A nomogram for predicting death for infants born at a gestational age of <28 weeks: a population-based analysis in 18 neonatal intensive care units in northern China
Highlight box
Key findings
• A combination of seven variables (birth weight, being inborn, chest compression in the delivery room, respiratory distress syndrome, pulmonary hemorrhage, invasive mechanical ventilation, and shock) is valuable for the prediction of mortality in extremely preterm infants (EPIs) receiving complete care in northern China.
What is known and what is new?
• Data regarding mortality of EPIs are valuable but the prognosis of EPIs in China cannot be predicted using existing prediction models due to great variations in conditions.
• This study retrospectively analyzed data of EPIs receiving complete care from multiple centers in northern China and provided a predictive model with a favorable discrimination capability, accuracy, and clinical utility.
What is the implication, and what should change now?
• Our findings might help clinicians identify EPIs with risk of poor prognoses early.
Introduction
Background
Extremely preterm infants (EPIs) are those born at a gestational age (GA) of less than 28 weeks. Due to their extremely immature organs, EPIs have a high risk of death, severe complications, and long-term neurological sequelae (1). China, as a populous country, has the second highest number of preterm infants globally. Since the introduction of the two-/three-child policies, the proportion of pregnancies with advanced maternal age and/or using assisted reproductive technology has increased, leading to the occurrence of extremely preterm births (2). With the rapid development of the social economy and medical technology, the number of EPIs receiving treatment in China is increasing, and this has helped increase their survival rates. Despite the progress made, there is still a large gap between the medical management strategies in China and those in developed countries (3-6). A cohort study on very low birth weight infants (VLBWIs), including 88,327 neonates (in ten developed countries, 2007–2013) with GA of 24–29 weeks, showed an average survival rate of 87% among neonates (7). Multiple studies regarding the outcomes of EPIs in China revealed that there was high in-hospital mortality and a high proportion of treatment abandonment in this cohort (4,6,8-10). The reasons for treatment abandonment are usually financial concerns or the fear of adverse outcomes (9,11). Treating EPIs requires higher medical resource allocation and treatment costs, regardless of a country’s level of development (12,13). In China, families with premature infants encounter comprehensive hospital care costs, encompassing both out-of-pocket expenditures and reimbursements from basic medical insurance (13). Furthermore, it is imperative for newborns to undergo the household registration procedure—a process that usually spans over several months—to become eligible for basic medical insurance reimbursement. Consequently, parents are required to pay the medical charges out-of-pocket during hospitalization and subsequently seek reimbursement once the infants are insured upon discharge. This means that Chinese families with EPIs face a heavy financial burden when they are first hospitalized.
Rationale and knowledge gap
Data regarding mortality and the regional therapeutic experience with EPIs are valuable to parents who need to be consulted about prognosis and involved in decision making. Multiple neonatal networks have attempted to construct predictive models for infant mortality rates. Tyson et al. (14) used birth weight (BW), sex, antenatal corticosteroid (ANS), and singleton or twin pregnancies as prognostic indicators (including death and severe unfavorable neurological outcomes) for EPIs at 22–25 weeks of gestation. Bader et al. (15) used GA, BW percentile by sex, ANS, and multiple pregnancies as indicators for predicting mortality in EPIs with a GA of 23–26 weeks. Moreira et al. (16) used three variables: BW, Apgar score at 5 minutes of age, and GA as indicators to predict mortality in EPIs with GA <28 weeks. These findings suggest that factors affecting mortality in preterm infants vary widely across regions. The prognosis of EPIs in China cannot be predicted using existing prediction models due to the great variations in conditions. Besides, there are large variations in the level of economic development and medical care in different regions of China, which lead to significant differences in outcomes and levels of care for EPIs among regions (17). Studies on the predictions of in-hospital mortality of EPIs have not been available in northern China.
Objective
Therefore, this study intended to identify predictors of neonatal in-hospital mortality among neonates who received complete treatment in northern China in recent years. We present this article in accordance with the TRIPOD reporting checklist (available at https://tp.amegroups.com/article/view/10.21037/tp-23-337/rc).
Methods
Study design
This study involved 18 neonatal intensive care units (NICUs) from four northern Chinese cities and provinces (Beijing, Hebei, Tianjin, and Inner Mongolia), of which seventeen were general hospitals or maternity and children’s hospitals with obstetric departments, and one was an independent children’s hospital that only admits transferred neonates. EPIs admitted to the hospitals involved in the research from January 1, 2015, to December 31, 2018, were included. The study was conducted in accordance with the Declaration of Helsinki (as revised in 2013), and approved by Peking University First Hospital’s ethics committee (No. 2019–21) with no informed consent required due to the retrospective nature of the study. The other 17 hospitals were informed and agreed with the study. A cooperation agreement was signed by all the institutions involved.
