- 1Department of Epidemiology and Biostatistics, School of Public Health, Anhui Medical University, Hefei, China
- 2Hefei Center for Disease Control and Prevention, Hefei, China
- 3The Second Hospital of Anhui Medical University, Hefei, China
- 4Department of Maternal, Child, and Adolescent Health, School of Public Health, Anhui Medical University, Hefei, China
Background: Smartphone addiction (SA) is associated with adverse consequences, especially for freshmen. Evidence indicates that SA is associated with depression, and it is necessary to conduct a longitudinal study to explore the association further.
Methods: SA (measured by the Smartphone Addiction Scale-Short Version) and depression (measured by the Zung's Self-Rating Depression Scale) among 1,186 freshmen were surveyed at baseline and a respective 12-month follow-up for each participant. The application of a cross-lagged panel model approach (CLPM) revealed an association between SA and depression after adjusting for demographic variables.
Results: The CLPM results showed a significant path from baseline SA to follow-up depression (β = 0.08, P < 0.001) and a significant path from baseline depression to follow-up SA (β = 0.08, P < 0.001). Compared with the overall cross-lagged model, the cross-lagged coefficient of the path from baseline SA to follow-up depression increased in the female group (β = 0.10, P = 0.015), and the cross-lagged coefficient of the path from baseline depression to follow-up SA also increased significantly (β = 0.15, P < 0.001). In contrast, the cross-lagged model in the male group showed no predictive effect between SA and depression (P > 0.05).
Conclusions: The current study showed a significant bidirectional association between smartphone addiction and depression among freshmen, but only in the female population.
1. Introduction
Smartphone addiction (SA) is characterized by excessive attention and uncontrolled dedication to one's smartphone (1). It has been observed that SA has worryingly increased worldwide in recent years. Zhong et al. (2) conducted a systematic review and found that the prevalence of SA among Asian medical students was 41.93%. Meng et al. (3) found that the global pooled prevalence of SA was 34.5% among college and high school student populations over the last 2 years. In addition, numerous studies have shown that SA is associated with several psychiatric comorbidities, including depression, anxiety, autism spectrum disorder and suicide attempt (4–7). Depression is also common among adolescents. A recent systematic review reported a global prevalence of depression among adolescents of 34% (95% CI: 30–38%), with a higher prevalence among females than males (8). Depression can have serious adverse health consequences for adolescents and may increase the risk of suicide attempts, substance abuse, anxiety disorders, and comorbid somatic disorders (9). Freshmen are a particular population transitioning from high school to college, facing new physical and interpersonal environments, and prone to mental health problems (10). After leaving the high school environment with a heavy academic load and little free time and entering relatively free college life, freshmen also have more time to use smartphones. In addition, they may become heavily dependent on smartphones, either as a tool to stay in touch with family and friends or as a necessary tool for studying and living in a new environment (11).
Different studies have provided preliminary empirical support for the association between SA and depression. In cross-sectional studies, some suggest that SA may be a risk factor for depression (12, 13), while others suggest that depression may be a risk factor for SA (14, 15). Li et al. pooled 21 studies for meta-analysis and showed that SA was weakly to moderately positively associated with depression (r=0.36) (16). Several hypotheses or approaches have been proposed to explain the interrelationship between SA and depression. The first is the Compensatory Internet Use Theory (CIUT) (17). This theory states that individuals' use of smartphones is a means for them to cope with painful emotional states and to access social needs that may not be met in the real world (17). In this model, depressed individuals have difficulty feeling pleasure gained from social interactions but tend to use their phones more frequently as an adaptive coping mechanism in difficult situations and may be prone to SA (18, 19). The second is the upward social comparison hypothesis, which refers to people comparing themselves with those they perceive to be in a more favorable position (20). For this hypothesis, repeated exposure to idealized information during smartphone use may lower the user's self-esteem, trigger depression, and enhance depression over time (21). In addition, Samra et al. (22) noted that females used social media more problematically and compared themselves more negatively to others on social media than males. This suggests the need to pay attention to gender differences while exploring the relationship between SA and depression.
It has been reported that female students report a higher prevalence of depressive symptoms than male students (9). Crockett et al. (23) found that females have more depressive moods but fewer problems with concentration and psychomotor retardation/agitation than males. Albursan et al. (24) indicated that although female students were more likely to use smartphones, the effect of over-reliance on smartphones on academic achievement appears to be more pronounced among male students. Zhu et al. (25) found that depression predicted Internet addiction only among males but not among females, suggesting that the bidirectional predictive relationship between Internet addiction and depression may depend on gender. Considering that the above study found gender differences in addictive behaviors and depressive symptoms, it is necessary to further explore the role of gender in the longitudinal association of SA and depression.
Longitudinal survey analysis to test these hypotheses concerning the potential etiological association between SA and depression will undoubtedly be valuable. Zhou et al. (26) conducted a 6-month follow-up of 313 high school students and found that depression unidirectionally predicted SA. Chen et al. (27) conducted a 9-month follow-up of 308 Hong Kong university students and found that the growth of SA was positively associated with depression. Published longitudinal studies are limited in several ways, as follows, relatively small sample sizes, short follow-up intervals, no studies have focused on the stability of SA or depression, and no consideration of the role of gender as an essential factor in the relationship. In light of the limitations of published studies, a longitudinal study is warranted to explore the association between SA and depression further. The present study aims to test the hypothesized bidirectional association between smartphone addiction and depression among freshmen using the CLPM model and to explore the role of gender in this association.
2. Methods
2.1. Participants
This is a one-year prospective study. In September 2020, we recruited 1226 freshmen from a medical college in Hefei, Anhui Province, China. An electronic questionnaire was used, with the investigator providing a QR code between classes and students scanning the code with their smartphones to fill out the questionnaire. Among the included respondents, 1,186 (96.47%) also completely responded to the questions relevant to the present study in a respective follow-up survey after 12 months. The questionnaire completion was anonymous, and data from the two surveys were matched according to a unique code assigned to each student. Informed consent was obtained from every participant prior to two questionnaire surveys. The Research Ethics Committee has approved this study of the Anhui Medical University (No. 20190495).
2.2. Measures
The data collected in the present study included socio-demographics, living habits, and health conditions. The variables examined in the socio-demographic section included gender, age, and their parents' education level (bachelor degree or higher). The lifestyle factors included body-mass index (BMI), daily exercise time (hours), daily smartphone use time (hours), and sleep time per night (hours). The health conditions included smartphone addiction (SA) and depression severity, measured by the Smartphone Addiction Scale-Short Version (SAS-SV) (Supplementary Tables 1, 2) and the Zung's Self-Rating Depression Scale (SDS) (Supplementary Tables 3, 4), respectively.
