- 1Translational Sciences Section, Jonsson Comprehensive Cancer Center, School of Nursing, University of California, Los Angeles, Los Angeles, CA, United States
- 2Division of Biostatistics, Department of Preventive Medicine, Keck School of Medicine, University of Southern California, Los Angeles, CA, United States
- 3Center for Genetic Epidemiology, Keck School of Medicine, University of Southern California, Los Angeles, CA, United States
- 4Norris Comprehensive Cancer Center, University of Southern California, Los Angeles, CA, United States
- 5Department of Epidemiology, Fielding School of Public Health, University of California, Los Angeles, Los Angeles, CA, United States
- 6Center for Human Nutrition, David Geffen School of Medicine, University of California, Los Angeles, Los Angeles, CA, United States
Background: Circulating glycemic traits (GTs) have been considered a risk factor for breast cancer, but studies using GT-associated genetic variants as an instrumental variable are limited and inconclusive.
Methods: Our Mendelian Randomization analysis used the most recent genome-wide datasets focusing on European women.
Results: Of 44 single-nucleotide polymorphisms (SNPs) with GTs, 38 fasting-glucose and 6 fasting-insulin SNPs showed heterogeneous associations with breast cancer, without significant directional pleiotropy observed.
Conclusion: Our findings indicate a null association between genetically determined GTs and breast cancer risk among European women. Our findings may contribute to more complete characterizing of metabolic pathways in GTs and breast cancer.
Introduction
Previous studies for circulating glycemic traits (GTs), including fasting glucose (FG) and insulin (FI) concentrations, have shown inconsistent associations with breast cancer development (Gunter et al., 2009; Sieri et al., 2012; Boyle et al., 2013; Hernandez et al., 2014). This is partially due to selection bias, confounding, short time exposure to such metabolic biomarkers, measurement errors, and reverse causation. We tried to address those challenges by using a 2-sample Mendelian Randomization (MR) approach and examined whether genetically determined GTs are causally associated with breast cancer risk. The MR method may provide a relatively unbiased causal relationship between phenotype and cancer outcome because it reduces potential bias and confounding and prevents reverse causation by the random assortment of alleles at meiosis, resulting in random assignment of exposure, which precedes the phenotype and clinical outcomes (Merino et al., 2017).
Materials and Methods
For the GT instrumental variables, we used the recently updated publicly available data in 2019 from genome-wide association studies (GWASs) of the Meta-Analysis of Glucose and Insulin-related traits Consortium (MAGIC) in non-diabetic European women1. Detailed rationale and design of the studies have been described elsewhere (Scott et al., 2012). For breast cancer outcomes, we pulled 4 datasets from 2 independent consortia: Breast Cancer Association Consortium (BCAC2): (i) OncoArray; the Atlas of GWAS Summary Statistics (ATLAS3): (ii) CGEMS Breast Cancer GWAS, (iii) GEE adjusted for age, and (iv) GEE for age and body mass index. Study participants from each dataset provided written informed consent. Genetic instruments for each dataset were single-nucleotide polymorphisms (SNPs) associated with the trait at the genome-wide level (p < 5E-08).
We performed MR analysis using the inverse-variance weighted method (Burgess et al., 2013) which quantifies the genetically determined association between GTs and breast cancer risk. We assume that summarized data are available for multiple genetic variants in relation to the risk factor of interest X and the outcome Y; genetic variant k, k = 1,…, K is associated with an observed Xk mean change in the risk factor per additional variant allele with standard error σXk and an observed Yk changes in the log-odds or the log-probability of an outcome per allele with standard error σYk. The inverse-variance weighted estimates combine the ratio estimates from each variant in a fixed-effect meta-analysis model:
The approximate standard error of the estimate is:
The results were reported as risk ratios and 95% confidence intervals for the change in breast cancer risk per unit increase in FG (mmol/L) or natural log-transformed FI (pmol/L). To determine the extent of pleiotropic signal, we applied Cochran’s Q test and the MR-Egger analysis. Given obesity and diabetes’s established role for breast cancer, we excluded those relevant SNPs from the analysis. R3.6.1 was used. The Institutional Review Board of the University of California, Los Angeles, approved this study.
