Virtual Reality and EEG-Based Intelligent Agent in Older Adults With Subjective Cognitive Decline: A Feasibility Study for Effects on Emotion and Cognition
- 1Research Centre, Institut Universitaire de Gériatrie de Montréal, Montreal, QC, Canada
- 2Department of Psychology, Université de Montréal, Montreal, QC, Canada
- 3Department of Computer Science and Operational Research, Université de Montréal, Montreal, QC, Canada
- 4Department of Psychiatry and Addictology, Université de Montréal, Montreal, QC, Canada
Objectives: Immersive virtual reality has tremendous potential to improve cognition in populations with cognitive impairment. We conducted a feasibility and proof-of-concept study to assess the potential of virtual reality and electroencephalography, with or without an intelligent agent, that adapts the presented material to the emotions elicited by the environment.
Method: Older adults with subjective cognitive decline recruited from the community received a virtual reality-based intervention taking place in one of two virtual environments, a train (Part 1, N = 19) or a music theatre, complemented by the intelligent agent (Part 2, N = 19). A comparative control group (N = 19) receiving no intervention was also included. All participants completed measures of affect and cognition before and after the intervention. The intervention groups completed measures of cybersickness and user experience after the intervention.
Results: Participants did not suffer from increased cybersickness following either intervention. They also reported a positive to highly positive user experience concerning the following aspects: attractivity, hedonic quality-identity and hedonic quality-stimulation. The measures of affect showed no pre-post change when comparing either intervention to the control condition. However, a reduction of negative affect was observed following the train intervention for participants with a high self-reported negative affect at baseline. Finally, there was a significant improvement in working memory when comparing either intervention group to the control condition.
Conclusion: Our results support the feasibility and tolerability of the technology, and a positive impact on cognition, paving the way for a larger-scale randomized clinical trial to confirm efficacy.
Introduction
Immersive virtual reality (VR) has been increasingly used in clinical neuropsychology research and to treat neuropsychiatric symptoms (Maples-Keller et al., 2017; Ouellet et al., 2018; La Corte et al., 2019; Corriveau Lecavalier et al., 2020; Dermody et al., 2020). With VR, the user is usually immersed in a virtual environment (VE) through a motion-tracked head-mounted display and controllers, and therefore isolated from external visual and auditive distractions. The user can engage and interact with the environment: it is possible to look around by moving the head, explore by walking and interact with objects by grabbing and throwing for instance. These features create a feeling of presence, i.e., a feeling of being immersed in the environment displayed. VR can be used to incorporate cognitive and physical demands within virtual scenarios that mimic situations that a person faces in his/her everyday life. Thus, VR is extremely promising to increase the ecological validity of measures and interventions.
Given its malleability and various functionalities, VR has become one of the most compelling developments in rehabilitation for diverse populations including older adults. VR has many assets: it allows a high level of interaction in a safe environment; it can be adapted to various characteristics and individual needs; and it offers the potential to develop engaging and effective training with optimal transfer of gains to daily life. This technology could therefore be particularly useful in older adults with or without cognitive impairment and could be associated with other approaches to optimize cognitive vitality and/or prevent cognitive decline. Intervention and feasibility studies using VR have shown encouraging results in this context. The technology has been well tolerated by older adults (Ouellet et al., 2018; Corriveau Lecavalier et al., 2020; Dermody et al., 2020), and was found to contribute to programs aiming to improve cognitive function (Dermody et al., 2020), reduce anxiety disorders and possibly depression (Maples-Keller et al., 2017). However, more interventional and feasibility studies are needed in older adults to assess usability, accessibility and user experience. These studies will address whether the technology is considered accessible and easy to use, and ultimately adopted by older adults either in a clinical context or everyday life (e.g., at home) (Proctor et al., 2011; Holthe et al., 2018).
It is also critical to expand the use of VR to a diversity of older adults, including those who start to experience cognitive challenges or develop psychiatric symptoms. Older adults with subjective cognitive decline (SCD) complain and worry about their cognition. People with SCD perform normally on current neuropsychological tests but they progress to dementia at a higher rate than people with no subjective impression of a decline. Many may be in a very mild phase of Alzheimer’s disease or a related disorder, prior to the presence of cognitive impairment (Caillaud et al., 2020). This phase may be ideal to provide cognitive training because older adults with SCD are concerned and aware, and still have the cognitive capabilities to learn and apply new strategies (Lin et al., 2018). Therefore, older adults with SCD are excellent candidates to study VR-based intervention programs.
Because VR immerses individuals into vivid real-life scenarios, it is particularly important to adapt these to individual preferences. One promising avenue is using an electroencephalogram (EEG) to measure brain waves as participants are immersed in the VE and use the signal to contiguously adapt the environment to the participant’s emotional reaction. Using artificial intelligence, this technique can be used to personalize the VE and its contents in response to the user’s emotional state. Recently, Abdessalem and Frasson developed an intelligent agent system, which is a system that can adapt the presentation of content based on the behaviour and emotions of the VR user. EEG readings are analysed in real-time and the content is adapted based on the recorded brain waves with the goal of creating positive emotions (Abdessalem and Frasson, 2017). The system could track the user’s excitement and frustration after extraction from the EEG. The authors reported changes in excitement and frustration of younger adults in response to changes in speed and difficulty of a VR game triggered by the intelligent agent. By having a positive impact on emotions, this approach could also positively impact cognition. Negative emotions have been associated with reduced cognitive and memory performance, and positive emotions with increased performance, particularly for working memory (WM) and controlled attention tasks (Williams et al., 1996; Blair et al., 2007; Verbruggen and De Houwer, 2007; Conway et al., 2013; Su et al., 2017; Garrison and Schmeichel, 2019). Emotions have therefore been proposed to have a mediating effect on these cognitive components. Older adults with SCD have higher levels of neuropsychiatric symptoms during the course of their cognitive decline (Robert et al., 2006; Köhler et al., 2010; Guercio et al., 2015; Lanctôt et al., 2017; Sherman et al., 2018), which in turn impact their emotional state. Therefore, they could greatly benefit from a technology that adapts the presentation of stimulus to its emotional impact. This intelligent agent combined with VR may support more personalized rehabilitation strategies in people with cognitive decline. These technologies could also be particularly useful for older adults who may have negative reactions to technology-based interventions but whose cognitive limitations prevent them from expressing their preferences. Thus, the combined use of the VR and EEG-based technology could be beneficial to promote well-being through emotional state and cognition improvement.
