The Metabolic Response to Infection With Wolbachia Implicates the Insulin/Insulin-Like-Growth Factor and Hypoxia Signaling Pathways in Drosophila melanogaster
- 1Center for Advanced Imaging, The University of Queensland, Brisbane, QLD, Australia
- 2Institute of Vector Borne Disease, Monash University, Monash, VIC, Australia
- 3School of Environment and Science, Griffith University, Nathan, QLD, Australia
- 4Environmental Futures Research Institute, Griffith University, Nathan, QLD, Australia
The endosymbiotic bacteria, Wolbachia, are best known for their ability to manipulate insect-host reproduction systems that enhance their vertical transmission within host populations. Increasingly, Wolbachia have been shown to depend on their hosts' metabolism for survival and in turn provision metabolites to their host. Wolbachia depends completely on the host for iron and as such iron has been speculated to be a fundamental aspect of Wolbachia-host interplay. However, the mechanisms by which dietary iron levels, Wolbachia, and its host interact remain to be elucidated. To understand the metabolic dependence of Wolbachia on its host, the possibility of metabolic provisioning and extraction, and the interplay with available dietary iron, we have used NMR-based metabolomics and compared metabolite profiles of Wolbachia-infected and uninfected Drosophila melanogaster flies raised on varying levels of dietary iron. We observed marked metabolite differences in the affected metabolite pathways between Wolbachia-infected and uninfected Drosophila, which were dependent on the dietary iron levels. Excess iron led to lipid accumulation, whereas iron deficiency led to changes in carbohydrate levels. This represents a major metabolic shift triggered by alterations in iron levels. Lipids, some amino acids, carboxylic acids, and nucleosides were the major metabolites altered by infection. The metabolic response to infection showed a reprogramming of the mitochondrial metabolism in the host. Based on these observations, we developed a physiological model which postulates that the host's insulin/insulin-like-growth factor pathway is depressed and the hypoxia signaling pathway is activated upon Wolbachia infection. This reprogramming leads to predominantly non-oxidative metabolism in the host, whereas Wolbachia maintains oxidative metabolism. Our data also support earlier predictions of the extraction of alanine from the host while provisioning riboflavin and ATP to the host.
1. Introduction
Wolbachia pipientis, a maternally-inherited endosymbiont of invertebrates are best known for their ability to manipulate insect-host reproduction systems that enhance their transmission within host populations. Increasingly Wolbachia, have been shown to affect other insect-host life history traits including reproduction, lifespan, behavior, protection against viral infections or in limited examples, metabolic provisioning and extraction.
Metabolic provisioning and extraction by Wolbachia has been well-characterized in strains that infect filarial nematodes, where they establish obligate mutalistic associations (Hoerauf et al., 1999; Langworthy et al., 2000; Foster et al., 2005; Darby et al., 2012; Godel et al., 2012; Gill et al., 2014). Genome sequencing of both the nematode and Wolbachia symbionts have shown that both partners are dependent upon the other to complete several metabolic pathways. For example, the filarial nematode Brugia malayi lacks complete biosynthetic pathways for riboflavin, heme, or flavin adenine dinucleotide and nucleotides, while its Wolbachia symbiont wBm encodes complete biosynthetic pathways (Foster et al., 2005). Conversely, wBm lacks complete biosynthetic pathways for a range of metobolites including biotin, coenzyme A, folate, lipoic acid, nicotinamide adenine dinucleotide, pyridoxal phosphate and ubiquinone, which it acquires from its nematode host (Foster et al., 2005). Therefore, as an obligate endosymbiont, Wolbachia is highly dependent on host-derived metabolites for survival, despite its potential to provision metabolites (Wu et al., 2004; Jiménez et al., 2019; Newton and Rice, 2020). Nevertheless, direct experimental evidence for metabolic provisioning and extraction by Wolbachia in insect hosts has been limited. The clearest example is that observed in the blood feeding bedbug Cimex lectularius which is provided riboflavin (Moriyama et al., 2015) and biotin (Nikoh et al., 2014) by Wolbachia. Removal of Wolbachia reduces growth rates and fecundity, but these fitness traits could be restored when riboflavin was supplemented (Moriyama et al., 2015).
Recent analysis of genome-scale metabolic models predict that most Wolbachia strains are dependent upon their host for several metabolites. For instance, wMel, a strain that naturally infects Drosophila melanogaster, is predicted to depend on its host for alanine, glycine and serine metabolism, as well as for biosynthesis of lipopolysaccharides, antibiotic precursors, and biotin (Jiménez et al., 2019; Newton and Rice, 2020). Moreover, Wolbachia completely depends on the host for iron (Gill et al., 2014; Jiménez et al., 2019). As iron levels are relatively low in the natural environment of Drosophila (Brownlie et al., 2009), both Wolbachia and the host compete for dietary iron (Newton and Rice, 2020). Thus, iron has been speculated to be a fundamental aspect of the interplay between Wolbachia and the host (Gill et al., 2014). Studies in wasps and flies have shown that Wolbachia directly influences host expression of two major iron-regulating proteins, ferritin and transferritin, in direct response to dietary iron (Kremer et al., 2009; Gill et al., 2014). In a related study, we showed that wMel provide mild or strong fecundity advantages to infected female D. melanogaster flies when subjected to diets with low or high iron, respectively, corresponding to iron-deficiency or -overload (Brownlie et al., 2009). However, the mechanisms by which these advantages were conferred and how iron levels in diet and Wolbachia interact are unknown.
Here we have used for the first time NMR-based metabolomics to characterize Wolbachia-host interactions. We compare metabolite profiles of Wolbachia-infected and uninfected D. melanogaster raised on varying levels of dietary iron to understand (1) the metabolic dependence of Wolbachia on its host, (2) the possibility of metabolic provisioning and extraction, and (3) the interplay with available dietary iron. We show that there are strong interactions between the levels of dietary iron and Wolbachia infection in Drosophila. High levels of dietary iron affect mostly lipid metabolism, whereas low dietary iron predominantly impinges on carbohydrate metabolism. Furthermore, we show that lipids, some amino acids, carboxylic acids, and nucleosides are all affected in Wolbachia-infected flies. Based on these observations, we have developed a physiological model that explains the mechanism behind these metabolic changes. We propose that a reprogramming of the mitochondrial metabolism occurs in Wolbachia-infected hosts, facilitated by the insulin/insulin-like growth factor and hypoxia signaling pathways. This reprogramming leads to predominately non-oxidative metabolism in the host, whereas Wolbachia maintains oxidative metabolism. Our work also relates how these underlying biochemical and regulatory pathways are involved in metabolic interactions between the symbiont and host.
2. Materials and Methods
2.1. Insects
The Drosophila melanogaster strain BNE was derived from field-caught female flies from Brisbane, Australia, and is described in detail elsewhere (Brownlie et al., 2009; Yamada et al., 2011). Two genetically paired fly lines, one infected by wMel the other Wolbachia-free, were maintained at ~25°C on a 12/12 h light/dark schedule throughout the study. Tetracycline treatments were performed as described previously (Hoffmann et al., 1986) to generate a genetically identical fly line that lacked the Wolbachia infection. To reconstitute gut flora standardized methods were used (Chrostek et al., 2013) and all experiments were conducted at a minimum of seven generations post tetracycline treatment. To minimize genetic drift between these fly lines, approximately every 10 generations reciprocal crosses (BNE-wMel Female × BNE Tet male; BNE Tet female × BNE-wMel Male) were performed using 1-week-old adults. Fly lines were reared on three types of diets.
Cornmeal fly diet was made from yellow corn meal medium (Sigma). As described in detail elsewhere (Brownlie et al., 2009), the amount of available dietary iron was reduced by substituting the water that was used to make up the medium with an aqueous extract of black tea (Camellia sinensis)—which reduces iron availability by chelating free iron—or increased by the addition of a FeCl3 solution to the cornmeal fly diet to a final concentration of 10 mM.
2.2. Elemental Analysis Content and Estimate of Wolbachia Infection Density
The total content of nine biologically relevant metals (manganese, iron, cobalt, nickel, copper, zinc, cadmium, lead, and arsenic) present in flies reared on each of the food types (listed above), was determined using inductively coupled plasma mass spectrometry (ICP-MS) at the Advanced Center for Isotope Research Excellence at The University of Queensland. The only metal responsive to diet was iron. Pools of ten 5-day old flies were used for each analysis (Wolbachia-infected and uninfected controls) and replicated 15 times. Comparisons of the average total iron levels across diet and infection status were made using two-way ANOVA (GraphPad Prism).
Wolbachia infection density in 5-day old flies reared on each food type were estimated using an established relative qPCR assay that compares the abundance of the single-copy Wolbachia ankyrin repeat gene WD0550 to that of the single-copy D. melanogaster gene Act88F (McMeniman et al., 2008). Briefly, ten adult flies reared on each food type were collected and genomic DNA isolated using a QIAGEN DNeasy Blood and Tissue Kit according to manufacturer instructions (QIAGEN, Doncaster, VIC) and target genes amplified using SYBR-Green pre-mix (Qiagen). Comparisons of the average relative density of Wolbachia were made using one-way ANOVA (GraphPad Prism).
2.3. Sample Preparation
A total of 20 five-day old mated female flies per sample were collected from each combination of strain (Wolbachia-infected, or uninfected controls) and diet (low-, standard-, or high-iron), snap frozen in liquid nitrogen and stored at −80°C. Table 1 lists the number of samples for each of these six groups. The frozen flies from each sample were funneled into pre-labeled microfuge tubes containing 400 μl of ice-cold acetonitrile (50%, v/v) and homogenized using silica beads in a tissue-lyser (QIAGEN) system. Samples were then centrifuged at 14,000 × g for 10 min at 4°C. The supernatant was transferred to another set of pre-labeled and pre-weighed microfuge tubes, and stored at −80°C until lyophilized.
The freeze-dried extracts were thawed and dissolved in 185 μL of 200 mM sodium phosphate buffer, pH 7.4, 10 μL 5 mM sodium 2,2-dimethyl-2-silapentane-5-sulfonate-d6 (DSS) in D2O as chemical shift reference, and 5 μL 5 mM difluorotrimethylsilanylphosphonic acid (DFTMP) in D2O as internal pH indicator, yielding a final sample volume of 200 μL with final concentrations of 250 μM DSS, 125 μM DFTMP, and 7.5% D2O. Samples were transferred into 3-mm NMR tubes for measurement. For sample numbers and group IDs see Table 1.
2.4. NMR Spectroscopy
1H NMR spectra were recorded on a Bruker AV900 NMR spectrometer (Bruker Biospin, Rheinstetten, Germany) operating at a 1H frequency of 900.13 MHz and equipped with a 5 mm self-shielded z-gradient triple resonance probe. For each sample a 1D NOESY spectrum was acquired at 298 K with the noesypr1d pulse sequence [(RD) – 90° – t1 – 90° – τm – 90° – acq] (Bruker Biospin pulse program library). The transmitter frequency was set to the frequency of the water signal, and water suppression was achieved by continuous wave irradiation during both the relaxation delay of 3.0 s and the mixing time (τm) of 100 ms. After 16 dummy scans, 256 transients were collected into 32,768 data points using a spectral width of 14 ppm, leading to a total experiment time of 18.9 min per spectrum. Samples were manually changed after each 1D spectrum, and spectra were recorded in four different datasets. All spectra were processed using TOPSPIN version 3.5 (Bruker Biospin, Rheinstetten, Germany). The free induction decays (FIDs) were multiplied by a sine bell window function shifted by π/2 along the direct dimension before Fourier transformation, manual phase and baseline correction. The resulting spectra were referenced to the DSS signal at δ = 0 ppm.