Results and Discussion
Of 430 GWA-based SNPs related to GTs in MAGIC, 44 SNPs within linkage disequilibrium (r2 < 0.1) were matched to either BCAC or ATLAS datasets (Table 1). The 38 FG-SNPs overall and stratified by cancer data source showed heterogeneous results but mostly showed a slightly increased effect on breast cancer risk without reaching statistical significance (Table 2 and Figure 1). After excluding top GWA-SNPs associated with type 2 diabetes and visceral obesity, the directions of the associations between GTs and breast cancer were changed in OncoArray but not in ATLAS-CGEMS. The 6 FI-SNPs showed similar patterns for the associations with breast cancer. No significant directional pleiotropy was observed (Table 3).
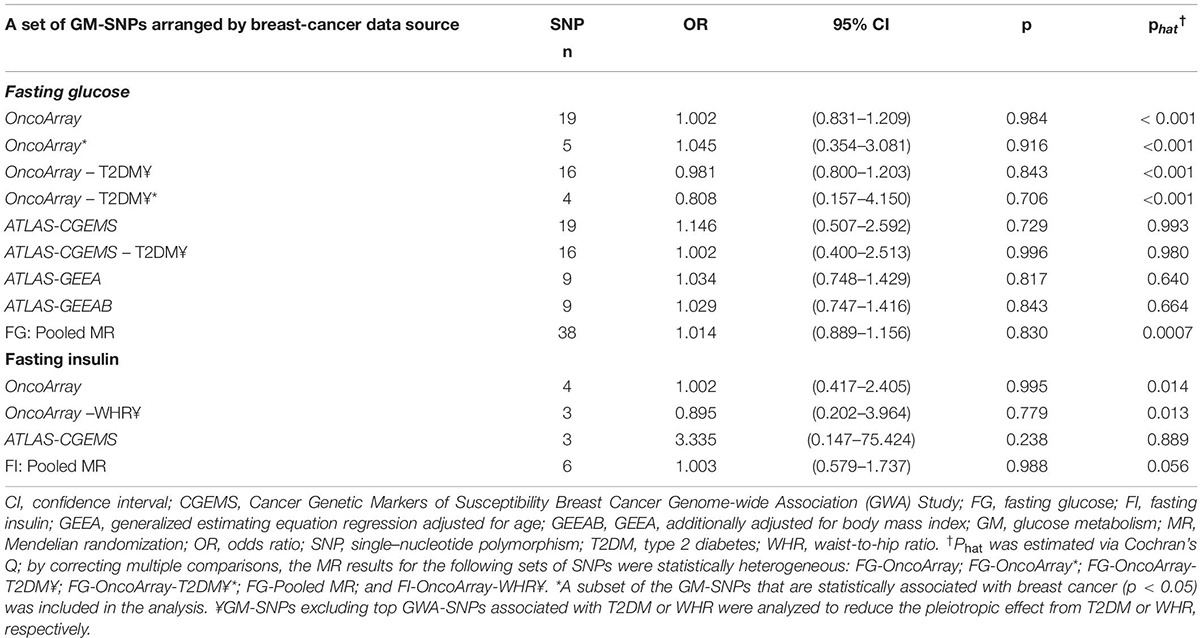
Table 2. Mendelian randomization analysis for the effect of genetically determined glucose-metabolism phenotypes on risk for breast cancer.