As a first step toward developing a VR- and EEG-based intelligent agent system, we created two VEs (Biamonti et al., 2014; Gómez Gallego and Gómez García, 2017): 1) a train (train intervention) and 2) a music theatre, complemented by the intelligent agent (music intervention). We tested the two VEs during a feasibility study in older adults with SCD1 and aimed to determine tolerability and user experience after their completion. For the proof-of-concept study, preliminary data on the VEs’ impact on self-reported emotion and objective measures of attention and WM, two cognitive domains that have been found to be sensitive to emotional variation (Abdessalem et al., 2020; Byrns et al., 2020), were compared to a no-contact control condition.
Material and Methods
Participants
Included participants were French-speaking, aged 60 and over, with normal or corrected-to-normal vision and hearing, and met recognized criteria for SCD (Belleville et al., 2019). Participants were excluded from the study if they had a history of major psychiatric illness, neurological disorders that could explain the cognitive complaint, alcoholism, significant impairment of physical mobility or the use of hands and known cybersickness or motion sickness. In total, 45 older adults with SCD participated in the study. Nineteen participants (11 female) were included in the train intervention, and nineteen (13 female) were included in the music intervention, of which twelve had also participated in the train intervention. Nineteen people (16 female) were included in the control condition. The sample size was not determined from a power analysis as this was a proof-of-concept study aiming to provide information on tolerability and user experience, and therefore did not target efficacy.
All participants were recruited through the bank of participants of the Institut universitaire de gériatrie de Montréal Research Centre (CRIUGM). Initial contact with prospective participants was conducted over the phone and individuals deemed eligible were invited to visit the CRIUGM. During their visit, they received the information and consent form and completed a brief clinical examination: MoCA (Nasreddine et al., 2005); WMS-IV Logical Memory subtest; Diagnostic Criteria for Apathy (DCA) (Robert et al., 2018); Mild Behavioral Impairment Checklist (MBI-C) (Ismail et al., 2017); Big Five Personality Test (50 items) (Goldberg, 1992).
The study was done in accordance with the Declaration of Helsinki and approved by the Research Ethics Board (REB) - vieillissement-neuroimagerie - of the CIUSSS-CSMTL (application #18–34).
Design
Both the interventions and the control condition were completed during a single visit to the CRIUGM. For participants who completed both the train and the music interventions, there was a delay of at least 3 months between visits. Participants began each visit with a pre-intervention assessment, during which they filled out questionnaires assessing cybersickness and emotions. They then completed six computerized cognitive tasks to measure WM and attention. Participants were then exposed to one of the VEs, which lasted 5 minutes for the train and 10 minutes for the music intervention. Finally, participants completed the post-intervention assessment, including measures of cybersickness and user experience (first goal), as well as questionnaires on emotions and cognitive tasks (second goal). Participants from the control group completed cognitive tasks and questionnaires on emotions twice, separated by a 10-min discussion with a member of the research team on topics unrelated to the study. Parallel versions of the cognitive tasks were used at pre- and post-intervention to reduce learning effects. These were presented in a fixed order across participants.
Interventions
Both VEs were created with Unity 3D and presented with the Fove0 VR headset (Fove Inc., Torrance, CA, US). Concurrently, brain waves were recorded with the Epoc + portable EEG headset (Emotiv, San Franscisco, CA, US) (Abdessalem et al., 2020; Byrns et al., 2020). The headset includes 14 electrodes spatially organized according to the international 10–20 system (AF3, AF4, F3, F4, F7, F8, FC5, FC6, P7, P8, T7, T8, O1, O2). Raw EEG was captured at the rate of 128Hz and provided the power of five frequency bands (theta, alpha, low and high beta, gamma). EEG recording was supplemented by an intelligent agent in the music intervention only.
In the train intervention, the participant was seated in a virtual train, where he/she could see different changing landscapes (e.g., forest, snowy mountains, sunny desert, Figure 1A). Participants could turn their head to explore the environment around them. The speed of the train was the same for all participants and was set to allow a proper observation of the environment without being too slow. There was no intelligent agent, as our goal was to assess the feasibility and experience related to a realistic and peaceful travel environment.
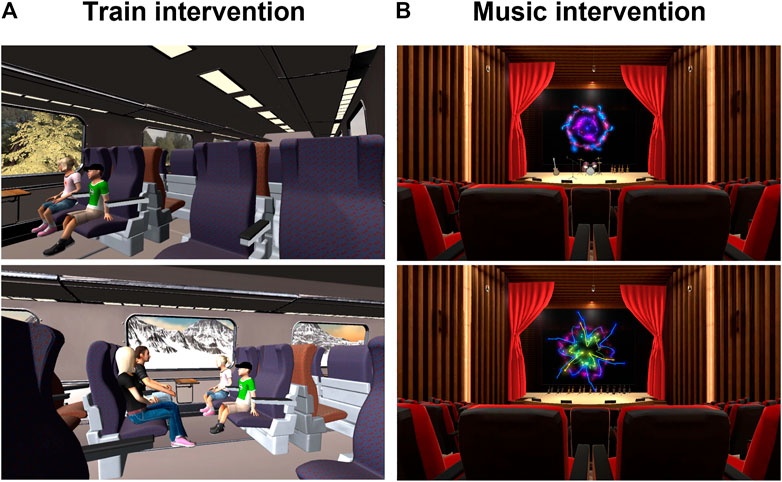
FIGURE 1. Virtual train and music theatre. Examples of what a user can experienced when immersed in the virtual train (A) or the virtual music theatre (B).
In the music intervention, participants were seated in a theater and were presented with eight different 30 s musical pieces from different repertoires (e.g., Clair De Lune, Debussy; La Vie En Rose, Piaf (de la Torre-Luque et al., 2017)) accompanied by animated instruments and firework-like visual effects (Figure 1B) (Valdez and Mehrabian, 1994). While pieces were being played, the intelligent agent recorded brain waves and analyzed them to provide an estimate of its emotional impact. This information was used to determine the piece that was associated with the highest positivity value. This piece was then presented for 2 minutes.