The assignment of peaks to specific metabolites (Supplementary Table 1) was performed with Chenomx NMR Suite, version 8.4 (Chenomx Inc., Edmonton, Canada) as well as by comparing spectra with the online databases Human Metabolome Database and Biological Magnetic Resonance Bank (Wishart et al., 2018, Ulrich et al., 2007). In addition, the assignment of metabolites to peaks in the 1D loadings plots of the multivariate statistics (see below), was aided by calculating the covariance matrix (STOCSY) (Cloarec et al., 2005a) at full spectral resolution—either over all NMR spectra, or only over the groups of spectra that were part of the respective multivariate model. This analysis also uncovered covariation between different individual metabolites.
Assignments were confirmed by 2-dimensional (2D) 1H–13C heteronuclear single quantum coherence (13C–HSQC) spectra measured on selected samples. The 2D NMR spectra were acquired on a Bruker Avance 900 spectrometer operating at a 1H frequency of 900.13 MHz, equipped with a 5 mm self-shielded z-gradient triple resonance cryogenic probe. In all 2D spectra, the 1H carrier frequency was positioned on the water resonance. The 13C–HSQC and experiments were performed with spectral widths of 14.03 ppm in the 1H dimension. The spectral width in the 13C dimension were 110 or 120 ppm, and the 13C carrier frequency was set at 45 or 50 ppm, respectively. A total of at least 128 increments with 128 transients were recorded into 4,096 data points in the direct dimension, and a relaxation delay of 1.1 s was used. GARP decoupling of the 13C channel was used during the acquisition time. The spectra were multiplied by a squared sine bell window function shifted by π/2 along the direct and indirect dimensions before two-dimensional Fourier transformation.
2.5. Data Pre-processing
To correct for pH and ionic strength-dependent shift variations of individual NMR signals, the 1H NMR spectra were aligned in specific segments with the icoshift algorithm (Savorani et al., 2010) in MATLAB (MathWorks, Massachusetts, U.S.A) and then data-reduced with an in-house MATLAB script to consecutive integral regions of 0.001 ppm width (“buckets”), covering the range of δ = 10.0–0.25 ppm. The chemical shift region at δ = 5.10–4.67 ppm was excluded to eliminate the effects of imperfect water suppression. For each spectrum, the resulting integral regions were normalized to the total intensity of the spectrum to correct for inter-sample differences in weight and dilution. Subsequently, the bucketed data matrices were imported into the SIMCA 16 software package (Sartorius Stedim AB, Umeå, Sweden) for multivariate statistical analysis.
2.6. Multivariate Statistical Analysis
The data matrix with the bucketed 1D spectra was Pareto scaled for the subsequent analysis. An initial principal components analysis (PCA) was performed to determine whether there were any sample outliers and to investigate inherent differences in the samples (Hotelling, 1933). Nine samples were considerably outside the tolerance range of three standard deviations of the average score values in at least two latent components. Three additional samples were outside the 99% Hotelling's T2 limit. All twelve samples were removed as outliers in the subsequent analysis.
To maximize the distinction between classes, a supervised orthogonal partial least-squares (OPLS) analysis was performed on all remaining spectra (M1) (Trygg and Wold, 2002). To characterize global differences as a result of infection or diet, three OPLS models were fitted, one comparing all infected to all uninfected samples, irrespective of diet (M2), and one model each comparing all low- vs. standard-iron and standard- vs. high-iron diet samples, respectively (M3, M4), irrespective of infection status. Finally, to compare systematic differences between pairs of sample groups in more detail, a series of pairwise OPLS models was subsequently fitted (M5-M13). Supervised multivariate methods need additional input data about the class membership of individual samples, which are provided in the form of a Y–table, against which the PLS or OPLS algorithm performs a regression of the X-matrix. In our study the group identity of the six sample groups (0–5) was used as the Y–table for M1, and M2-M13 used the Wolbachia infection status (0 or 1) or the type of diet (0, 1, or 2), as appropriate.
In SIMCA, the number of OPLS components (A) for all models was optimized by cross validation. R2X, R2Y, and Q2 were used to evaluate model quality. R2X and R2Y are the fraction of the sum of squares explained by the latent components in the model, representing the variance of the X and Y variables, respectively, and Q2 is the predictive ability parameter of the model, which is estimated by cross validation. All OPLS models were validated by CV-ANOVA (Eriksson et al., 2008). The figures of merit of all multivariate models are listed in Supplementary Table 2.
Scores plots and loadings plots were used to interpret the various OPLS models. Spectral features representing elevated metabolite levels were identified from bivariate 1D loadings plots, in which loadings coefficients p were plotted against the chemical shift values of their respective variables, and the correlation-scaled loadings coefficients |p(corr)| were superimposed on the loadings plot as a heatmap color scale (Cloarec et al., 2005b). These plots contain the same information as a traditional S-plot, which indicates which variables influence the model with high reliability and are of relevance in the search for significantly altered metabolites.
To obtain a list of significantly changing metabolites (Supplementary Table 3), we used a combination of the variable importance in projection (VIP) score, |p|, and |p(corr)| (Galindo-Prieto et al., 2014). Generally, variables with VIP scores greater than 1 are considered significant (Eriksson et al., 2013). This criterion was converted into cutoffs for |p| and |p(corr)| by inspecting the S-plots for variables with VIP values of >1. For all diet pairwise comparisons (Models M8-M13), cutoffs of |p| ≥ 0.015 and |p(corr)| ≥ 0.6 were defined. For all Wolbachia v. uninfected pairwise comparisons (Models M5-M7) a cutoff for |p| ≥ 0.015 and |p(corr)| ≥ 0.45 was defined. The respective cutoffs for the global comparisons (Models M2-M5) were |p| ≥ 0.015, and |p(corr)| ≥ 0.5 for the diet comparisons (Models M4-M5) and |p(corr)| ≥ 0.4 for the Wolbachia v. uninfected comparison (Model M2).
2.7. Univariate Statistical Analysis
The list of metabolites observed to change significantly in the multivariate analysis (Supplementary Table 4) was then further explored using univariate statistical analysis. Only metabolite signals with minimal peak overlap were used in this analysis (Supplementary Table 5). Metabolite signal intensity in each spectrum was calculated by peak integration of the spectral region(s) containing signals of the respective metabolite with an in-house MATLAB script on the non-normalized full resolution spectra. Subsequently, the signal intensities from each spectrum were averaged for each of the six experimental groups. The resulting average signal intensities for each metabolite are listed in Supplementary Tables 6, 7. In both tables, average signal intensities lower than the limit of quantification (LOQ) for the respective metabolite were labeled “ < LOQ” and omitted from further analysis. The LOQ for each metabolite was determined as follows. The noise level for each spectrum was calculated as the standard deviation of the baseline noise in the region between δ = 11−10 ppm. The noise levels for all spectra were then averaged to obtain the mean baseline noise level. This value is the error that is contributed by the baseline noise to the intensity of each data point of a spectrum. To obtain the total error contributed by the baseline noise to the integrated intensity of a metabolite, the average baseline noise level was multiplied by the number of data points in the integration regions for the particular metabolite (Supplementary Table 5). The LOQ was then conservatively defined as 5 times the resulting value, i.e., the threshold at which the baseline noise contributes more than 20% error to the intensity integral of a metabolite.
For each pairwise comparison the relative fold change in signal intensities was calculated as the ratio of the averaged signal intensities between the two groups (Supplementary Table 8). To determine significantly changing metabolites across each of the nine pairwise comparisons, the signal intensities of each metabolite (Supplementary Tables 6, 7) were subjected to Mann–Whitney U-tests. The Benjamini–Hochberg method for multiple testing correction was then applied with a false discovery rate of 0.05 on the obtained p values. Metabolite intensities with a p value less than the calculated critical value, Q, were deemed significantly altered in the respective comparison, and the Benjamini–Hochberg corrected p values are listed in Supplementary Table 9.
3. Results
3.1. Iron Content and Wolbachia Density Analysis
Genetically paired lines of Drosophila melanogaster (BNE) that differed by their Wolbachia (wMel) infection status were reared on one of three diets: a standard fly diet that contained black tea extract reducing the available dietary iron, standard fly diet, or a standard fly diet supplemented with additional iron (Table 1). The total content of iron, manganese, cobalt, nickel, copper, zinc, cadmium, lead and arsenic was determined using mass spectrometry in 5-day old flies. Only the total iron content was affected (Supplementary Figure 1), as had been observed previously (Brownlie et al., 2009). Furthermore, there were no significant differences in iron levels when comparing uninfected controls and Wolbachia-infected insects. To determine if altering dietary iron influenced Wolbachia densities within 5-day old flies, a standard quantitative-PCR assay was used (McMeniman et al., 2008). Wolbachia densities were equivalent in hosts reared on all diets (Supplementary Figure 2). Taken together, these two results mean that any metabolic changes we observe across the three different diets are directly related to the iron content in the insect.
3.2. Global Analysis
Multivariate statistical analysis (MVSA) was used to investigate whether there are any systematic differences between the metabolite profiles of the six experimental groups of insects. After identifying 12 outliers in a preliminary PCA, an OPLS model of all remaining samples (model M1) showed definitive differences in the metabolite profile between all six experimental groups, and that all groups can be clearly distinguished from each other in the 3D scores plot (Figure 1). Note that the relationship between the six experimental groups to each other is unconstrained in the OPLS model, and thus their spatial arrangement and the distances between the centers of the six sample groups (Table 2) reflect the biological effects that distinguish the groups from each other. Strikingly, the spatial arrangement of the six groups with respect to each other in the 3D scores plot (Figure 1) is such that none of the first three latent components (t[1], t[2], or t[3]) align directly with the two factors of the study—Wolbachia infection, or dietary iron levels. In fact, five latent components are needed to fully describe the spatial arrangement of all groups with respect to each other. This arrangement of the sample groups, and especially the fact that the six groups are arranged in a non-planar manner shows immediately that (a) there are prominent interactions between Wolbachia infection and dietary iron levels, and (b) the effects of dietary iron are non-linear.
