). Epigenetic differences arise during the lifetime of monozygotic twins. PNAS 102, 10604–10609. doi: 10.1073/pnas.0500398102
Gaillard, J. M., Garratt, M., and Lemaître, J. F. (2017). “Senescence in mammalian life history traits” in The Evolution of Senescence in the Tree of Life. eds. R. Shefferson, O. Jones, and R. Salguero-Gómez (Cambridge: Cambridge University Press), 126–155.
Gu, H., Smith, Z. D., Bock, C., Boyle, P., Gnirke, A., and Meissner, A. (2011). Preparation of reduced representation bisulfite sequencing libraries for genome-scale DNA methylation profiling. Nat. Prot. 6, 468–481. doi: 10.1038/nprot.2010.190
Hamilton, W. D. (1966). The moulding of senescence by natural selection. J. Theor. Biol. 12, 12–45. doi: 10.1016/0022-5193(66)90184-6
Hannum, G., Guinney, J., Zhao, L., Zhang, L., Hughes, G., Sadda, S., et al. (2013). Genome-wide methylation profiles reveal quantitative views of human aging rates. Mol. Cell 49, 359–367. doi: 10.1016/j.molcel.2012.10.016
Heyn, H., Li, N., Ferreira, H. J., Moran, S., Pisano, D. G., Gomez, A., et al. (2012). Distinct DNA methylomes of newborns and centenarians. Proc. Natl. Acad. Sci. U.S.A. 109, 10522–10527. doi: 10.1073/pnas.1120658109
Holliday, R. (2006). Epigenetics: a historical overview. Epigenetics 1, 76–80. doi: 10.4161/epi.1.2.2762
Horvath, S., Gurven, M., Levine, M. E., Trumble, B. C., Kaplan, H., Allayee, H., et al. (2016). An epigenetic clock analysis of race/ethnicity, sex, and coronary heart disease. Genome Biol. 17, 171–123. doi: 10.1186/s13059-016-1030-0
Horvath, S., Zoller, J. A., Haghani, A., Jasinska, A. J., Raj, K., Breeze, C. E., et al. (2021). Epigenetic clock and methylation studies in the rhesus macaque. Geroscience 43, 2441–2453. doi: 10.1007/s11357-021-00429-8
Howe, K., Chow, W., Collins, J., Pelan, S., Pointon, D. L., Sims, Y., et al. (2021). Significantly improving the quality of genome assemblies through curation. Giga Sci. 10, 1–9. doi: 10.1093/gigascience/giaa153
Husby, A. (2020). On the use of blood samples for measuring DNA methylation in ecological epigenetic studies. Integr. Comp. Biol. 60, 1558–1566. doi: 10.1093/icb/icaa123
Jaenisch, R., and Bird, A. (2003). Epigenetic regulation of gene expression: how the genome integrates intrinsic and environmental signals. Nat. Genet. 33, 245–254. doi: 10.1038/ng1089
Kim, J., Lee, C., Ko, B. J., Yoo, D. A., Won, S., Phillippy, A., et al. (2021). False gene and chromosome losses affected by assembly and sequence errors. bioRxiv. [Preprint]. (Accessed January 12, 2023).