The Smartphone Addiction Scale-Short Version (SAS-SV) consisted of 10 symptoms of excessive smartphone use (28). Six experts selected 10 items of this short version from the original 33-item SAS. Each item was scored on a six-point Likert scale, and a higher total score indicates a more severe level of smartphone addiction. The widely used SAS-SV cut-off scores of ≥31 for males and ≥33 for females were used as proposed by the scale developers (28). We used the version translated by Xiang et al., which has been shown in previous studies to have good reliability and validity of SAS-SV in Chinese populations (29). In this study, Cronbach's α for SAS-SV at baseline and follow-up surveys were 0.83 and 0.89, respectively. Confirmatory factor analysis showed an acceptable model fit of SAS-SV at the baseline survey (χ2/df = 12.83, CFI =0.87, GFI =0.92, and RMSEA = 0.10) and at the follow-up survey (χ2/df = 19.74, CFI =0.88, GFI =0.88, and RMSEA = 0.13).
Zung's Self-Rating Depression Scale (SDS) has been widely used to assess depression during the past week (30). It consists of 20 self-rated questions, each item rated on a 4-point scale ranging from 1 (a little of the time) to 4 (most of the time). The total score was acquired by multiplying the raw score by 1.25. A higher total score indicates a more severe level of depression. An SDS score of 50 (raw score = 40) suggests clinically significant symptoms (31). Many previous studies had used the Chinese versions of SDS (32) to evaluate the depression symptoms in Chinese population including adolescents (33). In this study, Cronbach's α for SDS at baseline and follow-up surveys were 0.80 and 0.84, respectively. Confirmatory factor analysis showed that the χ2/df, CFI, GFI, and RMSEA of SDS at baseline survey were 7.47, 0.75, 0.88, and 0.07, respectively; and the χ2/df, CFI, GFI, and RMSEA at follow-up survey were 19.08, 0.62, 0.68, and 0.12, respectively.
2.3. Statistical procedures
Descriptive characteristics were investigated at baseline between the responders and non-responders (Table 1). Categorical variables were reported as frequency (percentages), and continuous variables were reported as means (standard deviations).
Univariate differences in demographic variables frequencies and rates in individuals with SA and depression were tested with the Chi-square test. Adjusted odds ratios (ORs) and their 95% CIs for individuals with SA or depression and demographic groups were estimated using a binary logistic regression (Enter) model adjusted for confounding effects. For the binary logistic regression (Enter) model, the dependent variables were the status of SA or depression (Yes or No), and the independent variables were age, biological gender, parental education level, maternal education level, and BMI.
Furthermore, AMOS 26.0 was used to calculate structural equation models (SEM) in the cross-lagged panel model (CLPM) design, including the follow-up sample (n = 1,186) to examine possible bidirectional effects between SAS-SV scores and SDS scores. Model fit was evaluated using χ2 index (χ2/df ), comparative fit index (CFI), goodness-of-fit index (GFI), and root-mean-square error of approximation (RMSEA). Eventually, we performed a multi-group analysis by gender. Statistical significance was accepted at P < 0.05 in all analyses.
3. Results
3.1. Demographics
Characteristics between responders and non-responders of the study sample are presented in Table 1. There were 1,186 (96.74%) participants responding at follow-up. No significant differences were found between participants who responded to the baseline survey only and those who responded to both in terms of socio-demographics, living habits and health conditions. In view of this result, we focused mainly on reporting data from participants who responded to both surveys (n = 1,186) in the following sections.
3.2. The rates and associated factors of SA and depression at baseline and follow-up surveys
The rates of baseline SA and depression were 32.0% (95% CI: 29.4%, 34.7%) and 10.7% (95% CI: 8.9%, 12.5%), respectively; they increased to 52.5% (95% CI: 49.7%, 55.4%) and 28.2% (95% CI: 25.7%, 30.8%) at follow-up, respectively (Tables 2, 3).
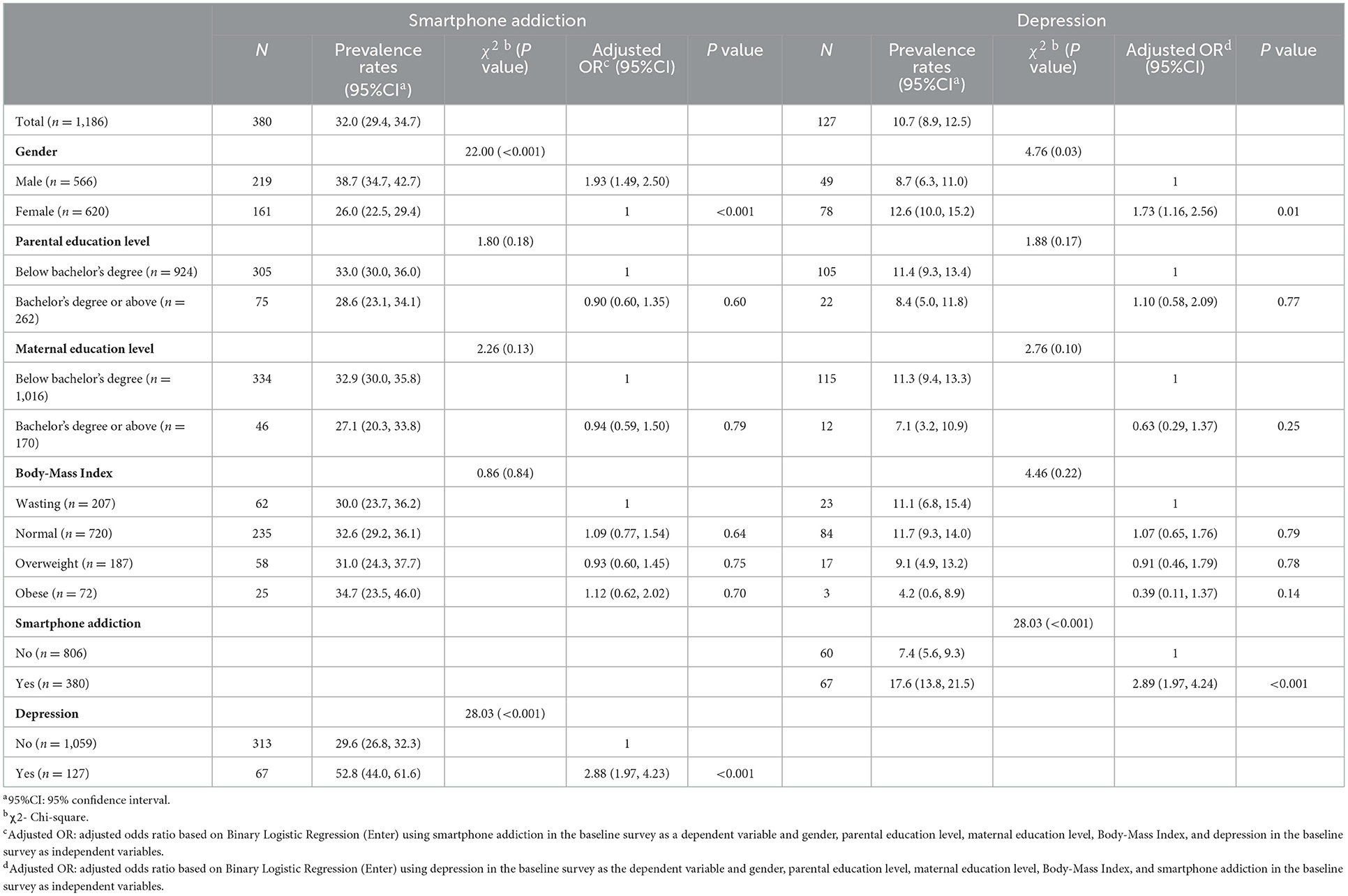
Table 2. The detection rates and predictors for smartphone addiction and depression in the baseline among respondents who responded to both surveys.