Outcome Measures
Cybersickness and User Experience Questionnaires
Cybersickness was measured with the Cybersickness Questionnaire (CSQ (Sevinc and Berkman, 2020)) using nine items rated on a 3-point Likert scale from 0 (not at all) to 2 (moderately). The CSQ comprises two subscales, “dizziness” (maximum scores: 7.52) and “difficulty in focusing” (maximum score: 5.66). The AttrakDiff 2 scale is a validated tool to evaluate user experience (Lallemand et al., 2015). The scale includes 28 items combined to measure different dimensions: Pragmatic Quality (PQ), Attractiveness (ATT) and Hedonic Quality (HQ), which is subdivided into Stimulation (HQ-S) and Identity (HQ-I) dimensions. Each item consisted of a pair of opposed adjectives ranked with a score from -3 (for example extremely unpleasant) to +3 (for example extremely pleasant). A score of 0 means that the item was considered neutral (for example neither unpleasant nor pleasant). Data are presented using the scores on individual word-pairs, the combined scores for the four categories (PQ, ATT, HQ-S, HQ-I) or by plotting HQ against PQ (known as the portfolio results).
Affect and Cognitive Measures
The positive and negative affect schedule (PANAS) scale (Watson et al., 1988) comprises 10 items measuring positive affect (PA) and 10 measuring negative affect (NA) (maximum score for each scale = 50).
Four computerized WM tasks and two attentional tasks were used. In WM task 1, participants were asked to memorize six images of objects and written words (maximum score = 6) (Nasreddine et al., 2005). In WM task 2, they had to memorize a sequence of two sets of four and six circles presented one by one (maximum score = 10) (Nasreddine et al., 2005). WM task three involved memorizing a set of three images presented simultaneously. This task was repeated twice (maximum score = 2) (Nasreddine et al., 2005). In WM task 4, participants were asked to record two series of orally-presented digits on a numerical pad (first series: five digits, order of presentation; second series: three digits, backward order; maximum score = 8) (Leark et al., 2007). In attention task 1, participants were asked to indicate when the letter “A” was displayed in a series of presented letters (“A” presented 11 times among a series of 30 letters: maximum score = 11) (Asato et al., 2006). In attention task 2, they were presented with four letters and asked to indicate the letter corresponding to the first letter of the name of an image that was previously presented. This task was repeated six times (maximum score = 6) (Leark et al., 2007).
Statistical Analyses
All statistical tests were done using IBM SPSS Statistics V26 (Armonk, NY, United States). They were two-tailed and a p value <0.05 was chosen for statistical significance. All analyses were done separately for the train and music interventions. Ordinal data were analyzed with parametric tests rather than non-parametric scales as they provide a more in-depth analysis, and they are robust to violations of data assumptions and are more versatile, powerful and comprehensive (Norman, 2010).
Group differences for clinical characteristics were tested between each intervention group and the controls at baseline, with a t-test or χ2 test as appropriate. For the cybersickness scale, the pre-post score difference was tested for each subscale using paired-sample t-tests.
Data for the word-pair dimensions and categories were analyzed with confidence intervals (CI) for the AttrakDiff two scales, which are only measured at post-intervention. A score was considered as significantly different from a neutral assessment when the 95% CI did not cross zero. Significantly different scores that were larger than two or minus two were considered as highly positive or highly negative, respectively. To assess the intervention effect on the PANAS, as PA and NA scales are correlated (Crawford and Henry, 2004), we used MANOVAs with group (train or music vs control) as a between-subject factor and time (pre-vs post-) as a within-subject factor. Per-item analysis of the PA and NA scales were also performed with MANOVAs. We conducted additional exploratory analyses to better assess pre-post intervention effects on PA and NA. We categorized participants with low vs high baseline PA and NA values and analyzed these data with mixed ANOVAs using baseline PANAS score (low vs high) as a between-subject factor and time (pre-vs post-) as a within-subject factor. This was done separately for each group (train, music and control) as our sample size was too small to test three-way interactions. Least Significant Difference (LSD) post-hoc tests were conducted when a significant baseline score x time interaction was found. The intervention effects on cognitive measures were analyzed separately for each task and intervention group using mixed ANOVAs with group (train or music vs control) as a between-subject factor and time (pre-vs post-) as a within-subject factor. LSD post-hoc tests were conducted when a significant interaction was found.
Results
Participants’ Demographic and Clinical Characteristics
Baseline characteristics of participants were similar when comparing controls to the train or music intervention groups, except for two personality traits (Table 1): Participants from the control condition were less extraverted than these from the music intervention (p < 0.05) and less agreeable than these from both train and music interventions (p < 0.01 for both).
Cybersickness Symptoms and User Experience
No significant post-vs. pre-intervention differences were found for both vertigo (0.23 (0.37) vs. 0.33 (0.68) for train intervention; 0.59 (0.81) vs. 0.80 (0.96) for music intervention) and difficulty to focus (0.13 (0.33) vs. 0.15 (0.40) for train intervention; 0.38 (0.58) vs. 0.64 (0.77)) for music intervention) subscales of the CSQ. Results on the user experience questionnaires are shown in Figures 2A,B. For both environments, a few word-pairs were considered in the neutral range (with 95% CI between -1 and 1): unpredictable-predictable; isolating-connective; separates me from people/brings me closer to other people. The technical-human word-pair was also perceived as neutral for the train environment while the music environment was considered technical. Several other word-pairs describing the music intervention were ranked with a highly positive score, while this was not the case for the train intervention (Figure 2B). All user experience dimensions were perceived as positive or highly positive, except for PQ in the music intervention. The portfolio presentation (Figure 2C), which focuses on HQ and PQs, indicates that both interventions were judged as self-oriented or desired. The train intervention could also be characterized as neutral or task-oriented.
