- 1Department of Southern Area Crop Science, National Institute of Crop Science, RDA, Miryang, Republic of Korea
- 2National Crops Resources Research Institute (NaCRRI), National Agricultural Research Organisation (NARO), Entebbe, Uganda
This study employed a joint strategy high-density SNP Chip DNA markers and multiple Genome Association and Prediction Integrated Tool (GAPIT) models [(Bayesian-information and Linkage-disequilibrium Iteratively Nested Keyway (BLINK), Fixed and random model Circulating Probability Uniform (FarmCPU), General Linear Model (GLM), and Settlement of Mixed Linear Model (MLM) Under Progressively Exclusive Relationship (SUPER)], to investigate novel genetic factors controlling mesocotyl elongation and seedling emergence for direct-seeded rice. Genotype data (230,526 SNP Chip DNA makers) of 117 doubled haploid lines (derived from a cross between 93–11 (Oryza sativa L. ssp. indica) and Milyang352 (O. sativa L. ssp. japonica) were used to perform a Genome-Wide Association Study (GWAS). Results revealed the association between five (5) topmost significant SNP markers, of which number two [AX-155741269, Chr2: 15422406 bp, and AX-155200917, Chr7: 23814085 bp, explaining 37.5% and 13.8% of the phenotypic variance explained (PVE)] are linked to the mesocotyl elongation loci, while three (AX-282097034 and AX-283652873, Chr9: 9882817 bp and 1023383 bp, PVE 64.5%, and 20.2%, respectively, and AX-154356231, Chr1: 17413989 bp, PVE 21.1%) are tightly linked to the loci controlling seedling emergence. The qMEL2-1 and qSEM9-1 are identified as major QTLs explaining 37.5% and 64.5% of the PVE for mesocotyl elongation and seedling emergence, respectively. The AX-282097034 (Chr9: 9882817 bp) was co-detected by four GAPIT models (BLINK, FarmCPU, SUPER, and GLM), while AX-155741269 was co-detected by BLINK and SUPER. Furthermore, a high estimated heritability (Mesocotyl elongation: h2 = 0.955; seedling emergence: h2 = 0.863; shoot length: h2 = 0.707) was observed. Genes harbored by qMEL2-1 and qSEM9-1 have interesting annotated molecular functions that could be investigated through functional studies to uncover their roles during mesocotyl elongation and seedling emergence events in rice. Furthermore, the presence of genes encoding transcription factors, growth- and stress response, or signaling-related genes would suggest that mesocotyl elongation and seedling emergence from deep direct-seeded rice might involve an active signaling cascade and transport of molecules, which could be elucidated through functional analysis. Likewise, genomic selection analysis suggested markers useful for downstream marker-assisted selection (MAS).
Introduction
The successful establishment of plants in the field largely depends on the ability of dry seeds to germinate, emerge, and fully differentiate. Failure in the emergence of seedlings may lead to crop failure and yield loss. Direct seeding is a common agricultural practice in many upland and lowland rice cultivation systems and helps save water and reduces labor costs (Kumar and Ladha, 2011; Jang et al., 2021). This agricultural practice is categorized as wet and dry direct seeding. Wet direct seeding refers to pre-germinated seeds being sown on wet or puddled soil. In contrast, dry direct seeding denotes the condition where seeds are drilled on unpuddled soil after dry tillage (Kumar and Ladha, 2011). In general, wet direct seeding is practiced in lowland rice cultivation environments, while dry direct seeding is commonly suitable for upland rice cultivation systems. Nevertheless, due to the variable seeding depths, and in some cases sub-optional seedbed conditions, direct seeding is often prone to poor seedling emergence and establishment. Consequently, a reduction in the number of individuals in the field is observed, leading to reduced yield and production (Alibu et al., 2012; Romaneckas et al., 2022). Seeds are sown at 5–10 cm depth, and their emergence after germination could be affected by factors such as soil texture and moisture, seed vigor or genetic makeup, and physiological and biochemical properties of seeds. Advanced statistical tools applied in plant breeding and genome sequencing offer new possibilities to gain new insights into the genetic control of agronomically important traits at the whole genome level.
In addition, poor leveling and uneven distribution of water in paddy fields lead to deep spots that affect the ability of wet-seeded rice to germinate and elongate due to hypoxia; therefore resulting in poor emergence and establishment (Hossain & Teixeira da Silva, 2013). Ideally, wet-seed lowland rice should be sown at a seeding depth of approximately 2.5 cm under wet soil. In upland rice, however, 2‒4 cm is recommended. The germination and emergence of upland rice sown at 2‒4 cm depth is generally affected by short dry spells that occur shortly after sowing and dry out the topsoil on which the seed is placed. Therefore, deep seeding may be necessary for direct dry-seeded upland as it can benefit from residual soil moisture in the deeper layers of soil, improving germination and emergence (Romaneckas et al., 2022). For that reason, rice accessions recommended for dry-direct seeding are required to have the ability to withstand deep sowing or tolerate variable seeding depths associated with machine sowing (Zhao et al., 2018).
The mesocotyl is a crucial agronomic trait for direct seeding because it can enhance seedling emergence and establishment from deep sowing (Lu et al., 2007; Zhan et al., 2020; Menard et al., 2021), and rice genotypes that rapidly elongate their mesocotyls are desirable for dry direct seeding (Alibu et al., 2012). The mesocotyl is a tubular, white stem-like tissue connecting the seed and the base of the coleoptile. Technically, the mesocotyl is the first internode of the stem. After germination, elongation of the mesocotyl elevates the coleoptile and its enclosed inner leaves towards the soil surface, thereby enhancing seedling emergence from deep sowing (Zhan et al., 2020; Menard et al., 2021). As the coleoptile nears the soil surface, exposure to light triggers dynamic endogenous changes in the phytohormone levels, which immediately halts mesocotyl elongation (Feng et al., 2017). Thus, rice cultivars that rapidly elongate their mesocotyls are desirable for direct seeding.
Mesocotyl elongation is a genetically determined trait that is measurable and controlled by the cumulative effect of many genes, influenced by environmental factors such as light, temperature, and water, and is responsive to sowing depth, soil water content, and salinity. Furthermore, plant hormones such as abscisic acid (ABA), Brassinosteroids (BR), strigolactone (SLs), cytokinin (CK), ethylene (ETH), jasmonic acid (JA), gibberellin (GA), and indole-3-acetic acid (IAA) play important roles in regulating mesocotyl elongation (Zhan et al., 2020). Mesocotyl elongation varies among cultivars of different rice groups. For example, indica rice accessions have been shown to exhibit longer mesocotyls when compared to the Japonicas (Uzair et al., 2022). Between indica and japonica rice, indica exhibited the longest mesocotyls with the highest length variation (Menard et al., 2021). In general, indica rice varieties exhibit longer mesocotyls with a larger variation than japonica rice.
In a study conducted by Zhao et al. (2018), 46.7% of japonica accessions showed shorter mesocotyls (0–1 cm) than their indica counterparts (30.2 %). However, more indica accessions had long mesocotyls of 4 cm or longer than in japonica. Likewise, Ming-guo et al. (2005) observed that an upland rice variety showed longer mesocotyls than lowland rice. Similarly, rice accessions originating from South and Southwest Asia assessed for their mesocotyl elongation patterns showed a larger variation than those originating from East Asia (Takahashi, 1978). This large natural genetic variation in mesocotyl elongation provides a basis for developing long mesocotyl cultivars (Zhan et al., 2020) and identifying genes by linkage mapping and/or Genome-Wide Association Study (GWAS) (Zhan et al., 2020). A large number of QTLs controlling rice mesocotyl elongation have been identified using different genetic populations, including recombinant inbred lines (RILs), chromosome segment substitution lines (CSSLs), backcross recombinant inbred lines (BILs) and doubled haploid (DH) populations (Zhan et al., 2020).
The advent of genome sequencing and advances in molecular breeding techniques, coupled with the emergence and application of bioinformatics (for gene discovery, GWAS, or genomic selection (GS) among others) have boosted the efficiency of plant breeding and variety development in a relatively short period. The Genome Association and Prediction Integrated Tool (GAPIT) package in R software is widely used in several genome-wide association studies and has successfully generated an abundance of data useful in plant breeding. Since it first launch of the first version in 2012, two more versions of the GAPIT package have been released, and the latest version 3 with multiple statistical models boosted the power and accuracy of GS and prediction (Wang et al., 2021). At the same time, these technologies and tools have offered tremendous opportunities for scientists to uncover novel genetic factors associated with desired and important traits in plant crops. The Single Nucleotide Polymorphism (SNP) marker system gained momentum in plant bioscience (Mammadov et al., 2012). Likewise, high-throughput SNP genotyping and DNA chip technology have emerged as powerful genomic tools in plant bioscience-related research to accelerate crops improvement (Thomson and Biotechnology, 2014; You et al., 2018; Yu et al., 2021; Carrier et al., 2022). Recently, with the rapid development of molecular marker technology, several quantitative trait loci (QTLs) for mesocotyl elongation were identified using bi-parental linkage mapping in rice (Lu et al., 2007). More than 40 mesocotyl elongation QTLs across almost all 12 chromosomes have been reported in rice using various segregating lines. Identification of QTLs associated with mesocotyl length could accelerate the genetic improvement of rice for wet and dry direct seeding.
This study aims to investigate novel QTLs controlling mesocotyl elongation and seedling emergence of direct-seeded rice through a GWAS with high-throughput SNP Chip DNA markers, using multiple GAPIT models with enhanced statistical power. The study also, capitalized on the multiple GAPIT to conduct a GS for the traits of interest.