Klughammer, J., Datlinger, P., Printz, D., Sheffield, N. C., Farlik, M., Hadler, J., et al. (2015). Differential DNA Methylation Analysis without a Reference Genome. Cell Rep 13, 2621–2633. doi: 10.1016/j.celrep.2015.11.024
Krueger, F., and Andrews, S. R. (2011). Bismark: a flexible aligner and methylation caller for Bisulfite-Seq applications. Bioinformatics 27, 1571–1572. doi: 10.1093/bioinformatics/btr167
Krueger, F., James, F., Ewels, P., Afyounian, E., and Schuster-Boeckler, B. (2021). FelixKrueger/TrimGalore. Zenodo. doi: 10.5281/zenodo.5127898
Langmead, B., and Salzberg, S. L. (2012). Fast gapped-read alignment with Bowtie 2. Nat. Methods 9, 357–359. doi: 10.1038/nmeth.1923
Lea, A. J., Vilgalys, T. P., Durst, P. A. P., and Tung, J. (2017). Maximizing ecological and evolutionary insight in bisulfite sequencing data sets. Nat. Ecol. Evol. 1, 1074–1083. doi: 10.1038/s41559-017-0229-0
Lemaître, J. F., Rey, B., Gaillard, J. M., Régis, C., Gilot, E., Débias, F., et al. (2021). DNA methylation as a tool to explore ageing in wild roe deer populations. Mol. Ecol. Resour. 22, 1002–1015. doi: 10.1111/1755-0998.13533
Lev Maor, G., Yearim, A., and Ast, G. (2015). The alternative role of DNA methylation in splicing regulation. Trends Genet. 31, 274–280. doi: 10.1016/j.tig.2015.03.002
Li, Q., Li, N., Hu, X., Li, J., du, Z., Chen, L., et al. (2011). Genome-wide mapping of DNA methylation in chicken. PLoS One 6:e19428. doi: 10.1371/journal.pone.0019428
Lindstedt, S. L., and Calder, W. A. (1976). Body size and longevity in birds. Condor 78, 91–94. doi: 10.2307/1366920
Lu, A. T., Fei, Z., Haghani, A., and Robeck, T. R. (2021). Universal DNA methylation age across mammalian tissues. bioRxiv. [Preprint], 537. (Accessed January 12, 2023).
Lüdecke, D. (2018). sjPlot - Data Visualization for Statistics in Social Science. Zenodo. doi: 10.5281/zenodo.2400856
Lüdecke, D., Ben-Shachar, M. S., Patil, I., and Makowski, D. (2020). Extracting, computing and exploring the parameters of statistical models using R. J. Open Source Soft. 5:2445. doi: 10.21105/joss.02445
Lüdecke, D., Ben-Shachar, M. S., Patil, I., Waggoner, P., and Makowski, D. (2021). performance: an R package for assessment, comparison and testing of statistical models. J. Open Source Soft. 6:3139. doi: 10.21105/joss.03139
Maegawa, S., Hinkal, G., Kim, H. S., Shen, L., Zhang, L., Zhang, J., et al. (2010). Widespread and tissue specific age-related DNA methylation changes in mice. Genome Res. 20, 332–340. doi: 10.1101/gr.096826.109
Medawar, P. B. (1952). An Unsolved Problem of Biology: An Inaugural Lecture Delivered at University College, London, 6 December, 1951. London: H.K. Lewis and Company.
Melzer, D., Pilling, L. C., and Ferrucci, L. (2020). The genetics of human ageing. Nat. Rev. Genet. 21, 88–101. doi: 10.1038/s41576-019-0183-6
Meng, H., Joyce, A. R., Adkins, D. E., Basu, P., Jia, Y., Li, G., et al. (2010). A statistical method for excluding non-variable CpG sites in high-throughput DNA methylation profiling. BMC Bioinformatics 11:227. doi: 10.1186/1471-2105-11-227
Miller, J. L., and Grant, P. A. (2013). The role of DNA methylation and histone modifications in transcriptional regulation in humans. Subcell. Biochem. 61, 289–317. doi: 10.1007/978-94-007-4525-4_13
Miranda, T. B., and Jones, P. A. (2007). DNA methylation: the nuts and bolts of repression. J. Cell. Physiol. 213, 384–390. doi: 10.1002/jcp.21224
Moiron, M., Araya-Ajoy, Y. G., Teplitsky, C., Bouwhuis, S., and Charmantier, A. (2020). Understanding the social dynamics of breeding phenology: indirect genetic effects and assortative mating in a long-distance migrant. Am. Nat. 196, 566–576. doi: 10.1086/711045
Monaghan, P., Charmantier, A., Nussey, D. H., and Ricklefs, R. E. (2008). The evolutionary ecology of senescence. Funct. Ecol. 22, 371–378. doi: 10.1111/j.1365-2435.2008.01418.x
Nisbet, I. C. T., Apanius, V., and Friar, M. S. (2002). Breeding performance of very old common terns. J. Field Ornithol. 73, 117–124. doi: 10.2307/4131134?refreqid=search-gateway:4bfb029fc6e56d8d4007bab37bb2ef48
Nisbet, I., Iles, D., Kaneb, A., Auk, C. M. T., and Makowski, D. (2020). Breeding performance of common terns (Sterna hirundo) does not decline among older age classes. Amer. Zool. 137, 1–17. doi: 10.2307/4131134?refreqid=search-gateway:9aef603bed33e461fec379819c7194de
Nussey, D. H., Coulson, T., Festa-Bianchet, M., and Gaillard, J. M. (2008). Measuring senescence in wild animal populations: towards a longitudinal approach. Funct. Ecol. 22, 393–406. doi: 10.1111/j.1365-2435.2008.01408.x
Prado, N. A., Brown, J. L., Zoller, J. A., Haghani, A., Yao, M., Bagryanova, L. R., et al. (2021). Epigenetic clock and methylation studies in elephants. Aging Cell 20:e13414. doi: 10.1111/acel.13414
R Core Team, (2021). R: A Language and Environment for Statistical Computing. Available at: https://www.r-project.org/.