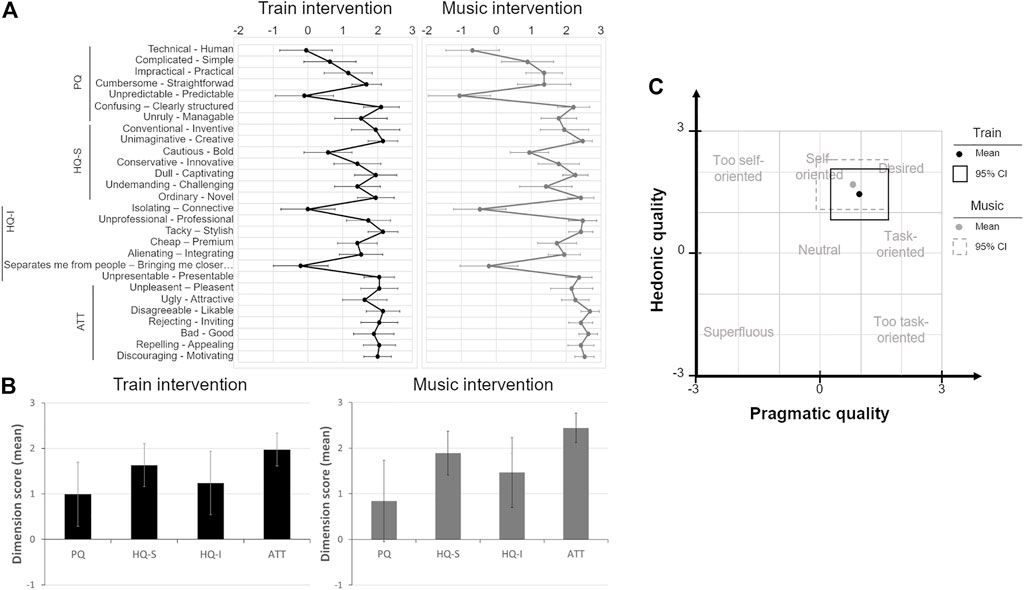
FIGURE 2. User experience for the train and music intervention Mean scores (±95% CI) for the AttrakDiff two word-pairs (A) and dimensions (B) for the train (dark black symbols and lines) and music intervention (grey symbols and lines). In (C), PQ and HQ are plotted on the x and y axes, respectively. Black and dashed grey boxes represent the 95% CI for the train and music interventions, respectively. Framed zones represent different characteristics based on the relationship between PQ and HQ (e.g., the virtual environment is considered as “desired” when a score is located within the box with scores ranging from two to three in both PQ and HQ). ATT, Attractivity; CI, Confidence Interval; HQ-I, Hedonic Quality-Identity; HQ-S, Hedonic Quality-Stimulation.
Affect Measures
The MANOVA on the PANAS scores for the train intervention revealed no significant main effect of group, F (2,32) = 2.24, p = 0.12, Wilk’s Λ = 0.88, time, F (2,32) = 0.10, p = 0.90, Wilk’s Λ = 0.99, or group x time interaction, F (Valdez and Mehrabian, 1994; Dermody et al., 2020) = 0.49, p = 0.62, Wilk’s Λ = 0.97 (Supplementary Figures 1A,B). For the music intervention, the MANOVA yielded no significant main effect of group, F (2,35) = 0.88, p = 0.42¸Wilk’s Λ = 0.95), time, F (2,35) = 0.46, p = 0.64, Wilk’s Λ = 0.97), or group × time interaction, F (2,35) = 0.62, p = 0.54, Wilk’s Λ = 0.97) (Supplementary Figures 1C,D). No significant effect was found for any of the fixed factors and their interaction when analyzing the different NA and PA items in both the train and the music intervention (Supplementary Table 1), except for a main effect of time in the NA items for the music intervention (F (10,26) = 2.41, p < 0.05, Wilk’s Λ = 0.52; Supplementary Table S1).
The ANOVA examining the train intervention effect on PA in participants with high vs low baseline scores (Supplementary Figure 2A) yielded a significant main effect of baseline score (F (1,14) = 8.02, p < 0.05, ωp2 = 0.31), but no main effect of time (F (1,14) = 0.18, p = 0.68) or baseline score × time interaction (F (1,14) = 1.63, p = 0.22). The ANOVA results on NA (Supplementary Figure 2B) indicated a significant main effect of baseline score (F (1,16) = 25.47, p < 0.001, ωp2 = 0.58), time (F (1,16) = 5.98, p < 0.05, ωp2 = 0.12), and baseline score × time interaction (F (1,16) = 6.61, p < 0.05, ωp2 = 0.14). This was due to a decrease of NA in the high baseline group only (mean difference of 4.0, p < 0.01; and 0.1, p = 0.93 for the high and low baseline groups, respectively). In addition, the significant difference observed between the two groups at baseline (mean difference of 6.17, p < 0.001) was no longer significant after the intervention (mean difference of 2.1, p = 0.13).
The ANOVA examining the music intervention effect on PA in high vs low baseline participants revealed a main effect of baseline score (Supplementary Figure 2C) (F (1,17) = 14.61, p < 0.001, ωp2 = 0.42) but no main effect of time (F (1,17) = 1.43, p = 0.25) or baseline score × time interaction (F (1,17) = 0.24, p = 0.63). The results of the ANOVA on NA showed (Supplementary Figure 2D) a main effect of baseline score (F (1,17) = 20.73, p < 0.001, ωp2 = 0.51), but no main effect of time (F (1,17) = 0.087, p = 0.77) or baseline score × time interaction (F (1,17) = 0.035, p = 0.85).
In the control group, there was a main effect of baseline score (F (1,17) = 37.4, p < 0.0001, ωp2 = 0.31) for PA, but no main effect of time (F (1,17) = 0.26, p = 0.61) or baseline score × time interaction (F (1,17) = 3.24, p = 0.09) (Supplementary Figure 2E), as expected. Similarly, the ANOVA on NA in the control group yielded no significant main effect of baseline score (F (1,17) = 6.9, p < 0.05, ωp2 = 0.31), time (F (1,17) = 0.30, p = 0.59); or baseline score × time interaction (F (1,17) = 0.10, p = 0.76) (Supplementary Figure 2F).