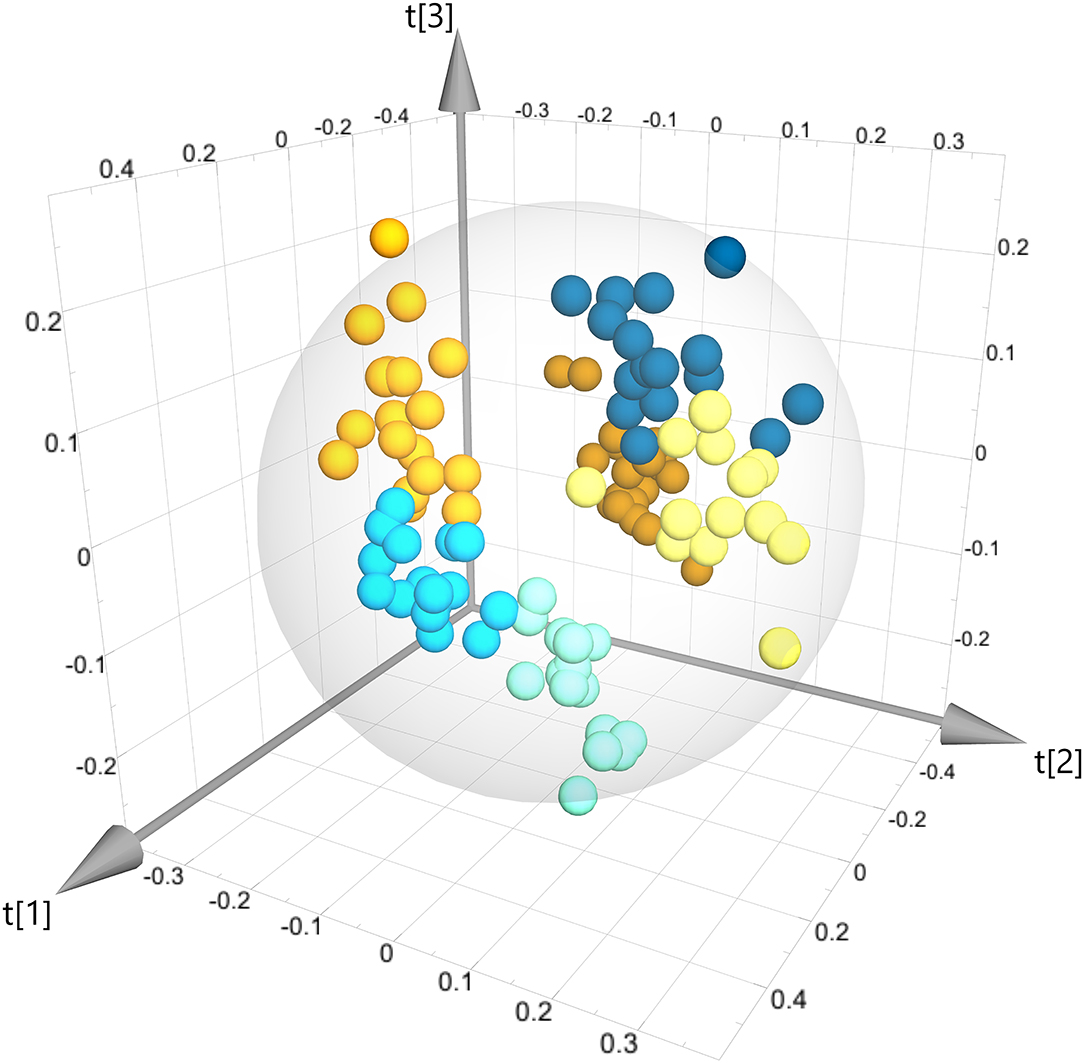
Figure 1. Three-dimensional OPLS scores plot of all sample groups (model M1). All six sample groups are clearly separated from each other. Each point represents one sample, and samples are colored according to their group identity. Dark orange: WH, orange: WS, yellow, WL, dark blue: TH, light blue: TS, teal: TL. This color scheme is maintained in all other scores plots of this paper.
The Euclidean distances between the six groups (Table 2) highlight that the effect of dietary iron levels is more pronounced in the Wolbachia-infected insects than in the uninfected controls. This bears witness to Wolbachia's dependency on the host for iron supply. The biological effect of Wolbachia infection is highly variable across the three different dietary conditions. It is the smallest in the context of the standard-iron diet. In the context of the high-iron diet the effect of infection is among the largest biological effects in the study. This highlights that the effects of infection are highly dependent on the diet context and that there are intricate interactions between infection and diet, which will complicate attempts to look at the two effects independently.
3.3. Overarching Effects of Wolbachia Infection or Diet
In a next step, we nevertheless attempted to characterize any overarching effects of Wolbachia infection or dietary iron levels in the study. We constructed three OPLS models that looked at each effect independently. Two models investigated the effects of diet, irrespective of infection (standard- vs. high-iron and low- vs. standard-iron diet), and the third model characterized the effects of infection, irrespective of diet. Clear group differences were noted in the scores plots of all three OPLS models (Supplementary Figure 3). In the corresponding loadings plots (Supplementary Figure 4), more dramatic global effects were seen for dietary iron levels than for Wolbachia infection.
Looking at the effect of diet first, the differences between the low- and standard-iron diet (Supplementary Figure 4B, model M4) are characterized by increased levels of complex carbohydrates in the standard-iron diet, and decreased levels of proline, glycine, and propionate. The differences between the standard- and the high-iron diet (Supplementary Figure 4A, model M3) are more severe, with the most noticeable changes being an increase in the levels of both saturated and unsaturated lipids, as well as increased levels of trehalose and tyrosine, and a decrease in the levels of maltose and complex carbohydrates, next to decreasing levels of inosine, guanosine, ortho-phosphocholine, choline, and dimethylamine.
Overall, the differences in dietary iron levels are predominantly reflected in alterations in carbohydrate and amino acid metabolism when comparing low- and standard-iron diets, but are dominated by lipids between standard- and high-iron diets. These overarching effects indicate that a metabolic switch occurs between carbohydrate and lipid metabolism when insects are subjected to iron deficiency vs. iron overload.
Interestingly, the levels of trehalose and lipids are strongly correlated with each other in this study. Thus, in a one-dimensional STOCSY plot the covariance between the anomeric signal of trehalose and all lipid signals is about 0.68, nearly as much as the covariance with the rest of the trehalose signals (0.7–0.8), which are heavily overlapped in the NMR spectra, leading to their correlation to the anomeric proton signal being statistically diluted by contributions from other metabolites. Maltose and complex carbohydrates show a biphasic non-linear behavior with respect to diet, as levels of maltose and complex carbohydrate are highest in the standard-iron diet and lower in both the low- and high-iron diet.
The overarching effects of Wolbachia infection were less pronounced. The metabolites that pass the significance filter (Supplementary Figure 4C, model M2) are generally only weakly correlated with the infection status of the insects—a stark contrast to the pronounced effects associated with dietary iron. Decreased levels of several nucleosides, glycerophosphocholine, asparagine and choline were observed in infected insects together with an increase in trehalose. These weak overarching effects of infection arise due to the strong interactions between Wolbachia infection and dietary iron, and thus no highly correlated overarching metabolite changes were observed when comparing all infected insects to all uninfected controls—a picture also reflected in the Euclidean distances of the global OPLS model.
3.4. Detailed Analysis of Infection and Diet Effects
Due to the intrinsic interplay between Wolbachia infection and dietary iron, it was necessary to examine the detailed effects of infection in the context of the three different diets, and at the differences between the three diets in the context of Wolbachia-infected insects and uninfected controls. Nine pairwise OPLS models (M5-M13) were subsequently fitted to investigate the effects of diet on infection status and vice versa (Supplementary Figure 5). Metabolites significantly changing in these nine pairwise models are listed in Supplementary Table 4. To complement the metabolite alterations identified in these multivariate models, univariate statistical analysis (UVSA) was also undertaken to analyse these metabolite changes semi-quantitatively and to calculate fold-changes and p-values for the significance of the fold-changes (Supplementary Tables 5–9).
3.4.1. Effects of Infection
As anticipated by the analysis so far, the metabolic effects of Wolbachia infection are indeed dramatically different in the context of the three iron diets (Figure 2). In the low-iron-diet (Figure 2C, model M7), Wolbachia infection leads to decreased levels of β-alanine, complex carbohydrates, and nucleosides such as inosine, IMP, guanosine and uridine. Conversely, Wolbachia-infected flies had increased levels of propionate, citrate, o-phosphocholine, tyrosine and adenosine.
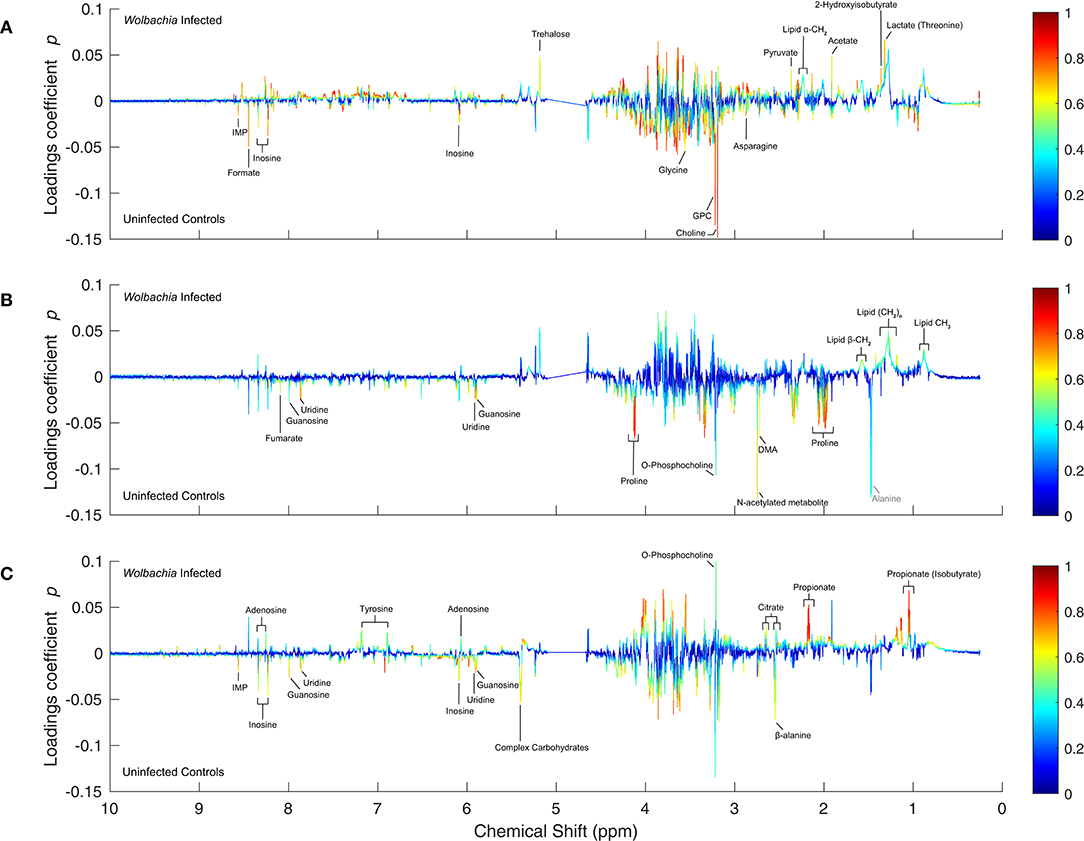
Figure 2. One-dimensional S-plots of the pairwise OPLS models comparing Wolbachia-infected insects with the uninfected controls. (A–C) For all panels, the loadings coefficients p were plotted against the chemical shift of the respective variables in the NMR spectrum, and the correlation-scaled loadings coefficients |p(corr)| were superimposed on the loadings plot as a heatmap color scale. The significantly altered metabolites (|p| ≥ 0.015 & |p(corr)| ≥ 0.45) are annotated in black in each panel: (A) High-iron diet (model M5), (B) Standard-iron diet (model M6), and (C) Low-iron diet (model M7). Gray annotations: metabolites referred to in the discussion.
Strikingly, the metabolic effects of infection in the context of the standard-iron diet are almost solely dominated by a decrease in proline levels (Figure 2B, model M6), which overshadows other less pronounced decreases in the levels of uridine, guanosine, DMA, furmarate, o-phosphocholine, and N-acetylated metabolites. Only lipids were noted to increase in infected flies. The fact that proline is dominating the bivariate loadings plots, suggests that this amino acid is a key part of the response to infection under standard iron-conditions. This observation also single-handedly explains why these sample groups are the two groups most similar to each other, as also demonstrated by their Euclidean distance in the global OPLS.
The metabolic consequences of infection are the most pronounced in the high-iron diet (Figure 2A, model M5), with the most notable effect being decreased levels in choline, GPC, glycine and formate, next to less pronounced decreases in inosine, IMP and asparagine. Levels of trehalose, pyruvate, acetate, lactate, lipids and 2-hydroxyisobutyrate were all increased in Wolbachia-infected flies. The changes in pyruvate, acetate and lactate indicate alterations to glycolysis and anaerobic cellular energy metabolism.
Because the metabolic effects of infection are highly dependent on the three different dietary iron levels, interpretation of the underlying pathways that are affected by infection requires careful consideration of the diet context.