Patient selection criteria and definitions
EPIs (infants with a GA <28 weeks) admitted to the research hospitals within 72 h after birth were eligible; those who had severe congenital deformities (e.g., congenital genetic metabolic disease or complex cardiovascular malformations), with incomplete patient information or whose parents gave up any life-sustaining treatment were excluded.
The included EPIs were followed up from birth to discharge, and the outcomes included death, discharge, or transfer. Factors that might affect the prognosis of EPIs, including the pregnancy condition, neonatal resuscitation, preterm complications, and interventions during hospitalization, were included.
Infants born and admitted to the same hospital were classified as inborn. GA was determined according to the best obstetric indicators, including last menstruation, obstetric variables, prenatal ultrasound, or a combination of the three. Small-for-gestational-age (SGA) referred to infants with a BW below the tenth percentile based on their sex and GA (18). The modified Bell classification was used to diagnose and grade necrotizing enterocolitis (NEC) (19). The diagnosis of periventricular leukomalacia (PVL) was confirmed with imaging examinations such as magnetic resonance imaging or cranial ultrasonography (20). Intraventricular hemorrhage (IVH) was diagnosed by referring to the Papile criteria, and grade III or a higher degree indicated severe IVH (21). The diagnosis of neonatal sepsis required the identification of pathogenic bacteria in blood or cerebrospinal fluid (CSF) cultures (22). If the cultures were tested negative, the clinical diagnosis of sepsis required the presence of aberrant manifestations and at least one of the following criteria: (I) ≥2 positive results in the non-specific blood test; or (II) CSF tests suggesting bacterial meningitis. Shock was defined as inadequate oxygen supply to vital organs resulting from circulatory failure that manifested as hypotension, oliguria, acidosis, and prolonged capillary refill time (23). Hypothermia was indicated by axillary temperature <36 ℃ within 1 h after admission. Respiratory distress syndrome (RDS) referred to respiratory distress characterized by classical clinical and imaging manifestations, including low-volume lungs with a diffuse reticulogranular pattern and air bronchogram. Patients presenting with lesions in the bilateral lungs leading to a blurred heart border, diaphragm, or white lung were considered to have severe RDS (24).
Pregnancy complications included chorioamnionitis, gestational diabetes, gestational hypertension, and premature rupture of the membrane (PROM). The diagnosis of the diseases described above was based on the national guidelines and international literatures (25-27). ANS was defined as any use of ANSs prior to birth to help accelerate fetal lung maturity. Complete ANS referred to the completion of ≥1 course of ANS (28).
Data collection and quality control
In this study, an Epidata database was devised using Epidata 3.02, and the data were then imported into SPSS 22.0 (IBM Corp., Armonk, NY, USA) for analysis. Eighteen physicians were selected from each institution to undergo training and were responsible for the data collection, entry, and review at their institution. Data were stored, analyzed, and summarized at the host hospital. Measures such as carefully designing the data extraction tool, regularly training staff for data entry, and arranging supervisors were taken to ensure data quality. Missing data entries were filled using multiple imputation.
Statistical analysis and development of the nomogram
Perinatal risk factors associated with in-hospital mortality were described using either the median [interquartile range] or rate. The training dataset that was used as an example of the model and the validation dataset that was used to check the performance of the model were randomly assigned with a ratio of 7:3. Risk factors for death were screened in the training dataset using a univariate Cox regression analysis. Factors with statistical significance were included in the stepwise multiple regression analysis (bidirectional elimination) to select the predictive factors and to construct the best-fitting model for predicting in-hospital mortality with smallest Akaike information criterion (AIC). A nomogram was plotted using R 3.4.1, and complex regression equations were transformed into a simple diagram, making the predictive model more readable. The nomogram assigned a score to every factor and inferred mortality by summing the scores of each factor.
Validation of the nomogram
An area under the receiver operating curve (AUROC) was employed to evaluate the discrimination ability of the model. A calibration curve combined with the Hosmer-Lemeshow (HL) test was employed to assess the calibration ability. A decision curve analysis (DCA) was employed to assess the clinical effectiveness of the model.
Results
Patient attributes
A total of 803 cases met the inclusion criteria, with one case being excluded because of incomplete data and 234 cases being excluded because the parents gave up life-sustaining treatment during hospitalization (Figure 1).
Among the included 568 EPIs, 465 (81.9%) survived to discharge, 75 (13.2%) died, and 28 (4.9%) were transferred with indefinite outcomes. The median time to death was 2.0 [0.9, 5.0] days; 77.3% of deaths occurred within 7 days of birth, of which 25.3%, 28.0%, and 24.0% infants died at <24 h, 1–<3 days, and 3–<7 days, respectively, whereas 22.7% died at ≥7 days of birth. The discharge status of EPIs from different GA and BW subgroups is described in Figure 2. With an increase in GA and BW, the survival rate of EPIs gradually improved, and the proportion of deaths gradually decreased.