Cognitive Measures
When assessing the effect of the train intervention on WM task 1 (Figure 3A), the ANOVA revealed no significant main effect of group (F (1,36) = 4.13, p = 0.05), or time (F (1,36) = 1.05, p = 0.31), but a significant interaction (F (1,36) = 9.44, p < 0.01; ωp2 = 0.06). Post-hoc tests revealed a significant mean post-pre change score in the intervention group (mean change = 0.63, p < 0.001) that was absent in controls (mean change = -0.32, p = 0.16). For WM task 2, the ANOVA yielded no main effect of group (F (1,36) = 0.12, p = 0.73), or time (F (1,36) = 0.59, p = 0.45), but a significant interaction (F (1,36) = 8.27, p < 0.01; ωp2 = 0.08) (Figure 3B). Pairwise comparisons show that the mean post-pre change score was significant in the intervention group (mean change = 2.0, p < 0.05), but not in the control group (mean change = -1.16, p = 0.16). No significant main effects or interactions were observed for WM tasks 3 (Figure 3C) and 4, and attention tasks one and 2. However, a significant main effect of time (F (1,36) = 4.56, p < 0.05; ωp2 = 0.06) was found on attention task two scores (Supplementary Table S2).
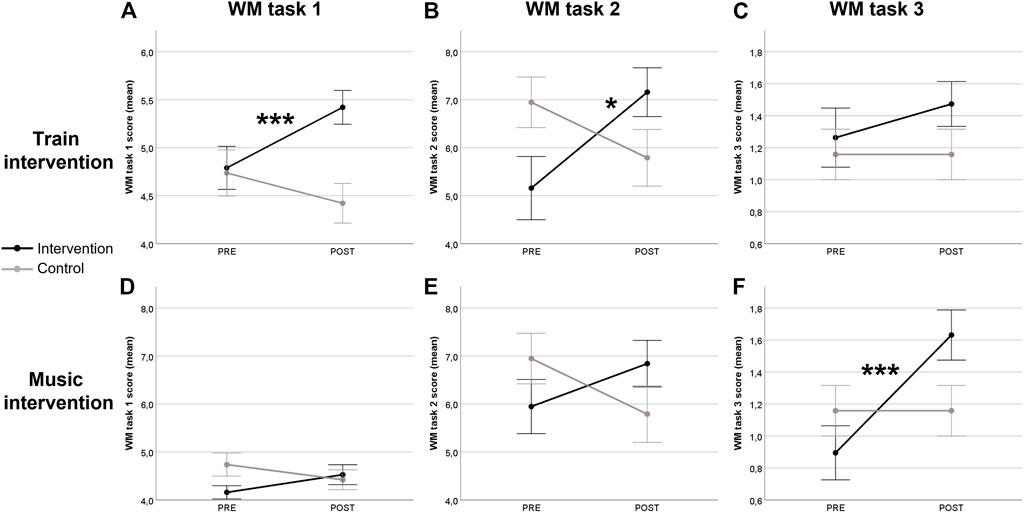
FIGURE 3. Pre- and post-intervention scores in working memory tasks 1, two and 3. Mean score (±SEM) on the working memory task 1 (A–D), 2 (B–E) and 3 (C–F) before (PRE) and after (POST) the train and the music intervention in comparison to the control condition. POST, post-intervention; PRE, pre-intervention; WM, Working Memory; #p < 0.05 and ##p < 0.01 for post-hoc tests comparing pre-vs post-intervention scores when interaction was significant.
The ANOVA examining the music intervention effect on WM task 1 (Figure 3D) indicated no main effect of group (F (1,36) = 0.95, p = 0.34), or time (F (1,36) = 0.03, p = 0.86), but a significant interaction (F (1,36) = 5.24, p < 0.05; ωp2 = 0.03). Post hoc tests indicated that the mean post-pre change score was not significant in both intervention and control groups (Music: mean change = 0.37, p = 0.09; Ctrl: mean change = -0.32, p = 0.14). The ANOVA on WM task 2 (Figure 3E) revealed no main effect of group, (F (1,36) = 0.002, p = 97, or time, F (1,36) = 0.08, p = 0.77), but a significant interaction (F (1,36) = 5.03, p < 0.01; ωp2 = 0.04). Pairwise comparisons showed no significant mean post-pre change score in both intervention and control groups (Music: mean change = 0.89, p = 0.17; Ctrl: mean change = -1.16, p = 0.08). The ANOVA on WM task 3 (Figure 3F) yielded no significant main effect of group (F (1,36) = 0.28, p = 0.60) or time, F (1,36) = 11.84, p < 0.01), but a significant interaction (F (1,36) = 11.84, p < 0.01; ωp2 = 0.06). Post hoc tests revealed that the mean post-pre change score was significant in the intervention group (mean change = 0.74; p < 0.0001) while it was absent in controls (mean change = 0.00; p = 1.00). No significant main effects or interactions were observed when analyzing the influence of the music intervention on WM task 4, and attention tasks one and 2 (Supplementary Table S3).
Discussion
To our knowledge, this is the first study to address the tolerability and user experience of fully immersive VEs aimed at improving emotional state and cognition in individuals with SCD. Our first goal was to measure cybersickness and user experience. Our results indicate that participants appreciated and tolerated both train and music VEs well. Our second goal was to assess the impact of each intervention on self-reported emotion and objective measures of WM and attention. NA decreased following the train intervention in participants reporting a high level of NA at baseline. Interestingly, preliminary results indicated that WM may benefit from both interventions, but not attention.
While interventions using VR have gained momentum (Kim et al., 2019), only a few feasibility studies have been conducted in populations of older adults (Manera et al., 2016; Ouellet et al., 2018; Corriveau Lecavalier et al., 2020). Feasibility studies are important to ensure that an effective intervention will be tolerated, appreciated and accepted, and therefore useful to the target population. Here, we extend results from previous studies by showing that older adults with SCD, who are at risk of dementia, did not experience cybersickness symptoms following brief exposure to fully immersive VR.