Materials and methods
Plant materials and growth conditions
A hundred seventeen (117) Doubled haploid (DH) lines developed through anther culture of the cross between 93–11 (Oryza sativa L. ssp. indica) and Milyang352 [O. sativa L. ssp. japonica (Lee et al., 2020)]. Milyang352 is a Korean cultivar earlier developed from a cross between a Chinese rice cultivar C18 and Ungwang (Korean cultivar). Initially, seeds of the parents and DH lines were soaked for 3 days to induce germination. Thereafter, 20 seeds per line were sown on about a 2-cm soil bed and covered with a 6-cm soil layer (depth) in a seed box with compartments. The phenotype parameters {mesocotyl length, seedling emergence percentage [(number of emerged seedlings/total number of seeds sown) ×100], and shoot length} were recorded 14 days after sowing.
Frequency distribution, quantile‒quantile plots, kinship matrix, and correlation analysis
The frequency distribution and the correlation between traits were assessed using GraphPad Prism 7.0. The heat map was generated after installing [install.packages (“package name”)] and executing the library [library (“package name”)] of R packages ggplot2, tidyverse, cluster, factoextra, and executing the following R scripts:
[Script 1]: gr <- colorRampPalette [c (“green,” “red”)]
[Script 2]: heatmap.2 [y,col = gr, main = “Input main title here,” trace = “none,” margins = c (10,12), cexRow = 0.5, cexCol = 0.9, scale = “column,” srtCol = 0, adjCol = c (1,1), tracecol = NA, reorderfun = function (d, w) reorder (d, w, agglo. FUN = mean)].
The Quantile‒Quantile (Q‒Q) plots and the pairwise kinship matrix were generated after installing and executing the GAPIT function for GWAS. Meanwhile, the principal component analysis was done using the R package ggplot2 [install.packages (“ggplot2″)] as follows:
install.packages (“ggplot2”)
library (ggplot2)
Dataset <- read. csv (“C://data file location pathway. csv,” sep = “,”)
data <- data. matrix (Dataset)
head (data)
myPr <-prcomp [data (,−1), scale = TRUE]
myPr
summary (myPr)
plot (myPr, type = “l”) #Generate a scree plot
biplot (myPr, scale = 0) #Generate a PCA plot
Genomic selection or prediction analysis
To assess the genetic merit of the DH lines for target traits, we conducted a genomic prediction analysis as described earlier (Zhang et al., 2007). For GS analysis, we used the mixed linear model (MLM) (Yu et al., 2006), also known as genomic best linear unbiased prediction (gBLUP), which has a higher prediction accuracy for traits controlled by a large number of genes.
The genotype data was converted from the Haplotype Map (HapMap) format to numerical (see R script below) prior to performing the analysis. Together with the phenotype data, genotyping data were used to conduct a GP in order to assess the genomic estimated breeding value (GEBV) of the DH population. The GS analysis was done using the gBLUP method to estimate the genomic breeding values of the mapping population.
To convert HapMap to numerical format:
myG <-fread (“file:///D:genotype data location. txt,” head = FALSE)
myGAPIT <- GAPIT (G = myG, output. numerical = TRUE)
myGD = myGAPIT$GD
myGM = myGAPIT$GM
To conduct a genomic prediction:
myY < -read.csv (“phenotype file location pathway. csv,” sep = “,”)
myGD = read.csv (“numerical genotype file location pathway. csv,” sep = “,”)
myGM = read.csv (“markers file location pathway. csv,” sep = “,”)
set.seed (99,163)
GAPIT.Validation [
Y = myY (,1:2),
model = c (“gBLUP”),
GD = myGD, GM = myGM,
PCA.total = 3,
file.output = T,
nfold = 5]
The genomic prediction of the inference groups, which is based on the ties with corresponding groups in the reference panel, is derived from Henderson’s formula as follows:
uI = KIRKRR–1uR, where KRR is the variance-covariance matrix for all groups in the reference panel, KRI is the covariance matrix between the groups in the reference and inference panels, KIR is the covariance matrix between the groups inference and reference panels, uR is the predicted genomic values of the individuals in the inference group. To assess the reliability of the genomic prediction, the following formula is used:
Reliability= 1–PEV/σ2a, where PEV is the prediction error variance, representing the diagonal element in the inverse left-hand side of the mixed model equation, and σ2a is the genetic variance.
Genome-wide association study
To investigate genetic factors associated with the control of mesocotyl elongation and seedling emergence in rice, 230,526 markers were used. A GWAS was run by GAPIT (Genome Association and Prediction Integrated Tool) version 3 (Wang et al., 2021) with multiple models. The GAPIT models included the Bayesian-information and Linkage-disequilibrium Iteratively Nested Keyway (BLINK) (Zhang et al., 2018), Fixed and random model Circulating Probability Uniform (FarmCPU) (Liu et al., 2016), General Linear Model (GLM) (Price et al., 2006), Mixed Linear Model (MLM), and Settlement of MLM Under Progressively Exclusive Relationship (SUPER) (Wang et al., 2014), were employed to perform the genomic association analysis in R programming software. R scripts proposed by:
To perform a GWAS analysis:
my_GAPIT <- GAPIT [Y = myY, G = myG, model = c (“SUPER,” “FarmCPU,” “BLINK”), PCA. total = 3, SNP. MAF = 0.05, Multiple_analysis = TRUE].
In silico analysis and gene ontology search
GWAS analysis provided useful information on major QTLs for mesocotyl elongation and seedling emergence in rice. Based on physical positions of associated significant SNP Chip markers, we unraveled the identity of genes found within the target genetic loci to gain more insights. To achieve that, we conducted a search using the browser of the Rice Genome Annotation Project database (http://rice.uga.edu/cgi-bin/gbrowse/rice/#search, accessed on 27 July 2023) and PlantPAN 3.0 (http://plantpan.itps.ncku.edu.tw/plantpan3/search.php?#results, accessed on 27 July 2023) for each specific gene locus ID. Genes encoding similar domain-containing proteins were searched in the literature (https://funricegenes.github.io/geneKeyword.table.txt, accessed on 27 July 2023).
To assess the similarity of target genes in qSEM9 and qMEL2 between japonica and indica groups, the coding sequences (CDS) of putative candidate genes, obtained from the Nipponbare rice database (http://rice.uga.edu/#search, accessed on 13 October 2023) and indica rice database (https://plants.ensembl.org/Oryza_indica/Info/Index, accessed on 13 October 2023), were aligned using the ClustalW algorithm in BioEdit software (BioEdit Sequence Alignment Editor, Copyright ©1997–2013 Tom Hall) (Hall, 1999).
Total RNA extraction, cDNA synthesis, and qPCR analysis
Total RNA was extracted from leaf, roots, and mesocotyl of two-week-old seedlings, following the manufacturer’s instructions, using the RNeasy Plant Mini Kit (Qiagen, Netherlands). In essence, approximately 50–100 mg of frozen samples in liquid nitrogen were ground to a fine powder in 2 mL Eppendorf tubes (e-tubes) using beads by vigorous vortex. Then 450 µL buffer RLT (lysis buffer containing β-mercaptoethanol) was added, and the tubes were vigorously vortexed. The lysate was transferred to a QIAshredder spin column (lilac) placed in a 2 mL collection tube, followed by centrifugation for 2 min at 12,000 rpm. The supernatant of the flow-through was transferred to a new microcentrifuge tube (1.5 mL). The clear lysate was mixed with 0.5 volume of ethanol by pipetting up and down for a few seconds, and about 650 µL of the sample mixture was transferred to an RNeasy Mini spin column (with a membrane) with a 2 mL collection tube. The tubes were centrifuged for 15 s at 10,000 rpm. The flow-through was discarded and 500 µL of Buffer RPE was added to the RNeasy spin column, followed by centrifugation at 10,000 rpm for 2 min. To elute the RNA, 50 µL RNase-free water was added to the membrane of the RNeasy spin column, and the tubes were centrifuged for 1 min at 10,000 rpm. The concentration RNA (ng µL–1) was measured using a NanoDrop2000 Spectrophotometer (Thermo Fisher Scientific, USA), and samples were kept in −20°C fridge for downstream analysis.
For complementary DNA (cDNA) synthesis, the ProtoScript II First cDNA Synthesis Kit (New England BioLabs Inc., USA) was employed. Briefly, 1 µg RNA samples was preheated with 1 µL Oligo dT at 65°C for 5 min. Thereafter, 2 µL ProtoScript II Enzyme Mix (10X) was added, and the reaction mixture was adjusted to 20 µL reaction volume with RNase-free water. The cDNA synthesis reaction was incubated at 42°C for 1 h in a Reverse transcriptase PCR using a Thermocycler.
To validate the expression of genes found within qSEM9 and qMEL2, qPCR analysis was performed using the amfiSure qGreen Q-PCR Kit (GenDEPOT Corporation ©2017, USA) in a (QuantStudioTM real-time PCR systems, Thermo Fisher Scientific, USA). qPCR machine. In essence, a three-step reaction consisting of an initial enzyme activation at 95°C for 3 min, followed by 40 cycles of denaturation at 95°C for 10 s, annealing 60°C for 20 s, and extension at 72°C for 30 s.