3.4.2. Diet Effects in Uninfected Controls
In uninfected control insects, the differences between low- and standard-iron diet are relatively mild with none of the metabolites reaching high |p(corr)| values (Figure 3B, model M9). The most pronounced changes are increases in the levels of glucose, and o-phosphocholine in the standard-iron diet, and decreased levels of glycine, the branched-chain amino acids, proline, and lipids. These minor metabolite changes are likely indicating that Drosophila possesses robust mechanisms for adaptation to sub-optimal dietary iron levels.
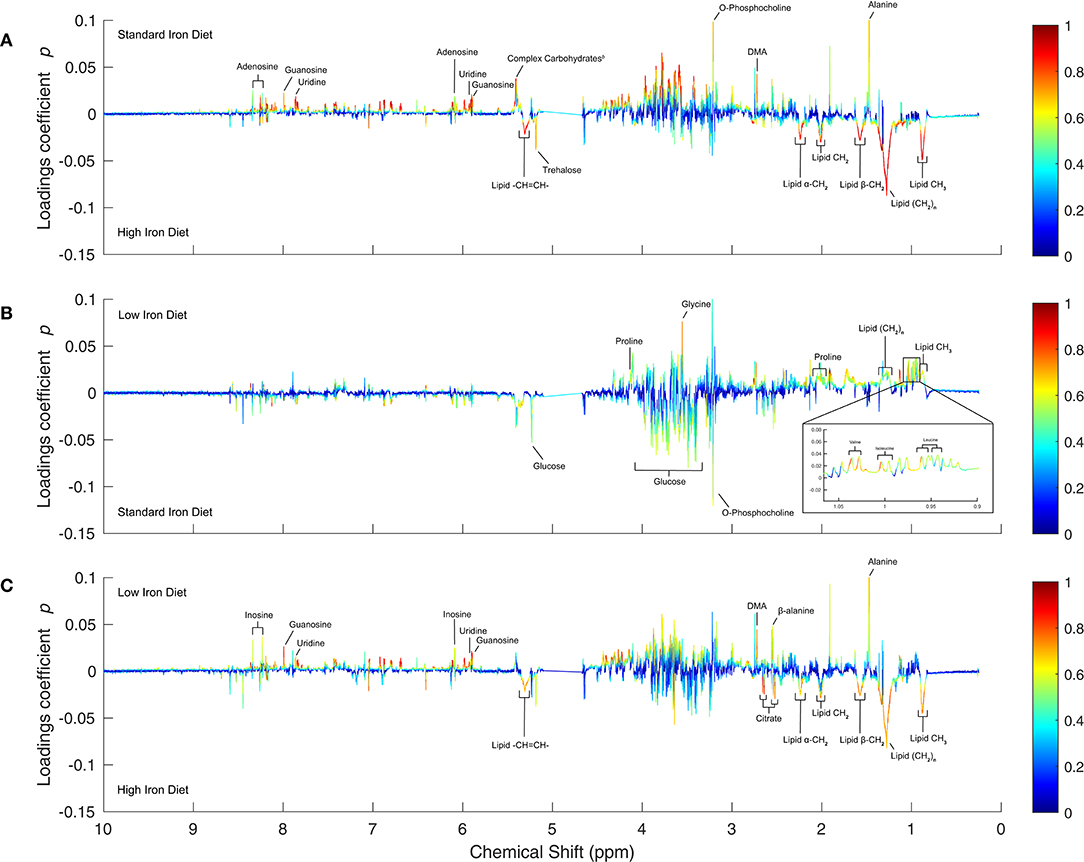
Figure 3. OPLS 1D S-Plots of the pairwise diet comparisons for uninfected controls. (A–C) The 1D S-plots are analogous to Figure 2, but show the diet comparisons for uninfected controls. The significantly altered metabolites (|p| ≥ 0.015 & |p(corr)| ≥ 0.6) are annotated in each panel: (A) High- v. standard-iron diet (model M8), (B) Standard- v. low-iron diet (model M9), and (C) High- v. low-iron diet (model M10).
In contrast, the metabolic differences between the standard- and high-iron diets (Figure 3A, model M8) are much more pronounced, and dominated by highly correlated increases in the levels of both saturated and unsaturated lipids, as well as trehalose in the high-iron diet. Levels of nucleosides, including adenosine, guanosine and uridine, are decreased in the high-iron diet, as are the levels of complex carbohydrates, o-phosphocholine, DMA, and alanine. The changes to fatty acids and carbohydrates suggest regulatory pathways that link carbohydrate and lipid metabolism upon iron overload.
The direct comparison of low- and high-iron diet (Figure 3C, model M10) shows essentially a superposition of the low- to standard- and standard- to high-iron diet comparisons, with a few alterations. The differences are also dominated by an increase in lipid levels, but this is not as pronounced as between standard- and high-iron diet, because lipid levels decreased between the low-and standard-iron diets. In addition, citrate levels are increased in the high-iron diet, whereas levels of guanosine and inosine are decreased, as are the levels of DMA, alanine and β-alanine.
In several NMR spectra, broad signals of lipids overlap with sharper signals of other metabolites, specifically lactate and proline. This offsets the peak loadings values [p and p(corr)] of those metabolites and means proper deconvolution is required to accurately quantify these metabolites. Nevertheless, because our bucket table has a resolution of 0.001 ppm, we were able to determine accurately that in the uninfected controls the levels of both lactate and proline decrease in the high-iron diet when compared to the standard- and low-iron diets (Figures 3A,C and Supplementary Figures 6A–D), despite the overlap from the broad signal of the lipid methyl and methylene protons, respectively.
In summary, the metabolic effects of dietary iron in the insect are more dramatic for iron overload, in contrast to the milder effects of iron-deficiency. This is perhaps unsurprising as flies in the wild have equivalent total iron levels to that of flies reared on low-iron conditions (Brownlie et al., 2009), and thus presumably have adapted to these conditions.
3.4.3. Diet Effects in Wolbachia-Infected Insects
In Wolbachia-infected Drosophila, the differences between low- and standard-iron diet (Figure 4B, model M12) comprise only a few metabolites, but their concentration changes are pronounced and highly correlated. The standard-iron diet is characterized by increased levels of maltose and complex carbohydrates compared to the low-iron diet, and decreased levels of proline, glycine and propionate. The prominent changes to maltose and complex oligosaccharides indicate the involvement of carbohydrate metabolism in the response to iron deficiency.
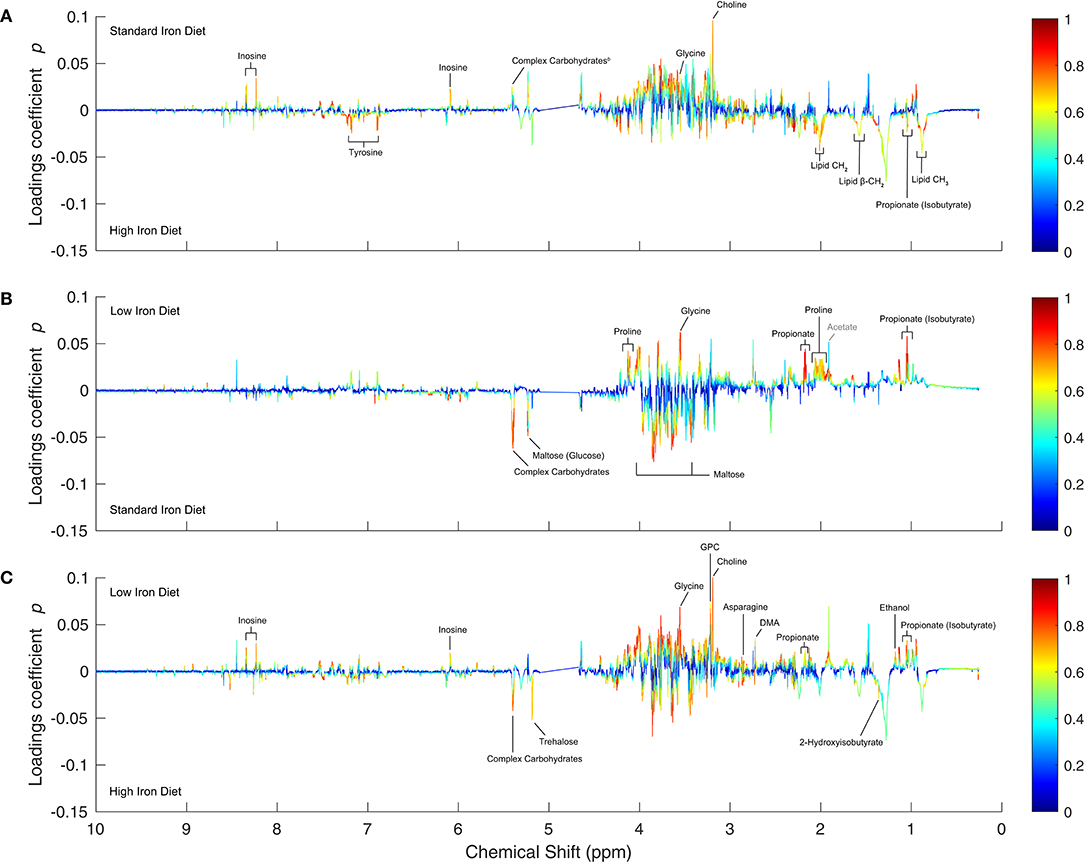
Figure 4. OPLS 1D S-Plots of the pairwise diet comparisons for Wolbachia-infected samples. (A–C) The 1D S-plots are analogous to Figure 2, but show the diet comparisons for Wolbachia-infected samples. The significantly altered metabolites (|p|≥ 0.015 & |p(corr)| ≥ 0.6) are annotated in black in each panel: (A) High- v. standard-iron diet (model M11), (B) Standard- v. low-iron diet (model M12), and (C) High- v. low-iron diet (model M13). Gray annotations: metabolites referred to in the discussion.
The differences between the standard- and high-iron diet (Figure 4A, model M11) comprise more metabolites, but not all metabolite changes are as highly correlated as between the low- and standard-iron diet. In the high-iron diet levels of tyrosine, propionate, and lipids are elevated, whereas the levels of complex carbohydrates, glycine, inosine, and choline are decreased. This metabolic profile difference is strikingly similar to that of the uninfected controls, meaning the regulatory mechanism upon iron overload are common to both groups and independent of infection status.
The comparison between low- and high-iron diet (Figure 4C, model M13) is dominated by increased levels of trehalose and complex carbohydrates in the high-iron diet, next to elevated levels of 2-hydroxyisobutyrate. Levels of glycine, asparagine, choline, GPC, propionate, DMA, ethanol, and inosine are decreased.
Similarly to the analysis of overlapping lipid and proline signals in the uninfected controls, we were able to determine that in the Wolbachia-infected insects proline levels are increased in the high-iron diet when compared to the standard-iron diet (Figure 4A and Supplementary Figure 6E), but decreased when compared to the low-iron diet (Figure 4C and Supplementary Figure 6F)—in accordance with the result showing strongly increased proline levels in the low- over the standard-iron diet (Figure 4B).
Overall, the effects of dietary iron are strongly pronounced in the Wolbachia-infected insects for both high and low levels of dietary iron, when compared to the uninfected insects.
4. Discussion
4.1. Global and Dietary Iron Effects
In this study we have characterized the metabolic changes as a result of Wolbachia infection in Drosophila melanogaster under different levels of dietary iron. The Euclidean distances between experimental groups in the global analysis already provide some insight into the relative biological effects. For example, the metabolic differences between infected insects and uninfected controls under standard iron conditions was the smallest distance in the whole study. This highlights that Wolbachia places only a minimal burden on Drosophila under standard-iron conditions, and reflects Wolbachia's adaptation as a mutualistic symbiont. Furthermore, the standard diet is nutritionally rich, and as such masks any obvious Wolbachia-host effects. In contrast, the high-iron diet is substantially different to the standard- and low-iron diets in both the uninfected controls and the Wolbachia-infected insects. This reflects the impost that iron levels in excess of the natural environment have on both groups. Of further interest are the distances between dietary conditions, which are always larger in the Wolbachia-infected groups when compared to the respective distance in the uninfected controls. This is especially evident under conditions of iron deficiency, as the distance between low- and standard-iron conditions in Wolbachia-infected insects is almost twice the corresponding distance for the uninfected controls. This bears witness to Wolbachia's dependency on the host for iron and the extra demands it makes on the host for iron supply.