User experience is a critical element to determine future use of the technology and to guide developers, who can adapt applications to provide the best experience for end users (Law, 2011). This is especially true for older adults, who are not as experienced with technology as younger people and will adopt it only when it is adapted to their capabilities (Proctor et al., 2011; Holthe et al., 2018). Here, participants positively perceived the hedonic quality (i.e., quality aspects not directly related to functionalities) of both VEs, perceiving them positively for the following: creativity, novelty, professionalism, stylishness and presentability. Attractivity (i.e., level of appeal) was also perceived positively in both VEs. The music environment was considered highly likable, inviting, good, appealing and motivating. However, there were some word-pairs that were seen as neutral: technical/human, unpredictable/predictable, favouring isolation/connection and separates me from people/brings me closer to other people. As these characteristics are inherent to VR technology (i.e., VR is a technology and being immersed in a VE separates the user from others), neutral values should not be interpreted as reflecting a non-satisfactory user experience. Instead, this suggests that our VEs have a good ecological value as this purely technical experience was rated as equally technical and human. Nevertheless, these impersonality factors must be carefully examined for future applications in people who may be more sensitive to these factors, such as individuals with affective or social disorders.
No pre-post intervention effect on self-reported affect was observed when examining the whole group of participants. However, a decrease in NA was detected following the train intervention in participants who had reported a higher NA score at baseline. This is aligned with previous results indicating decreased frustration measured with EEG markers (Abdessalem et al., 2020). Surprisingly, the music intervention personalized with the intelligent agent did not improve PA or NA. EEG analyses may possibly provide more sensitive measures of negative emotions (Byrns et al., 2020). Future studies will need to assess these variables further with a larger sample size.
In addition to the effects on emotions, both interventions induced a small improvement in WM compared to the control group. WM is a cognitive function that decreases greatly with age (Saba and Blanchet, 2020) and has been found to be sensitive to emotional variability (Garrison and Schmeichel, 2019). This indicates that WM may benefit from VR interventions rather than being due to a practice effect. This effect was not observed consistently across the different tasks and interventions for reasons that cannot be fully understood, given the differences between the two studies and the small sample size (Abdessalem et al., 2020; Byrns et al., 2020). Regardless, these results are in accordance with previous studies indicating that emotional states are linked to cognitive processes, such as WM (Williams et al., 1996; Blair et al., 2007; Verbruggen and De Houwer, 2007; Conway et al., 2013; Su et al., 2017; Garrison and Schmeichel, 2019).
There are some limitations to this study. First, as this was designed as a feasibility and proof-of-concept study, our sample size was small, and the study was not designed to assess efficacy. Given these encouraging results, we plan to conduct a larger-scale study to confirm them. Second, this is not a fully balanced design: There was no intelligent agent presented in the train intervention because its primary goal was to assess whether a travel environment was well-tolerated and reduced negative emotions. Lastly, we only tested older adults with SCD, and it would be interesting to address the same questions in older adults with cognitive impairments and/or neuropsychiatric symptoms. These populations could greatly benefit from such interventions contributing to well-being.
In conclusion, this feasibility study successfully showed that VR interventions are well-tolerated and appreciated, and may induce beneficial changes in emotional states and improvements in cognition. This study opens the way to the completion of a larger-scale study focusing on the interventions’ efficacy.
Data Availability Statement
The raw data supporting the conclusion of this article will be made available by the authors, without undue reservation.
Ethics Statement
The studies involving human participants were reviewed and approved by the Research Ethics Board (REB) vieillissement-neuroimagerie of the CIUSSS-CSMTL. The patients/participants provided their written informed consent to participate in this study.
Author Contributions
MC, HBA, M-AB, AB, CF, and SB participated in the conceptualization of the study and design. MC wrote the first version of the manuscript. SB revised and contributed to the writing of the first version. All authors carefully revised the final manuscript and accepted the final submitted version.
Funding
This work was supported by a grant received from NSERC-CRD, Prompt and Beam Me Up (Grant number CRSNG CRDPJ-522279-17). SB holds a Canada Research Chair in Cognitive Neuroscience of Aging and Brain Plasticity.
Conflict of Interest
The authors declare that the research was conducted in the absence of any commercial or financial relationships that could be construed as a potential conflict of interest.
Publisher’s Note
All claims expressed in this article are solely those of the authors and do not necessarily represent those of their affiliated organizations, or those of the publisher, the editors and the reviewers. Any product that may be evaluated in this article, or claim that may be made by its manufacturer, is not guaranteed or endorsed by the publisher.
Acknowledgments
We warmly thank the members of the research team who have been involved in various aspects of the preparation of the study: Mehdi Essounni, Vincent Marcangeli and Marie-Claude Veilleux.
Supplementary Material
The Supplementary Material for this article can be found online at: https://www.frontiersin.org/articles/10.3389/frvir.2021.807991/full#supplementary-material
Footnotes
1Partial data on emotional EEG measures were published in conference proceedings (Abdessalem et al., 2020; Byrns et al., 2020)
References
Abdessalem, H. B., Byrns, A., Cuesta, M., Manera, V., Robert, P., Bruneau, M-A., et al. (2020). “Application of Virtual Travel for Alzheimer's Disease,” in Conference: 9th International Conference on Sensor Networks (SENSORNETS). doi:10.5220/0008976700520060
Abdessalem, H. B., and Frasson, C. (2017). “Real-time Brain Assessment for Adaptive Virtual Reality Game: A Neurofeedback Approach,” in international conference on brain function assessment in learning (Springer). doi:10.1007/978-3-319-67615-9_12
Asato, M. R., Sweeney, J. A., and Luna, B. (2006). Cognitive Processes in the Development of TOL Performance. Neuropsychologia 44 (12), 2259–2269. doi:10.1016/j.neuropsychologia.2006.05.010
Belleville, S., LeBlanc, A. C., Kergoat, M. J., Calon, F., Gaudreau, P., Hébert, S. S., et al. (2019). The Consortium for the Early Identification of Alzheimer's Disease-Quebec (CIMA‐Q). Alzheimer's Demen. Diagn. Assess. Dis. Monit. 11 (1), 787–796. doi:10.1016/j.dadm.2019.07.003
Biamonti, A., Gramegna, S., and Imamogullari, B. (2014). A Design Experience for the Enhancement of the Quality of Life in People with Alzheimer's. What's on. Aveiro: PRT.