Raj, K., Szladovits, B., Haghani, A., Zoller, J. A., Li, C. Z., Black, P., et al. (2021). Epigenetic clock and methylation studies in cats. Geroscience 43, 2363–2378. doi: 10.1007/s11357-021-00445-8
Rhie, A., Mccarthy, S. A., Fedrigo, O., Damas, J., Formenti, G., Koren, S., et al. (2021). Towards complete and error-free genome assemblies of all vertebrate species. Nature 592, 737–746. doi: 10.1038/s41586-021-03451-0
Sen, P., Shah, P. P., Nativio, R., and Berger, S. L. (2016). Epigenetic Mechanisms of Longevity and Aging. Cell 166, 822–839. doi: 10.1016/j.cell.2016.07.050
Sepers, B., van den Heuvel, K., Lindner, M., Viitaniemi, H., Husby, A., and van Oers, K. (2019). Avian ecological epigenetics: pitfalls and promises. J. Ornithol. 160, 1183–1203. doi: 10.1007/s10336-019-01684-5
Shefferson, R. P., Jones, O. R., and Salguero-Gomez, R. (2017). The Evolution of Senescence in the Tree of Life. Cambridge: Cambridge University Press.
Sheldon, E. L., Schrey, A. W., Hurley, L. L., and Griffith, S. C. (2020). Dynamic changes in DNA methylation during postnatal development in zebra finches Taeniopygia guttata exposed to different temperatures. J. Avian Biol. 51:e02294. doi: 10.1111/jav.02294
Smith, Z. D., Gu, H., Bock, C., Gnirke, A., and Meissner, A. (2009). High-throughput bisulfite sequencing in mammalian genomes. Methods 48, 226–232. doi: 10.1016/j.ymeth.2009.05.003
Suzuki, M. M., and Bird, A. (2008). DNA methylation landscapes: provocative insights from epigenomics. Nat. Rev. Genet. 9, 465–476. doi: 10.1038/nrg2341
Sziráki, A., Tyshkovskiy, A., and Gladyshev, V. N. (2018). Global remodeling of the mouse DNA methylome during aging and in response to calorie restriction. Aging Cell 17:e12738. doi: 10.1111/acel.12738
Tan, Q., Heijmans, B. T., Hjelmborg, J. V. B., Soerensen, M., Christensen, K., and Christiansen, L. (2016). Epigenetic drift in the aging genome: a ten-year follow-up in an elderly twin cohort. Int J Epidemiol 45, dyw132–dyw1158. doi: 10.1093/ije/dyw132
Tharakan, R., Ubaida-Mohien, C., Moore, A. Z., Hernandez, D., Tanaka, T., and Ferrucci, L. (2020). Blood DNA methylation and aging: a cross-sectional analysis and longitudinal validation in the in CHIANTI study. J Gerontol A Biol Sci Med Sci 75, 2051–2055. doi: 10.1093/gerona/glaa052
Unnikrishnan, A., Freeman, W. M., Jackson, J., Wren, J. D., Porter, H., and Richardson, A. (2019). The role of DNA methylation in epigenetics of aging. Pharmacol. Ther. 195, 172–185. doi: 10.1016/j.pharmthera.2018.11.001
van de Pol, M., and Wright, J. (2009). A simple method for distinguishing within-versus between-subject effects using mixed models. Animal Behav. 77, 753–758. doi: 10.1016/j.anbehav.20
van Noordwijk, A. J., and de Jong, G. (1986). Acquisition and Allocation of Resources - Their Influence on Variation in Life-History Tactics. Am. Nat. 128, 137–142. doi: 10.1086/284547
Vaupel, J. W., and Yashin, A. I. (1985). Heterogeneity's ruses: some surprising effects of selection on population dynamics. Am Stat 39, 176–185. doi: 10.2307/2683925
Vedder, O., Moiron, M., Bichet, C., Bauch, C., Verhulst, S., Becker, P. H., et al. (2021a). Telomere length is heritable and genetically correlated with lifespan in a wild bird. Mol. Ecol. 31, 6297–6307. doi: 10.1111/mec.15807