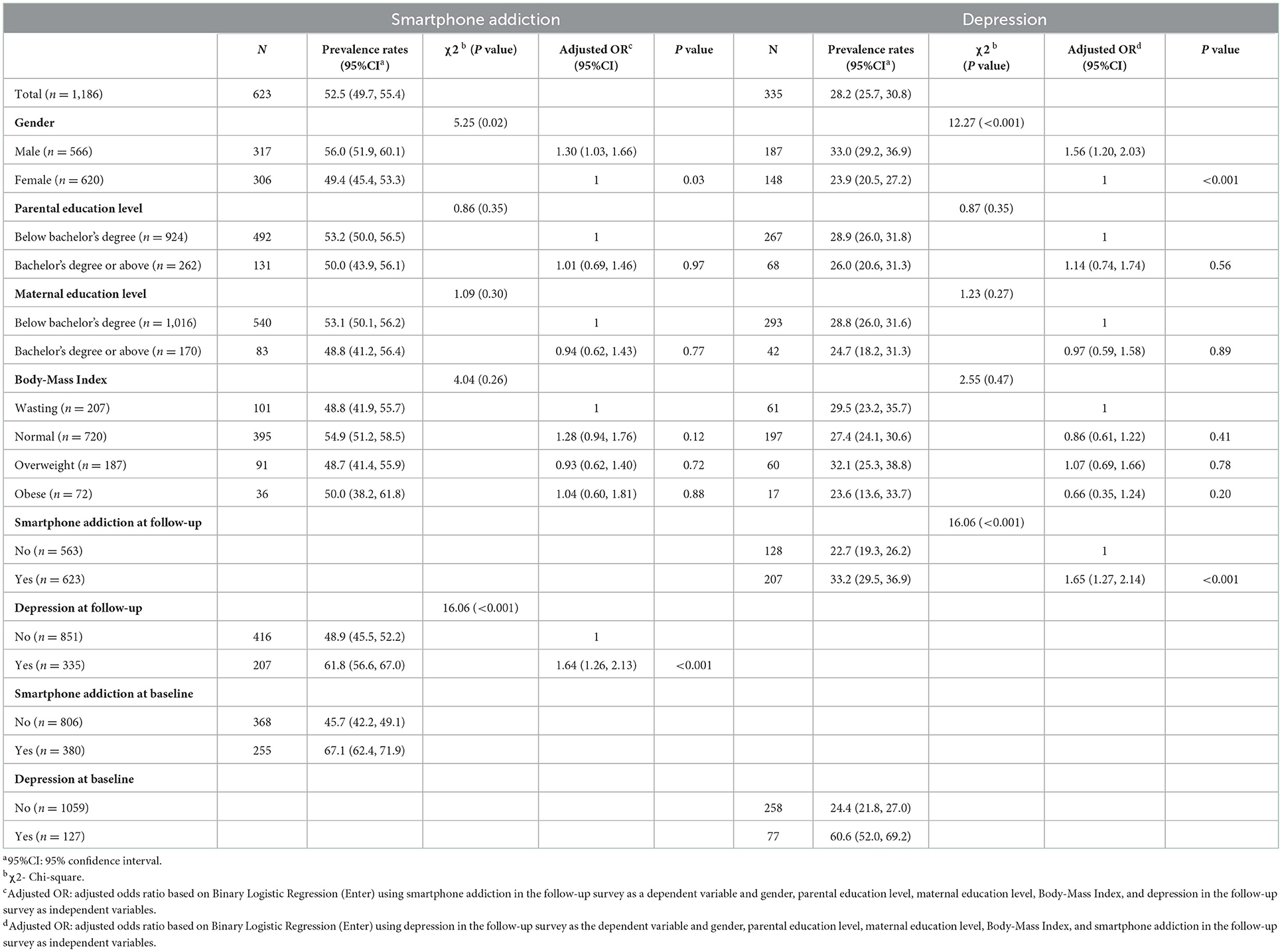
Table 3. The detection rates and predictors for smartphone addiction and depression in the following among respondents who responded to both surveys.
SA was more prevalent among males than in females, both at baseline and follow-up survey [aOR at baseline: 1.93 (95% CI: 1.49, 2.50); aOR at follow-up: 1.30 (95% CI: 1.03, 1.66)]. Depression was more prevalent among females at baseline [aOR: 1.73 (95% CI: 1.16, 2.56)]; but more prevalent among males at follow-up [aOR: 1.56 (95% CI: 1.20, 2.03)] (Tables 2, 3).
A strong cross-sectional association was observed between SA and depression for both the baseline and follow-up surveys. The adjusted OR (aOR) values after controlling for demographics were 2.88 (95% CI: 1.97, 4.23) and 1.65 (95% CI: 1.27, 2.14) for the baseline and follow-up surveys, respectively (Tables 2, 3).
3.3. The stability and new incidence of SA and depression over 12 months
Regarding the stability of SA and depression over 12 months, we observed that 67.1% (95% CI: 62.4%, 71.9%) of the participants in the baseline still scored over the SAS-SV threshold after 12 months; in addition, 60.6% (95% CI: 52.0%, 69.2%) of the participants in the cohort with baseline depressive symptoms still scored over the SDS threshold in the follow-up survey (Table 3).