The Euclidean distances are a result of marked metabolite profile differences between the six groups of insects in this study. In both Wolbachia-infected insects and uninfected controls, differences in dietary iron levels lead predominantly to alterations in carbohydrate and amino acid metabolism, between low- and standard-iron diets, but are dominated by lipids between standard- and high-iron diets. This indicates that a metabolic shift occurs between carbohydrate metabolism and fatty acid metabolism when comparing low- and high-iron levels, highlighting fundamental changes in metabolism. Interestingly, this metabolic shift is slightly different in Wolbachia-infected insects and uninfected controls. Across the board, the changes in carbohydrates and lipids are accompanied by slightly different sets of other metabolites. In addition, under iron deficiency the changes in carbohydrate metabolism are dominated by changes in glucose levels in the uninfected controls, whereas in the Wolbachia-infected insects levels of maltose and complex carbohydrates are affected.
When considering the biological and regulatory mechanisms that are likely to underpin the observed metabolic changes, it is worthwhile to keep in mind that metabolic effects that occur in both Wolbachia-infected insects and uninfected controls are likely to result from regulatory mechanisms in Drosophila itself. Examples of this are the strongly increased lipid levels and decreased levels of complex carbohydrates in the high-iron diet which are observed in both Wolbachia-infected insects and uninfected controls. Our observation is confirmed by other studies who have also observed lipid accumulation under high-iron conditions in a variety of organisms, including Drosophila (Navarro et al., 2010; Wang et al., 2016; Rockfield et al., 2018) and humans (Ahmed et al., 2012). Unsurprisingly, lipid and carbohydrate metabolism are intrinsically interlinked with the energy metabolism and mitochondrial function of the cell. A potential mechanism for this link between different parts of the metabolism is the outer mitochondrial membrane protein mitoNEET, which modulates mitochondrial function and iron content (Ferecatu et al., 2014). Upon iron excess, mitoNEET is upregulated leading to lipid uptake and storage within the host as well as to downregulated β-oxidation, and thus to increased adipose tissue mass (Kusminski et al., 2012). In addition, upregulated mitoNEET increases the rate of glycolysis (Kusminski et al., 2012; Wang et al., 2017), which could explain the lower levels of complex carbohydrates observed in our study. Furthermore, upregulated mitoNEET inhibits iron transport into the mitochondrial matrix, thus limiting both oxidative phosphorylation and ROS damage.
Under iron-deficiency conditions one might expect to see metabolic adaptations that lead to conservation of iron, iron scavenging, and a move to or reliance on metabolic pathways that do not require iron, such as glycolysis. Indeed, alterations to carbohydrate metabolism are among the most prominent metabolic changes we observed in both infected and uninfected insects under the low-iron diet. Interestingly, mitoNEET could also be involved in these metabolic alterations. Recently, a glycogen branching enzyme was established to be a key regulator of iron homeostasis under iron-depleted conditions in Drosophila. This enzyme controls cellular iron homeostasis via binding to the Iron-regulatory protein 1A (IRP1A) and mitoNEET (Huynh et al., 2019). This link between cellular iron homeostasis and glycogen metabolism may explain why carbohydrates change significantly upon iron deficiency. Additionally, the interaction between the glycogen-branching enzyme, mitoNEET, and IRP1A leads to the downregulation of iron-intensive processes as well as increasing the levels of bio-available iron for the cell (Huynh et al., 2019).
4.2. Effects of Wolbachia Infection
There are marked differences in the affected metabolic pathways between Wolbachia-infected insects and controls, which are also dependent on the iron levels in the diet. Indeed, when comparing the metabolite profiles of infected insects and uninfected controls, the major metabolites affected are carboxylic acids, nuclosides, cholines, and amino acids in the low- and high-iron diets. In contrast, in the standard-iron diet the major metabolic effect of infection is a decrease in proline levels. Taken together, these observations mean that the detected metabolic differences between the six study groups are driven by an interaction of both the Wolbachia symbiont and the iron levels inside the host cells. This highlights that signaling pathways and/or mechanisms that regulate iron homeostasis in the cell play a pivotal role in the interaction between Wolbachia and the host.
Earlier studies have shown that Wolbachia affects iron homeostasis of the host by regulating the expression of ferritin (Kremer et al., 2009; Gill et al., 2014). Iron homeostasis and ferritin expression are regulated in multiple organisms including D. melanogaster via the insulin/insulin-like-growth factor signaling (IIS) pathway and the hypoxia signaling pathway (Ackerman and Gems, 2012; Nässel et al., 2015; Altintas et al., 2016). Based on these studies and our results, we postulate that Wolbachia affects both of these pathways in the host. Previous studies have predicted that Wolbachia competes for iron as well as oxygen with the mitochondria of the host cell to run its own oxidative phosphorylation, leading to host cells becoming hypoxic in the presence of Wolbachia (Kremer et al., 2009; Gill et al., 2014; Dutra et al., 2017). Hypoxia depresses the IIS pathway (Wong et al., 2014; Texada et al., 2019; Barretto et al., 2020) and upregulates the hypoxia signaling pathway, as shown in Figure 5. The hypoxia signaling pathway, mediated by hypoxia-inducible factor 1 (HIF-1) (Mylonis et al., 2019), represses ferritin expression, leading to increased bioavaliability of iron in the cell (Kremer et al., 2009). A previous study has indeed shown reduced ferritin expression in Wolbachia-infected host cells (Kremer et al., 2009). Wolbachia competes for the intracellular iron to maintain oxidative phosphorylation, which will lead to the production of reactive-oxygen species (ROS) by the symbiont (Gill et al., 2014). In addition, the increased iron bioavailability in the host cells leads to secondary ROS production (Gill et al., 2014). In support of this interpretation, we have previously shown that ROS are being induced as a result of Wolbachia infection even in co-evolved hosts such as Drosophila (Wong et al., 2015). These metabolic effects observed here are likely to be driven by Wolbachia, modified by the context of the dietary iron levels, rather than being a primarily iron-driven response.
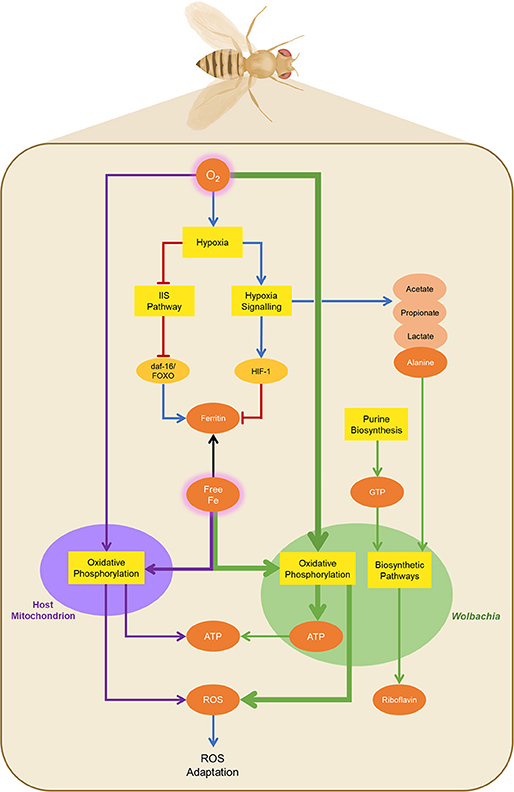
Figure 5. General regulatory and metabolic pathways involved in Wolbachia infection. The presence of Wolbachia (green oval) inside Drosophila cells (beige rectangle) leads to competition for oxygen, causing hypoxia in the host cells. Hypoxia leads to the downregulation of the insulin/insulin-like growth factor signaling (IIS) pathway and upregulation of the hypoxia signaling pathway, and subsequently to reprogramming of mitochondrial metabolism (purple oval). The hypoxia signaling pathway leads to increased bioavailability of free iron in the host cells, as iron sequestration by ferritin is reduced. Iron is redirected to Wolbachia, which relies on the host for iron supply, leading to ROS production as a consequence of oxidative phosphorylation in the symbiont (green arrows). ATP produced by Wolbachia via this route can be exported to the host. The increased bioavailability of iron also leads to ROS production in Drosophila (purple arrows). Both pathways of ROS production lead to the subsequent activation of ROS adaptation mechanisms. In contrast to the oxidative metabolism inside Wolbachia, the hypoxic metabolism in Drosophila leads to the production of end products, such as alanine, lactate, acetate, and propionate, of which alanine is provided to Wolbachia as a precursor for several biosynthetic pathways, as is GTP. Wolbachia also synthesizes riboflavin, which is provided to the host. Black Arrows: general metabolite flow. Purple and green arrows: metabolite flow to/from host mitochondria and Wolbachia, respectively. Blue arrows: Activatory regulation. Red arrows: Inhibitory regulation. Yellow boxes: biochemical modules. Beige ovals: general metabolites. Brown ovals: metabolites involved in provisioning/extraction with Wolbachia.
Interestingly, the ATP generated by Wolbachia for its own purposes can potentially be provided to the host (Darby et al., 2012; Gill et al., 2014). In accordance with that hypothesis we observed during Wolbachia infection at low-iron conditions a significant increase of adenosine levels, which are likely to be a proxy for the whole pool of adenosine, AMP, ADP, and ATP, due to our extraction procedure. The increase in adenosine levels we observed is arguably from the symbiont, because recent studies noted that Wolbachia infection in Drosophila yields significantly reduced ATP levels in the host (Carneiro Dutra et al., 2020, Carneiro Dutra et al., 2020).
On the other hand, depressing the IIS pathway leads to the induction of mechanisms that will modulate the increased ROS production induced by Wolbachia infection (Van Heemst, 2010). This aligns with an earlier hypothesis (Zug and Hammerstein, 2015) that in native hosts Wolbachia infection is associated with both increased ROS production and increased mechanisms of redox homeostasis and ROS protection. Firstly, the hypoxia-induced reduction of the IIS pathway leads via phosphoinositide 3-kinase (PI3K)/Akt signaling to an activation of the daf-16/FoxO transcription factor (Barretto et al., 2020), which mitigates oxidative stress resistance through regulating antioxidants (Mattila and Hietakangas, 2017) and increasing ferritin expression (Ackerman and Gems, 2012). The latter effect is likely to provide some counterbalance to the ferritin repression triggered by hypoxia and HIF-1. Secondly, proline catabolism is promoted, which regulates both ROS production and homeostasis (Zarse et al., 2012; Tang and Pang, 2016). In agreement with this interpretation, we observed significantly reduced proline levels in Wolbachia infected insects on the standard iron diet. However, this Wolbachia-related reduction in proline levels is diet dependent, as significant proline accumulation is promoted upon iron deficiency (Kitajima et al., 2003). Likewise, we observe in our data less pronounced changes in proline levels in low-iron conditions.
Reprogramming of lipid metabolism occurs under HIF-1 and insulin signaling. Upregulation of HIF-1 enhances lipogenesis through modulation of proteins involved in fatty acid uptake, synthesis, storage and usage, and diminishes fatty acid catabolism (Mylonis et al., 2019). Similarly, the disruption of the IIS pathway can also induce lipogenesis via the daf-16/FoxO transcription factors (Perez and Van Gilst, 2008). Subsequently, the fatty acids are converted into triacylglycerols and stored in lipid droplets (Mylonis et al., 2019). An increase in localized lipid droplets has indeed been noted as a result of Wolbachia infection (Geoghegan et al., 2017). Similarly, we observed increased lipid levels in Wolbachia-infected insects compared to the uninfected controls under standard and high-iron diet conditions.