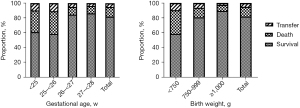
The maternal characteristics, neonatal characteristics, morbidities, and interventions of the EPIs are summarized in Table 1 and Table 2. Among the 568 EPIs, the median GA and BW were 27.1 [26.3, 27.6] weeks and 990.0 [860.0, 1,100.0] g, respectively. A total of 56.2% (319/568) were male infants, 34.0% (193/568) comprised multiple births, and 1.4% (8/568) were SGA. The Apgar scores were 8 [7, 9] at 5 min.
Table 1
Variables | Overall (n=568) | Training (n=398) | Validation (n=170) | P |
---|---|---|---|---|
Maternal characteristics | ||||
Age (years), median [IQR] | 32.0 [29.0, 36.0] | 32.0 [29.0, 35.0] | 32.0 [29.0, 35.0] | 0.869 |
Pregnancy complications, n (%) | 406 (71.5) | 293 (73.6) | 113 (66.5) | 0.104 |
ANS, n (%) | 341 (60.0) | 239 (60.1) | 102 (60.0) | 1.000 |
Cesarean delivery, n (%) | 128 (22.5) | 90 (22.6) | 38 (22.4) | 1.000 |
Chorioamnionitis and/or PROM, n (%) | 287 (50.5) | 209 (52.5) | 78 (45.9) | 0.175 |
Neonatal characteristics, n (%) | ||||
GA | 0.197 | |||
<25 w | 31 (5.5) | 20 (5.0) | 11 (6.5) | |
25–<26 w | 56 (9.9) | 33 (8.3) | 23 (13.5) | |
26–<27 w | 143 (25.2) | 105 (26.4) | 38 (22.4) | |
27–<28 w | 338 (59.5) | 240 (60.3) | 98 (57.6) | |
BW | 0.878 | |||
<750 g | 63 (11.1) | 43 (10.8) | 20 (11.8) | |
750–999 g | 276 (48.6) | 196 (49.2) | 80 (47.1) | |
≥1,000 g | 229 (40.3) | 159 (39.9) | 70 (41.2) | |
Male | 319 (56.2) | 223 (56.0) | 96 (56.5) | 0.996 |
Twin or multiple pregnancy | 193 (34.0) | 128 (32.2) | 65 (38.2) | 0.193 |
SGA | 8 (1.4) | 7 (1.8) | 1 (0.6) | 0.487 |
Inborn | 491 (86.4) | 347 (87.2) | 144 (84.7) | 0.511 |
IQR, interquartile range; ANS, antenatal steroid; PROM, premature rupture of membranes; GA, gestational age; w, weeks; BW, birth weight; SGA, small for gestational age.
Table 2
Variables | Overall (n=568) | Training (n=398) | Validation (n=170) | P |
---|---|---|---|---|
Resuscitation in DR | ||||
CPAP, n (%) | 128 (22.5) | 97 (24.4) | 31 (18.2) | 0.135 |
Intubation, n (%) | 359 (63.2) | 253 (63.6) | 106 (62.4) | 0.857 |
Chest compression, n (%) | 54 (9.5) | 40 (10.1) | 14 (8.2) | 0.785 |
Epinephrine, n (%) | 41 (7.2) | 30 (7.5) | 11 (6.5) | 0.935 |
5-min Apgar, median [IQR] | 8 [7, 9] | 8 [7, 9] | 8 [7, 9] | 0.220 |
Morbidities and interventions, n (%) | ||||
Hypothermia | 118 (20.8) | 83 (20.9) | 35 (20.6) | 1.000 |
Weight loss >7% | 329 (57.9) | 228 (57.3) | 101 (59.4) | 0.706 |
Severe RDS | 163 (28.7) | 109 (27.4) | 54 (31.8) | 0.340 |
≥2 doses of PS | 108 (19.0) | 72 (18.1) | 36 (21.2) | 0.458 |
Invasive MV | 333 (58.6) | 233 (58.5) | 100 (58.8) | 1.000 |
Pulmonary hemorrhage | 78 (13.7) | 51 (12.8) | 27 (15.9) | 0.401 |
NEC stage II–III | 26 (4.6) | 19 (4.8) | 7 (4.1) | 0.902 |
Shock | 59 (10.4) | 41 (10.3) | 18 (10.6) | 1.000 |
Sepsis | 85 (15.0) | 55 (13.8) | 30 (17.6) | 0.297 |
Severe IVH or PVL | 99 (17.4) | 70 (17.6) | 29 (17.1) | 0.975 |
DR, delivery room; CPAP, continuous positive airway pressure; IQR, interquartile range; RDS, respiratory distress syndrome; PS, pulmonary surfactant; MV, mechanical ventilation; NEC, necrotizing enterocolitis; IVH, intraventricular hemorrhage; PVL, periventricular leukomalacia.