Vedder, O., Pen, I., and Bouwhuis, S. (2021b). How fitness consequences of early-life conditions vary with age in a long-lived seabird: A Bayesian multivariate analysis of age-specific reproductive values. J Anim Ecol 90, 1505–1514. doi: 10.1111/1365-2656.13471
Watson, H., Salmón, P., and Isaksson, C. (2019). Dynamic changes in DNA methylation during embryonic and postnatal development of an altricial wild bird. Ecol. Evol. 9, 9580–9585. doi: 10.1002/ece3.5480
Williams, G. C. (1957). Pleiotropy, Natural-Selection, and the Evolution of Senescence. Evolution 11, 398–411. doi: 10.2307/2406060
Wilson, A. J., Charmantier, A., and Hadfield, J. D. (2008). Evolutionary genetics of ageing in the wild: empirical patterns and future perspectives. Funct. Ecol. 22, 431–442. doi: 10.1111/j.1365-2435.2008.01412.x
Yuan, T., Jiao, Y., de Jong, S., Ophoff, R. A., Beck, S., and Teschendorff, A. E. (2015). An integrative multi-scale analysis of the dynamic DNA methylation landscape in aging. PLoS Genet. 11:e1004996. doi: 10.1371/journal.pgen.1004996
Zampieri, M., Ciccarone, F., Calabrese, R., Franceschi, C., Buerkle, A., and Caiafa, P. (2015). Reconfiguration of DNA methylation in aging. Mech. Ageing Dev. 151, 60–70. doi: 10.1016/j.mad.2015.02.002
Zhang, G., Li, C., Li, Q., Li, B., Larkin, D. M., Lee, C., et al. (2014). Comparative genomics reveals insights into avian genome evolution and adaptation. Science 346, 1311–1320. doi: 10.1126/science.1251385
Zhang, H., Rebke, M., Becker, P. H., and Bouwhuis, S. (2015a). Fitness prospects: effects of age, sex and recruitment age on reproductive value in a long-lived seabird. J Anim Ecol 84, 199–207. doi: 10.1111/1365-2656.12259
Zhang, H., Vedder, O., Becker, P. H., and Bouwhuis, S. (2015b). Age-dependent trait variation: the relative contribution of within-individual change, selective appearance and disappearance in a long-lived seabird. J Anim Ecol 84, 797–807. doi: 10.1111/1365-2656.12321
Keywords: aging, avian senescence, epigenetics, ontogeny, RRBS, Sterna hirundo
Citation: Meyer BS, Moiron M, Caswara C, Chow W, Fedrigo O, Formenti G, Haase B, Howe K, Mountcastle J, Uliano-Silva M, Wood J, Jarvis ED, Liedvogel M and Bouwhuis S (2023) Sex-specific changes in autosomal methylation rate in ageing common terns. Front. Ecol. Evol. 11:982443. doi: 10.3389/fevo.2023.982443
Edited by:
Jean-Francois Lemaitre, UMR5558 Biométrie et Biologie Evolutive (LBBE), FranceReviewed by:
Gerald S. Wilkinson, University of Maryland, United StatesMarianthi Tangili, University of Groningen, Netherlands
Copyright © 2023 Meyer, Moiron, Caswara, Chow, Fedrigo, Formenti, Haase, Howe, Mountcastle, Uliano-Silva, Wood, Jarvis, Liedvogel and Bouwhuis. This is an open-access article distributed under the terms of the Creative Commons Attribution License (CC BY). The use, distribution or reproduction in other forums is permitted, provided the original author(s) and the copyright owner(s) are credited and that the original publication in this journal is cited, in accordance with accepted academic practice. No use, distribution or reproduction is permitted which does not comply with these terms.
*Correspondence: Britta S. Meyer, ✉ britta@bsmeyer.com
†These authors have contributed equally to this work and share senior authorship