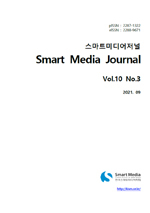
Finding the best suited autoencoder for reducing model complexity
- 한국스마트미디어학회
- 스마트미디어저널
- Vol10, No.3
- 등재여부 : KCI등재후보
- 2021.09
- 9 - 22 (14 pages)
Basically, machine learning models use input data to produce results. Sometimes, the input data is too complicated for the models to learn useful patterns. Therefore, feature engineering is a crucial data preprocessing step for constructing a proper feature set to improve the performance of such models. One of the most efficient methods for automating feature engineering is the autoencoder, which transforms the data from its original space into a latent space. However certain factors, including the datasets, the machine learning models, and the number of dimensions of the latent space (denoted by k), should be carefully considered when using the autoencoder. In this study, we design a framework to compare two data preprocessing approaches: with and without autoencoder and to observe the impact of these factors on autoencoder. We then conduct experiments using autoencoders with classifiers on popular datasets. The empirical results provide a perspective regarding the best suited autoencoder for these factors.
Ⅰ. INTRODUCTION
Ⅱ. AUTOENCODER
Ⅲ. CLASSIFIERS
Ⅳ. METHODOLOGY
Ⅴ. EXPERIMENTS
Ⅵ. SUGGESTIONS
Ⅶ. CONCLUSIONS
Ⅷ. REFERENCES