Blood platelet RNA profiles do not enable for nivolumab response prediction at baseline in patients with non-small cell lung cancer
Abstract
BACKGROUND:
Anti-PD-(L)1 immunotherapy has emerged as a promising treatment approach for non-small cell lung cancer (NSCLC), though the response rates remain low. Pre-treatment response prediction may improve patient allocation for immunotherapy. Blood platelets act as active immune-like cells, thereby constraining T-cell activity, propagating cancer metastasis, and adjusting their spliced mRNA content.
OBJECTIVE:
We investigated whether platelet RNA profiles before start of nivolumab anti-PD1 immunotherapy may predict treatment responses.
METHODS:
We performed RNA-sequencing of platelet RNA samples isolated from stage III-IV NSCLC patients before treatment with nivolumab. Treatment response was scored by the RECIST-criteria. Data were analyzed using a predefined thromboSeq analysis including a particle-swarm-enhanced support vector machine (PSO/SVM) classification algorithm.
RESULTS:
We collected and processed a 286-samples cohort, separated into a training/evaluation and validation series and subjected those to training of the PSO/SVM-classification algorithm. We observed only low classification accuracy in the 107-samples validation series (area under the curve (AUC) training series: 0.73 (95% -CI: 0.63–0.84, n = 88 samples), AUC evaluation series: 0.64 (95% -CI: 0.51–0.76, n = 91 samples), AUC validation series: 0.58 (95% -CI: 0.45–0.70, n = 107 samples)), employing a five-RNAs biomarker panel.
CONCLUSIONS:
We concluded that platelet RNA may have minimally discriminative capacity for anti-PD1 nivolumab response prediction, with which the current methodology is insufficient for diagnostic application.
1Introduction
Lung cancer, especially non-small cell lung cancer (NSCLC), is the most common cause of cancer-related mortality in the world, causing over one million deaths annually [1]. Interestingly, PD-(L)1 checkpoint inhibitors have shown remarkable effectiveness, durability of response and tolerability [2], although overall response rates remain low (∼20%) [3, 4]. Numerous studies have identified correlations between smoking, tumor tissue mutational load, infiltration of CD8-positive T-cells and response to anti-PD-(L)1 immunotherapy [5, 6]. At the moment, current stratification of patients for anti-PD-(L)1 targeted therapy is hampered by limited accuracy and concordance of available biomarkers, including the currently used tumor tissue PD-L1-protein expression marker. Identification of patients with a low likelihood of response to anti-PD-(L)1 immunotherapy, while still correctly identifying individuals who most likely benefit from this therapy, may prevent unnecessary treatment and concomitant costs, and potential exposure of patients to serious immunological adverse events.
Blood platelets are cell fragments involved in hemostasis, initiation of wound healing and metastasis of cancer [7, 8]. They originate from the megakaryocytes that reside in the bone marrow and lung parenchyma [7, 9], and are loaded with pre-mature RNA molecules [10–12]. Upon environmental cues, platelets splice their mRNA molecules resulting in platelet RNA repertoires of both spliced and unspliced mRNAs [10, 13, 14]. In addition, platelets have the capability to sequester RNAs [15] and load platelet microparticles with RNAs that can be transferred to other cells, such as endothelial and tumor cells [16, 17]. Functionally, platelet-derived TGFß and direct physical platelet-tumor cell interactions can induce tumor cell epithelial-mesenchymal transition [18], a process that has previously also been associated with resistance to anti-PD1 immunotherapy [19]. In addition, platelets can behave as immunomodulators in inflammatory conditions [20, 21], regulate immune cell extravasation [22, 23], and cross-communicate with lymphocytes [24] and neutrophils [25]. It was shown that platelets armed with immunotherapeutic antibodies home to lung tumors in mice [26], exhibiting profound anti-tumor activity. Also, previously pretreatment increased platelet-to-lymphocyte ratio in patients with metastatic NSCLC treated with nivolumab was associated with worse overall survival and a reduced response rate [27]. Platelets are thus potentially involved in the immune response towards tumor cells, and might thereby alter their RNA composition.
We recently provided evidence that platelets may serve as blood-based liquid biopsies for the detection and subclassification of cancer [14, 15, 28], as well as for therapy response monitoring [29, 30]. In this prospective study, we investigated whether platelet RNA signatures may provide classification power for nivolumab immunotherapy response prediction before start of treatment.
2Methods
2.1Study design
In this prospective, observational, multi-center study in patients with advanced (stage III-IV NSCLC blood samples at moment of baseline for nivolumab treatment were collected. The aim was to optimally identify patients who respond to nivolumab, for which clinical imaging data was available as a gold standard for response evaluation. Sample size calculation was performed using the method of Dobbin and Simon [31], assuming an overall response rate of 17% [32], employing the results from a pilot study (data not shown). We estimated a required samples size for the training series of 180 patients (i.e. ∼31 responders and ∼149 non-responders) under assumption that the gene with maximal mean fold difference between groups will exhibit a fold change of 2.5. From a clinical perspective we expected a successful biomarker to have 95% sensitivity and at least 50% specificity, i.e. halving the number of unnecessarily treated patients while keeping the number of false negatives low. We defined the new biomarker to be successful if the lower boundary of the 95% -confidence interval for the specificity lies above 38% at the threshold/cut-off where its specificity is 95%. The rationale for this is that at the 95% sensitivity level a specificity of 38% will yield an overall response rate (ORR) in the positively tested group of 24% which is the rate achieved by the tissue PD-L1-test [32] at the much lower sensitivity of 46%. A validation series of 90 patients (75 non-responders and 15 responders) has 90% power to achieve this assuming a true specificity of the test of 55%.