Regarding the new incidence of SA and depression over 12 months, 45.7% (95% CI: 42.2%, 49.1%) of participants without baseline SA scored over the SAS-SV threshold after 12 months; in the follow-up survey, 24.4% (95% CI: 21.8%, 27.0%) of the participants without baseline depressive symptoms scored over the SDS threshold (Table 3).
3.4. The CLPM analysis between severities of SA and depression
A well-fitted CLPM between severities of SA and depression was revealed by SEM analysis using continuous data, based on SAS-SV and SDS scores. The χ2 index (χ2/df ) was 32.16 (χ2 = 32.16, df =1, P < 0.001), CFI =0.94, GFI =0.99, and RMSEA = 0.16 (95% CI: 0.12, 0.21). All of these observed fit-indices reached the recommended cut-off values based on previous studies, except RMSEA. After adjusting for the effect of the covariates, although the severity of SA in the follow-up survey was predicted by baseline depression severity (path coefficient: 0.08; P = 0.008), the path coefficient was smaller than the predictive effect of the baseline SA severity relative to follow-up SA severity (0.26, P < 0.001) and the cross-sectional association between baseline SA severity and depression severity at baseline (0.34, P < 0.001) and follow-up (0.23, P < 0.001). Again, although depression severity in the follow-up survey was predicted by baseline SA severity (path coefficient: 0.08; P = 0.008), the path coefficient was smaller than that of the predictive effect of the severity of baseline depression to the severity of follow-up depression (0.37; P < 0.001) and cross-sectional associations between SA and depression in the baseline and follow-up surveys, respectively (Figure 1 and Table 4).
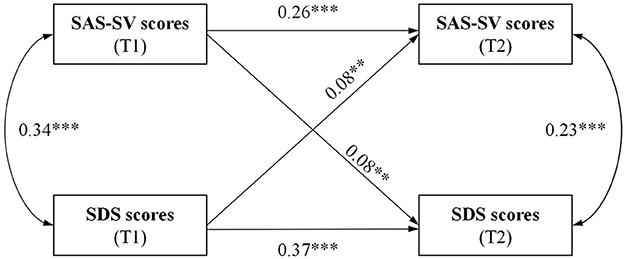
Figure 1. CLPM of SAS-SV and SDS scores at baseline (T1) and follow-up (T2, over 12 months) with standardized path coefficients. **P < 0.01; ***P < 0.001; T1 = assessed at baseline, T2 = assessed at follow-up. SAS-SV, Smartphone Addiction Scale-Short Version; SDS, Zung's Self-Rating Depression Scale; Single-headed arrows denote regressions, double-headed arrows denote correlations.
3.5. Multi-group analysis by gender
Multi-group analyses by gender of the CLPM was conducted, and the models revealed satisfactory model fit [male group: χ2/df = 15.08 (χ2 = 15.08, df =1, P < 0.001), CFI = 0.93, GFI = 0.99, and RMSEA = 0.16 (95% CI: 0.09, 0.23); female group: χ2/df = 23.13 (χ2 = 23.13, df =1, P < 0.001), CFI =0.93, GFI =0.98, and RMSEA = 0.19 (95% CI: 0.13, 0.26)]. Among the male group, the relationship between SAS-SV and SDS scores was not significant in either direction. In contrast, among the female group, baseline depression severity predicted the SA severity in the follow-up survey (path coefficient: 0.15; P < 0.001), and also, baseline SA severity predicted depression severity in the follow-up survey (path coefficient: 0.10; P = 0.015) (Figure 2 and Table 4).
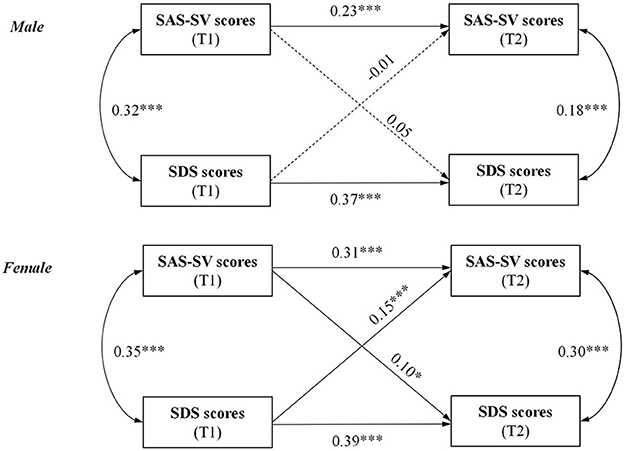
Figure 2. Path diagram of the CLPM by gender. *P < 0.05; **P < 0.01; ***P < 0.001; T1, assessed at baseline; T2, assessed at follow-up. SAS-SV, Smartphone Addiction Scale-Short Version; SDS, Zung's Self-Rating Depression Scale; Single-headed arrows denote regressions, double-headed arrows denote correlations; Solid lines present statistically significant associations and dashed lines present nonsignificant associations.
4. Discussion
In this study, we adopted a two-wave longitudinal design and constructed a cross-lagged panel model (CLPM) model to examine the bidirectional relationship between SA and depression among freshmen. This study showed that, (1) high rates of SA and depression exist in the freshman cohort; (2) both SA and depression rates among freshmen are highly stable during the first year of college, while this period is also a high prevalence of SA and depression among baseline normal freshmen; (3) SA was significantly associated with depression in cross-sectional analyses at both time points; (4) CLPM showed that SA and depression were bidirectionally associated and this relationship was significant in the female group but not in the male group.
The rate of SA among incoming freshmen was 32.0%; at the time of follow-up, at the beginning of the sophomore year, this rate increased to 52.5%. This indicates that college freshmen may use their smartphones more and have a higher risk of SA. Of these, a significant number (67.1%) of students with baseline SA were still addicted to their smartphones at 12 months. This finding is important because some researchers have expressed doubts about the stability of SA and similar conditions when debating the validity of SA as a disorder (34, 35). Also, close to half of the students (45.7%) with non-SA at baseline developed SA symptoms at follow-up. Actually, the rate of SA (36, 37) among teenagers has risen dramatically during the COVID-19 pandemic. The incidence of SA among Italian adolescents has also increased, from 26.1% before the pandemic to 46.7% during the pandemic (36). Fund et al. (37) investigated a population of elementary school students in China and found that SA was significantly higher during the outbreak. Olvera et al. (38) indicated that TikTok saw a phenomenal increase in popularity during the COVID-19 pandemic, while Marengo et al. (39) found that TikTok was the most addictive application during this period. This also proves that it is important to guide and correct smartphone use behavior during the pandemic for college students, especially freshmen.