Results
Differential mesocotyl length and seedling emergence between parental lines
We observed differential mesocotyl elongation and shoot emergence patterns between parental lines 14 days after sowing. Results indicate that the indica parent (P1, 93–11) developed longer mesocotyl (7.9 cm) and taller seedlings (43.4 cm) (compared to its japonica counterpart (P2, Milyang352; mesocotyl length: 2 cm, shoot length: 18 cm) (Figures 1A, F). Likewise, the emergence percentage (here expressing the number of seedlings that emerged at the last count) was much higher in 93–11 (on average 50%) than that recorded in Milyang352 (on average 5%) (Figures 1A, B, D). The poor emergence of Milyang352 resulted in poor growth and shoot elongation (Figures 1A, B, H). Under the same conditions, about 74.4% of the mapping population (117 doubled haploid lines) showed a mesocotyl elongation pattern similar to that of Milyang352, while 25.6% resembled the mesocotyl elongation of 93–11-like. Likewise, nearly 84.6% of the DH lines had a Milyang352-like emergence percentage against 15.4% with a 93–11-like emergence pattern (Figures 1A, B, D, F, H). In addition, 67.5% of the DH lines recorded relatively taller seedlings, while 32.5% had shorter seedlings similar to 93–11 or Milyang352, respectively. Furthermore, panels Figures 1C, E indicate a positive (right) skewness distribution for seedling emergence and mesocotyl elongation, while Figure 1G shows that DH lines exhibited a normal distribution for shoot length. Furthermore, the quantile-quantile (Q–Q) plots show the distribution of data to the theoretical values for the traits of interest (Figures 1I–K).
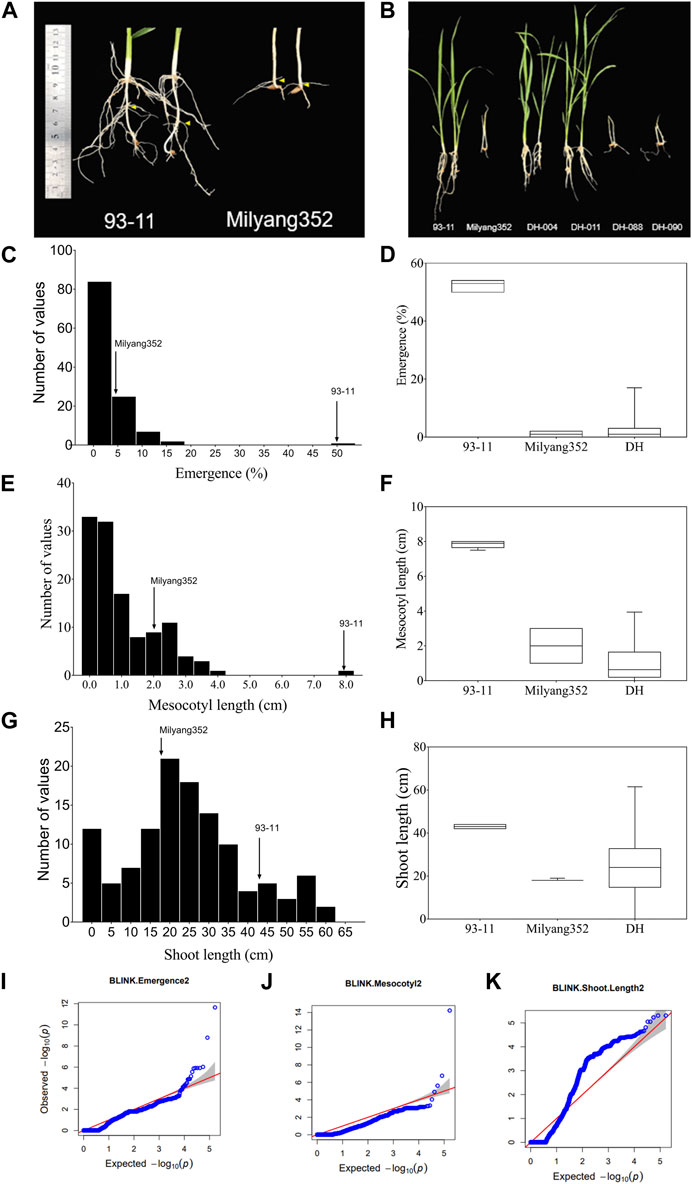
FIGURE 1. Frequency distribution of traits and quantile–quantile plots. (A,B) Mesocotyl length and shoot emergence pattern of parental lines (93–11 and Milyang352) and a few DH lines, (C–H) Frequency distribution and box plots for seedling emergence, mesocotyl length, and shoot length of 117 rice doubled haploid lines. (I–K) Quantile–Quantile (Q–Q) plots for seedling emergence, mesocotyl length, and shoot length. –log10(p) is the negative logarithm base 10 quantile–quantile (Q–Q) of the p-values (expected and observed) for traits.
Cluster analysis, principal component analysis, and correlation between traits
Figures 2A, B show that DH lines were grouped into two distinct clusters based on their mesocotyl elongation pattern (93–11 or Milyang352-type). From another perspective, the data in panel Figure 2C (heat map) groups seedling emergence and shoot length in the same cluster, while mesocotyl elongation is assigned to a different cluster (column dendrogram on top). Panel Figure 2C also allows visualizing the respective phenotypic pattern of all DH lines (lines with a high phenotypic values are shown by the red color map, while those with low phenotypic values are indicated in green). In addition, the principal component analysis (PCA) proposes that PC1, PC2, and PC3 explained 75.1% and 80% of the proportion of variance for seedling emergence and mesocotyl elongation, respectively (Figure 2D). We were also interested to see the relationship between traits; we assessed the correlation of all traits. Data in Figure 2E indicate an existing strong positive correlation between seedling emergence and shoot elongation (R2 = 0.386***). Similarly, mesocotyl elongation and seedling emergence exhibited a positive relationship (R2 = 0.246***) (Figure 2F). In contrast, no correlation was found between mesocotyl elongation and shoot length (R2 = 0.067) (Figure 2G).
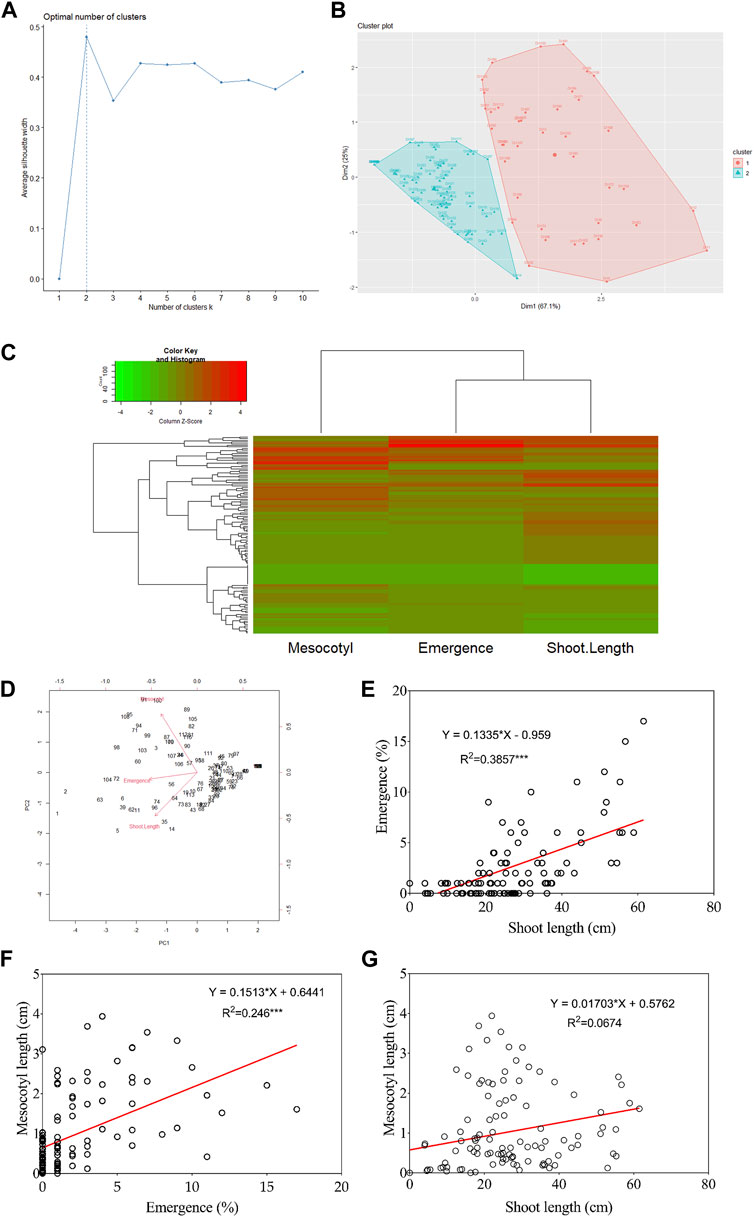
FIGURE 2. Cluster, principal component analysis, and correlation results. (A) Scree plot defining the number of clusters of the population, (B) Population clustered into two clusters, (C) Heat map with groups of DH lines based on phenotypes, (D) Principal Component Analysis results, and (E–G) Correlation analysis results between traits.
Co-ancestry, marker density, heritability, and genome prediction
We constructed a kinship matrix to assess the population’s relatedness. Results in Figure 3A show the SNP Chip DNA marker-based kinship, also referred to as the co-ancestry or half relatedness. This data reveals the distribution of coefficients of co-ancestry of DH lines, confirming their genetic variability. In addition, Figure 3B displays the density map of used SNP Chip markers on all 12 chromosomes of rice. The phenotypic variance explained (PVE %) of significant SNP Chip, the logarithm of the odds (LOD) values, expressed as–log10(p), and the estimated effects are shown in panels Figures 3C–E.