2.2Patient and sample collection
All patients with advanced (stage III/IV) NSCLC who were scheduled to start treatment with nivolumab were recruited for voluntary inclusion in this study. Patients were included in the Amsterdam UMC, Amsterdam, the Netherlands Cancer Institute (NKI/AvL), Amsterdam and the University Medical Center Groningen, Groningen, all from The Netherlands. Patients were included from December 2013 till June 2017, with follow-up until June 2018. The median time of follow-up was 195 days (Interquartile range (IQR): 97–399,5 days). All patients received treatment in accordance with recent literature [3, 33] and local guidelines. Platelet samples were collected up to one month before start of treatment, but not after start of treatment. Other clinical data, including computed tomography (CT) scans, blood tests and relevant clinical data such as the use of medication used in this study were collected for routine clinical practice. These were handled according to the Dutch Personal Data Protection Act (WPB). Response assessment of patients treated with nivolumab was performed by CT-imaging at baseline, 6–8 weeks, 3 months and 6 months after start of treatment. Treatment response was assessed according to the updated RECIST version 1.1 criteria and was scored as progressive disease (PD), stable disease (SD), partial response (PR), or complete response (CR) [34, 35]. A responder was defined as those patients with PR or CR at three months, despite possible PD at six months. A patient was also regarded a responder with SD at three and six months.
Tumor tissue was stained using either the PD-L1 22C3 (Agilent) or PD-L1 28-8 (Abcam) antibodies and scored according to the manufacturer’s instructions. This study was conducted in accordance with the principles of the Declaration of Helsinki, and all participant provided written informed consent. Our study was approved by the local medical ethical committee (PTC NKI-AvL, Amsterdam, NL45524.031.13; medical ethics committee, UMCG, Groningen, 2010/109 [36]; Dutch trial register NL7839) and the patient privacy committee, and was performed according to the institutional patient privacy protocols.
2.3Platelet isolation
Peripheral whole blood was drawn by regular venipuncture in EDTA-coated Vacutainer tubes. Whole blood samples in 6- or 10 mL EDTA-coated Vacutainer tubes were processed using standardized protocols within 12 hours as described previously [14, 15, 37] at room temperature (21°C). Here, using a 20-minute 120×g centrifugation step platelet rich plasma (PRP) was separated from nucleated blood cells. Following, 9/10th of the PRP needs to be collected carefully without disturbing the buffy coat, with the risk of contaminating the platelet preparation with nucleated cells. Then the platelets were pelleted from the PRP by a 20-minute 360×g centrifugation step. Platelet pellets were carefully resuspended in RNAlater (Life Technologies), followed by an overnight incubation at 4°C frozen at –80°C.
2.4Platelet RNA-sequencing
The thromboSeq wet-lab platelet mRNA-sequencing protocol and dry-lab software modules have recently been described in detail and are publicly available [37]. In brief, first platelet total RNA was isolated using the mirVana miRNA isolation kit (Ambion, Thermo Scientific, AM1560). All samples were subjected to cDNA synthesis and amplification using the SMARTer Ultra Low RNA Kit for Illumina Sequencing v3 (Clontech, cat. nr. 634853) with an input of 500 picogram of total RNA. All amplified platelet cDNA was subjected to nucleic acid shearing by sonication (Covaris Inc) and subsequently labeled with single index barcodes for Illumina sequencing using the Truseq Nano DNA Sample Prep Kit (Illumina, cat nr. FC-121–4001). All bead clean-up steps were performed using a 15-minute bead-cDNA binding step and a 10-cycle enrichment PCR. Other steps were according to manufacturer’s protocol. During this protocol, multiple quality control steps were performed using RNA and DNA chips available for the Bioanalyzer 2100 (Agilent). High-quality samples with product sizes between 300–500 bp were pooled (12–24 samples per pool) in equimolar concentrations for superficial thromboSeq and submitted for 100 bp Single Read sequencing on the Illumina Hiseq 2500 and 4000 platform. During whole blood, blood platelet and platelet RNA processing, the researchers were blinded to the clinical outcome measures.
2.5Processing of raw RNA-sequencing data
We employed Trimmomatic (v. 0.22) [38] for trimming and clipping of sequence adapters from the RNA-seq reads, of which the resulting reads were mapped to the human reference genome (hg19) using STAR (v. 2.3.0) [39]. The mapped reads were summarized using HTSeq (v. 0.6.1), guided by the Ensembl gene annotation version 75 [40]. All following statistical and analytical analyses were performed in R (version 3.3.0) and R-studio (version 0.99.902). Genes encoded on the mitochondrial DNA and the Y-chromosome were excluded from downstream analyses. To circumvent potential contamination from cell-free DNA, only intron-spanning spliced RNA reads were employed for data analysis [41]. Sample filtering was performed by assessing the library complexity, which is partially associated with the intron-spanning reads library size. The raw sequencing data has been deposited at the GEO database under accession number GSE216297.
2.6thromboSeq classification algorithm
We employed our previously standardized and optimized thromboSeq algorithm for data analysis [28, 37, 41], which is extensively described elsewhere. For this study, software-version v1.5 of the thromboSeq source code was employed, and is for research purposes publicly available via GitHub (https://github.com/MyronBest/thromboSeq_source_code_v1.5) [28]. In short, the particle-swarm-optimization (PSO-) enhanced support vector machine (SVM)-based thromboSeq algorithm employs an SVM-algorithm for RNA expression classification tasks, supplemented by a PSO algorithm that iteratively selects parameters for gene panel selection. The thromboSeq algorithm is developed with the use of a training, evaluation, and independent validation series. The training series is used for selection of stable genes that are used for remove unwanted variation (RUV)-mediated data normalization, gene panel selection and SVM-training. Here, RUV is employed to minimize variability among the samples introduced by potential confounding factors [41]. This correction measure is determined based on the training series to enable for independent validation of the remaining samples. The gene panel is selected using ANOVA-statistics, supplemented by filtering for highly correlated RNAs as well as filtering for RNAs most contributive to the SVM-model. Based on this selected gene panel an SVM-model including class weight correction is trained. Next, the evaluation series is employed to evaluate the classification performance of the SVM-model with the particular gene panel and algorithm settings. Here, a PSO-algorithm is used to repeatedly provide more optimized thresholds to select the gene panel and set other algorithm settings, of which the optimization steps are evaluated using the classification scores in the evaluation series. Finally the algorithm and gene panel is locked following which the validation series is used for validation of the PSO-optimized SVM-classification algorithm.