SA was more prevalent among males than females at baseline and follow-up surveys. It is inconsistent with previous studies, which suggested that females were significantly associated with a propensity for SA (34, 40, 41). We argued that such inconsistent findings might partially be attributed to the fact that the study population in this study was freshmen, that male students may be more proficient in using technology (42) and that there are some differences in the content of smartphone use between males and females (40, 43). Consistent with our results, a study conducted at an Indian medical school found that the SA rate reached 52.0% and was more prevalent in males than females (44). In addition, several other studies have found no significant gender differences in the prevalence of SA (45, 46). Further studies still need to unravel the inconsistent prevalence of SA in males and females.
As for depression, in our study, the prevalence of depression among freshmen was 10.7%, while one year later, this rate rose to 28.8%, and the new incidence during this period was 24.4%. On the one hand, this indicates that the prevalence of depression is high among Chinese adolescents. Duan et al. (13) indicated that the COVID-19 outbreak has had a significant psychosocial impact on Chinese adolescents and that high school graduates affected by the outbreak were significantly associated with depression. On the other hand, it points to a high prevalence of depression among freshmen. Ebert et al. (10) found that the incidence of major depressive disorder (MDD) within the first year of college was 6.9%, suggesting that the first year in college constitutes a risk period for the onset of MDD. Considering the context of life transition, freshmen are in an unfamiliar transition period and are vulnerable to depression and other mental health problems (47). During this period, students face a change in their living environment, a change in their learning methods, and a change from dependence to autonomy, and if they cannot adapt to this collective life in time, they can easily fall into emotional distress. As to gender, this is a controverted matter, with some studies suggesting that females are more likely to suffer from depression (48, 49), while in contrast, other researchers insist that males are more likely to experience depression than females (50). Our study found that the prevalence of depression was higher among freshmen females, and after 1 year, it was higher among males. A plausible explanation is that gender may have a significant effect only at the stage from moderate to severe depression (49), and the present study did not classify the severity level of depression. Therefore, the problem of depression among college students continues to require close attention and effort.
The CLPM results showed that the overall association between SA and depression was bidirectional; however, multi-group analysis by gender revealed that this bidirectional association persisted in the female group, whereas in the male group, the association was not significant in either direction. Among the female group, the cross-lagged effect coefficient from depression to SA was relatively larger than the reverse effect, suggesting that depressive symptoms may worsen SA to a greater extent. This echoes the previous findings, which suggested that SA or similar conditions can be caused by depressive symptoms (14, 51). Studies have found that depressed individuals derive less pleasure from social interactions and have increased sensitivity to social rejection (52, 53), but have a tendency to use social media more frequently (54). Frequent smartphone use as an avoidance-coping strategy appears to present them with a viable alternative to uncomfortable face-to-face contact in social situations. Furthermore, Elhai et al. (55) argued that smartphone use has also been proposed as a coping process for depressed mood (boredom tendencies, mood dysregulation, and pain intolerance). This avoidance-coping tends to foster reliance on online activities (52, 56). In the context of excessive smartphone use, not only may irrational beliefs or perceptions arise (feelings of inferiority, insecurity and self-esteem fluctuations) (57), but also social comparisons, a phenomenon of “emotional contagion” (58), leading to negative emotions such as depression and anxiety (59). Studies have also found that SA can lead to sleep disorders and delayed sleep, which are predictors of depression (60). Thus, SA and depression may form a vicious circle. Therefore, preventive control measures targeting depression should focus on smartphone use, while interventions targeting SA should incorporate a psychological component and appropriately increase attention to the female group.
This finding of gender differences is inconsistent with the studies of Park et al. (61), who observed significant changes in the longitudinal relationship between SA and depression among Korean adolescents across time, but no gender differences were found in the strength of these relationships. Possible mechanisms lie in physiological differences between the sexes (e.g., genetic vulnerability) (62), differences in self-concept (63), and differences in stress perception (64) that result in different emotional responses and behavioral patterns. Compared to males, females are more likely to perceive stress, and self-distraction may be considered one of the effective coping mechanisms (64). Females are more likely to internalize their negative emotions, whereas males' resort to externalizing behaviors, such as aggression and substance use (65, 66). Tang et al. (67) found that although female freshmen received more social support and had better help-seeking skills than males, females exhibited higher rates of depression. One possible explanation may be that females are perceived as more frequently, emotionally, and relatively exposed to stressful situations compared to males (68). Another reason could be that females are more likely to seek support through mobile social software, and their dependence on smartphones leads to a great sense of loss when they face real life (69). The findings suggest that researchers and practitioners have to take gender differences in understanding the bidirectional influences between SA and depression.
The current study has several limitations. Firstly, we recruited only freshmen from the same university to ensure consistent learning patterns. It may be possible to obtain richer results if college students in different years could be surveyed simultaneously at the same time. Therefore, further studies with a larger sample of representative Chinese college students are necessary to confirm our present findings. Secondly, only two-time points were assessed in this study. More waves and longer years of follow-up are warranted to understand better the stability of and changes in SA and depression. Third, the results of the validated factor analysis of SDS in this study were unsatisfactory, which we thought might be related to the fact that the study subjects were medical students with some medical background and were relatively familiar with the SDS scale. Attention should be paid to the selection of scales in future studies of depressive tendencies in college students, especially medical students. Fourth, other relevant questions, such as type of smartphone use, objective smartphone use time, and frequency of use, were not investigated, so we could not provide explicit confirmation of addicts' use patterns to support the discussion around this topic.
5. Conclusions
In conclusion, the study found that both smartphone addiction (SA) and depression were prevalent among freshmen and that there was a bidirectional predictive association between SA and depression, especially and only in the female group. Therefore, we should strengthen early intervention for behaviors related to SA among freshmen while focusing on related mental health issues.
Data availability statement
The raw data supporting the conclusions of this article will be made available by the authors, without undue reservation.
Ethics statement
The studies involving human participants were reviewed and approved by Anhui Medical University (No. 20190495). Written informed consent from the participants' legal guardian/next of kin was not required to participate in this study in accordance with the national legislation and the institutional requirements.
Author contributions
KZ: conceptualization, formal analysis, writing–original draft, writing–review, and editing. HG: methodology, investigation, and data curation. TW: investigation, data curation, and formal analysis. JZ, GY, JR, XZ, and HY: investigation and data curation. XL, ZZhu, JD, HS, and GJ: investigation. JH, YS, and PS: validation and project administration. ZZha: validation and funding acquisition. All authors contributed to the article and approved the submitted version.