Clinical characteristics of EPIs with complete and incomplete care
Among EPIs receiving incomplete care (treatment abandoned by the parents before discharge), those who were discharged with unstable vital signs (requiring invasive/non-invasive mechanical ventilation (MV), vasoactive drug infusion, or lack of adequate feeding) were predicted to die, while those with relatively stable vital signs were predicted to have with indefinite outcomes. Among the 234 EPIs with incomplete care, 133 (56.8%) were suspected to be dead. The clinical characteristics of EPIs with complete and incomplete care are compared in Table S1 and Table S2. The EPIs who received incomplete care had a lower percentage of ANS, lower 5-min Apgar score, and higher percentage of invasive MV and pulmonary hemorrhage (all P<0.05; Table S1). Comparing the clinical characteristics of EPIs that died at different time points (<7 vs. ≥7 days) and from different groups (complete care group vs. incomplete care group), the EPIs who died at <7 days in the complete care group had a higher incidence of pulmonary hemorrhage (48.3% vs. 28.9%, P<0.05), whereas among those who died at ≥7 days, the incomplete care group had a higher incidence of severe IVH or PVL (53.7% vs. 23.5%, P<0.05, Table S2).
Univariate and multivariate analysis of prescient variables
EPIs were assigned to the training and validation datasets at random in a ratio of 7:3. No significant differences were identified in maternal and neonatal characteristics and in-hospital data of EPIs between the two datasets (all P>0.05, Table 1 and Table 2).
In the training dataset, univariate Cox regression analysis suggested that GA, BW, SGA, being inborn, chest compression in the delivery room (DR), adrenaline administration in the DR, hypothermia, severe RDS, ≥2 doses of pulmonary surfactant administration, invasive MV, pulmonary hemorrhage, NEC stages II–III, and shock were associated with the risk of in-hospital death among EPIs (P<0.05, Table 3). Multiple regression suggested that BW <750 g [hazard ratio (HR), 1.168; 95% confidence interval (CI): 1.052–1.297; P=0.004], pulmonary hemorrhage (HR, 1.262; 95% CI: 1.145–1.391; P<0.001), being inborn (HR, 0.877; 95% CI: 0.802–0.959; P=0.004), severe RDS (HR, 1.076; 95% CI: 1.003–1.154; P=0.043), invasive ventilation (HR, 1.083; 95% CI: 1.012–1.159, P=0.021), and shock (HR, 1.181; 95% CI: 1.068–1.306; P=0.001) were independent factors (P<0.05) associated with in-hospital death (Table 3).
Table 3
Variables and category | Univariate Cox regression | Multiple regression | |||
---|---|---|---|---|---|
HR (95% CI) | P | HR (95% CI) | P | ||
Maternal characteristics | |||||
Age | 1.001 (0.994, 1.009) | 0.718 | – | – | |
Pregnancy complications | 0.965 (0.894, 1.042) | 0.362 | – | – | |
ANS | 0.945 (0.882, 1.012) | 0.105 | – | – | |
Cesarean delivery | 1.026 (0.947, 1.112) | 0.533 | – | – | |
Chorioamnionitis and/or PROM | 0.986 (0.922, 1.055) | 0.692 | – | – | |
Infant characteristics | |||||
GA | |||||
<25 w | 1.162 (0.998, 1.353) | 0.054 | – | – | |
25–<26 w | 1.342 (1.188, 1.515) | <0.001 | – | – | |
26–<27 w | 1.014 (0.940, 1.095) | 0.715 | – | – | |
27–<28 w | Ref | – | – | – | |
BW | |||||
<750 g | 1.298 (1.159, 1.453) | <0.001 | 1.168 (1.052, 1.297) | 0.004 | |
750–999 g | 1.040 (0.970, 1.116) | 0.270 | 0.999 (0.936, 1.066) | 0.983 | |
≥1,000 g | Ref | – | Ref | – | |
Male | 1.013 (0.946, 1.084) | 0.712 | – | – | |
Twin or multiple pregnancy | 0.996 (0.926, 1.070) | 0.909 | – | – | |
SGA | 1.558 (1.210, 2.007) | 0.001 | – | – | |
Inborn | 0.813 (0.738, 0.895) | <0.001 | 0.877 (0.802, 0.959) | 0.004 | |
Resuscitation in the DR | |||||
CPAP | 0.945 (0.874, 1.022) | 0.157 | – | – | |
Intubation | 1.052 (0.981, 1.128) | 0.156 | – | – | |
Chest compression | 1.200 (1.075, 1.341) | 0.001 | 1.097 (0.991, 1.215) | 0.074 | |
Epinephrine | 1.238 (1.092, 1.405) | 0.001 | – | – | |
5-min Apgar | 0.992 (0.971, 1.014) | 0.482 | – | – | |
Morbidities and Interventions | |||||
Hypothermia | 1.125 (1.036, 1.221) | 0.005 | – | – | |
Weight loss >7% | 0.990 (0.925, 1.060) | 0.783 | – | – | |
Severe RDS | 1.167 (1.083, 1.257) | <0.001 | 1.076 (1.003, 1.154) | 0.043 | |
≥2 doses of PS | 1.111 (1.019, 1.212) | 0.018 | – | – | |
Invasive MV | 1.222 (1.145, 1.305) | <0.001 | 1.083 (1.012, 1.159) | 0.021 | |
Pulmonary hemorrhage | 1.436 (1.306, 1.578) | <0.001 | 1.262 (1.145, 1.391) | <0.001 | |
NEC stage II–III | 1.208 (1.033, 1.414) | 0.019 | – | – | |
Shock | 1.328 (1.193, 1.479) | <0.001 | 1.181 (1.068, 1.306) | 0.001 | |