For this study, the variables ‘Age’ and ‘lib.size’ were applied as potential confounding factors for RUV-correction with both a default threshold of 0.8. The swarm-parameters ‘lib.size’, ‘FDR’, ‘correlatedTranscripts’, and ‘rankedTranscripts’ were employed using the following boundaries, respectively: –0.1–1.0; 5–number of genes with ANOVA FDR < 0.5; 0.5–1.0; 5–number of genes with ANOVA FDR < 0.5. In total 100 particles were deployed with eight iterations. Class-weights and a rule-out optimization step forcing towards at least 95% sensitivity in the evaluation series during particle optimization were enabled. The algorithm employed has selected the following thresholds: ‘lib.size’: 0.132; ‘FDR’: 5; ‘correlatedTranscripts’: 0.9898; ‘rankedTranscripts’: 12.292.
An ANOVA comparison was performed using the thromboSeq.anova-function in the thromboSeq software. The variables ‘Age’ and ‘lib.size’ were subjected to RUV-correction, with the thresholds ‘0.8’ and ‘0.8’, and with k.variables=3. The heatmap clustering was optimized by a PSO-function thereby adjusting the FDR-threshold evaluated by column-dendrogram clustering.
2.7Statistical analyses
SPSS (v25; SPSS, Chicago, USA) was used for the descriptive statistics. For a between-group-comparison the One way ANOVA, Fisher-Freeman Halton exact test, or chi-square test was used depending on the variable.
3Results
We prospectively collected 300 blood samples from patients with advanced NSCLC that were selected for treatment with the PD-1 monoclonal antibody nivolumab. These were subjected to the thromboSeq protocol (Fig. 1A). 14 samples (4%) were excluded from follow-up analyses according to previously introduced thromboSeq quality measures (Supplemental Figure 1) [37, 41]. The remaining 286 samples were included for the analyses (Table 1). We observed no statistically significant differences between responders and non-responders regarding age, gender, and hospital of patient inclusion (Table 1). We also observed no statistically significant difference in total platelet counts between responders and non-responders of whom this data was available (p = 0.660, n = 185, unpaired Student’s t-test, Table 1). Tumor tissue was partially available for correlative PD-L1 immunohistochemistry analysis. Additional demographic variables are presented in Table 1 and Table S1.
Fig. 1
Platelet RNA-based nivolumab response prediction. (A) Schematic overview of the experimental setup. Blood of patients eligible for treatment with PD-1 inhibitor nivolumab was included from one month before till start of treatment (baseline. t = 0). Tumor response read-out based on CT-imaging and according to the RECIST 1.1 criteria were performed at 6–8 weeks. 3 months. and 6 months. after start of nivolumab therapy. A responder was defined as PR. CR at three months and SD at three and six months. A non-responder was defined as PD and SD after three. but PD after six months. (B) Heatmap and unsupervised hierarchical clustering analysis of RNAs with differential RNA levels between nivolumab responders (n = 88) and non-responders (n = 198). Columns indicate samples. rows indicate RNAs. and color intensity represents the Z-score transformed RNA expression values. Clustering of samples showed non-random partitioning (p < 0.003. Fisher’s exact test). The clinical and technical variables ‘hospital of sample location’. ‘library size’. ‘sex’. ‘age’. ‘smoking status’. ‘tumor stage’. and ‘PD-L1 tumor tissue status’ are indicated on top of the heatmap with each owns color coding (legend on the right). (C) Receiver operating characteristics curve of the thromboSeq nivolumab response prediction algorithm at moment of treatment baseline. Indicated are the training series (dashed gray line). evaluation series (gray line). and validation series (red line). Also indicated are sample numbers. AUC values. and the 95% confidence intervals (95% -CI). (D) 2×2 tables summarizing the classification results for the evaluation series (upper table) and validation series (lower table) of the thromboSeq nivolumab response prediction algorithm at moment of treatment baseline geared towards a rule-out setting. Indicated are sample numbers and percentage of total samples.