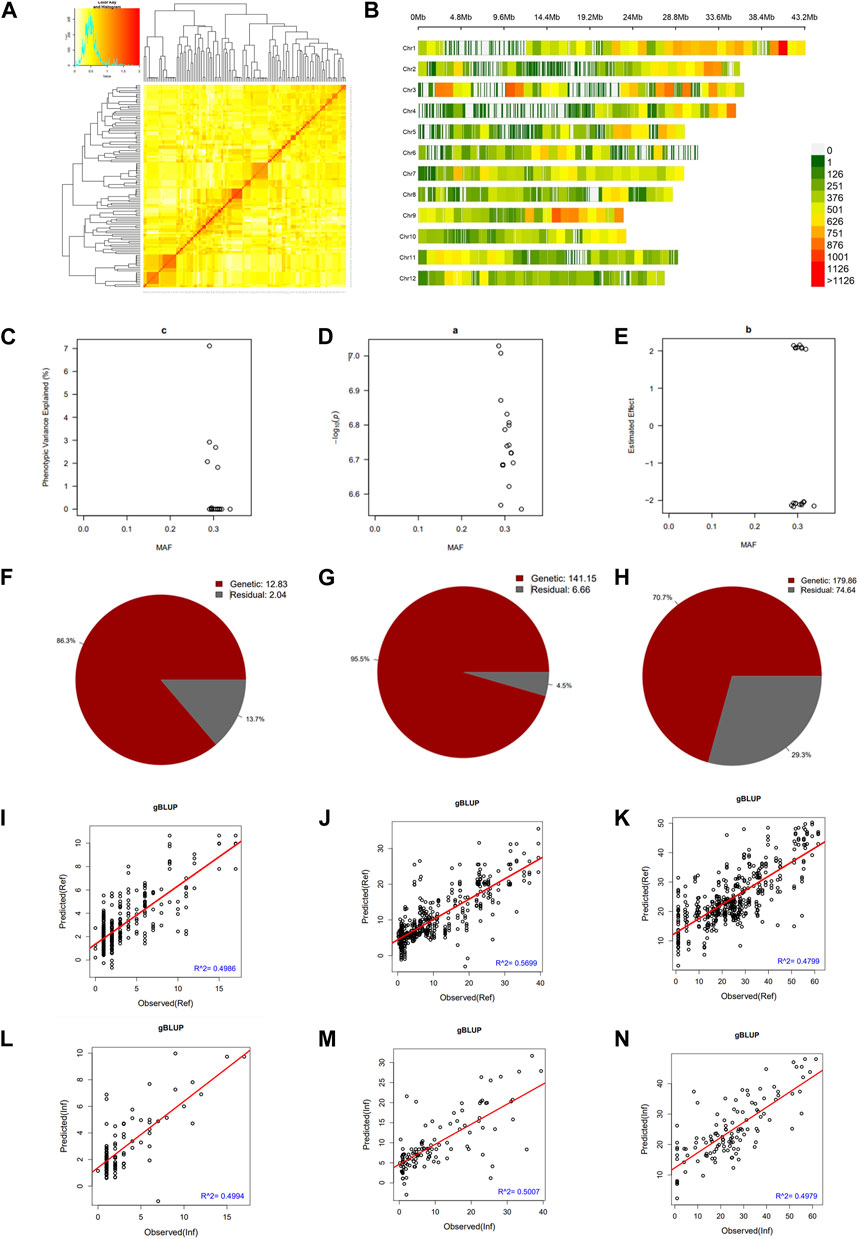
FIGURE 3. Pairwise kinship matrix, marker density map, genomic selection, and heritability results. (A) Pairwise kinship matrix showing the relatedness or co-ancestry level, (B) Density map of the markers per chromosome, (C) Phenotypic variance explained (PVE), (D) logarithm of the odds [LOD, –log10(p)] values, (E) estimated effect of the significant markers, (F–H) narrow sense heritability (h2) for mesocotyl elongation, seedling emergence, and seedling height, respectively, (I–K) predicted versus observed genomic estimated breeding value in the reference group and (L–N) in the inference group.
We estimated the heritability of target traits to understand the proportion of variation explained by the individuals’ breeding values for each trait. Results in panels Figures 3F–H reveal that mesocotyl elongation had a heritability (h2) of 0.863, while seedling emergence and shoot length recorded an h2 of 0.955 and 0.707, respectively. To further gain insights, we used the GAPIT models to conduct a GS based on MLM (gBLUP), which is known to have a higher prediction accuracy to assess the genomic estimated breeding value (GEBV) of individuals for traits controlled by a large number of genes. Therefore, using this method, we investigated the genetic merit of DH lines for the target traits. Results in Figures 3I–N show the predicted and observed GEBV of individuals in the mapping population for mesocotyl elongation and seedling emergence, in the reference group (Ref) (Figures 3I–K) and the Inference group (Inf) (Figure 3L–N).
Detected genetic loci controlling mesocotyl elongation and seedling emergence by GWAS
Parental recorded differential phenotypes for the selected traits (mesocotyl elongation, seedling emergence, and shoot length), which was reflected in their derived population. In this regard, we performed GWAS with 230,526 SNP Chip DNA markers selected from initial 538,983 markers (after removing those with a high missing rate and heterozygosity above 5%). To explore the statistical powers of proposed GAPIT models to detect significant SNP markers through GWAS, we employed multiple GAPIT models (BLINK, FarmCPU, GLM, and SUPER) to investigate novel genetic loci associated with the control of the traits of interest. In panels Figures 4A–S, we can see the effect of SNP Chip markers on the distribution of phenotypes, grouped by GAPIT models.
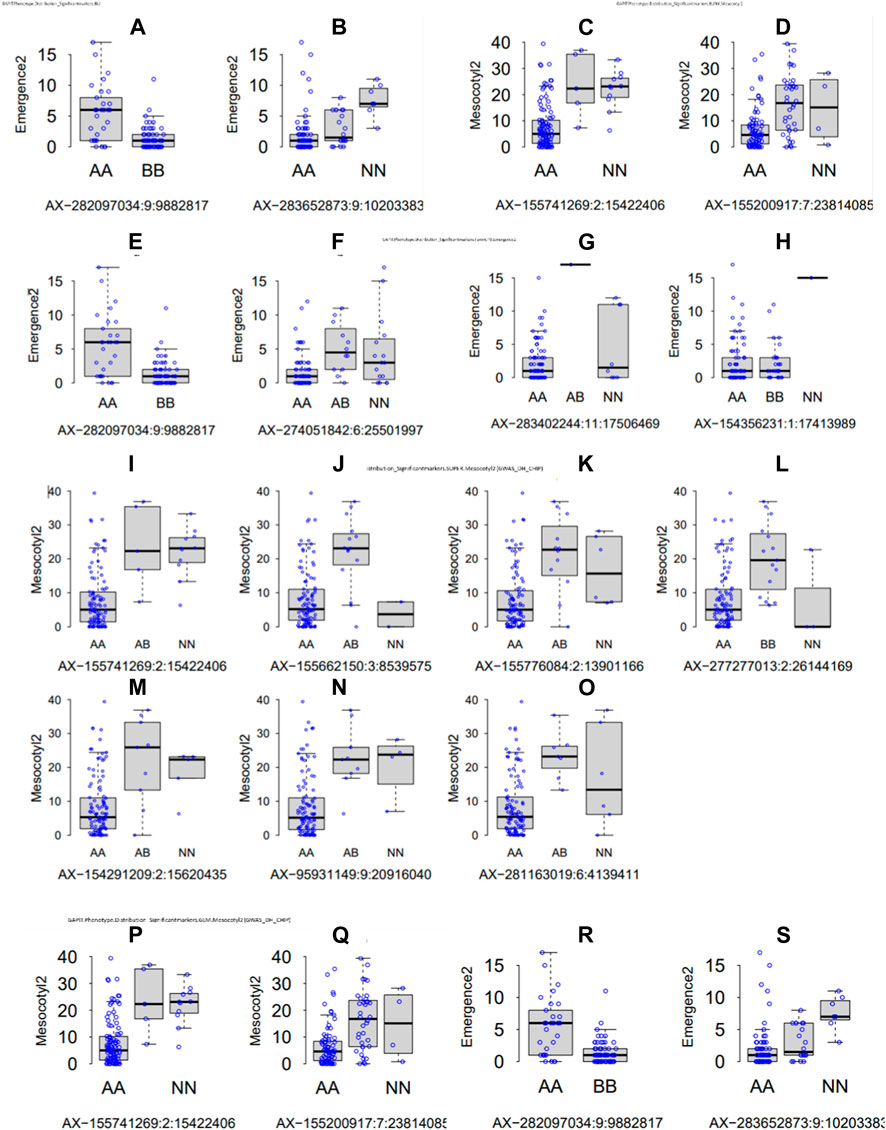
FIGURE 4. Significant SNP Chip marker-based phenotypic distribution. GAPIT phenotypic distribution based on significant SNP Chip DNA markers for each GAPIT model BLINK (A–H) FarmCPU, (I–O) SUPER, and (P–S) GLM.