When oxygen is sparse, HIF-1 upregulation leads to inhibition of the pyruvate dehydrogenase complex decreasing the flow of pyruvate into the TCA cycle. In uninfected Drosophila, this causes an accumulation of lactate, acetate and alanine as anaerobic end-products (Feala et al., 2007). Wolbachia extracts alanine from the host as precursor of several other metabolites, as it cannot synthesize alanine itself (Jiménez et al., 2019), thus redirecting metabolic flux away from lactate and acetate. Consistent with this interpretation, we observed reduced levels of alanine, acetate and lactate in Wolbachia-infected individuals under standard-iron conditions. Keeping host cells in a hypoxic state that favors the production of an essential metabolite for Wolbachia is a strategy that is advantageous for the bacterium.
Wolbachia is able to synthesize riboflavin and provision it to the host (Jiménez et al., 2019). Riboflavin supplementation significantly contributes to prolonged lifespan, growth and fecundity in both Cimex lectularius and Drosophila melanogaster (Moriyama et al., 2015; Zou et al., 2017)—effects that are similar to Wolbachia infection. In several bacterial species there is evidence for increased riboflavin biosynthesis overcoming iron-restrictive conditions (as reviewed in Sepúlveda Cisternas et al., 2018). The riboflavin biosynthesis pathway starts with GTP, which in turn is formed through the purine biosynthesis pathway from IMP. Thus, increased riboflavin production after Wolbachia infection would be associated with a decrease of IMP and GTP levels compared to the uninfected controls. Indeed, during Wolbachia infection in iron-deficient conditions we observed a significant reduction of both inosine/IMP and guanosine—supporting the hypothesis of riboflavin provisioning by Wolbachia.
Wolbachia infection under low-iron conditions leads to a significant increase of propionate levels with a smaller and correlated increase in acetate levels. This could arise from two potential metabolic pathways. The first possibility is malate dismutation, an ATP generating pathway known in helminths, and nematodes, including C. elegans in which the second half of the TCA cycle runs backwards under low-oxygen conditions, leading to the production of both propionate and acetate (Müller et al., 2012; Stairs et al., 2015). Although the malate dismutation pathway has not been actively studied in either Drosophila or Wolbachia yet, both organisms possess the majority of enzymes required for this pathway, including some enzymes whose presence only seems to make sense if the whole malate dismutation pathway is present. In agreement with this hypothesis, Drosophila are strongly hypoxia and anoxia tolerant (Krishnan et al., 1997; O'Farrell, 2001; Haddad, 2006). Moreover, depressing the IIS pathway in C. elegans leads to high propionate levels (Fuchs et al., 2010). Thus, malate dismutation is a possible contender for the observed propionate production. Secondly, Drosophila possess a direct metabolic pathway for production of propionate from pyruvate (Kanehisa and Goto, 2000), and thus the production of acetate and propionate could be a simple follow-on from anaerobic glycolysis. In both of these scenarios, the production of propionate and acetate is more likely to be an outcome of Drosophila metabolism relying on anaerobic pathways to compensate and accommodate the reduced availability of oxygen induced by Wolbachia infection.
It is interesting to note that the metabolic alterations as a consequence of Wolbachia infection described here are characteristic of metabolic reprogramming, rather than an immune response. A study by Wong et al. confirms this observation as they noted no measurably increased expression of immune genes in wMel-infected Drosophila (Wong et al., 2011). In other words, there is no priming of the immune system by wMel. In addition, we see no evidence for a loss of lipogenesis and glycogenesis in our study, which are metabolic markers for an immune response (Clark et al., 2013; Davoodi et al., 2019). However, we do observe an effect of diet on these pathways irrespective of infection status. Thus, the observed metabolic changes are a result of host-symbiont-environment interactions, rather than an immune response by the host. The above findings also fit with the general hypothesis by Zug and Hammerstein who postulated that strains of Wolbachia that have coevolved with their respective hosts do not stimulate an immune response (Zug and Hammerstein, 2015).
Previous studies that have compared host and Wolbachia genomes have predicted biochemical and physiological interactions unique to host and symbiont (Min and Benzer, 1997; Foster et al., 2005; Gill et al., 2014; Kosmidis et al., 2014; Newton and Rice, 2020). Indeed, in other host-Wolbachia pairs interactions range from facultative parasitism to obligate mutualism (Gill et al., 2014). Nevertheless, iron has been repeatedly shown to be of crucial importance for the interaction between Wolbachia and host (Gill et al., 2014), and thus the regulatory pathways implicated here are likely to have broader ramifications for Wolbachia-host pairings in general, or potentially even other endosymbiont-host interactions.
In summary, the presence of Wolbachia depresses the IIS cascade in D. melanogaster whilst inducing the hypoxia signaling pathway. This in turn causes both ROS production and ROS adaptations, as well as other metabolic changes, that steer metabolism away from oxygen-intensive pathways and enable metabolite extraction by the symbiont and metabolite provisioning to the host (Figure 5). Finally, this metabolic reprogramming is likely to be driven by Wolbachia, and modified by the dietary iron levels.
Earlier work has suggested that Wolbachia increases insulin signaling (Ikeya et al., 2009) rather than the downregulation inferred by our own work. However, these results were omitting important wild-type or Wolbachia-infected controls when assessing direct activation levels of Akt and FoxO, two of the key proteins in the IIS pathway. Furthermore, the authors' observation of an accumulation of triacylglycerides in the absence of Wolbachia is not supported by the experimental observations made by us—that lipid levels increase in the presence of Wolbachia. Increased IIS signaling in Wolbachia-infected Drosophila was also inferred by a recent study (Carneiro Dutra et al., 2020). However, that study used different strains of Wolbachia and Drosophila than those used by us, and reared flies on a carbohydrate-rich diet. Given the specificity outlined above of the metabolic interactions between strain, host and diet, any differences in results and interpretation are not surprising.
Our study has three limitations. Firstly, Wolbachia are known to establish high infection densities in the fat-body and adult brain of Drosophila (Albertson et al., 2013)—two tissues that are also known to be interlinked by the IIS pathway, supporting the likely involvement of this pathway in Wolbachia infection (Carneiro Dutra et al., 2020). However, because our experimental setup used the entire insect for NMR analysis, we cannot resolve any tissue-specific metabolic changes. Secondly, for the same reason, we can not directly distinguish metabolite changes associated with Wolbachia vs. the host. Lastly, we can only infer the regulatory pathways in our model from the metabolic changes observed here. Other proteins or signaling pathways also regulate iron, e.g., transferrin or iron-regulating proteins (IRPs) (Tang and Zhou, 2013; Cronin et al., 2019), but the pathways inferred here are supported by previous experimental observations (Kremer et al., 2009; Gill et al., 2014; Wong et al., 2014; Dutra et al., 2017; Texada et al., 2019; Barretto et al., 2020) and are thus the most probable explanation for our results. Future experiments aimed at direct manipulation of the IIS and hypoxia pathways will be able to confirm their involvement.
4.3. Future Directions
Apart from their direct manipulation as suggested above, the involvement of both the IIS and hypoxia signaling pathways as a consequence of Wolbachia infection opens up several exciting avenues for further discovery. Given the strain-host specificity discussed above, it would be interesting to apply the same NMR techniques to explore the metabolomics of different Wolbachia strain/host pairings, such as facultative Wolbachia strains that infect filarial nematodes, wMel that have been established in novel insect hosts, or Wolbachia strains (e.g., wAu) that impose no obvious effect on host reproduction (Hoffmann et al., 1996; Cao et al., 2019). Moreover, extending such investigations to pairings of Wolbachia and Aedes aegypti would be interesting, given the potential of the former in limiting the spread of tropical diseases carried by the latter (McGraw and O'Neill, 2013; O'Neill et al., 2018; Ryan et al., 2019). Furthermore, due to the strong involvement of lipid metabolism, a complementary lipidomics study would allow for greater metabolome coverage in that area of metabolism. If some of the metabolic effects are indeed localized to certain tissues, then performing tissue-specific metabolomics experiments is an obvious further step. Such experiments could also shed light on which of the metabolic changes are derived from Wolbachia metabolism and which are due to insect metabolism, given the preferential localization of Wolbachia in specific tissues. In addition, the present analyses are a static snapshot of Wolbachia infection, and as such changes in metabolite levels do not directly provide information about changes in metabolic fluxes, as the relationship between metabolic fluxes and metabolite levels is complex. Future fluxomics experiments are well-placed to resolve this point. In this context, it would also be intriguing to further explore the hypothesis that Wolbachia produces riboflavin for the Drosophila host cells, by designing fluxomics experiments that target specifically the fate of riboflavin. The metabolic interactions between Wolbachia and Drosophila also lend themselves to exploration via genome-scale modeling using available genome-scale metabolic models for both organisms (Coquin et al., 2008; Jiménez et al., 2019; Schönborn et al., 2019).
5. Conclusions
Here we have shown that in D. melanogaster infected with Wolbachia wMel, an endosymbiont with an oxidative metabolism, host metabolism is characterized by a hypoxic response. We have deduced the involvement of both the insulin/insulin-like growth factor and hypoxia signaling pathways in this reprogramming of mitochondrial metabolism. The downstream signaling cascades of both of these pathways support previous studies on Wolbachia infection in several insects. Furthermore, we show that the effects of Wolbachia infection are highly dependent on dietary iron levels in the host. The burden of iron overload is evident on both infected and uninfected Drosophila. In contrast, iron deficiency conditions lead to extra demands of Wolbachia on the host. Our results also support the hypothesis of metabolite extraction and provisioning between Wolbachia and the host, specifically the consumption of alanine by Wolbachia and the export of riboflavin and ATP. Lastly, our analysis has put forward a potential mechanism on how Wolbachia maintains infection, as both the metabolic reprogramming of mitochondrial metabolism and metabolic provisioning and extraction enables Wolbachia to survive inside the host cells.
Data Availability Statement
The datasets presented in this study can be found in online repositories. The names of the repository/repositories and accession number(s) can be found below: The raw NMR data for this study have been deposited in the MetaboLights database (Haug et al., 2020) under accession number MTBLS2090 (http://www.ebi.ac.uk/metabolights/MTBLS2090).
Author Contributions
JB and SO'N: experimental design. JB: insects. HS, GP, and JB: sample preparation. HS and GP: NMR spectroscopy. HS and LH: NMR data processing. DC-R, LH, NM, and HS: data analysis. DC-R, HS, and JB: biological interpretation. DC-R, LH, HS, JB, GP, NM, and SO'N: manuscript preparation, review and editing. HS, JB, and SO'N: supervision and funding resources. All authors contributed to the article and approved the submitted version.
Funding
The authors are grateful for financial support from the Australian Research Council DP0772992. HS acknowledges funding through a Queensland Smart State Fellowship (2005-2008), and Strategic Initiatives Funding by The University of Queensland (DVCR4480). The funders had no role in study design, data collection and analysis, decision to publish, or preparation of the manuscript.
Conflict of Interest
The authors declare that the research was conducted in the absence of any commercial or financial relationships that could be construed as a potential conflict of interest.
Acknowledgments
We thank Gene Wijffels and Paul R. Ebert for helpful discussions and internal review of this manuscript. We would also like to thank Alexandra Gloria for developing Figure 5. We thank Joshua Simpson for helpful advice with the color scheme of Figure 1 and Supplementary Figures 3, 5.