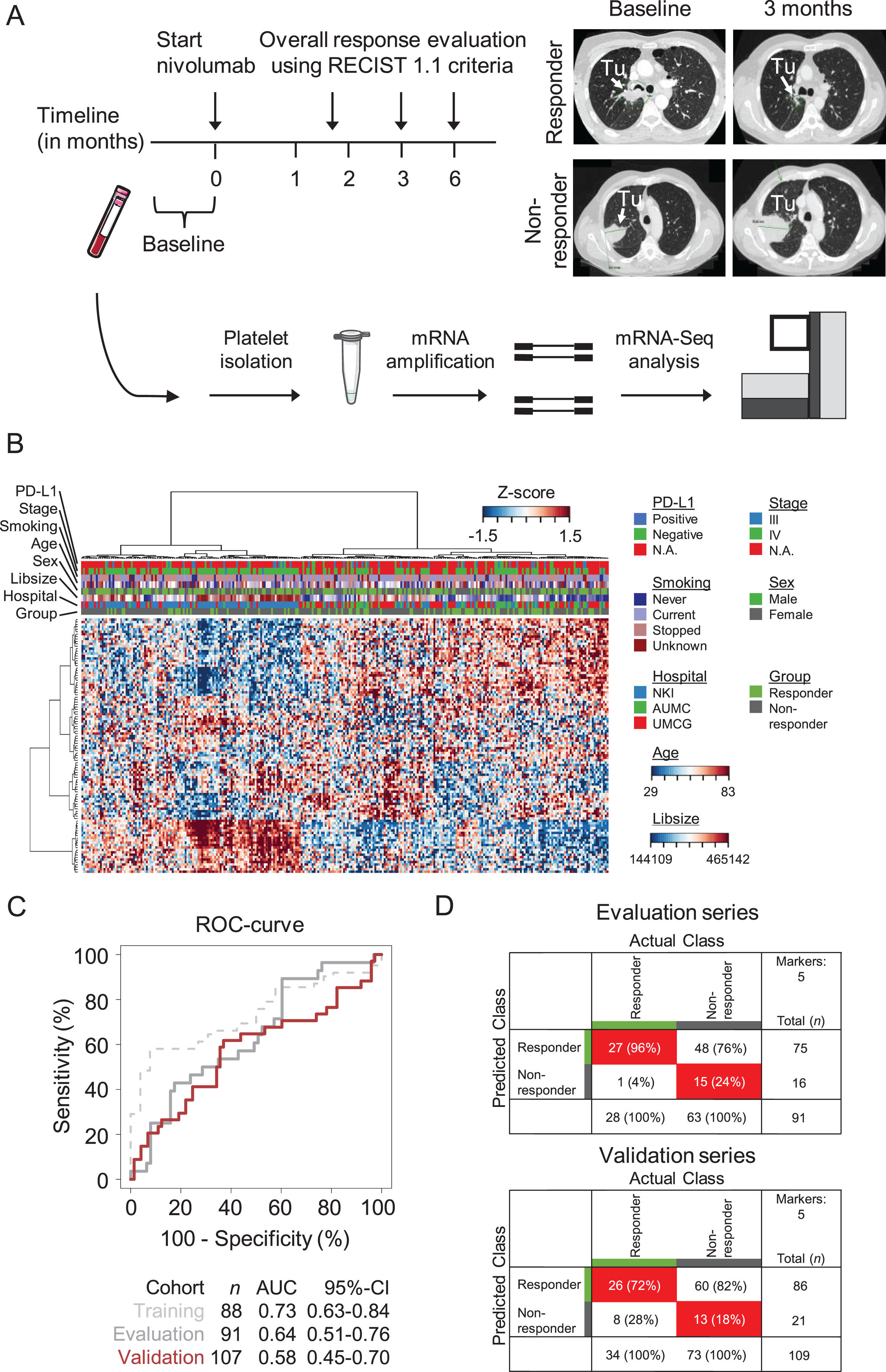
Table 1
Baseline characteristics of responders versus non-responders
Non-responder N = 198 | Responder N = 88 | Total (N = 286) | P-value | |
Patient | ||||
Male sex–No. (%) | 115 (58.1) | 43 (48.9) | 158 (55.2) | 0.15A |
Age (yr)–Mean (95% CI) | 63.8 (62.5–65.1) | 64.9 (62.8–67.1) | 64.1 (63.0–65.3) | 0.61B |
Smoking status | 20 (10.1) | 4 (4.5) | 24 (8.4) | 0.05C |
Never / Current / Stop–No. (%) | 33 (16.7) | 25 (28.4) | 58 (20.3) | |
132 (66.7) | 57 (64.8) | 189 (66.1) | ||
Pack Years–Mean (95% CI) | 32.6 (29.6–35.6) | 37.4 (33.8–41.1) | 34.2 (31.9–36.5) | 0.01B |
WHO Performance status≥2–no / total known patients | 31/182 | 2/84 | 33/266 | 0.003C |
Platelet not normal rangeA *–no. / total known patients | 27/128 | 7/57 | 34/185 | 0.15A |
Platelet Count Baseline–Mean (95% CI) | 338.0 (314.5–361.6) | 326.7 (295.4–357.9) | 334.5(315.7–353.3) | 0.66B |
Brain metastasis | 43 | 17 | 60 | 0.65A |
Pathology–no. | ||||
Adenocarcinoma | 141 | 60 | 201 | 0.46A |
Squamous cell carcinoma | 47 | 22 | 69 | |
Other | 7 | 6 | 13 | |
Unknown | 3 | 0 | 3 | |
PD-L1–no. | ||||
Negative | 26 | 12 | 38 | 0.48C |
Positive 1–50% | 10 | 5 | 15 | |
Positive> 50% | 3 | 4 | 7 | |
Unknown | 159 | 67 | 226 | |
Previous treatment–no. | ||||
CCRT | 19 | 10 | 29 | 0.86A |
Platinum chemotherapy | 109 | 47 | 156 | |
Unknown | 67 | 29 | 96 | |
Line of treatment–no. | ||||
1 | 2 | 1 | 3 | 0.58 A |
2 | 101 | 43 | 144 | |
< 2 | 25 | 14 | 39 | |
Unknown | 70 | 30 | 100 | |
Previous RT / total known patients | 87/127 | 34/58 | 121/185 | 0.24 A |
Thoracic RT / total known patients | 53/127 | 26/58 | 79/185 | 0.75 A |
Platelet related medication–no. | ||||
None | 82 | 38 | 120 | 0.43 A |
Acetylsalicyclic acid | 23 | 9 | 32 | |
Other | 22 | 11 | 33 | |
Unknown | 71 | 30 | 101 | |
Hospital–no. | ||||
NKI | 90 | 38 | 128 | 0.84 A |
AUMC | 39 | 20 | 59 | |
UMCG | 69 | 30 | 99 | |
Survival | ||||
OS–mean (95% CI) | 165.3 (146.1–184.55) | 505.8 (465.7–545.8) | 270.4(244.7–296.2) | < 0.001 B |
PFS–mean (95% CI) | 72.6 (59.4–85.8) | 455.8 (413.3–498.4) | 190.5 (164.5–216.5) | < 0.001 B |
Abbreviations: N: Number of patients; no.: Number of patients; yr: years; 95% CI: 95% confidence interval; WHO performance-status score: World Health Organization performance status score. this is a score ranging from 0 to 5. where 0 indicates no symptom. 1 indicates mild symptoms and above 1 indicates greater disability; PD-L1: Programmed death ligand 1; CCRT: chemoradiation therapy; RT: radiation therapy; RECIST: Response evaluation criteria in Solid Tumors; NKI: Netherlands Cancer Institute. AUMC: Amsterdam University Medical Centers. UMCG: University Medical Center Groningen. OS: Overall Survival; PFS: progression free survival. *Either below under limit of normal or above limit of normal. as defined by the clinical laboratory. In the Netherlands a normal platelet count is between 150–350 platelets×10∧9 / liter (150.000–350.000 platelets per microliter). Used statistical tests: A: Chi Square, B: One way ANOVA, C: Fisher-Freeman Halton exact test.
The full dataset was subdivided into training (n = 88), evaluation (n = 91), and validation series (n = 107; Supplemental Figure 1C), as previously performed according to our thromboSeq dry-lab protocol [37]. For comparative analysis, we forced the patients from whom the tumor tissue PD-L1 status was available into the validation series. The hospital of origin (i.e. sample collection) was equally distributed among the three series (Table S2). The separation of samples among the three series was defined in the study protocol and based on preliminary data and power calculations (see Methods).