Sepsis | 0.949 (0.867, 1.040) | 0.265 | – | – | |
Severe IVH or PVL | 0.941 (0.861, 1.028) | 0.179 | – | – |
HR, hazard ratio; CI, confidence interval; ANS, antenatal steroid; PROM, premature rupture of membranes; GA, gestational age; w, weeks; Ref, reference; BW, birth weight; SGA, small for gestational age; DR, delivery room; CPAP, continuous positive airway pressure; RDS, respiratory distress syndrome; PS, pulmonary surfactant; MV, mechanical ventilation; NEC, necrotizing enterocolitis; IVH, intraventricular hemorrhage; PVL, periventricular leukomalacia.
Construction of the nomogram predicting the risk of in-hospital mortality among EPIs
The variables included in the predictive model were BW, being inborn, chest compression in the DR, severe RDS, invasive MV, pulmonary hemorrhage, and shock. To make it easier for clinicians to understand, a nomogram was plotted using factors selected by the multivariate regression analysis to predict in-hospital mortality among the EPIs (Figure 3).
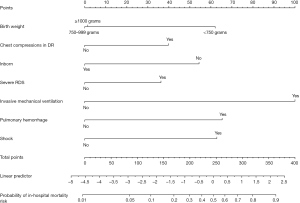
Validation of the nomogram
The validation of the nomogram is shown in Figure 4. ROC curves were adopted to evaluate the accuracy of the model, which was estimated to be 0.863 (95% CI: 0.813–0.914) and 0.886 (95% CI: 0.827–0.945) for in-hospital mortality prediction in the training and validation datasets, respectively, suggesting good discrimination of the model (Figure 4A,4D). The negative predictive value (NPV) and positive predictive value (PPV) were 0.962 and 0.383, respectively, for the training dataset and 1.0 and 0.288, respectively, for the validation dataset. The calibration plot in both datasets showed that the predicted values were in agreement with the actual values, indicating satisfactory accuracy of the model (Figure 4B,4E). DCA was used in both datasets to test the clinical usefulness of the model, demonstrating that positive net benefits were shown when the threshold was higher than 0.6 both in the training and validation datasets (Figure 4C,4F).
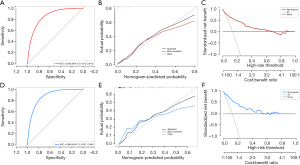
Discussion
EPIs are at high risk of mortality and long-term morbidity including respiratory, gastrointestinal complications and neurodevelopmental impairments in China. Early identification of the risk of death is an essential and controversial issue regarding EPIs. In this study, 568 EPIs from eighteen NICUs were included from 2015 to 2018 in northern China, and risk factors for in-hospital mortality were analyzed. To our knowledge, this is the first multicenter clinical study to identify predictors of in-hospital death among EPIs in northern China, which is valuable for family consultation and early identification of infants at high risk of mortality. In this study, the proportions of EPIs’ survival to discharge and in-hospital mortality were 81.9% and 13.2%, respectively. Compared to the results of a study conducted by Cao et al. (10) in which the outcomes of 9,952 cases of very preterm infants (VPIs) from 57 tertiary hospitals in China from January to December 2019 were analyzed, we reported a close but a slightly lower survival rate for EPIs with GA of 26–27 weeks (89.0% vs. 85.9%) receiving complete care.
Several risk factors associated with in-hospital mortality were identified, and a predictive model for in-hospital mortality was constructed. BW, being inborn, chest compression in the DR, MV, severe RDS, pulmonary hemorrhage, and shock were used to plot a nomogram model. It was shown that the nomogram had a favorable discrimination capability, accuracy, and clinical utility according to statistical indicators. By combining the scores from each prognostic factor of the EPIs in the nomogram, doctors can determine the likelihood of in-hospital mortality, and therefore, should pay more attention to those with a high risk. Compared with prediction models that only incorporate prenatal and birth information, or data in the first several hours or days after NICU admission, this model incorporated extensive information combining multicenter data in northern China and included indicators that might affect the prognosis of EPI, including the pregnancy condition, neonatal resuscitation, complications, and interventions during hospitalization, making it more precise for use in the clinical settings (14-16,29-31). Sheikhtaheri et al. (32) developed a prediction model of neonatal deaths in the NICU with a combination of a machine learning algorithm and seventeen variables, which is more complex.