GWAS results reveal that 13 significant SNP Chip makers are linked to genetic loci controlling mesocotyl elongation or seedling emergence in rice, and are located on 7 chromosomes (Chr) of rice (Figures 5A–H). Eight SNP Chip markers were linked to mesocotyl elongation, found on Chr2, 3, 6, 7, and 9, while those associated with seedling emergence were located on Chr1, 6, 9, and 11 (Table 1). As shown in Table 1, the significant SNP Chip DNA marker AX-282097034 (9882817 bp), linked to the locus controlling seedling emergence on Chr9, was co-detected by several GAPIT models (BLINK, PVE 64.5%; FarmCPU, PVE 13%; SUPER, PVE 3.3%; GLM, PVE 2.1%). The additive effects, −1.41 for AX-282097034 and –3.58 for AX-283652873, indicate that the allele from Milyang352 (japonica parent) enhanced the trait value (Table 2). On the same Chr9, five other SNP Chip markers, including AX-283652873 (10203383 bp, PVE 20.2%; additive effect: Milyang352) detected via BLINK, AX-279109564 (9882978 bp, PVE 7.1%, additive effect: Milyang352), AX-155630054 (9922608 bp, PVE 2.9%, additive effect: 93–11), AX-283347022 (6332807 bp, PVE 2.7%, additive effect: 93–11) and AX-117372040 (9910913 bp, PVE 1.8%, additive effect: Milyang352) detected via GLM, were found. Five additional SNP Chip markers with small effects were detected on Chr1, 8, and 9 but their effects were specific to any alleles from either parental lines (Table 2). Likewise, via FarmCPU GAPIT model, other SNP Chip markers, such as AX-154356231 (Chr1:17413989 bp, PVE 21.1%; additive effect: 93–11), AX-274051842 (Chr6: 25501997 bp, PVE 4.2%, additive effect: 93–11), and AX-283402244 (Chr11: 17506469 bp, PVE 36.6%, additive effect: 93–11) were identified.
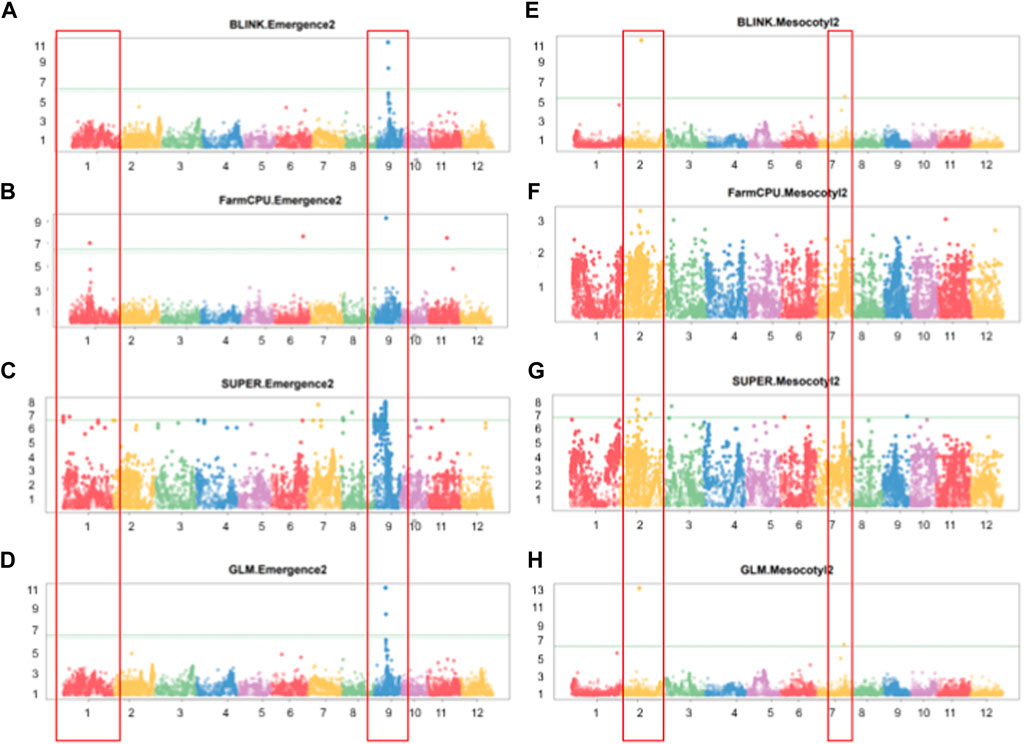
FIGURE 5. Manhattan plots showing GWAS results for multiple GAPIT models. (A–D) Manhattan plots showing significant SNP Chip DNA markers linked to seedling emergence, and (E–H) for mesocotyl elongation loci, based on BLINK, FarmCPU, SUPER, and GLM GAPIT models. The frame across the Manhattan plots highlights the detected genetic loci at specific chromosomes, above the genome-wide line.
Because mesocotyl elongation contributes to seedling emergence in rice, we were interested to see genetic loci associated with this trait of interest. As displayed in Tables 1, 2; Figure 5, two SNP Chip markers, AX-155741269 (Chr2: 15422406 bp) detected via BLINK (PVE 37.5%, additive effect: 93–11) and SUPER (PVE 6.3%, additive effect: not specific), and AX-155200917 (Chr7: 23814085 bp) detected via BLINK (PVE 13.8%, additive effect: 93–11) were identified.
Considering that AX-282097034 (Chr9: 9882817 bp) exhibited the highest PVE value (64.5%) for seedling emergence, in addition to being co-detected by four GAPIT models, followed by AX-283652873 (Chr9: 10203383 bp), we were interested to unravel the identity of genes found within the target loci controlling seedling emergence in rice. In the same way, the physical position of the SNP Chip marker AX-155741269 (Chr2: 15422406 bp), co-detected by BLINK and SUPER, and AX-154291209 (Chr2: 15620435 bp) were used to unveil genetic factors associated with the control of mesocotyl elongation in rice. Putative candidate genes (Table 3) for seedling emergence, found within qSEM9 QTL, were pooled from a region covering 320.6 kbp (with AX-282097034 and AX-283652873 as flanking markers, http://rice.uga.edu/cgi-bin/gbrowse/rice/#search (Chr9:9882817..10203383), accessed on 27 July 2023). Whereas, genes associated with qMEL2 QTL (Table 4) were pooled from a region (Chr2:15620435..15454000) covering 166.4 kbp.
We excluded genes with no specific functional conserved domain (hypothetical protein, retrotransposons, etc.). However, genes encoding transposon protein and other domain-containing proteins were included due to their emerging role in plant metabolism.
Organ-specific validation of qSEM9 and qMEL2-related genes by qPCR
We were interested to investigate the change in the transcript accumulation level of a set of genes found in qSEM9 and qMEL2 regions at basal level. These genes were selected based on the predicted differential coding sequences identified by aligning the japonica (Nipponbare rice database: http://rice.uga.edu/#search, accessed on 13 October 2023) (Supplementary Table S1) and indica rice database (https://plants.ensembl.org/Oryza_indica/Info/Index, accessed on 13 October 2023) (Supplementary Table S2). qPCR results show that when expressed in the leaf, genes associated with emergence (qSEM9) and mesocotyl elongation (qMEL2) exhibited a high transcript accumulation level in 93–11 (the indica parent with an enhanced emergence rate and longer mesocotyl); while, in Milyang352 (japonica parent with low emergence and shorter mesocotyl), the same set of genes maintained a low expression level (Figure 6A). In addition, panel Figure 6B indicates that, except Os09g16260 and Os09g16510 (qSEM9), and Os02g26560 and Os02g26400 (qMEL2) which exhibited an enhanced transcriptional level in 93–11 compared to Milyang352, Os09g16240 (qSEM9) expression level was lower in roots of 93–11 but enhanced in Milyang352. Under the same conditions, Os09g16700 (qSEM9) was barely expressed and showed no significant difference between both parents. Likewise, panel Figure 3C shows that the transcript accumulation level of all genes was higher in the mesocotyl of 93–11 than Milyang352, except Os02g26400 (qMEL2), which showed no significant different between the two parental cultivars.
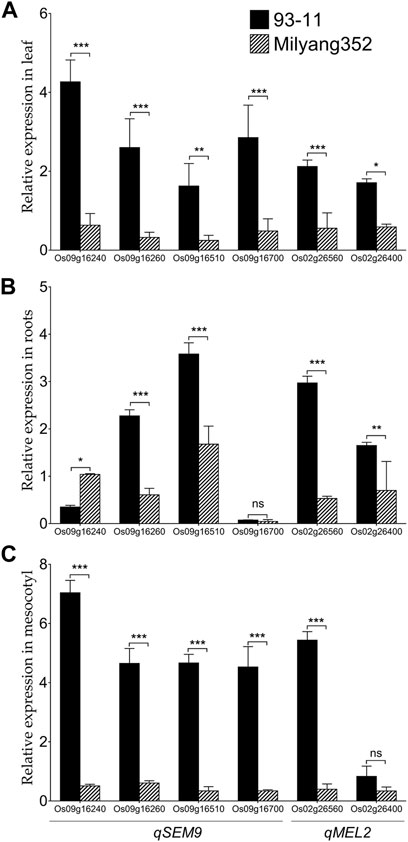
FIGURE 6. Transcriptional pattern of qSEM9 and qMEL2-related genes in different plant organs of rice. Relative expression of qSEM9 (Os09g16240, Os09g16260, Os09g16510, and Os09g16700) and qMEL2 (Os02g26560 and Os02g26400) in (A) the leaf, (B) roots, and (C) mesocotyl of parental lines 93–11 (indica) and Milyang352 (japonica). Black bars are average expression data in 93–11 (indica), while bars with hatches are average expression in Milyang352 (japonica) background. Error bars are mean values ± SE. ***p < 0.001, **p < 0.01, *p < 0.05, ns non-significant.