We first performed an ANOVA comparison on all platelet RNA samples, identifying platelet RNAs with discriminative power between nivolumab responders and non-responders. From the 3750 platelet RNAs that were detected in the dataset in total, 27 had statistically significant differential RNA levels (False Discovery Rate (FDR)< 0.05, Table S3). From these RNAs, only one RNA from the GSEA inflammatory response gene data set [42] had a statistically significant differential expression level (NAMPT, FDR = 0.0002).
Heatmap dendrogram clustering of the samples applying these 27 RNAs resulted in non-significant clustering between responders and non-responders (p-value: 0.24, Fisher’s exact test, data not shown). Adjusting the ANOVA-statistics significance threshold employing a PSO-function (see Methods) resulted in dendrogram clustering discriminating responders from non-responders (p-value< 0.003, Fisher’s exact test, used FDR-threshold: 0.419696, Fig. 1B). Improving discriminating power by adjusting the ANOVAs FDR-threshold implies that also statistically non-significant RNAs can contribute to the clustering accuracy. This provides the rationale for the PSO-enhanced classification algorithm development selecting an RNA panel based on most contributive power to a discriminative biomarker panel.
We next performed PSO-enhanced training of an SVM-classification algorithm, forcing the algorithm to identify optimal algorithm settings including the RNA biomarker panel for a rule-out nivolumab response prediction classifier. This would enable accurate identification of patients who do respond to nivolumab, while providing the opportunity to exclude patients that will not respond. This training process resulted into an area under the curve (AUC) for the training series of 0.73 (95% -CI: 0.63–0.84, n = 88 samples, as determined by LOOCV), and for the evaluation series of 0.64 (95% -CI: 0.51–0.76, n = 91 samples) for the evaluation series. Subsequent validation of the 107-samples validation series resulted into an AUC of 0.58 (95% -CI: 0.45–0.70, n = 5 spliced RNA biomarker panel, Fig. 1C). The five-gene biomarker panel as unbiasedly selected by the thromboSeq software was composed of the RNAs HPSE, HBD, PRSS50, CRYM, and LARP1 (SupplementalFigure 2A). Using the classification score readout towards a ‘rule-out’ application, in a sensitivity of 96% (95% -CI: 82–100%, n = 28) with a specificity of 24% (95% -CI: 14–36%, n = 63) in the evaluation series, resulted in a concomitant sensitivity in the validation series of 76% (95% -CI: 59–89%, n = 34) and a specificity of 18% (95% -CI: 10–29%, n = 73, Fig. 1D), with which our predefined threshold of a successful new biomarker is not reached.
Subsequent analysis of classification accuracies according to PD-L1 tumor tissue status of whom the data was available showed on average increased classification scores for patients responding to nivolumab as compared to patients not responding to nivolumab (Supplemental Figure 2B).
4Discussion
Anti-PD-(L)1 therapies have revolutionized cancer treatment, although, (non-invasive) predictive biomarkers are highly desirable [43]. In this proof-of-concept study, we aimed to associate spliced platelet RNA profiles to nivolumab response prediction measures at moment of therapy baseline.
Platelets act as local systemic responders during tumorigenesis [8], thereby suffering from tumor-mediated platelet education, and altering its behavior [18, 22, 44]. In previous studies, we have demonstrated that platelet RNA can function as a biomarker trove to detect and classify cancer from blood [14, 28]. Using our previously developed thromboSeq platform, we could not find solid RNA repertoires associated with tumor response at moment of start of therapy. Therefore, we were in this setting and study setup unable to successfully validate a nivolumab response prediction thromboSeq algorithm. Therefore at this point, interrogation of platelet RNA signatures before start of nivolumab treatment seems not to be useful for nivolumab therapy selection.
Because platelets are regarded as local immuno-like responders, the role of platelets in anti-tumor activity prior to and during anti-PD-(L)1 therapy, including direct and indirect physical interactions with lymphocytes, macrophages, and tumor cells should not be underestimated. In our five-gene platelet biomarker panel, both HPSE (heparanase) and LARP1 (La RibonucleoProtein 1) have lower expression values in nivolumab responders. HPSE is active in tumor invasion and inflammatory processes [45, 46], where-as LARP1 is associated with dengue virus and coronavirus disease-19 (COVID-19) [47, 48]. Higher expression levels in nivolumab non-responders may suggest either a different inflammatory process required for anti-tumor response, or a highly progressive tumor, which may explain the insensitivity to nivolumab treatment.
In this light, the current results were unexpected. We have several explanations for our findings; 1) platelet RNA is only very subtly altered in the presence of immunotherapy-sensitive as opposed to immunotherapy-resistant NSCLC tumors for which insufficient sample numbers were available to uncover such differences. Alternatively, the employed machine learning algorithms and gene panel selection approach had insufficient discriminatory power; 2) the alterations are masked by the presence of multiple platelet subpopulations; 3) the alterations may be best measured after start of treatment, e.g. a couple of weeks following the first nivolumab exposure; 4) insufficient samples were included into the training process; 5) despite matching of several confounding factors, remaining (unmeasured) factors and/or pre-analytical variables may increase the gene expression noise as opposed to the (low-level) signal and 6) in the power calculation the estimated RNA expression difference was overestimated, resulting into a too small anticipated cohorts sample size and therefore to a reduced algorithm’s performance. Also, we cannot exclude that platelet RNA is of value as a predictive biomarker platform for other (immuno) therapeutics. Here, re-analysis of the data using other machine learning approaches is of interest and the data is publicly available for such purposes.
Acknowledgments
We are thankful to the NKI-AVL Core Facility Molecular Pathology and Biobanking (CFMPB) for supplying NKI-AVL Biobank material and lab support and Sebastiaan van de Sand (SIT B.V.) for computational resources. We also thank Maarten Slagter for his support. Financial support was provided by European Research Council E8626 (R.J.A.N., E.F.S., T.W.) and 336540 (T.W.), the Dutch Organisation of Scientific Research 93612003 and 91711366 (T.W.), the Dutch Cancer Society (T.W.), BMS IION (M.M.v.d.H., T.W.), and Stichting STOPHersentumoren.nl.