Among the factors affecting the mortality rate in EPIs, MV during hospitalization showed the greatest effect. This may be related to the fact that EPIs requiring invasive MV suffer from more critical conditions and complications due to volume and pressure damage, resulting in higher mortality rates and incidence of impairments such as pneumonia, air leaks, bronchopulmonary dysplasia, and severe IVH (33). It was reported that the modes of MV were important for reducing damage in EPIs, with many studies recommending conventional MV with volume-targeted ventilation and high-frequency oscillatory ventilation as the first alternative strategies (34,35). The 2022 European Consensus Guidelines recommend early extubation for preterm infants if it is judged clinically safe, and the use of a relatively high nasal intermittent positive pressure or continuous positive airway pressure ventilation mode may improve the success rate of weaning MV (35,36). Japanese neonatologists believe that for extremely immature EPIs, the timing of extubation and transition to non-invasive ventilation may usually be 28–32 weeks of corrected GA (37).
This study supports that BW <750 g serves as a risk factor for in-hospital mortality. GA had no contribution to this model, which might be due to the limitation that EPIs included in this study had a GA within a narrow range and the proportion of premature infants with extremely low GA was small. Being inborn is one of the protective prognostic determinants for in-hospital mortality. Previous study has shown that inborn preterm infants have a lower mortality and incidence of complications than outborn infants (38). A key point, according to Japan’s successful treatment experience, is a low neonatal transfer rate (39). Conversely, another study also found higher mortality rates among inborn infants, possibly because outborn infants were usually, relatively stable, at the time of referral (40).
Chest compression in the DR was one of the prognostic determinants in the predictive model, as also reported in previous studies (41,42). Fischer et al. (41) included 2,068 preterm infants with GA <29 weeks from the Canadian Neonatal Network, 190 of whom received cardiopulmonary resuscitation in the DR (DR-CPR). The results showed that DR-CPR was associated with higher mortality and neurological impairment. A meta-analysis in 2009 showed that DR-CPR was an independent risk factor for higher mortality [odds ratio (OR), 2.83, 95% CI: 1.92–4.16], but there is limited evidence about the long-term outcome of survivors (43).
Severe RDS was a decisive factor for prognosis in this study. Patel et al. (44) reported that RDS was the leading cause of in-hospital death among EPIs with a GA of 24–27 weeks. Another predictive models also reported RDS as a critical indicator for the prognoses of preterm infants, especially in underdeveloped countries (45). Pulmonary hemorrhage and shock are prognostic determinants. According to previous studies, the systemic arterial resistance of infants surges after birth and disconnection from the placenta, and immature cardiac muscles have a limited response capability toward increased preload and afterload, especially among EPIs, which may lead to hemorrhagic complications including pulmonary hemorrhage and IVH (39,46,47). Scroll et al. (48) reported that pulmonary hemorrhage was related to patent ductus arteriosus (PDA), early extubation, and the absence of prophylactic indomethacin administration. Shock was reported to be a strong predictor of mortality of VLBW infants in another study (relative risk, 3.24); however, the specific definition of shock was not mentioned in that study (49).
The advantage of this study is that it is the first large-scale, multicenter clinical study regarding the mortality predictive model of EPIs receiving complete care in northern China. Under the unequal distribution of medical resources in China, large-scale, multicenter, epidemiological investigations with uniform standards are beneficial for raising awareness of the prognosis of EPIs among the general population and medical workers. We collected detailed and complete information, which is conducive to further exploring the main factors affecting the mortality of EPIs. Seventeen of the research hospitals were tertiary hospitals with level III NICUs, and one was a comprehensive private hospital with a level III NICU; all of them had good treatment capacities and well reflected the health care level of this region.
Some limitations of this study are that it was a retrospective study. The study only included the data of EPIs who received neonatal resuscitation and were transferred to the NICU. Infants who died in the DR (did not receive resuscitation or with resuscitation failed), who were transferred to the hospital after 72 h of birth, or whose parents abandoned treatment during hospitalization were not included, which might have contributed to the distribution bias of GA. The predictive model in this study included mortality during hospitalization as an outcome, whereas death after discharge or other complications that might impact long-term prognosis were not included as outcomes. There was a correlation between two risk factors (severe RDS and MV) included in the predictive model, and statistical methods showed that simultaneous inclusion was better for the discrimination ability of the model. Since these two indicators were crucial for the prognosis of EPIs and their inclusion did not conflict with the purpose of our study, we finally included both indicators simultaneously. The number of EPIs with GA <25 weeks, and/or BW <750 g, was limited in this study, and the PPV of the model was low; thus, practitioners should be careful when evaluating the prognoses of these infants. Further studies with larger sample sizes are required to optimize and validate the predictive model.