Blair, K. S., Smith, B. W., Mitchell, D. G. V., Morton, J., Vythilingam, M., Pessoa, L., et al. (2007). Modulation of Emotion by Cognition and Cognition by Emotion. Neuroimage 35 (1), 430–440. doi:10.1016/j.neuroimage.2006.11.048
Byrns, A., Abdessalem, H. B., Cuesta, M., Bruneau, M-A., Belleville, S., and Frasson, C. (2020). “Adaptive Music Therapy for Alzheimer’s Disease Using Virtual Reality,” in International Conference on Intelligent Tutoring Systems (Springer).
Caillaud, M., Hudon, C., Boller, B., Brambati, S., Duchesne, S., Lorrain, D., et al. (2020). Evidence of a Relation between Hippocampal Volume, white Matter Hyperintensities, and Cognition in Subjective Cognitive Decline and Mild Cognitive Impairment. The Journals Gerontol. Ser. B. 75 (7), 1382–1392. doi:10.1093/geronb/gbz120
Conway, A. M., Tugade, M. M., Catalino, L. I., and Fredrickson, B. L. (2013). The Broaden-And-Build Theory of Positive Emotions: Form, Function and Mechanisms. Oxford handbook of happiness, 17–34.
Corriveau Lecavalier, N., Ouellet, É., Boller, B., and Belleville, S. (2020). Use of Immersive Virtual Reality to Assess Episodic Memory: a Validation Study in Older Adults. Neuropsychological Rehabil. 30 (3), 462–480. doi:10.1080/09602011.2018.1477684
Crawford, J. R., and Henry, J. D. (2004). The Positive and Negative Affect Schedule (PANAS): Construct Validity, Measurement Properties and Normative Data in a Large Non-clinical Sample. Br. J. Clin. Psychol. 43 (3), 245–265. doi:10.1348/0144665031752934
de la Torre-Luque, A., Caparros-Gonzalez, R. A., Bastard, T., Vico, F. J., and Buela-Casal, G. (2017). Acute Stress Recovery through Listening to Melomics Relaxing Music: a Randomized Controlled Trial. Nordic J. Music Ther. 26 (2), 124–141. doi:10.1080/08098131.2015.1131186
Dermody, G., Whitehead, L., Wilson, G., and Glass, C. (2020). The Role of Virtual Reality in Improving Health Outcomes for Community-Dwelling Older Adults: Systematic Review. J. Med. Internet Res. 22 (6), e17331. doi:10.2196/17331
Garrison, K. E., and Schmeichel, B. J. (2019). Effects of Emotional Content on Working Memory Capacity. Cogn. Emot. 33 (2), 370–377. doi:10.1080/02699931.2018.1438989
Goldberg, L. R. (1992). The Development of Markers for the Big-Five Factor Structure. Psychol. Assess. 4 (1), 26–42. doi:10.1037/1040-3590.4.1.26
Gómez Gallego, M., and Gómez García, J. (2017). Music Therapy and Alzheimer's Disease: Cognitive, Psychological, and Behavioural Effects. Neurología (English Edition) 32 (5), 300–308. doi:10.1016/j.nrleng.2015.12.001
Guercio, B. J., Donovan, N. J., Ward, A., Schultz, A., Lorius, N., Amariglio, R. E., et al. (2015). Apathy Is Associated with Lower Inferior Temporal Cortical Thickness in Mild Cognitive Impairment and normal Elderly Individuals. Jnp 27 (1), e22–e27. doi:10.1176/appi.neuropsych.13060141
Holthe, T., Halvorsrud, L., Karterud, D., Hoel, K.-A., and Lund, A. (2018). Usability and Acceptability of Technology for Community-Dwelling Older Adults with Mild Cognitive Impairment and Dementia: a Systematic Literature Review. Cia Vol 13, 863–886. doi:10.2147/cia.s154717
Ismail, Z., Agüera-Ortiz, L., Brodaty, H., Cieslak, A., Cummings, J., Fischer, C. E., et al. (2017). The Mild Behavioral Impairment Checklist (MBI-C): a Rating Scale for Neuropsychiatric Symptoms in Pre-dementia Populations. Jad 56 (3), 929–938. doi:10.3233/jad-160979
Kim, O., Pang, Y., and Kim, J.-H. (2019). The Effectiveness of Virtual Reality for People with Mild Cognitive Impairment or Dementia: a Meta-Analysis. BMC psychiatry 19 (1), 219. doi:10.1186/s12888-019-2180-x
Köhler, S., van Boxtel, M. P. J., van Os, J., Thomas, A. J., O'Brien, J. T., Jolles, J., et al. (2010). Depressive Symptoms and Cognitive Decline in Community-Dwelling Older Adults. J. Am. Geriatr. Soc. 58 (5), 873–879. doi:10.1111/j.1532-5415.2010.02807.x
La Corte, V., Sperduti, M., Abichou, K., and Piolino, P. (2019). Episodic Memory Assessment and Remediation in normal and Pathological Aging Using Virtual Reality: a Mini Review. Front. Psychol. 10, 173. doi:10.3389/fpsyg.2019.00173
Lallemand, C., Koenig, V., Gronier, G., and Martin, R. (2015). Création et validation d'une version française du questionnaire AttrakDiff pour l'évaluation de l'expérience utilisateur des systèmes interactifs. Eur. Rev. Appl. Psychol. 65 (5), 239–252. doi:10.1016/j.erap.2015.08.002
Lanctôt, K. L., Amatniek, J., Ancoli-Israel, S., Arnold, S. E., Ballard, C., Cohen-Mansfield, J., et al. (2017). Neuropsychiatric Signs and Symptoms of Alzheimer's Disease: New Treatment Paradigms. Alzheimer's Demen. Translational Res. Clin. Interventions 3 (3), 440–449. doi:10.1016/j.trci.2017.07.001
Law, E. L-C. (2011). “The Measurability and Predictability of User Experience,” in Proceedings of the 3rd ACM SIGCHI symposium on Engineering interactive computing systems.
Leark, R. A., Greenberg, L. M., Kindschi, C. L., Dupuy, T. R., and Hughes, S. J. (2007). Test of Variables of Attention Continuous Performance Test. Los Alamitos: The TOVA Company.