Author contributions
CONCEPTION: M.M., M.G.B, V.v.d.N, T.W., M.M.v.d.H.
DATA CURATION: A-L.N.N., N.S., R.S., L.V., K.H., K.M., J.N.H., A.J.d.L., E.Sm
ANALYSIS OF DATA: M.M., M.G.B., V.v.d.N, E.P., N.S., S.G.J.G.I.’t.V, M.S., T.N., D.v.d.B, L.W., H.G. J.N.H., H.G., E.Sc., E.Sm.,
PREPARATION OF THE MANUSCRIPT: M.M., M.G.B., V.v.d.N., T.W., M.M.v.d.H.
REVISION FOR IMPORTANT INTELLECTUAL CONTENT: D.v.d.B, K.M., L.W., H.G., J.N.H., E.Sc., E.Sm. E.J.d.L,
SUPERVISION: T.W., M.M.v.d.H.
Conflict of interest
M.M. has no conflict of interest to report; M.G.B. holds patents related to the use of platelet RNA panel for blood-based diagnostics test.; V.v.d.N. has no conflict of interest to report; J.N.H. has no conflict of interest to report; A-L.N.N. has no conflict of interest to report; E.P. has no conflict of interest to report; N.S. has no conflict of interest to report; S.G.J.G.I.t.V. has no conflict of interest to report; M.S. has no conflict of interest to report; T.N. has no conflict of interest to report; L.V. has no conflict of interest to report; R.S. has no conflict of interest to report; D.B. Reports: Roche Diagnostics. Payment or honoraria for lectures, presentations, manuscript writing or educational events. Expert testimony: Roche Diagnostics. Both paid to the institute; K.H. has no conflict of interest to report; K.M. Reports over the last 5 years: Research grants: Astra Zeneca, Speakers fee: MSD, Roche, Astra Zeneca, Benecke, consultant fee: Pfizer, BMS, Roche, MSD, Abbvie, AstraZeneca, Diaceutics, Lilly, Bayer, Boehringer Ingelheim, Merck.; A.J.d.L. received grants from BMS, MSD, Boehringer, AstraZeneca and non-financial support from Merck Serono and Roche, outside the submitted work.; E.Sc. received honoraria as consultant in advisory boards from MSD/Merck, GSK, AstraZeneca, Astellas Pharma, Roche, Pfizer, Novartis, Bayer, BMS, Lilly, Amgen, Biocartis, Illumina, Agena Bioscience, Janssen Cilag (Johnson&Johnson), Sinnovisionlab, Diaceutics, CC Diagnostics; and received research grants from Pfizer, Biocartis, Invitae-ArcherDX, AstraZeneca, Agena Bio-science, BMS, Bio-Rad, Roche, Boehringer Ingelheim, CC Diagnostics and Abbott (honoraria paid to UMCG account; all outside this study); E.Sm. has no conflict of interest to report; H.J.M.G. has no conflict of interest to report; T.W. holds patents related to the use of platelet RNA panel for blood-based diagnostics test, received funding from Illumina and is shareholders of GRAIL, Inc.; M.M.v.d.H. received research funding or honoraria from AbbVie, AstraZeneca, Bristol Myers Squibb, Eli Lilly, Janssen Pharmaceuticals, Merck & Co., Inc., Merck Sharp & Dohme, Novartis, PamGene, Pfizer, Roche, and Stichting Treatmeds. Moreover, M.M.v.d.H. is a guest editor in the special issue “Lung Cancer in Tumor Markers” but had no participation in the peer review process of this paper.
Supplementary material
[1] The supplementary material is available in the electronic version of this article: https://dx.doi.org/10.3233/TUB-220037">https://dx.doi.org/10.3233/TUB-220037.
References
[1] | Ferlay J , Soerjomataram I , Ervik M , Dikshit R , Eser S , Mathers C , et al. GLOBOCAN 2012 v1.0, Cancer Incidence and Mortality Worldwide. Vol. 11, Lyon, France: International Agency for Research on Cancer. 2013. p.http://globocan.iarc.f. |
[2] | Gibney GT , Weiner LM , Atkins MB Predictive biomarkers for checkpoint inhibitor-based immunotherapy. Vol. 17, The Lancet Oncology. 2016. p. e542–51. DOI: 10.1016/S1470-2045(16)30406-5. |
[3] | Borghaei H , Paz-Ares L , Horn L , Spigel DR , Steins M , Ready NE , et al. Nivolumab versus Docetaxel in Advanced Nonsquamous Non-Small-Cell Lung Cancer, N Engl J Med ((2015) );373: (17):1627–39. DOI: 10.1056/NEJMoa1507643. |
[4] | Brahmer J , Reckamp KL , Baas P , Crinò L , Eberhardt WEE , Poddubskaya E , et al. Nivolumab versus Docetaxel in Advanced Squamous-Cell Non-Small-Cell Lung Cancer, N Engl J Med ((2015) );373: (2):123–35. DOI: 10.1056/NEJMoa1504627. |
[5] | Rizvi NA , Hellmann MD , Snyder A , Kvistborg P , Makarov V , Havel JJ , et al. Cancer immunology, Mutational landscape determines sensitivity to PD-1 blockade in non-small cell lung cancer. Science ((2015) );348: (6230):124–8. DOI: 10.1126/science.aaa1348. |
[6] | McGranahan N , Furness AJS , Rosenthal R , Ramskov S , Lyngaa R , Saini SK , et al. Clonal neoantigens elicit T cell immunoreactivity and sensitivity to immune checkpoint blockade, Science ((2016) );351: (6280):1463–9. DOI: 10.1126/science.aaf1490. |
[7] | George JN Platelets, Lancet ((2000) );355: (9214):1531–9. DOI: 10.1016/S0140-6736(00)02175-9. |
[8] | McAllister SS , Weinberg RA The tumour-induced systemic environment as a critical regulator of cancer progression and metastasis, Nat Cell Biol ((2014) );16: (8):717–27. DOI: 10.1038/ncb3015. |