Conclusions
The model constructed in this study combined seven clinical indicators to predict the risk of in-hospital mortality in EPIs and may be conducive to the early identification of EPIs at risk of poor prognoses. It is necessary to expand the sample size to optimize and validate the predictive model in future studies.
Acknowledgments
The following hospitals have contributed to this study: Tianjin Central Hospital of Gynecology Obstetrics; Peking University Third Hospital; Tangshan Maternal and Child Health Hospital; Handan Central Hospital; Xingtai People’s Hospital; Baogang Third Hospital of HongCi Group; The Second Hospital of Hebei Medical University; Hebei Children’s Hospital; Tianjin Medical University General Hospital; The First Hospital of Tsinghua University; Inner Mongolia Maternal and Child Health Hospital; Beijing Children’s Hospital, Capital Medical University; The Affiliated Hospital of Inner Mongolia Medical University; Affiliated Hospital of Chifeng University; Beijing Luhe Hospital, Capital Medical University; Beijing United Family Hospital; Chifeng Municipal Hospital.
Funding: None.
Footnote
Reporting Checklist: The authors have completed the TRIPOD reporting checklist. Available at https://tp.amegroups.com/article/view/10.21037/tp-23-337/rc
Data Sharing Statement: Available at https://tp.amegroups.com/article/view/10.21037/tp-23-337/dss
Peer Review File: Available at https://tp.amegroups.com/article/view/10.21037/tp-23-337/prf
Conflicts of Interest: All authors have completed the ICMJE uniform disclosure form (available at https://tp.amegroups.com/article/view/10.21037/tp-23-337/coif). The authors have no conflicts of interest to declare.
Ethical Statement: The authors are accountable for all aspects of the work in ensuring that questions related to the accuracy or integrity of any part of the work are appropriately investigated and resolved. The study was conducted in accordance with the Declaration of Helsinki (as revised in 2013), and approved by Peking University First Hospital’s ethics committee (No. 2019–21) with no informed consent required due to the retrospective nature of the study. The other 17 hospitals were informed and agreed with the study.
Open Access Statement: This is an Open Access article distributed in accordance with the Creative Commons Attribution-NonCommercial-NoDerivs 4.0 International License (CC BY-NC-ND 4.0), which permits the non-commercial replication and distribution of the article with the strict proviso that no changes or edits are made and the original work is properly cited (including links to both the formal publication through the relevant DOI and the license). See: https://creativecommons.org/licenses/by-nc-nd/4.0/.
References
- Schmidt B, Asztalos EV, Roberts RS, et al. Impact of bronchopulmonary dysplasia, brain injury, and severe retinopathy on the outcome of extremely low-birth-weight infants at 18 months: results from the trial of indomethacin prophylaxis in preterms. JAMA 2003;289:1124-9. [Crossref] [PubMed]
- Deng K, Liang J, Mu Y, et al. Preterm births in China between 2012 and 2018: an observational study of more than 9 million women. Lancet Glob Health 2021;9:e1226-41. [Crossref] [PubMed]
- Zhu Z, Yuan L, Wang J, et al. Mortality and Morbidity of Infants Born Extremely Preterm at Tertiary Medical Centers in China From 2010 to 2019. JAMA Netw Open 2021;4:e219382. [Crossref] [PubMed]
- Jiang S, Yan W, Li S, et al. Mortality and Morbidity in Infants <34 Weeks' Gestation in 25 NICUs in China: A Prospective Cohort Study. Front Pediatr 2020;8:33. [Crossref] [PubMed]
- Kong X, Xu F, Wu R, et al. Neonatal mortality and morbidity among infants between 24 to 31 complete weeks: a multicenter survey in China from 2013 to 2014. BMC Pediatr 2016;16:174. [Crossref] [PubMed]
- Wu F, Liu G, Feng Z, et al. Short-term outcomes of extremely preterm infants at discharge: a multicenter study from Guangdong province during 2008-2017. BMC Pediatr 2019;19:405. [Crossref] [PubMed]
- Helenius K, Sjörs G, Shah PS, et al. Survival in Very Preterm Infants: An International Comparison of 10 National Neonatal Networks. Pediatrics 2017;140:e20171264. [Crossref] [PubMed]