Lin, Y., Shan, P. Y., Jiang, W. J., Sheng, C., and Ma, L. (2018). Subjective Cognitive Decline: Preclinical Manifestation of Alzheimer's Disease. Neurol. Sci. 40 (1), 41–49. doi:10.1007/s10072-018-3620-y
Manera, V., Chapoulie, E., Bourgeois, J., Guerchouche, R., David, R., Ondrej, J., et al. (2016). A Feasibility Study with Image-Based Rendered Virtual Reality in Patients with Mild Cognitive Impairment and Dementia. PloS one 11 (3), e0151487. doi:10.1371/journal.pone.0151487
Maples-Keller, J. L., Bunnell, B. E., Kim, S.-J., and Rothbaum, B. O. (2017). The Use of Virtual Reality Technology in the Treatment of Anxiety and Other Psychiatric Disorders. Harv. Rev. Psychiatry 25 (3), 103–113. doi:10.1097/hrp.0000000000000138
Nasreddine, Z. S., Phillips, N. A., Bã©dirian, V. r., Charbonneau, S., Whitehead, V., Collin, I., et al. (2005). The Montreal Cognitive Assessment, MoCA: a Brief Screening Tool for Mild Cognitive Impairment. J. Am. Geriatr. Soc. 53 (4), 695–699. doi:10.1111/j.1532-5415.2005.53221.x
Norman, G. (2010). Likert Scales, Levels of Measurement and the “Laws” of Statistics. Adv. Health Sci. Educ. 15 (5), 625–632. doi:10.1007/s10459-010-9222-y
Ouellet, É., Boller, B., Corriveau-Lecavalier, N., Cloutier, S., and Belleville, S. (2018). The Virtual Shop: A New Immersive Virtual Reality Environment and Scenario for the Assessment of Everyday Memory. J. Neurosci. Methods 303, 126–135. doi:10.1016/j.jneumeth.2018.03.010
Proctor, E., Silmere, H., Raghavan, R., Hovmand, P., Aarons, G., Bunger, A., et al. (2011). Outcomes for Implementation Research: Conceptual Distinctions, Measurement Challenges, and Research Agenda. Adm. Pol. Ment Health 38 (2), 65–76. doi:10.1007/s10488-010-0319-7
Robert, P. H., Berr, C., Volteau, M., Bertogliati, C., Benoit, M., Mahieux, F., et al. (2006). Neuropsychological Performance in Mild Cognitive Impairment with and without Apathy. Dement Geriatr. Cogn. Disord. 21 (3), 192–197. doi:10.1159/000090766
Robert, P., Lanctôt, K. L., Agüera-Ortiz, L., Aalten, P., Bremond, F., Defrancesco, M., et al. (2018). Is it Time to Revise the Diagnostic Criteria for Apathy in Brain Disorders? the 2018 International Consensus Group. Eur. Psychiatr. 54, 71–76. doi:10.1016/j.eurpsy.2018.07.008
Saba, M., and Blanchet, S. (2020). Working Memory Training in normal and Pathological Aging: Neurocognitive Gains and Generalization. Geriatrie Psychol. neuropsychiatrie du vieillissement 18 (2), 187–195. doi:10.1684/pnv.2020.0860
Sevinc, V., and Berkman, M. I. (2020). Psychometric Evaluation of Simulator Sickness Questionnaire and its Variants as a Measure of Cybersickness in Consumer Virtual Environments. Appl. Ergon. 82, 102958. doi:10.1016/j.apergo.2019.102958
Sherman, C., Liu, C. S., Herrmann, N., and Lanctôt, K. L. (2018). Prevalence, Neurobiology, and Treatments for Apathy in Prodromal Dementia. Int. Psychogeriatr. 30 (2), 177–184. doi:10.1017/s1041610217000527
Su, J., Duan, D., Zhang, X., Lei, H., Wang, C., Guo, H., et al. (2017). The Effect of Negative Emotion on Multiple Object Tracking Task: An ERP Study. Neurosci. Lett. 641, 15–20. doi:10.1016/j.neulet.2017.01.038
Valdez, P., and Mehrabian, A. (1994). Effects of Color on Emotions. J. Exp. Psychol. Gen. 123 (4), 394–409. doi:10.1037/0096-3445.123.4.394
Verbruggen, F., and De Houwer, J. (2007). Do emotional Stimuli Interfere with Response Inhibition? Evidence from the Stop Signal Paradigm. Cogn. Emot. 21 (2), 391–403. doi:10.1080/02699930600625081
Watson, D., Clark, L. A., and Tellegen, A. (1988). Development and Validation of Brief Measures of Positive and Negative Affect: the PANAS Scales. J. Personal. Soc. Psychol. 54 (6), 1063–1070. doi:10.1037/0022-3514.54.6.1063
Keywords: cognition, emotions, subjective cognitive decline, cybersickness, user experience, virtual reality
Citation: Cuesta M, Verty LV, Ben Abdessalem H, Byrns A, Bruneau M-A, Frasson C and Belleville S (2022) Virtual Reality and EEG-Based Intelligent Agent in Older Adults With Subjective Cognitive Decline: A Feasibility Study for Effects on Emotion and Cognition. Front. Virtual Real. 2:807991. doi: 10.3389/frvir.2021.807991
Received: 02 November 2021; Accepted: 09 December 2021;
Published: 19 January 2022.
Edited by:
Ali Oker, Université de Reims Champagne-Ardenne, FranceReviewed by:
Ryan Hampton, University of Wisconsin-Madison, United StatesJoan Llobera, University of Barcelona, Spain
Copyright © 2022 Cuesta, Verty, Ben Abdessalem, Byrns, Bruneau, Frasson and Belleville. This is an open-access article distributed under the terms of the Creative Commons Attribution License (CC BY). The use, distribution or reproduction in other forums is permitted, provided the original author(s) and the copyright owner(s) are credited and that the original publication in this journal is cited, in accordance with accepted academic practice. No use, distribution or reproduction is permitted which does not comply with these terms.
*Correspondence: Sylvie Belleville, sylvie.belleville@umontreal.ca