[9] | Lefrançcais E , Ortiz-Muñoz G , Caudrillier A , Mallavia B , Liu F , Sayah DM , et al. The lung is a site of platelet biogenesis and a reservoir for haematopoietic progenitors, Nature ((2017) );544: (7648):105–9. DOI: 10.1038/nature21706. |
[10] | Denis MM , Tolley ND , Bunting M , Schwertz H , Jiang H , Lindemann S , et al. Escaping the nuclear confines: signal-dependent pre-mRNA splicing in anucleate platelets, Cell ((2005) );122: (3):379–91. DOI: 10.1016/j.cell.2005.06.015. |
[11] | Schubert S , Weyrich AS , Rowley JW A tour through the transcriptional landscape of platelets, Blood ((2014) );124: (4):493–502. DOI: 10.1182/blood-2014-04-512756. |
[12] | Cecchetti L , Tolley ND , Michetti N , Bury L , Weyrich AS , Gresele P Megakaryocytes differentially sort mRNAs for matrix metalloproteinases and their inhibitors into platelets: a mechanism for regulating synthetic events, Blood ((2011) );118: (7):1903–11. DOI: 10.1182/blood-2010-12-324517. |
[13] | Rondina MT , Schwertz H , Harris ES , Kraemer BF , Campbell R a , Mackman N , et al. The septic milieu triggers expression of spliced tissue factor mRNA in human platelets, J Thromb Haemost ((2011) );9: (4):748–58. DOI: 10.1111/j.1538-7836.2011.04208.x. |
[14] | Best MG , Sol N , Kooi I , Tannous J , Westerman BA , Rustenburg F , et al. RNA-Seq of Tumor-Educated Platelets Enables Blood-Based Pan-Cancer, Multiclass, and Molecular Pathway Cancer Diagnostics, Cancer Cell ((2015) );28: (5):666–76. DOI: 10.1016/j.ccell.2015.09.018. |
[15] | Nilsson RJA , Balaj L , Hulleman E , van Rijn S , Pegtel DM , Walraven M , et al. Blood platelets contain tumor-derived RNA biomarkers, Blood ((2011) );118: (13):3680–3. DOI: 10.1182/blood-2011-03-344408. |
[16] | Kirschbaum M , Karimian G , Adelmeijer J , Giepmans BNG , Porte RJ , Lisman T Horizontal RNA transfer mediates platelet-induced hepatocyte proliferation, Blood ((2015) );126: (6):798–806. DOI: 10.1182/blood-2014-09-600312. |
[17] | Michael J V , Wurtzel JGT , Mao GF , Rao AK , Kolpakov MA , Sabri A , et al. Platelet microparticles infiltrating solid tumors transfer miRNAs that suppress tumor growth. Blood. 2017;blood-2016-11-751099. DOI: 10.1182/blood-2016-11-751099. |
[18] | Labelle M , Begum S , Hynes RO Direct signaling between platelets and cancer cells induces an epithelial-mesenchymal-like transition and promotes metastasis, Cancer Cell ((2011) );20: (5):576–90. DOI: 10.1016/j.ccr.2011.09.009. |
[19] | Hugo W , Zaretsky JM , Sun L , Song C , Moreno BH , Hu-Lieskovan S , et al. Genomic and Transcriptomic Features of Response to Anti-PD-1 Therapyin Metastatic Melanoma, Cell ((2016) );165: (1):35–44. DOI: 10.1016/j.cell.2016.02.065. |
[20] | Boilard E , Nigrovic PA , Larabee K , Watts GFM , Coblyn JS , Weinblatt ME , et al. Platelets amplify inflammation in arthritis via collagen-dependent microparticle production, Science ((2010) );327: (5965):580–3. DOI: 10.1126/science.1181928. |
[21] | Zamora C , Cantó E , Nieto JC , Bardina J , Diaz-Torné C , Moya P , et al. Binding of Platelets to Lymphocytes: A Potential Anti-Inflammatory Therapy in Rheumatoid Arthritis, J Immunol ((2017) );198: (8):3099–108. DOI: 10.4049/jimmunol.1601708. |
[22] | Schumacher D , Strilic B , Sivaraj KK , Wettschureck N , Offermanns S Platelet-derived nucleotides promote tumor-cell transendothelial migration and metastasis via P2Y2 receptor, Cancer Cell ((2013) );24: (1):130–7. DOI: 10.1016/j.ccr.2013.05.008. |
[23] | Labelle M , Begum S , Hynes RO Platelets guide the formation of early metastatic niches, Proc Natl Acad Sci U S A ((2014) );111: (30):E3053–61. DOI: 10.1073/pnas.1411082111. |
[24] | Li N Platelet-lymphocyte cross-talk, J Leukoc Biol ((2008) );83: (5):1069–78. DOI: 10.1189/jlb.0907615. |
[25] | Rossaint J , Kühne K , Skupski J , Van Aken H , Looney MR , Hidalgo A , et al. Directed transport of neutrophil-derived extracellular vesicles enables platelet-mediated innate immune response, Nat Commun ((2016) );7: :13464. DOI: 10.1038/ncomms13464. |
[26] | Wang C , Sun W , Ye Y , Hu Q , Bomba HN , Gu Z In situ activation of platelets with checkpoint inhibitors for post-surgical cancer immunotherapy, Nat Biomed Eng ((2017) );1: (2):0011. DOI: 10.1038/s41551-016-0011. |
[27] | Diem S , Schmid S , Krapf M , Flatz L , Born D , Jochum W ,et al. Neutrophil-to-Lymphocyte ratio (NLR) and Platelet-to-Lymphocyte ratio (PLR) as prognostic markers in patients with non-small cell lung cancer (NSCLC) treated with nivolumab, Lung Cancer ((2017) );111: :176–81. DOI: 10.1016/j.lungcan.2017.07.024. |
[28] | In ’t Veld SGJG , Arkani M , Post E , Antunes-Ferreira M , D’Ambrosi S , Vessies DCL , et al. Detection and localization of early- and late-stage cancers using platelet RNA, Cancer Cell ((2022) );40: (9):999–1009.e6. DOI: 10.1016/J.CCELL.2022.08.006. |