Funding
This work was supported in part by the National Natural Science Foundation of China (No. 81973064).
Acknowledgments
The authors would like to thank all the college students who participated in the studies, research personnel, and master students for their valuable contributions to this research. The authors are indebted to the others of research group of Anhui Medical University, Anhui Provincial Center for Disease Control and Prevention, for their excellent operation and helpful discussion.
Conflict of interest
The authors declare that the research was conducted in the absence of any commercial or financial relationships that could be construed as a potential conflict of interest.
Publisher's note
All claims expressed in this article are solely those of the authors and do not necessarily represent those of their affiliated organizations, or those of the publisher, the editors and the reviewers. Any product that may be evaluated in this article, or claim that may be made by its manufacturer, is not guaranteed or endorsed by the publisher.
Supplementary material
The Supplementary Material for this article can be found online at: https://www.frontiersin.org/articles/10.3389/fpubh.2023.1083856/full#supplementary-material
References
1. De-Sola Gutiérrez J, Rodríguez de Fonseca F, Rubio G. Cell-phone addiction: a review. Front Psychiatry. (2016) 7:175. doi: 10.3389/fpsyt.2016.00175
2. Zhong Y, Ma H, Liang YF, Liao CJ, Zhang CC, Jiang WJ. Prevalence of smartphone addiction among Asian medical students: a meta-analysis of multinational observational studies. Int J Soc Psychiatry. (2022) 68:1171–83. doi: 10.1177/00207640221089535
3. Meng SQ, Cheng JL, Li YY, Yang XQ, Zheng JW, Chang XW, et al. Global prevalence of digital addiction in general population: a systematic review and meta-analysis. Clin Psychol Rev. (2022) 92:102128. doi: 10.1016/j.cpr.2022.102128
4. Lapierre MA, Zhao P, Custer BE. Short-term longitudinal relationships between smartphone use/dependency and psychological well-being among late adolescents. J Adolesc Health. (2019) 65:607–12. doi: 10.1016/j.jadohealth.2019.06.001
5. Ratan ZA, Parrish AM, Zaman SB, Alotaibi MS, Hosseinzadeh H. Smartphone Addiction and Associated Health Outcomes in Adult Populations: a Systematic Review. Int J Environ Res Public Health. (2021) 18:12257. doi: 10.3390/ijerph182212257
6. Fineberg NA, Menchón JM, Hall N, Dell'Osso B, Brand M, Potenza MN, et al. Advances in problematic usage of the internet research - A narrative review by experts from the European network for problematic usage of the internet. Compr Psychiatry. (2022) 118:152346. doi: 10.1016/j.comppsych.2022.152346
7. Weinstein A, Siste K. Editorial: excessive and problematic smartphone usage. Front Psychiatry. (2022) 13:972613. doi: 10.3389/fpsyt.2022.972613
8. Shorey S, Ng ED, Wong CHJ. Global prevalence of depression and elevated depressive symptoms among adolescents: a systematic review and meta-analysis. Br J Clin Psychol. (2022) 61:287–305. doi: 10.1111/bjc.12333
9. Zhang X, Yang H, Zhang J, Yang M, Yuan N, Liu J. Prevalence of and risk factors for depressive and anxiety symptoms in a large sample of Chinese adolescents in the post-COVID-19 era. Child Adolesc Psychiatry Ment Health. (2021) 15:80. doi: 10.1186/s13034-021-00429-8
10. Ebert DD, Buntrock C, Mortier P, Auerbach R, Weisel KK, Kessler RC, et al. Prediction of major depressive disorder onset in college students. Depress Anxiety. (2019) 36:294–304. doi: 10.1002/da.22867
11. Liu H, Zhou Z, Zhu E, Huang L, Zhang M. Smartphone addiction and its associated factors among freshmen medical students in China: a cross-sectional study. BMC Psychiatry. (2022) 22:308. doi: 10.1186/s12888-022-03957-5
12. Ng KC, Wu LH, Lam HY, Lam LK, Nip PY, Ng CM, et al. The relationships between mobile phone use and depressive symptoms, bodily pain, and daytime sleepiness in Hong Kong secondary school students. Addict Behav. (2020) 101:105975. doi: 10.1016/j.addbeh.2019.04.033
13. Duan L, Shao X, Wang Y, Huang Y, Miao J, Yang X, et al. An investigation of mental health status of children and adolescents in china during the outbreak of COVID-19. J Affect Disord. (2020) 275:112–8. doi: 10.1016/j.jad.2020.06.029
14. Pera A. The psychology of addictive smartphone behavior in young adults: problematic use, social anxiety, depressive stress. Front Psychiatry. (2020) 11:573473. doi: 10.3389/fpsyt.2020.573473
15. Matar Boumosleh J, Jaalouk D. Depression, anxiety, and smartphone addiction in university students- a cross sectional study. PLoS ONE. (2017) 12:e0182239. doi: 10.1371/journal.pone.0182239
16. Li Y, Li G, Liu L, Wu H. Correlations between mobile phone addiction and anxiety, depression, impulsivity, and poor sleep quality among college students: a systematic review and meta-analysis. J Behav Addict. (2020) 9:551–71. doi: 10.1556/2006.2020.00057
17. Kardefelt-Winther D. A conceptual and methodological critique of internet addiction research: towards a model of compensatory internet use. Comput Human Behav. (2014) 31:351–4. doi: 10.1016/j.chb.2013.10.059
18. Vally Z. Anger and worry are related to problematic smartphone use: a cross-sectional examination of novel psychopathological constructs in a college-aged sample in the United Arab Emirates. Heliyon. (2022) 8:e10917. doi: 10.1016/j.heliyon.2022.e10917
19. Long J, Liu TQ, Liao YH, Qi C, He HY, Chen SB, et al. Prevalence and correlates of problematic smartphone use in a large random sample of Chinese undergraduates. BMC Psychiatry. (2016) 16:408. doi: 10.1186/s12888-016-1083-3
20. Feinstein BA, et al. Negative social comparison on facebook and depressive symptoms: rumination as a mechanism. Psychol Pop Media Cult. (2013) 2:161–70. doi: 10.1037/a0033111
21. Boers E, Afzali MH, Newton N, Conrod P. Association of screen time and depression in adolescence. JAMA Pediatr. (2019) 173:853–9. doi: 10.1001/jamapediatrics.2019.1759