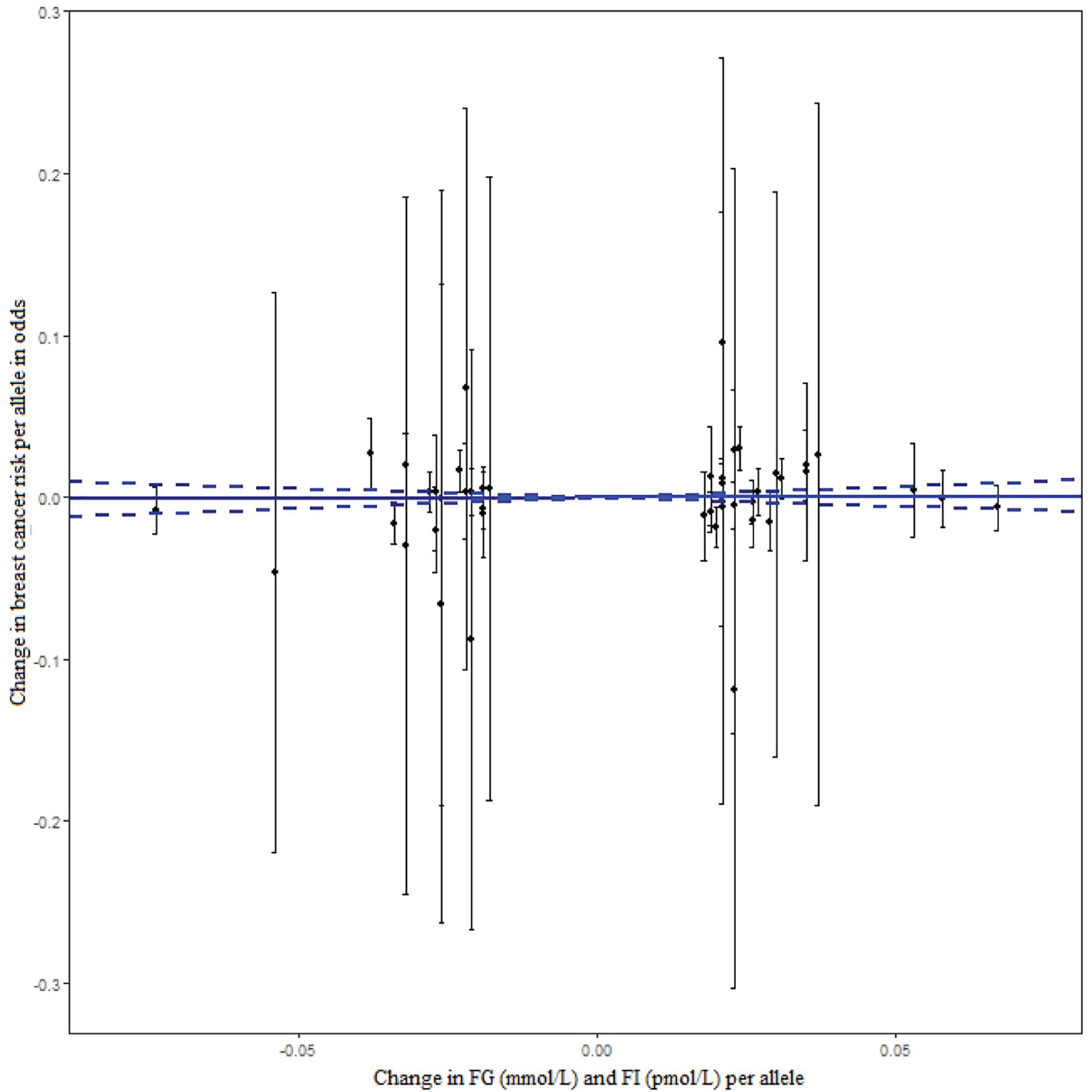
Figure 1. The effect of individual genetic instrumental variables for GTs on breast cancer risk. Each black dot represents a genome-wide GT-associated genetic variant. The blue lines indicate regression and 95% CIs of GTs on breast cancer risk (OR = 1.014, 95% CI: 0.895–1.147). CI, confidence interval; FG, fasting glucose; FI, fasting insulin; OR, odds ratio; GTs, glycemic traits.
We analyzed the relatively large and most-updated GWA-datasets for causality between GTs and breast cancer. Given that associations between metabolic markers and breast cancer risk can differ by menopausal status, our findings may be confounded. However, data was not available on the menopausal status, thus warranting future studies that account for this difference. In addition, whereas MR is considered a conservative approach, it may be confounded when modeled SNPs independently affect breast cancer risk through intermediate traits other than GTs.
Our study results should be interpreted with caution because of population structure bias (i.e., results biased due to tagged environmental factors) and unmeasured confounding factors that could have introduced bias. MR analysis might also be subject to non-linearity between exposure and outcome, but potential violation of the linearity assumption tends to bias MR estimates toward the null, rather than generating a spurious association (Smith and Ebrahim, 2003). Moreover, our study may not be generalized to other races or ethnicity, in which the association between genetic instruments, GTs, and breast cancer risk may be different.
Our findings indicate a null association between genetically determined GTs and breast cancer risk among European women. Our study may contribute to more complete characterizing of molecular pathways in GTs and breast cancer. It also highlights the need to conduct a more comprehensive and individual-level analysis using more detailed trait information, including risk causing confusion in this field of research.