[29] | Nilsson RJA , Karachaliou N , Berenguer J , Gimenez-Capitan A , Schellen P , Teixido C , et al. Rearranged EML4-ALK fusion transcripts sequester in circulating blood platelets and enable blood-based crizotinib response monitoring in non-small-cell lung cancer, Oncotarget ((2015) );7: (1):1066–75. DOI: 10.18632/oncotarget.6279. |
[30] | Sol N , in ‘t Veld SGJG , Vancura A , Tjerkstra M , Leurs C , Rustenburg F , et al. Tumor-Educated Platelet RNA for the Detection and (Pseudo)progression Monitoring of Glioblastoma, Cell Reports Med.01 ((2020) );1: (7):100101. DOI: 10.1016/j.xcrm.2020.100101. |
[31] | Dobbin KK , Simon RM Sample size planning for developing classifiers using high-dimensional DNA microarray data, Biostatistics ((2007) );8: (1):101–17. DOI: 10.1093/BIOSTATISTICS/KXJ036. |
[32] | Rizvi NA , Mazières J , Planchard D , Stinchcombe TE , Dy GK , Antonia SJ , et al. Activity and safety of nivolumab, an anti-PD-1 immune checkpoint inhibitor, for patients with advanced, refractory squamous non-small-cell lung cancer (CheckMate 063): a phase 2, single-arm trial, Lancet Oncol ((2015) );16: (3):257–65. DOI: 10.1016/S1470-2045(15)70054-9. |
[33] | Planchard D , Popat S , Kerr K , Novello S , Smit EF , Faivre-Finn C , et al. Metastatic non-small cell lung cancer: ESMO Clinical Practice Guidelines for diagnosis, treatment and follow-up, Ann Oncol Off J Eur Soc Med Oncol ((2018) );29: (Suppl 4):iv192–237. DOI: 10.1093/annonc/mdy275. |
[34] | Eisenhauer EA , Therasse P , Bogaerts J , Schwartz LH , Sargent D , Ford R , et al. New response evaluation criteria in solid tumours: Revised RECIST guideline (version 1.1), Eur J Cancer ((2009) );45: (2):228–47. DOI: 10.1016/j.ejca.2008.10.026. |
[35] | Schwartz LH , Litière S , de Vries E , Ford R , Gwyther S , Mandrekar S , et al. RECIST 1.1-Update and clarification: From the RECIST committee, Eur J Cancer ((2016) );62: ;132–7. DOI: 10.1016/j.ejca.2016.03.081. |
[36] | Sidorenkov G , Nagel J , Meijer C , Duker JJ , Groen HJM , Halmos GB , et al. The OncoLifeS data-biobank for oncology: a comprehensive repository of clinical data, biological samples, and the patient’s perspective, J Transl Med. ((2019) );17: (1). DOI: 10.1186/S12967-019-2122-X. |
[37] | Best MG , In ’t Veld SGJG , Sol N , Wurdinger T RNA sequencing and swarm intelligence–enhanced classification algorithm development for blood-based disease diagnostics using spliced blood platelet RNA, Nat Protoc ((2019) );14: (4):1206–34. DOI: 10.1038/s41596-019-0139-5. |
[38] | Bolger AM , Lohse M , Usadel B Trimmomatic: a flexible trimmer for Illumina sequence data, Bioinformatics ((2014) );30: (15):2114–20. DOI: 10.1093/bioinformatics/btu170. |
[39] | Dobin A , Davis CA , Schlesinger F , Drenkow J , Zaleski C , Jha S , et al. STAR: ultrafast universal RNA-seq aligner, Bioinformatics ((2013) );29: (1):15–21. DOI: 10.1093/bioinformatics/bts635. |
[40] | Anders S , Pyl PT , Huber W HTSeq - A Python framework to work with high-throughput sequencing data, Bioinformatics ((2014) );31: (2):166–9. DOI: 10.1093/bioinformatics/btu638. |
[41] | Best MG , Sol N , In ‘t Veld SGJG , Vancura A , Muller M , Niemeijer ALN , et al. Swarm Intelligence-Enhanced Detection of Non-Small-Cell Lung Cancer Using Tumor-Educated Platelets, Cancer Cell ((2017) );32: (2):238–52. DOI: 10.1016/j.ccell.2017.07.004. |
[42] | GSEA Human Gene Set: HALLMARK INFLAMMATORY RESPONSE Available from: http://www.gseamsigdb.org/gsea/msigdb/human/geneset/HALLMARK_INFLAMMATORY_RESPONSE.html. Last accessed on November 2, 2022. |
[43] | Hofman P , Heeke S , Alix-Panabières C , Pantel K Liquid biopsy in the era of immuno-oncology: is it ready for prime-time use for cancer patients? Ann Oncol ((2019) );30: (9):1448–59. DOI: 10.1093/annonc/mdz196. |
[44] | Kerr B a , McCabe NP , Feng W , Byzova T V Platelets govern pre-metastatic tumor communication to bone, Oncogene ((2013) );32: (36):4319–24. DOI: 10.1038/onc.2012.447. |
[45] | Ostrovsky O , Vlodavsky I , Nagler A Mechanism of HPSE Gene SNPs Function: From Normal Processes to Inflammation, Cancerogenesis and Tumor Progression, Adv Exp Med Biol ((2020) );1221: :231–49. DOI: 10.1007/978-3-030-34521-1_8. |
[46] | GeneCards HPSE [Internet]. Available from: https://www.genecards.org/cgi-bin/carddisp.pl?gene=HPSE. Last accessed on November 2, 2022. |
[47] | Schwenzer H , Abdel Mouti M , Neubert P , Morris J , Stockton J , Bonham S , et al. LARP1 isoform expression in human cancer cell lines, RNA Biol ((2021) );18: (2):237–47. DOI: 10.1080/15476286.2020.1744320. |
[48] | GeneCards LARP1 Available from: https://www.genecards.org/cgi-bin/carddisp.pl?gene=LARP1. Last accessed on November 2, 2022. |