Discussion
Seedling emergence is closely related to mesocotyl elongation in rice but not shoot length
Mesocotyl elongation is controlled by a wide range of genetic factors, including a combinational action of plant growth regulators and hormonal signaling and biosynthetic pathway genes, working in a synergetic or antagonistic relationship (Feng et al., 2017). It is widely acknowledged that mesocotyl elongation is essential for seedling emergence in rice (Lee et al., 2017). A study conducted by Wang et al. (2023) found that phytohormones (SLs, CKs, ABA, BRs, IAA, and JA) have a direct influence on mesocotyl elongation by affecting cell division or elongation. Likewise, Liang et al. (2016) supported that an increase in endogenous gibberellin content, which in turn caused changes in cortical microtubule organization, promoted rice mesocotyl elongation. Furthermore, poor emergence of directed-seeded crops is commonly associated with a weak mesocotyl elongation, which may result in poor field occupation and low productivity. In the same way, lines of evidence established that mesocotyl and coleoptile are primarily responsible for the emergence of rice seedlings in deep-sowing and direct-seeding cultivation systems (Wu et al., 2015; Lee et al., 2017). In this regard, we could argue that the observed positive correlation between seedling emergence and mesocotyl elongation on the one hand, and seedling emergence and shoot length, on the other hand, would suggest that the emergence of rice seedlings out of soil from a deep sown cultivation system is likely to depend on the ability of seedlings to develop longer mesocotyls. In turn, a high seedling emergence percentage would be a transition to plant differentiation and the development of aboveground plant parts, which are essential for achieving high productivity and yield potential. In contrast, the non-existing correlation between mesocotyl elongation and seedling shoot length would imply that, after emergence, shoot length might not directly depend on the length of mesocotyl in rice.
Novel genetic loci for mesocotyl elongation in rice
Mesocotyl elongation and seedling emergence are important traits for the later expression of the yield potential and rice productivity per unit area, and their association has been demonstrated (Lee et al., 2017). Mesocotyl elongation and seedling emergence are determinant factors conditioning the number of individuals present in the field. Since the advent of genome sequencing technologies, several techniques have been used to identify QTLs for mesocotyl elongation in rice, including QTL-sequencing (QTL-seq), GWAS (Lu et al., 2016; Wang et al., 2021) or linkage or association mapping (Edzesi et al., 2023; Feng et al., 2023), and employing diverse mapping populations/germplasm and various DNA marker systems (Huang et al., 2022; Edzesi et al., 2023). Previous studies reported QTLs associated with mesocotyl elongation (qMEL) on almost all chromosomes of rice 1–5, 7, 9, 11, 12 (Lee et al., 2012; Lu et al., 2016). According to Wang et al. (2021), genes involved in mesocotyl elongation are also associated with phytohormone metabolic pathways, cell elongation, and cell division. In the present study, eight (8) QTLs for mesocotyl elongation were detected on Chr2 (qMEL2-1, qMEL2-2, qMEL2-3, qMEL2-4), 3 (qMEL3), 6 (qMEL6), 7 (qMEL7), and 9 (qMEL9), of which number qMEL2-1 recorded the highest LOD value and was detected by two GAPIT models (BLINK and SUPER). In contrast to previous reports, qMEL2 is a novel QTL associated with the control mesocotyl elongation in rice. The physical position of qMEL2 indicates no overlapping region with previously reported mesocotyl-related QTLs in rice (Redona et al., 1996; Cai et al., 2002; Lee et al., 2012; Edzesi et al., 2023). The qMEL2, a major mesocotyl QTL explaining nearly 37.5% of the observed phenotypic variance, harbors a set of genes proposed to have diverse molecular functions or be involved in biological processes such as transcription factor activity (Os02g26430, encoding a WRKY42 transcription factor, TF), growth and development (Os02g26490, no apical meristem (NAM) domain-containing protein, zinc-ribbon domain-containing protein (Os02g26560), a Ubiquitin carboxyl-terminal hydrolase (Os02g26550), a Serine carboxypeptidase homologue (OsSCP7, Os02g26480), and a gene encoding the Wiskott-Aldrich syndrome protein (WAS, Os02g26370: a conserved domain analysis revealed that this gene has no functional conserved domain. Therefore, it may not be a useful gene (see Supplementary Table S2). Because no conserved domains were detected in Os02g26600 and Os02g26370 (WAS), their putative functions would be hypothetical.
The role of WRKY TF in plant metabolism has been widely investigated (Finatto et al., 2018), of which we can mention the mediation of the crosstalk between plant growth (through the BR signaling) and abiotic stress (Chen et al., 2017). It was interesting to see that OsWKRY42 TF, found within the qMEL2-1 region was earlier reported to suppress OsMT1d and induce reactive oxygen species (Han et al., 2014), or mediate resistance to fungal pathogens (Cheng et al., 2015). In addition, Pillai et al. (2018) observed that plants overexpressing OsWRKY42 TF (described as a cell wall damage-induced TF) enhanced callose deposition (for cell membrane integrity) and anthocyanin content, leading to enhanced salt tolerance in rice, by acting as a negative regulator of the jasmonic acid.
Likewise, members of the NAC family proteins regulate NAM (no apical meristem), regulate drought stress response in rice (Jeong et al., 2010), salt stress in garlic (Wang et al., 2022), heat stress and grain filling in rice (Ren et al., 2021), floral organ identity and lateral organ separation in Medicago truncatula (Cheng et al., 2012), pattern formation in embryo and flowers in Petunia (Souer et al., 1996), among others. Mutation in the NAM domain resulted in changes in shoot apical meristem (SAM) development in rice (Prakash et al., 2022) or failure to develop SAM in Petunia embryos (Souer et al., 1996). In the same way, mutant plants lacking the NAM showed abnormal organ differentiation in rice embryos (Nagato et al., 1989).
Similarly, genes encoding the zinc ribbon domain of the general transcription factor TFIIB family play various roles in the transcription initiation events and are highly conserved in plants (Hahn et al., 2000). The TFIIB is essential for RNA Polymerase (Pol) II recruitment (Chen and Hahn, 2003), with which it physically interacts (Elsby and Roberts, 2008) to govern the basal transcription initiation machinery.
The qPCR validation of qSEM9 and qMEL2-related genes revealed differential transcript accumulation patterns of tested genes, except a few. The observed differences in the expression level of target genes between 93–11 (indica) and Milyang352 (japonica), in leaf, roots, and mesocotyl (see Figures 6A–C), would suggest their differential transcriptional regulation during seedling mesocotyl elongation and seedling emergence. Functional studies are required to elucidate their actual roles during mesocotyl elongation and seedling emergence in rice.
New genes associated with seedling emergence in rice are identified
As shown in the correlation analysis results, mesocotyl elongation and seedling emergence are closely related. However, as per to the results in Table 3, the novel QTL (qSEM9) associated with the control of seedling emergence in direct-seed rice is located on chromosome 9, while the qMEL2 was detected on chr2. Looking at genes found within the qSEM9, based on their annotated functions, they could be grouped into five groups. The first group includes genes associated with protein binding (Os09g16200 and Os09g16240, and Os09g1652 encoding Ankyrin repeat domain-containing protein and cytochrome b5-like Heme/steroid binding domain-containing protein, respectively). The second and third groups consist of genes proposed to be involved in carbohydrates metabolic process and transport activity [Os09g16260 encoding an ABC-2 type transporter (PDR20); Os09g16330, Os09g16380, Os09g16449, Os09g16458, and Os09g16520 encoding a pleiotropic drug resistance (ABC transporter-like domain), and legume lectins β-domain containing proteins (Os09g16650 and Os09g16700)]. In addition, the majority of genes harbored by the two QTLs are proposed to be involved in abiotic or biotic stress response mechanisms or signaling events (fourth and fifth groups).
Genomic estimated breeding value of individuals
The advent of molecular breeding techniques and the application of bioinformatics tools in plant breeding have paved new paths toward gene discovery and advancing generations for variety development in a relatively short time. In contrast to conventional breeding, where phenotypic expression of target traits was the major way to assess the genetic merit of individuals, GS or genomic prediction has proven essential to investigate the genetic merit of breeding lines with high accuracy to identify promising lines. This prediction is based on the gBLUP (Zhang et al., 2007) using the MLM approach proposed for GWAS (Zhang et al., 2010), which assesses the genetic potential for a group of individuals derived from the BLUP of group effects in the compressed mixed model and is used as a prediction for all individuals in the group (in the reference or inference cluster). This prediction lies on the assumption that all groups in the reference panel have at least one individual with phenotypic data, and all groups in the inference panel have no individuals with phenotypic data. Hence, the genomic prediction for groups in the inference panel is based on phenotypic ties with their corresponding groups in the reference panel derived from Henderson’s formula (Ferdosi et al., 2019).
Conclusion and perspectives
Direct-seeded rice is commonly used for several benefits, including cost-effectiveness and seed use efficiency. This study explored the possibility of detecting QTLs using multiple GAPIT models with enhanced statistical power and predicting genomic breeding value in target lines or populations for desired traits. Results identified two major QTLs for mesocotyl elongation and three for seedling emergence in rice. Significant SNP Chip DNA markers tightly linked to the mesocotyl elongation and seedling emergence loci were detected on Chr2 (qMEL2-1) and Chr9 (qSEM9-1), with 37.5% and 64.5% of PVE, respectively. The qSEM9-1 (AX-282097034, Chr9: 9882817 bp) was co-detected by four GAPIT models (BLINK, FarmCPU, SUPER, and GLM), while qMEL2-1 (AX-155741269, Chr2: 15422406 bp) was co-detected by BLINK and SUPER. Furthermore, a high estimated heritability (Mesocotyl elongation: h2 = 0.955; seedling emergence: h2 = 0.863; shoot length: h2 = 0.707) was observed. Genes harbored by qMEL2-1 and qSEM9-1 have interesting annotated molecular functions that could be elucidated through functional studies to uncover their roles in mesocotyl elongation and seedling emergence events in rice. Moreover, the presence of genes encoding transcription factors, signaling as well as transport-related genes would suggest that mesocotyl elongation and seedling emergence would involve an active transport of molecules like sugar (a source of energy) and signaling cascade, driven by an active transcriptional regulatory network. Therefore, investigating novel QTLs through GWAS with multiple GAPIT models and high-density SNP Chip DNA marker system could boost the power and accuracy of plant breeding through genome association and prediction, and generate useful data for genomic selection and marker-assisted selection (MAS).