22. Samra A, Warburton WA, Collins AM. Social comparisons: a potential mechanism linking problematic social media use with depression. J Behav Addict. (2022) 11:607–14. doi: 10.1556/2006.2022.00023
23. Crockett MA, Martínez V, Jiménez-Molina Á. Subthreshold depression in adolescence: Gender differences in prevalence, clinical features, and associated factors. J Affect Disord. (2020) 272:269–76. doi: 10.1016/j.jad.2020.03.111
24. Albursan IS, Qudah MFA, Al-Barashdi HS, Bakhiet SF, Darandari E, Al-Asqah SS, et al. Smartphone addiction among university students in light of the COVID-19 pandemic: prevalence, relationship to academic procrastination, quality of life, gender and educational stage. Int J Environ Res Public Health. (2022) 19:10439. doi: 10.3390/ijerph191610439
25. Zhu X, Shek DTL, Chu CKM. Internet addiction and emotional and behavioral maladjustment in mainland chinese adolescents: cross-lagged panel analyses. Front Psychol. (2021) 12:781036. doi: 10.3389/fpsyg.2021.781036
26. Zhou H, Dang L, Lam LW, Zhang MX, Wu AMS. A cross-lagged panel model for testing the bidirectional relationship between depression and smartphone addiction and the influences of maladaptive metacognition on them in Chinese adolescents. Addict Behav. (2021) 120:106978. doi: 10.1016/j.addbeh.2021.106978
27. Chen IH, Pakpour AH, Leung H, Potenza MN, Su JA, Lin CY, et al. Comparing generalized and specific problematic smartphone/internet use: longitudinal relationships between smartphone application-based addiction and social media addiction and psychological distress. J Behav Addict. (2020) 9:410–9. doi: 10.1556/2006.2020.00023
28. Kwon M, Kim DJ, Cho H, Yang S. The smartphone addiction scale: development and validation of a short version for adolescents. PloS ONE. (2013) 8:e83558. doi: 10.1371/journal.pone.0083558
29. Mingqiang X, Zirong W, Ben M. Reliability and validity of chinese version of the smartphone addiction scale in adolescents (In Chinese). Chin J Clin Psychol. (2019) 27:959–64. doi: 10.16128/j.cnki.1005-3611.2019.05.022
30. Zung WW. A self-rating depression scale. Arch Gen Psychiatry. (1965) 12:63–70. doi: 10.1001/archpsyc.1965.01720310065008
31. Dunstan DA, Scott N, Todd AK. Screening for anxiety and depression: reassessing the utility of the Zung scales. BMC Psychiatry. (2017) 17:329. doi: 10.1186/s12888-017-1489-6
32. Shu L. [Self-rating depression scale (SDS)]. In:Wang X, Wang X, Ma H, , editors. Rating Scales for Mental Health. Beijing: Chinese Mental Health Journal Publisher. (1999). p. 194–6.
33. Gong J, Chan RCK. Early maladaptive schemas as mediators between childhood maltreatment and later psychological distress among Chinese college students. Psychiatry Res. (2018) 259:493–500. doi: 10.1016/j.psychres.2017.11.019
34. Yu S, Sussman S. Does smartphone addiction fall on a continuum of addictive behaviors? Int J Environ Res Public Health. (2020) 17:422. doi: 10.3390/ijerph17020422
35. Panova T, Carbonell X. Is smartphone addiction really an addiction? J Behav Addict. (2018) 7:252–9. doi: 10.1556/2006.7.2018.49
36. Serra G, Scalzo LL, Giuffrè M, Ferrara P, Corsello G. Smartphone use and addiction during the coronavirus disease 2019 (COVID-19) pandemic: cohort study on 184 Italian children and adolescents. Ital J Pediatr. (2021) 47:150. doi: 10.1186/s13052-021-01102-8
37. Fung XCC, Siu AMH, Potenza MN, O'Brien KS, Latner JD, Chen CY, et al. Problematic use of internet-related activities and perceived weight stigma in schoolchildren: a longitudinal study across different epidemic periods of COVID-19 in China. Front Psychiatry. (2021) 12:675839. doi: 10.3389/fpsyt.2021.675839
38. Olvera C, Stebbins GT, Goetz CG, Kompoliti K. TikTok tics: a pandemic within a pandemic. Mov Disord Clin Pract. (2021) 8:1200–5. doi: 10.1002/mdc3.13316
39. Marengo D, Fabris MA, Longobardi C, Settanni M. Smartphone and social media use contributed to individual tendencies towards social media addiction in Italian adolescents during the COVID-19 pandemic. Addict Behav. (2022) 126:107204. doi: 10.1016/j.addbeh.2021.107204
40. Carbonell X, Chamarro A, Oberst U, Rodrigo B, Prades M. Problematic use of the internet and smartphones in University Students: 2006-2017. Int J Environ Res Public Health. (2018) 15:475. doi: 10.3390/ijerph15030475
41. Garrote GPd, Rubio L, Gómez BM, Buedo-Guirado C. Smartphone abuse amongst adolescents: the role of impulsivity and sensation seeking. Front Psychol. (2021) 12:746626. doi: 10.3389/fpsyg.2021.746626
42. Wu J, Siu ACK. Problematic mobile phone use by Hong Kong adolescents. Front Psychol. (2020) 11:551804. doi: 10.3389/fpsyg.2020.551804
43. Park J, Jeong JE, Rho MJ. Predictors of habitual and addictive smartphone behavior in problematic smartphone use. Psychiatry Investig. (2021) 18:118–25. doi: 10.30773/pi.2020.0288
44. Dhamija S, Shailaja B, Chaudhari B, Chaudhury S, Saldanha D. Prevalence of smartphone addiction and its relation with sleep disturbance and low self- esteem among medical college students. Ind Psychiatry J. (2021) 30:S189–94. doi: 10.4103/0972-6748.328813
45. Chen B, Liu F, Ding S, Ying X, Wang L, Wen Y. Gender differences in factors associated with smartphone addiction: a cross-sectional study among medical college students. BMC Psychiatry. (2017) 17:341. doi: 10.1186/s12888-017-1503-z
46. Okasha T, Saad A, Ibrahim I, Elhabiby M, Khalil S, Morsy M. Prevalence of smartphone addiction and its correlates in a sample of Egyptian university students. Int J Soc Psychiatry. (2022) 68:1580–8. doi: 10.1177/00207640211042917
47. Chan V, Moore J, Derenne J, Fuchs DC. Transitional age youth and college mental health. Child Adolesc Psychiatr Clin N Am. (2019) 28:363–75. doi: 10.1016/j.chc.2019.02.008
48. Liu Y, Zhang N, Bao G, Huang Y, Ji B, Wu Y, et al. Predictors of depressive symptoms in college students: a systematic review and meta-analysis of cohort studies. J Affect Disord. (2019) 244:196–208. doi: 10.1016/j.jad.2018.10.084