Supplementary Material
The Supplementary Material for this article can be found online at: https://www.frontiersin.org/articles/10.3389/fevo.2021.623561/full#supplementary-material
References
Ackerman, D., and Gems, D. (2012). Insulin/IGF-1 and hypoxia signaling act in concert to regulate iron homeostasis in Caenorhabditis elegans. PLoS Genet. 8:e1002498. doi: 10.1371/journal.pgen.1002498
Ahmed, U., Latham, P. S., and Oates, P. S. (2012). Interactions between hepatic iron and lipid metabolism with possible relevance to steatohepatitis. World J. Gastroenterol. 18:4651. doi: 10.3748/wjg.v18.i34.4651
Albertson, R., Tan, V., Leads, R. R., Reyes, M., Sullivan, W., and Casper-Lindley, C. (2013). Mapping Wolbachia distributions in the adult Drosophila brain. Cell. Microbiol. 15, 1527–1544. doi: 10.1111/cmi.12136
Altintas, O., Park, S., and Lee, S.-J. V. (2016). The role of insulin/IGF-1 signaling in the longevity of model invertebrates, C. elegans and D. melanogaster. BMB Rep. 49:81. doi: 10.5483/BMBRep.2016.49.2.261
Barretto, E. C., Polan, D. M., Beevor-Potts, A. N., Lee, B., and Grewal, S. S. (2020). Tolerance to hypoxia is promoted by FOXO regulation of the innate immunity transcription factor NF-κB/Relish in Drosophila. Genetics 215, 1013–1025. doi: 10.1534/genetics.120.303219
Brownlie, J. C., Cass, B. N., Riegler, M., Witsenburg, J. J., Iturbe-Ormaetxe, I., McGraw, E. A., et al. (2009). Evidence for metabolic provisioning by a common invertebrate endosymbiont, Wolbachia pipientis, during periods of nutritional stress. PLoS Pathog. 5:e1000368. doi: 10.1371/journal.ppat.1000368
Cao, L.-J., Jiang, W., and Hoffmann, A. A. (2019). Life history effects linked to an advantage for wAu Wolbachia in Drosophila. Insects 10:126. doi: 10.3390/insects10050126
Carneiro Dutra, H. L., Deehan, M. A., and Frydman, H. (2020). Wolbachia and Sirtuin-4 interaction is associated with alterations in host glucose metabolism and bacterial titer. PLoS Pathog. 16:e1008996. doi: 10.1371/journal.ppat.1008996
Chrostek, E., Marialva, M. S., Esteves, S. S., Weinert, L. A., Martinez, J., Jiggins, F. M., et al. (2013). Wolbachia variants induce differential protection to viruses in Drosophila melanogaster: a phenotypic and phylogenomic analysis. PLoS Genet. 9:e1003896. doi: 10.1371/journal.pgen.1003896
Clark, R. I., Tan, S. W., Péan, C. B., Roostalu, U., Vivancos, V., Bronda, K., et al. (2013). MEF2 is an in vivo immune-metabolic switch. Cell 155, 435–447. doi: 10.1016/j.cell.2013.09.007
Cloarec, O., Dumas, M.-E., Craig, A., Barton, R. H., Trygg, J., Hudson, J., et al. (2005a). Statistical total correlation spectroscopy: an exploratory approach for latent biomarker identification from metabolic 1H NMR data sets. Anal. Chem. 77, 1282–1289. doi: 10.1021/ac048630x
Cloarec, O., Dumas, M. E., Trygg, J., Craig, A., Barton, R. H., Lindon, J. C., et al. (2005b). Evaluation of the orthogonal projection on latent structure model limitations caused by chemical shift variability and improved visualization of biomarker changes in 1H NMR spectroscopic metabonomic studies. Anal. Chem. 77, 517–526. doi: 10.1021/ac048803i
Coquin, L., Feala, J. D., McCulloch, A. D., and Paternostro, G. (2008). Metabolomic and flux-balance analysis of age-related decline of hypoxia tolerance in Drosophila muscle tissue. Mol. Syst. Biol. 4:233. doi: 10.1038/msb.2008.71
Cronin, S. J., Woolf, C. J., Weiss, G., and Penninger, J. M. (2019). The role of iron regulation in immunometabolism and immune-related disease. Front. Mol. Biosci. 6:116. doi: 10.3389/fmolb.2019.00116
Darby, A. C., Armstrong, S. D., Bah, G. S., Kaur, G., Hughes, M. A., Kay, S. M., et al. (2012). Analysis of gene expression from the Wolbachia genome of a filarial nematode supports both metabolic and defensive roles within the symbiosis. Genome Res. 22, 2467–2477. doi: 10.1101/gr.138420.112
Davoodi, S., Galenza, A., Panteluk, A., Deshpande, R., Ferguson, M., Grewal, S., et al. (2019). The immune deficiency pathway regulates metabolic homeostasis in Drosophila. J. Immunol. 202, 2747–2759. doi: 10.4049/jimmunol.1801632
Dutra, H. L. C., Rodrigues, S. L., Mansur, S. B., De Oliveira, S. P., Caragata, E. P., and Moreira, L. A. (2017). Development and physiological effects of an artificial diet for Wolbachia-infected Aedes aegypti. Sci. Rep. 7, 1–11. doi: 10.1038/s41598-017-16045-6
Eriksson, L., Byrne, T., Johansson, E., Trygg, J., and Vikström, C. (2013). Multi-and Megavariate Data Analysis Basic Principles and Applications, Vol. 1. Malmö: Umetrics Academy.
Eriksson, L., Trygg, J., and Wold, S. (2008). CV-ANOVA for significance testing of PLS and OPLS® models. J. Chemometr. J. Chemometr. Soc. 22, 594–600. doi: 10.1002/cem.1187
Feala, J. D., Coquin, L., McCulloch, A. D., and Paternostro, G. (2007). Flexibility in energy metabolism supports hypoxia tolerance in Drosophila flight muscle: metabolomic and computational systems analysis. Mol. Syst. Biol. 3:99. doi: 10.1038/msb4100139
Ferecatu, I., Gonçalves, S., Golinelli-Cohen, M.-P., Clémancey, M., Martelli, A., Riquier, S., et al. (2014). The diabetes drug target mitoNEET governs a novel trafficking pathway to rebuild an Fe-S cluster into cytosolic aconitase/iron regulatory protein 1. J. Biol. Chem. 289, 28070–28086. doi: 10.1074/jbc.M114.548438
Foster, J., Ganatra, M., Kamal, I., Ware, J., Makarova, K., Ivanova, N., et al. (2005). The Wolbachia genome of Brugia malayi: endosymbiont evolution within a human pathogenic nematode. PLoS Biol. 3:e121. doi: 10.1371/journal.pbio.0030121
Fuchs, S., Bundy, J. G., Davies, S. K., Viney, J. M., Swire, J. S., and Leroi, A. M. (2010). A metabolic signature of long life in Caenorhabditis elegans. BMC Biol. 8:14. doi: 10.1186/1741-7007-8-14
Galindo-Prieto, B., Eriksson, L., and Trygg, J. (2014). Variable influence on projection (VIP) for orthogonal projections to latent structures (OPLS). J. Chemometr. 28, 623–632. doi: 10.1002/cem.2627
Geoghegan, V., Stainton, K., Rainey, S. M., Ant, T. H., Dowle, A. A., Larson, T., et al. (2017). Perturbed cholesterol and vesicular trafficking associated with dengue blocking in Wolbachia-infected Aedes aegypti cells. Nat. Commun. 8, 1–10. doi: 10.1038/s41467-017-00610-8
Gill, A. C., Darby, A. C., and Makepeace, B. L. (2014). Iron necessity: the secret of Wolbachia's success? PLoS Negl. Trop. Dis. 8:e3224. doi: 10.1371/journal.pntd.0003224
Godel, C., Kumar, S., Koutsovoulos, G., Ludin, P., Nilsson, D., Comandatore, F., et al. (2012). The genome of the heartworm, Dirofilaria immitis, reveals drug and vaccine targets. FASEB J. 26, 4650–4661. doi: 10.1096/fj.12-205096
Haddad, G. G. (2006). Tolerance to low O2: lessons from invertebrate genetic models. Exp. Physiol. 91, 277–282. doi: 10.1113/expphysiol.2005.030767
Haug, K., Cochrane, K., Nainala, V. C., Williams, M., Chang, J., Jayaseelan, K. V., et al. (2020). MetaboLights: a resource evolving in response to the needs of its scientific community. Nucleic Acids Res. 48, D440–D444. doi: 10.1093/nar/gkz1019
Hoerauf, A., Nissen-Pähle, K., Schmetz, C., Henkle-Dührsen, K., Blaxter, M. L., Büttner, D. W., et al. (1999). Tetracycline therapy targets intracellular bacteria in the filarial nematode Litomosoides sigmodontis and results in filarial infertility. J. Clin. Investig. 103, 11–18. doi: 10.1172/JCI4768
Hoffmann, A. A., Clancy, D., and Duncan, J. (1996). Naturally-occurring Wolbachia infection in Drosophila simulans that does not cause cytoplasmic incompatibility. Heredity 76, 1–8. doi: 10.1038/hdy.1996.1
Hoffmann, A. A., Turelli, M., and Simmons, G. M. (1986). Unidirectional incompatibility between populations of Drosophila simulans. Evolution 40, 692–701. doi: 10.1111/j.1558-5646.1986.tb00531.x
Hotelling, H. (1933). Analysis of a complex of statistical variables into principal components. J. Educ. Psychol. 24:417. doi: 10.1037/h0071325
Huynh, N., Ou, Q., Cox, P., Lill, R., and King-Jones, K. (2019). Glycogen branching enzyme controls cellular iron homeostasis via iron regulatory protein 1 and mitoNEET. Nat. Commun. 10, 1–18. doi: 10.1038/s41467-019-13237-8
Ikeya, T., Broughton, S., Alic, N., Grandison, R., and Partridge, L. (2009). The endosymbiont Wolbachia increases insulin/IGF-like signalling in Drosophila. Proc. R. Soc. B Biol. Sci. 276, 3799–3807. doi: 10.1098/rspb.2009.0778
Jiménez, N. E., Gerdtzen, Z. P., Olivera-Nappa, Á., Salgado, J. C., and Conca, C. (2019). A systems biology approach for studying Wolbachia metabolism reveals points of interaction with its host in the context of arboviral infection. PLoS Negl. Trop. Dis. 13:e0007678. doi: 10.1371/journal.pntd.0007678
Kanehisa, M., and Goto, S. (2000). KEGG: kyoto encyclopedia of genes and genomes. Nucleic Acids Res. 28, 27–30. doi: 10.1093/nar/28.1.27
Kitajima, H., Shiomoto, H., Osada, K., and Yokogoshi, H. (2003). Co-administration of proline and inorganic iron enhance the improvement of behavioral and hematological function of iron-deficient anemic rats. J. Nutr. Sci. Vitaminol. 49, 7–12. doi: 10.3177/jnsv.49.7
Kosmidis, S., Missirlis, F., Botella, J. A., Schneuwly, S., Rouault, T. A., and Skoulakis, E. (2014). Behavioral decline and premature lethality upon pan-neuronal ferritin overexpression in Drosophila infected with a virulent form of Wolbachia. Front. Pharmacol. 5:66. doi: 10.3389/fphar.2014.00066
Kremer, N., Voronin, D., Charif, D., Mavingui, P., Mollereau, B., and Vavre, F. (2009). Wolbachia interferes with ferritin expression and iron metabolism in insects. PLoS Pathog. 5:e1000630. doi: 10.1371/journal.ppat.1000630