Data Availability Statement
The datasets generated for this study are available on request to the corresponding author.
Author Contributions
SJ, NM, SH, and Z-FZ designed the study. SJ and SH performed the genomic data QC and statistical analysis and interpreted the data. NM and Z-FZ supervised the genomic data QC and analysis and participated in the study coordination and interpreted the data. SJ secured funding for this project. All authors participated in the manuscript writing and editing, read and approved the submission of the manuscript.
Funding
This study was supported by the National Institute of Nursing Research of the National Institutes of Health under Award Number K01NR017852 and a University of California Cancer Research Coordinating Committee grant (CRN-18-522722).
Conflict of Interest
The authors declare that the research was conducted in the absence of any commercial or financial relationships that could be construed as a potential conflict of interest.
Footnotes
- ^ https://www.magicinvestigators.org/
- ^ http://bcac.ccge.medschl.cam.ac.uk/bcacdata
- ^ https://atlas.ctglab.nl
References
Boyle, P., Koechlin, A., Pizot, C., Boniol, M., Robertson, C., Mullie, P., et al. (2013). Blood glucose concentrations and breast cancer risk in women without diabetes: a meta-analysis. Eur. J. Nutr. 52, 1533–1540. doi: 10.1007/s00394-012-0460-z
Burgess, S., Butterworth, A., and Thompson, S. G. (2013). Mendelian randomization analysis with multiple genetic variants using summarized data. Genet Epidemiol. 37, 658–665. doi: 10.1002/gepi.21758
Gunter, M. J., Hoover, D. R., Yu, H., Wassertheil-Smoller, S., Rohan, T. E., Manson, J. E., et al. (2009). Insulin, insulin-like growth factor-I, and risk of breast cancer in postmenopausal women. J. Natl. Cancer Inst. 101, 48–60. doi: 10.1093/jnci/djn415
Hernandez, A. V., Guarnizo, M., Miranda, Y., Pasupuleti, V., Deshpande, A., Paico, S., et al. (2014). Association between insulin resistance and breast carcinoma: a systematic review and meta-analysis. PLoS One 9:e99317. doi: 10.1371/journal.pone.0099317
Merino, J., Leong, A., Posner, D. C., Porneala, B., Masana, L., Dupuis, J., et al. (2017). Genetically driven hyperglycemia increases risk of coronary artery disease separately from type 2 diabetes. Diabetes Care 40, 687–693. doi: 10.2337/dc16-2625
Scott, R. A., Lagou, V., Welch, R. P., Wheeler, E., Montasser, M. E., Luan, J., et al. (2012). Large-scale association analyses identify new loci influencing glycemic traits and provide insight into the underlying biological pathways. Nat. Genet. 44, 991–1005. doi: 10.1038/ng.2385
Sieri, S., Muti, P., Claudia, A., Berrino, F., Pala, V., Grioni, S., et al. (2012). Prospective study on the role of glucose metabolism in breast cancer occurrence. Int. J. Cancer 130, 921–929. doi: 10.1002/ijc.26071
Keywords: genetically determined glucose and insulin, breast cancer, Mendelian randomization, obesity, diabetes
Citation: Jung SY, Mancuso N, Han S and Zhang Z-F (2020) The Role of Genetically Determined Glycemic Traits in Breast Cancer: A Mendelian Randomization Study. Front. Genet. 11:540724. doi: 10.3389/fgene.2020.540724
Received: 05 March 2020; Accepted: 18 August 2020;
Published: 16 September 2020.
Edited by:
Cheryl Ann Winkler, Frederick National Laboratory for Cancer Research (NIH), United StatesReviewed by:
Jing Dong, Baylor College of Medicine, United StatesSarah Buxbaum, Jackson State University, United States
Copyright © 2020 Jung, Mancuso, Han and Zhang. This is an open-access article distributed under the terms of the Creative Commons Attribution License (CC BY). The use, distribution or reproduction in other forums is permitted, provided the original author(s) and the copyright owner(s) are credited and that the original publication in this journal is cited, in accordance with accepted academic practice. No use, distribution or reproduction is permitted which does not comply with these terms.
*Correspondence: Su Yon Jung, sjung@sonnet.ucla.edu; suyonj@gmail.com