49. Sun XJ, Niu GF, You ZQ, Zhou ZK, Tang Y. Gender, negative life events and coping on different stages of depression severity: a cross-sectional study among Chinese university students. J Affect Disord. (2017) 209:177–81. doi: 10.1016/j.jad.2016.11.025
50. Song Y, Liu Z, Chen H, Guo Q, Huang Y. Incidence and risk factors of depressive symptoms in Chinese college students. Neuropsychiatr Dis Treat. (2020) 16:2449–57. doi: 10.2147/NDT.S264775
51. West R, Ash C, Dapore A, Kirby B, Malley K, Zhu S. Problematic smartphone use: The role of reward processing, depressive symptoms and self-control. Addict Behav. (2021) 122:107015. doi: 10.1016/j.addbeh.2021.107015
52. Haskell AM, Britton PC, Servatius RJ. Toward an assessment of escape/avoidance coping in depression. Behav Brain Res. (2020) 381:112363. doi: 10.1016/j.bbr.2019.112363
53. Yuan Y, Jiang S, Yan S, Chen L, Zhang M, Zhang J, et al. The relationship between depression and social avoidance of college students: a moderated mediation model. J Affect Disord. (2022) 300:249–54. doi: 10.1016/j.jad.2021.12.119
54. Jeri-Yabar A, Sanchez-Carbonel A, Tito K., Ramirez-delCastillo J, Torres-Alcantara A, Denegri D, et al. Association between social media use (Twitter, Instagram, Facebook) and depressive symptoms: Are Twitter users at higher risk? Int J Soc Psychiatry. (2019) 65:14–9. doi: 10.1177/0020764018814270
55. Elhai JD, Yang H, Montag C. Cognitive- and emotion-related dysfunctional coping processes: transdiagnostic mechanisms explaining depression and anxiety's relations with problematic smartphone use. Curr Addict Rep. (2019) 6:410–7. doi: 10.1007/s40429-019-00260-4
56. Kang Y, Liu S, Yang L, Xu B, Lin L, Xie L, et al. Testing the bidirectional associations of mobile phone addiction behaviors with mental distress, sleep disturbances, and sleep patterns: a one-year prospective study among Chinese college students. Front Psychiatry. (2020) 11:634. doi: 10.3389/fpsyt.2020.00634
57. Jun S. Longitudinal influences of depressive moods on problematic mobile phone use and negative school outcomes among Korean adolescents. Sch Psychol Int. (2019) 40:294–308. doi: 10.1177/0143034319830452
58. Coviello L, Sohn Y, Kramer ADI, Marlow C, Franceschetti M, Christakis NA, et al. Detecting emotional contagion in massive social networks. PLoS ONE. (2014) 9:e90315. doi: 10.1371/journal.pone.0090315
59. Pantic I, Damjanovic A, Todorovic J, Topalovic D, Bojovic-Jovic D, Ristic S, et al. Association between online social networking and depression in high school students: behavioral physiology viewpoint. Psychiatr Danub. (2012) 24:90–3.
60. Liu M, Lu C. Mobile phone addiction and depressive symptoms among Chinese University students: the mediating role of sleep disturbances and the moderating role of gender. Front Public Health. (2022) 10:965135. doi: 10.3389/fpubh.2022.965135
61. Park SY, et al. Long-term symptoms of mobile phone use on mobile phone addiction and depression among Korean adolescents. Int J Environ Res Public Health. (2019) 16:3584. doi: 10.3390/ijerph16193584
62. Liu XQ, Guo YX, Zhang WJ, Gao WJ. Influencing factors, prediction and prevention of depression in college students: a literature review. World J Psychiatry. (2022) 12:860–73. doi: 10.5498/wjp.v12.i7.860
63. Li J, Liu Y, Song J. The relationship between gender self-stereotyping and life satisfaction: the mediation role of relational self-esteem and personal self-esteem. Front Psychol. (2021) 12:769459. doi: 10.3389/fpsyg.2021.769459
64. Graves BS, Hall ME, Dias-Karch C, Haischer MH, Apter C. Gender differences in perceived stress and coping among college students. PLoS ONE. (2021) 16:e0255634. doi: 10.1371/journal.pone.0255634
65. Rollero C, De Piccoli N. Self-objectification and personal values. An exploratory study. Front Psychol. (2017) 8:1055. doi: 10.3389/fpsyg.2017.01055
66. Shulla RM, Toomey RB. Sex differences in behavioral and psychological expression of grief during adolescence: a meta-analysis. J Adolesc. (2018) 65:219–27. doi: 10.1016/j.adolescence.2018.04.001
67. Tang WDai Q. Depressive symptoms among first-year Chinese undergraduates: The roles of socio-demographics, coping style, and social support. Psychiatry Res. (2018) 270:89–96. doi: 10.1016/j.psychres.2018.09.027
68. Wu X, Tao S, Zhang Y, Li S, Ma L, Yu Y, et al. Geographic distribution of mental health problems among Chinese college students during the COVID-19 pandemic: nationwide, web-based survey study. J Med Internet Res. (2021) 23:e23126. doi: 10.2196/23126
Keywords: smartphone addiction, depression, college students, longitudinal study, cross-lagged panel model (CLPM)
Citation: Zhang K, Guo H, Wang T, Zhang J, Yuan G, Ren J, Zhang X, Yang H, Lu X, Zhu Z, Du J, Shi H, Jin G, Hao J, Sun Y, Su P and Zhang Z (2023) A bidirectional association between smartphone addiction and depression among college students: A cross-lagged panel model. Front. Public Health 11:1083856. doi: 10.3389/fpubh.2023.1083856
Received: 29 October 2022; Accepted: 05 January 2023;
Published: 24 January 2023.
Edited by:
Peige Song, Zhejiang University, ChinaReviewed by:
Xiaoyuan Chu, Beijing University of Posts and Telecommunications (BUPT), ChinaHiroyuki Enoki, Hiroshima International University, Japan
Copyright © 2023 Zhang, Guo, Wang, Zhang, Yuan, Ren, Zhang, Yang, Lu, Zhu, Du, Shi, Jin, Hao, Sun, Su and Zhang. This is an open-access article distributed under the terms of the Creative Commons Attribution License (CC BY). The use, distribution or reproduction in other forums is permitted, provided the original author(s) and the copyright owner(s) are credited and that the original publication in this journal is cited, in accordance with accepted academic practice. No use, distribution or reproduction is permitted which does not comply with these terms.
*Correspondence: Zhihua Zhang, zhangzh@ahmu.edu.cn
†These authors have contributed equally to this work