Krishnan, S. N., Sun, Y. A., Mohesin, A., Wyman, R. J., and Haddad, G. G. (1997). Behavioral and electrophysiologic responses of Drosophila melanogaster to prolonged periods of anoxia. J. Insect Physiol. 43, 203–210. doi: 10.1016/S0022-1910(96)00084-4
Kusminski, C. M., Holland, W. L., Sun, K., Park, J., Spurgin, S. B., Lin, Y., et al. (2012). MitoNEET, a key regulator of mitochondrial function and lipid homeostasis. Nat. Med. 18:1539. doi: 10.1038/nm.2899
Langworthy, N. G., Renz, A., Mackenstedt, U., Henkle-Dührsen, K., Bronsvoort, M. B., Tanya, V. N., et al. (2000). Macrofilaricidal activity of tetracycline against the filarial nematode Onchocerca ochengi: elimination of Wolbachia precedes worm death and suggests a dependent relationship. Proc. R. Soc. Lond. Ser. B Biol. Sci. 267, 1063–1069. doi: 10.1098/rspb.2000.1110
Mattila, J., and Hietakangas, V. (2017). Regulation of carbohydrate energy metabolism in Drosophila melanogaster. Genetics 207, 1231–1253. doi: 10.1534/genetics.117.199885
McGraw, E. A., and O'Neill, S. L. (2013). Beyond insecticides: new thinking on an ancient problem. Nat. Rev. Microbiol. 11, 181–193. doi: 10.1038/nrmicro2968
McMeniman, C. J., Lane, A. M., Fong, A. W., Voronin, D. A., Iturbe-Ormaetxe, I., Yamada, R., et al. (2008). Host adaptation of a Wolbachia strain after long-term serial passage in mosquito cell lines. Appl. Environ. Microbiol. 74, 6963–6969. doi: 10.1128/AEM.01038-08
Min, K.-T., and Benzer, S. (1997). Wolbachia, normally a symbiont of Drosophila, can be virulent, causing degeneration and early death. Proc. Natl. Acad. Sci. U.S.A. 94, 10792–10796. doi: 10.1073/pnas.94.20.10792
Moriyama, M., Nikoh, N., Hosokawa, T., and Fukatsu, T. (2015). Riboflavin provisioning underlies Wolbachia's fitness contribution to its insect host. mBio 6:e01732–e01715. doi: 10.1128/mBio.01732-15
Müller, M., Mentel, M., van Hellemond, J. J., Henze, K., Woehle, C., Gould, S. B., et al. (2012). Biochemistry and evolution of anaerobic energy metabolism in eukaryotes. Microbiol. Mol. Biol. Rev. 76, 444–495. doi: 10.1128/MMBR.05024-11
Mylonis, I., Simos, G., and Paraskeva, E. (2019). Hypoxia-inducible factors and the regulation of lipid metabolism. Cells 8:214. doi: 10.3390/cells8030214
Nässel, D. R., Liu, Y., and Luo, J. (2015). Insulin/IGF signaling and its regulation in Drosophila. Gen. Comp. Endocrinol. 221, 255–266. doi: 10.1016/j.ygcen.2014.11.021
Navarro, J. A., Ohmann, E., Sanchez, D., Botella, J. A., Liebisch, G., Moltó, M. D., et al. (2010). Altered lipid metabolism in a Drosophila model of Friedreich's ataxia. Hum. Mol. Genet. 19, 2828–2840. doi: 10.1093/hmg/ddq183
Newton, I. L., and Rice, D. W. (2020). The Jekyll and Hyde symbiont: could Wolbachia be a nutritional mutualist? J. Bacteriol. 202:e00589-19. doi: 10.1128/JB.00589-19
Nikoh, N., Hosokawa, T., Moriyama, M., Oshima, K., Hattori, M., and Fukatsu, T. (2014). Evolutionary origin of insect-Wolbachia nutritional mutualism. Proc. Natl. Acad. Sci. U.S.A. 111, 10257–10262. doi: 10.1073/pnas.1409284111
O'Farrell, P. H. (2001). Conserved responses to oxygen deprivation. J. Clin. Investig. 107, 671–674. doi: 10.1172/JCI12562
O'Neill, S. L., Ryan, P. A., Turley, A. P., Wilson, G., Retzki, K., Iturbe-Ormaetxe, I., et al. (2018). Scaled deployment of Wolbachia to protect the community from dengue and other Aedes transmitted arboviruses. Gates Open Res. 2:36. doi: 10.12688/gatesopenres.12844.1
Perez, C. L., and Van Gilst, M. R. (2008). A 13C isotope labeling strategy reveals the influence of insulin signaling on lipogenesis in C. elegans. Cell Metab. 8, 266–274. doi: 10.1016/j.cmet.2008.08.007
Rockfield, S., Chhabra, R., Robertson, M., Rehman, N., Bisht, R., and Nanjundan, M. (2018). Links between iron and lipids: implications in some major human diseases. Pharmaceuticals 11:113. doi: 10.3390/ph11040113
Ryan, P. A., Turley, A. P., Wilson, G., Hurst, T. P., Retzki, K., Brown-Kenyon, J., et al. (2019). Establishment of wMel Wolbachia in Aedes aegypti mosquitoes and reduction of local dengue transmission in Cairns and surrounding locations in northern Queensland, Australia. Gates Open Res. 3:1547. doi: 10.12688/gatesopenres.13061.1
Savorani, F., Tomasi, G., and Engelsen, S. B. (2010). icoshift: a versatile tool for the rapid alignment of 1D NMR spectra. J. Magnet. Reson. 202, 190–202. doi: 10.1016/j.jmr.2009.11.012
Schönborn, J. W., Jehrke, L., Mettler-Altmann, T., and Beller, M. (2019). FlySilico: flux balance modeling of Drosophila larval growth and resource allocation. Sci. Rep. 9, 1–16. doi: 10.1038/s41598-019-53532-4
Sepúlveda Cisternas, I., Salazar, J. C., and Garcia-Angulo, V. A. (2018). Overview on the bacterial iron-riboflavin metabolic axis. Front. Microbiol. 9:1478. doi: 10.3389/fmicb.2018.01478
Stairs, C. W., Leger, M. M., and Roger, A. J. (2015). Diversity and origins of anaerobic metabolism in mitochondria and related organelles. Philos. Trans. R. Soc. B Biol. Sci. 370:20140326. doi: 10.1098/rstb.2014.0326
Tang, H., and Pang, S. (2016). Proline catabolism modulates innate immunity in Caenorhabditis elegans. Cell Rep. 17, 2837–2844. doi: 10.1016/j.celrep.2016.11.038
Tang, X., and Zhou, B. (2013). Iron homeostasis in insects: insights from Drosophila studies. IUBMB Life 65, 863–872. doi: 10.1002/iub.1211
Texada, M. J., Jørgensen, A. F., Christensen, C. F., Koyama, T., Malita, A., Smith, D. K., et al. (2019). A fat-tissue sensor couples growth to oxygen availability by remotely controlling insulin secretion. Nat. Commun. 10, 1–16. doi: 10.1038/s41467-019-09943-y
Trygg, J., and Wold, S. (2002). Orthogonal projections to latent structures (O-PLS). J. Chemometr. 16, 119–128. doi: 10.1002/cem.695
Ulrich, E. L., Akutsu, H., Doreleijers, J. F., Harano, Y., Ioannidis, Y. E., Lin, J., et al. (2007). BioMagResBank. Nucleic Acids Res. 36(Suppl_1), D402–D408. doi: 10.1093/nar/gkm957
Wang, H., Jiang, X., Wu, J., Zhang, L., Huang, J., Zhang, Y., et al. (2016). Iron overload coordinately promotes ferritin expression and fat accumulation in Caenorhabditis elegans. Genetics 203, 241–253. doi: 10.1534/genetics.116.186742
Wang, Y., Landry, A. P., and Ding, H. (2017). The mitochondrial outer membrane protein mitoNEET is a redox enzyme catalyzing electron transfer from FMNH2 to oxygen or ubiquinone. J. Biol. Chem. 292, 10061–10067. doi: 10.1074/jbc.M117.789800
Wishart, D. S., Feunang, Y. D., Marcu, A., Guo, A. C., Liang, K., Vázquez-Fresno, R., et al. (2018). HMDB 4.0: the human metabolome database for 2018. Nucleic Acids Res. 46, D608–D617. doi: 10.1093/nar/gkx1089
Wong, D. M., Shen, Z., Owyang, K. E., and Martinez-Agosto, J. A. (2014). Insulin-and warts-dependent regulation of tracheal plasticity modulates systemic larval growth during hypoxia in Drosophila melanogaster. PLoS ONE 9:e115297. doi: 10.1371/journal.pone.0115297
Wong, Z. S., Brownlie, J. C., and Johnson, K. N. (2015). Oxidative stress correlates with Wolbachia-mediated antiviral protection in Wolbachia-Drosophila associations. Appl. Environ. Microbiol. 81, 3001–3005. doi: 10.1128/AEM.03847-14
Wong, Z. S., Hedges, L. M., Brownlie, J. C., and Johnson, K. N. (2011). Wolbachia-mediated antibacterial protection and immune gene regulation in Drosophila. PLoS ONE 6:e25430. doi: 10.1371/journal.pone.0025430
Wu, M., Sun, L. V., Vamathevan, J., Riegler, M., Deboy, R., Brownlie, J. C., et al. (2004). Phylogenomics of the reproductive parasite Wolbachia pipientis wMel: a streamlined genome overrun by mobile genetic elements. PLoS Biol. 2:e69. doi: 10.1371/journal.pbio.0020069
Yamada, R., Iturbe-Ormaetxe, I., Brownlie, J., and O'Neill, S. (2011). Functional test of the influence of Wolbachia genes on cytoplasmic incompatibility expression in Drosophila melanogaster. Insect Mol. Biol. 20, 75–85. doi: 10.1111/j.1365-2583.2010.01042.x
Zarse, K., Schmeisser, S., Groth, M., Priebe, S., Beuster, G., Kuhlow, D., et al. (2012). Impaired insulin/IGF1 signaling extends life span by promoting mitochondrial L-proline catabolism to induce a transient ROS signal. Cell Metab. 15, 451–465. doi: 10.1016/j.cmet.2012.02.013
Zou, Y.-X., Ruan, M.-H., Luan, J., Feng, X., Chen, S., and Chu, Z.-Y. (2017). Anti-aging effect of riboflavin via endogenous antioxidant in fruit fly Drosophila melanogaster. J. Nutr. Health Aging 21, 314–319. doi: 10.1007/s12603-016-0752-8
Keywords: Wolbachia, Drosophila melanogaster, iron, metabolomics, NMR, insulin/insulin-like growth factor signaling (IIS) pathway, hypoxia
Citation: Currin-Ross D, Husdell L, Pierens GK, Mok NE, O'Neill SL, Schirra HJ and Brownlie JC (2021) The Metabolic Response to Infection With Wolbachia Implicates the Insulin/Insulin-Like-Growth Factor and Hypoxia Signaling Pathways in Drosophila melanogaster. Front. Ecol. Evol. 9:623561. doi: 10.3389/fevo.2021.623561
Received: 30 October 2020; Accepted: 25 February 2021;
Published: 22 March 2021.
Edited by:
Anne Duplouy, Lund University, SwedenReviewed by:
Nathan Rank, Sonoma State University, United StatesThomas Dandekar, Julius Maximilian University of Würzburg, Germany
Copyright © 2021 Currin-Ross, Husdell, Pierens, Mok, O'Neill, Schirra and Brownlie. This is an open-access article distributed under the terms of the Creative Commons Attribution License (CC BY). The use, distribution or reproduction in other forums is permitted, provided the original author(s) and the copyright owner(s) are credited and that the original publication in this journal is cited, in accordance with accepted academic practice. No use, distribution or reproduction is permitted which does not comply with these terms.
*Correspondence: Jeremy C. Brownlie, j.brownlie@griffith.edu.au; Horst Joachim Schirra, h.schirra@uq.edu.au