Data availability statement
The original contributions presented in the study are included in the article/Supplementary Material, further inquiries can be directed to the corresponding authors.
Author contributions
NK: Conceptualization, Data curation, Formal Analysis, Investigation, Methodology, Software, Visualization, Writing–original draft. SA: Formal Analysis, Investigation, Methodology, Writing–original draft. YK: Formal Analysis, Investigation, Writing–original draft. S-ML: Formal Analysis, Investigation, Writing–original draft. K-WO: Funding acquisition, Project administration, Supervision, Validation, Writing–review and editing. J-HL: Funding acquisition, Project administration, Supervision, Validation, Visualization, Writing–review and editing.
Funding
The author(s) declare financial support was received for the research, authorship, and/or publication of this article. This work was supported by the Project No. PJ016030032023 of the Rural Development Administration, Republic of Korea.
Conflict of interest
The authors declare that the research was conducted in the absence of any commercial or financial relationships that could be construed as a potential conflict of interest.
Publisher’s note
All claims expressed in this article are solely those of the authors and do not necessarily represent those of their affiliated organizations, or those of the publisher, the editors and the reviewers. Any product that may be evaluated in this article, or claim that may be made by its manufacturer, is not guaranteed or endorsed by the publisher.
Supplementary material
The Supplementary Material for this article can be found online at: https://www.frontiersin.org/articles/10.3389/fgene.2023.1282620/full#supplementary-material
References
Alibu, S., Saito, Y., Shiwachi, H., and Irie, K. (2012). Genotypic variation in coleoptile or mesocotyl lengths of upland rice (Oryza sativa L.) and seedling emergence in deep sowing. Afr. J. Agric. Res. 7, 6239–6248. doi:10.5897/ajar12.022
Cai, H., Morishima, H. J. T., and Genetics, A. (2002). QTL clusters reflect character associations in wild and cultivated rice. Theor. Appl. Genet. 104, 1217–1228. doi:10.1007/s00122-001-0819-7
Carrier, A., Prunier, J., Poisson, W., Trottier-Lavoie, M., Gilbert, I., Cavedon, M., et al. (2022). Design and validation of a 63K genome-wide SNP-genotyping platform for caribou/reindeer (Rangifer tarandus). BMC Genomics 23 (1), 687. doi:10.1186/s12864-022-08899-6
Chen, H.-T., and Hahn, S. J. M. c. (2003). Binding of TFIIB to RNA polymerase II: mapping the binding site for the TFIIB zinc ribbon domain within the preinitiation complex. Mol. Cell 12 (2), 437–447. doi:10.1016/s1097-2765(03)00306-x
Chen, J., and Yin, Y. J. P. (2017). WRKY transcription factors are involved in brassinosteroid signaling and mediate the crosstalk between plant growth and drought tolerance. Plant Signal Behav. 12 (11), e1365212. doi:10.1080/15592324.2017.1365212
Cheng, H., Liu, H., Deng, Y., Xiao, J., Li, X., and Wang, S. J. P. P. (2015). The WRKY45-2 WRKY13 WRKY42 transcriptional regulatory cascade is required for rice resistance to fungal pathogen. Plant Physiol. 167 (3), 1087–1099. doi:10.1104/pp.114.256016
Cheng, X., Peng, J., Ma, J., Tang, Y., Chen, R., Mysore, K. S., et al. (2012). NO APICAL MERISTEM (MtNAM) regulates floral organ identity and lateral organ separation in Medicago truncatula. Medicago truncatula 195 (1), 71–84. doi:10.1111/j.1469-8137.2012.04147.x
Edzesi, W. M., Dang, X., Liu, E., Bandoh, W. K. N., Gakpetor, P. M., Ofori, D. A., et al. (2023). Screening germplasm and detecting QTLs for mesocotyl elongation trait in rice (Oryza sativa L.) by association mapping. BMC Genom Data 24 (1), 8–14. doi:10.1186/s12863-023-01107-8
Elsby, L. M., and Roberts, S. G. (2008). Interaction of the TFIIB zinc ribbon with RNA polymerase II. London, United Kingdom: Portland Press Ltd.
Feng, F., Ma, X., Yan, M., Zhang, H., Mei, D., Fan, P., et al. (2023). Identification of genetic loci for rice seedling mesocotyl elongation in both natural and artificial segregating populations. Artif. Segreg. Popul. 12 (14), 2743. doi:10.3390/plants12142743
Feng, F., Mei, H., Fan, P., Li, Y., Xu, X., Wei, H., et al. (2017). Dynamic transcriptome and phytohormone profiling along the time of light exposure in the mesocotyl of rice seedling. Sci. Rep. 7 (1), 11961. doi:10.1038/s41598-017-12326-2
Ferdosi, M. H., Connors, N. K., and Tier, B. J. F. (2019). An efficient method to calculate genomic prediction accuracy for new individuals. Front. Genet. 10, 596. doi:10.3389/fgene.2019.00596
Finatto, T., Viana, V. E., Woyann, L. G., Busanello, C., Maia, L. C. d., Oliveira, A. C. J. G., et al. (2018). Can WRKY transcription factors help plants to overcome environmental challenges? Genet. Mol. Biol. 41, 533–544. doi:10.1590/1678-4685-GMB-2017-0232
Hahn, S., and Roberts, S. J. G. (2000). The zinc ribbon domains of the general transcription factors TFIIB and Brf: conserved functional surfaces but different roles in transcription initiation. Genes Dev. 14 (6), 719–730. doi:10.1101/gad.14.6.719
Hall, T. A. (1999). BioEdit: a user-friendly biological sequence alignment editor and analysis program for Windows 95/98/NT. Nucleic acids symp. Ser. 41, 95–98.
Han, M., Kim, C. Y., Lee, J., Lee, S.-K., and Jeon, J. S. J. M. (2014). OsWRKY42 represses OsMT1d and induces reactive oxygen species and leaf senescence in rice. Mol. Cells 37 (7), 532–539. doi:10.14348/molcells.2014.0128
Hossain, A., and Teixeira da Silva, J. A. (2013). Wheat production in Bangladesh: its future in the light of global warming. AoB Plants 5, pls042. doi:10.1093/aobpla/pls042
Huang, Q., Ju, C., Cheng, Y., Cui, D., Han, B., Zhao, Z., et al. (2022). QTL mapping of mesocotyl elongation and confirmation of a QTL in dongxiang common wild rice in China. Dongxiang common wild rice China 12 (8), 1800. doi:10.3390/agronomy12081800
Jang, S.-G., Park, S.-Y., Lar, S. M., Zhang, H., Lee, A.-R., Cao, F.-Y., et al. (2021). Genome-wide association study (GWAS) of mesocotyl length for direct seeding in rice. Agron. (Basel). 11 (12), 2527. doi:10.3390/agronomy11122527
Jeong, J. S., Kim, Y. S., Baek, K. H., Jung, H., Ha, S.-H., Do Choi, Y., et al. (2010). Root-specific expression of OsNAC10 improves drought tolerance and grain yield in rice under field drought conditions. Plant Physiol. 153 (1), 185–197. doi:10.1104/pp.110.154773
Kumar, V., and Ladha, J. K. J. A. (2011). Direct seeding of rice: recent developments and future research needs. Adv. Agron. 111, 297–413. doi:10.1016/B978-0-12-387689-8.00001-1
Lee, H.-S., Sasaki, K., Higashitani, A., Ahn, S.-N., and Sato, T. J. R. (2012). Mapping and characterization of quantitative trait loci for mesocotyl elongation in rice (Oryza sativa L.). Rice (N Y) 5 (1), 13–10. doi:10.1186/1939-8433-5-13
Lee, H.-S., Sasaki, K., Kang, J.-W., Sato, T., Song, W.-Y., and Ahn, S.-N. J. R. (2017). Mesocotyl elongation is essential for seedling emergence under deep-seeding condition in rice. Rice (N Y) 10 (1), 32–11. doi:10.1186/s12284-017-0173-2
Lee, S. M., Kang, J. W., Lee, J. Y., Seo, J., Shin, D., Cho, J. H., et al. (2020). QTL analysis for Fe and Zn concentrations in rice grains using a doubled haploid population derived from a cross between rice (Oryza sativa) cultivar 93-11 and milyang 352. Plant Breed. Biotechnol. 8 (1), 69–76. doi:10.9787/pbb.2020.8.1.69
Liang, Q., Wang, C., Ma, D., Li, L., Cui, Z., Wang, X., et al. (2016). Cortical microtubule disorganized related to an endogenous gibberellin increase plays an important role in rice mesocotyl elongation. Plant Biotechnol. 33 (2), 59–69. doi:10.5511/plantbiotechnology.16.0205a
Liu, X., Huang, M., Fan, B., Buckler, E. S., and Zhang, Z. J. P. g. (2016). Iterative usage of fixed and random effect models for powerful and efficient genome-wide association studies. PLoS Genet. 12 (2), e1005767. doi:10.1371/journal.pgen.1005767
Lu, Q., Zhang, M., Niu, X., Wang, C., Xu, Q., Feng, Y., et al. (2016). Uncovering novel loci for mesocotyl elongation and shoot length in indica rice through genome-wide association mapping. Planta 243, 645–657. doi:10.1007/s00425-015-2434-x
Lu, X.-L., Niu, A.-L., Cai, H.-Y., Zhao, Y., Liu, J.-W., Zhu, Y.-G., et al. (2007). Genetic dissection of seedling and early vigor in a recombinant inbred line population of rice. Plant Sci. 172 (2), 212–220. doi:10.1016/j.plantsci.2006.08.012
Mammadov, J., Aggarwal, R., Buyyarapu, R., and Kumpatla, S. J. I. (2012). SNP markers and their impact on plant breeding. Int. J. Plant Genomics 2012, 728398. doi:10.1155/2012/728398
Menard, G., Sandhu, N., Anderson, D., Catolos, M., Hassall, K. L., Eastmond, P. J., et al. (2021). Laboratory phenomics predicts field performance and identifies superior indica haplotypes for early seedling vigour in dry direct-seeded rice. Genomics 113 (6), 4227–4236. doi:10.1016/j.ygeno.2021.11.006
Ming-guo, W., Guang-heng, Z., Jian-rong, L., and Shi-hua, C. (2005). Screening for rice germplasms with specially-elongated mesocotyl. Rice Sci. 12 (3), 226.
Nagato, Y., Kitano, H., Kamijima, O., Kikuchi, S., Satoh, H. J. T., and genetics, a. (1989). Developmental mutants showing abnormal organ differentiation in rice embryos. Theor. Appl. Genet. 78, 11–15. doi:10.1007/BF00299746
Pillai, S. E., Kumar, C., Patel, H. K., and Sonti, R. V. J. B. (2018). Overexpression of a cell wall damage induced transcription factor, OsWRKY42, leads to enhanced callose deposition and tolerance to salt stress but does not enhance tolerance to bacterial infection. BMC Plant Biol. 18 (1), 177. doi:10.1186/s12870-018-1391-5
Prakash, S., Rai, R., Zamzam, M., Ahmad, O., Peesapati, R., and Vijayraghavan, U. J. F. (2022). OsbZIP47 is an integrator for meristem regulators during rice plant growth and development. plant growth Dev. 13, 865928. doi:10.3389/fpls.2022.865928
Price, A. L., Patterson, N. J., Plenge, R. M., Weinblatt, M. E., Shadick, N. A., and Reich, D. J. N. (2006). Principal components analysis corrects for stratification in genome-wide association studies. Nat. Genet. 38 (8), 904–909. doi:10.1038/ng1847
Redona, E., Mackill, D. J. T., and Genetics, A. (1996). Mapping quantitative trait loci for seedling vigor in rice using RFLPs. RFLPs 92, 395–402. doi:10.1007/BF00223685
Ren, Y., Huang, Z., Jiang, H., Wang, Z., Wu, F., Xiong, Y., et al. (2021). A heat stress responsive NAC transcription factor heterodimer plays key roles in rice grain filling. J. Exp. Bot. 72 (8), 2947–2964. doi:10.1093/jxb/erab027
Romaneckas, K., Steponavičius, D., Jasinskas, A., Kazlauskas, M., Naujokienė, V., Bručienė, I., et al. (2022). How to analyze, detect and adjust variable seedbed depth in site-specific sowing systems: a case study. Agronomy 12 (5), 1092. doi:10.3390/agronomy12051092
Souer, E., van Houwelingen, A., Kloos, D., Mol, J., and Koes, R. J. C. (1996). The no apical meristem gene of Petunia is required for pattern formation in embryos and flowers and is expressed at meristem and primordia boundaries. Cell 85 (2), 159–170. doi:10.1016/s0092-8674(00)81093-4
Takahashi, N. (1978). Adaptive importance of mesocotyl and coleoptile growth in rice under different moisture regimes. Funct. Plant Biol. 5 (4), 511–517. doi:10.1071/pp9780511
Thomson, M. J. J. P. B. (2014). High-throughput SNP genotyping to accelerate crop improvement. Plant Breed. Biotechnol. 2 (3), 195–212. doi:10.9787/pbb.2014.2.3.195
Uzair, M., Patil, S. B., Zhang, H., Kumar, A., Mkumbwa, H., Zafar, S. A., et al. (2022). Screening direct seeding-related traits by using an improved mesocotyl elongation assay and association between seedling and maturity traits in rice. Agronomy 12 (4), 975. doi:10.3390/agronomy12040975
Wang, G.-L., An, Y.-H., Zhou, C.-L., Hu, Z.-Z., Ren, X.-Q., and Xiong, A.-S. J. P. (2022). Transcriptome-wide identification of NAC (no apical meristem/Arabidopsis transcription activation factor/cup-shaped cotyledon) transcription factors potentially involved in salt stress response in garlic. PeerJ 10, e14602. doi:10.7717/peerj.14602
Wang, J., and Zhang, Z. J. G. (2021). GAPIT version 3: boosting power and accuracy for genomic association and prediction. Genomics Proteomics Bioinforma. 19 (4), 629–640. doi:10.1016/j.gpb.2021.08.005
Wang, Q., Tian, F., Pan, Y., Buckler, E. S., and Zhang, Z. J. P. (2014). A SUPER powerful method for genome wide association study. PLoS One 9 (9), e107684. doi:10.1371/journal.pone.0107684
Wang, Y., Liu, H., Meng, Y., Liu, J., and Ye, G. J. F. (2023). Validation of genes affecting rice mesocotyl length through candidate association analysis and identification of the superior haplotypes. Front. Plant Sci. 14, 1194119. doi:10.3389/fpls.2023.1194119
Wang, Y., Liu, J., Meng, Y., Liu, H., Liu, C., and Ye, G. J. F. (2021). Rapid identification of QTL for mesocotyl length in rice through combining QTL-seq and genome-wide association analysis. Front. Genet. 12, 713446. doi:10.3389/fgene.2021.713446
Wu, J., Feng, F., Lian, X., Teng, X., Wei, H., Yu, H., et al. (2015). Genome-wide Association Study (GWAS) of mesocotyl elongation based on re-sequencing approach in rice. BMC Plant Biol. 15 (1), 218. doi:10.1186/s12870-015-0608-0
You, Q., Yang, X., Peng, Z., Xu, L., and Wang, J. J. F. (2018). Development and applications of a high throughput genotyping tool for polyploid crops: single nucleotide polymorphism (SNP) array. Front. Plant Sci. 9, 104. doi:10.3389/fpls.2018.00104
Yu, G.-E., Shin, Y., Subramaniyam, S., Kang, S.-H., Lee, S.-M., Cho, C., et al. (2021). Machine learning, transcriptome, and genotyping chip analyses provide insights into SNP markers identifying flower color in Platycodon grandiflorus. Platycodon Gd. 11 (1), 8019. doi:10.1038/s41598-021-87281-0
Yu, J., Pressoir, G., Briggs, W. H., Vroh Bi, I., Yamasaki, M., Doebley, J. F., et al. (2006). A unified mixed-model method for association mapping that accounts for multiple levels of relatedness. Nat. Genet. 38 (2), 203–208. doi:10.1038/ng1702
Zhan, J., Lu, X., Liu, H., Zhao, Q., and Ye, G. (2020). Mesocotyl elongation, an essential trait for dry-seeded rice (Oryza sativa L.): a review of physiological and genetic basis. Planta 251, 27–14. doi:10.1007/s00425-019-03322-z
Zhang, Z., Ersoz, E., Lai, C. Q., Todhunter, R. J., Tiwari, H. K., Gore, M. A., et al. (2010). Mixed linear model approach adapted for genome-wide association studies. Nat. Genet. 42 (4), 355–360. doi:10.1038/ng.546
Zhang, Z., Liu, X., Zhou, Y., and Summers, R. J. G. (2018). BLINK: a package for the next level of genome-wide association studies with both individuals and markers. Meng Huang 8, 1–12. doi:10.1093/gigascience/giy154
Zhang, Z., Todhunter, R., Buckler, E., and Van Vleck, L. D. J. J. (2007). Technical note: use of marker-based relationships with multiple-trait derivative-free restricted maximal likelihood. J. Anim. Sci. 85 (4), 881–885. doi:10.2527/jas.2006-656
Keywords: mesocotyl, emergence, GWAS, GAPIT, SNP chip DNA markers
Citation: Kabange NR, Alibu S, Kwon Y, Lee S-M, Oh K-W and Lee J-H (2023) Genome-wide association study (GWAS) with high-throughput SNP chip DNA markers identified novel genetic factors for mesocotyl elongation and seedling emergence in rice (Oryza sativa L.) using multiple GAPIT models. Front. Genet. 14:1282620. doi: 10.3389/fgene.2023.1282620
Received: 24 August 2023; Accepted: 08 November 2023;
Published: 20 November 2023.
Edited by:
Li Ma, University of Maryland, College Park, United StatesReviewed by:
Yingpeng Han, Northeast Agricultural University, ChinaSeong-Gyu Jang, Pusan National University, Republic of Korea
Copyright © 2023 Kabange, Alibu, Kwon, Lee, Oh and Lee. This is an open-access article distributed under the terms of the Creative Commons Attribution License (CC BY). The use, distribution or reproduction in other forums is permitted, provided the original author(s) and the copyright owner(s) are credited and that the original publication in this journal is cited, in accordance with accepted academic practice. No use, distribution or reproduction is permitted which does not comply with these terms.
*Correspondence: Nkulu Rolly Kabange, rollykabange@korea.kr; Jong-Hee Lee, ccriljh@korea.kr
†These authors have contributed equally to this work