- Zhang WW, Yu YH, Dong XY, Reddy S. Treatment status of extremely premature infants with gestational age < 28 weeks in a Chinese perinatal center from 2010 to 2019. World J Pediatr 2022;18:67-74. [Crossref] [PubMed]
- Wang H, Gao X, Liu C, et al. Morbidity and mortality of neonatal respiratory failure in China: surfactant treatment in very immature infants. Pediatrics 2012;129:e731-40. [Crossref] [PubMed]
- Cao Y, Jiang S, Sun J, et al. Assessment of Neonatal Intensive Care Unit Practices, Morbidity, and Mortality Among Very Preterm Infants in China. JAMA Netw Open 2021;4:e2118904. [Crossref] [PubMed]
- Liao XP, Chipenda-Dansokho S, Lewin A, et al. Advanced Neonatal Medicine in China: A National Baseline Database. PLoS One 2017;12:e0169970. [Crossref] [PubMed]
- Mangham LJ, Petrou S, Doyle LW, et al. The cost of preterm birth throughout childhood in England and Wales. Pediatrics 2009;123:e312-27. [Crossref] [PubMed]
- Zhu Z, Wang J, Chen C, et al. Hospitalization charges for extremely preterm infants: a ten-year analysis in Shanghai, China. J Med Econ 2020;23:1610-7. [Crossref] [PubMed]
- Tyson JE, Parikh NA, Langer J, et al. Intensive care for extreme prematurity--moving beyond gestational age. N Engl J Med 2008;358:1672-81. [Crossref] [PubMed]
- Bader D, Kugelman A, Boyko V, et al. Risk factors and estimation tool for death among extremely premature infants: a national study. Pediatrics 2010;125:696-703. [Crossref] [PubMed]
- Moreira A, Benvenuto D, Fox-Good C, et al. Development and Validation of a Mortality Prediction Model in Extremely Low Gestational Age Neonates. Neonatology 2022;119:418-27. [Crossref] [PubMed]
- Bai R, Jiang S, Guo J, et al. Variation of Neonatal Outcomes and Care Practices for Preterm Infants <34 Weeks' Gestation in Different Regions of China: A Cohort Study. Front Pediatr 2021;9:760646. [Crossref] [PubMed]
- Fenton TR, Kim JH. A systematic review and meta-analysis to revise the Fenton growth chart for preterm infants. BMC Pediatr 2013;13:59. [Crossref] [PubMed]
- Bell MJ, Ternberg JL, Feigin RD, et al. Neonatal necrotizing enterocolitis. Therapeutic decisions based upon clinical staging. Ann Surg 1978;187:1-7. [Crossref] [PubMed]
- Volpe JJ. Neurobiology of periventricular leukomalacia in the premature infant. Pediatr Res 2001;50:553-62. [Crossref] [PubMed]
- Papile LA, Munsick-Bruno G, Schaefer A. Relationship of cerebral intraventricular hemorrhage and early childhood neurologic handicaps. J Pediatr 1983;103:273-7. [Crossref] [PubMed]
- Stoll BJ, Hansen N, Fanaroff AA, et al. Late-onset sepsis in very low birth weight neonates: the experience of the NICHD Neonatal Research Network. Pediatrics 2002;110:285-91. [Crossref] [PubMed]
- Barrington KJ. Hypotension and shock in the preterm infant. Semin Fetal Neonatal Med 2008;13:16-23. [Crossref] [PubMed]
- Li SJ, Feng Q, Tian XY, et al. Delivery room resuscitation and short-term outcomes of extremely preterm and extremely low birth weight infants: a multicenter survey in North China. Chin Med J (Engl) 2021;134:1561-8. [Crossref] [PubMed]
- Hypertensive Disorders in Pregnancy Subgroup, Chinese Society of Obstetrics and Gynecology, Chinese Medical Association. Hypertensive Disorders in Pregnancy Subgroup Chinese Society of Obstetrics and Gynecology Chinese Medical Association. Zhonghua Fu Chan Ke Za Zhi 2015;50:721-8.
- Obstetrics Subgroup, Chinese Society of Obstetrics and Gynecology, Chinese Medical Association. Group of Pregnancy with Diabetes Mellitus, Chinese Society of Perinatal Medicine, Chinese Medical Association; Obstetrics Subgroup Chinese Society of Obstetrics and Gynecology Chinese Medical Association; Group of Pregnancy with Diabetes Mellitus Chinese Society of Perinatal Medicine Chinese Medical Association. Zhonghua Fu Chan Ke Za Zhi 2014;49:561-9.
- Goldenberg RL, Culhane JF, Iams JD, et al. Epidemiology and causes of preterm birth. Lancet 2008;371:75-84. [Crossref] [PubMed]
- Williams MJ, Ramson JA, Brownfoot FC. Different corticosteroids and regimens for accelerating fetal lung maturation for babies at risk of preterm birth. Cochrane Database Syst Rev 2022;8:CD006764. [Crossref] [PubMed]
- Ravelli AC, Schaaf JM, Mol BW, et al. Antenatal prediction of neonatal mortality in very premature infants. Eur J Obstet Gynecol Reprod Biol 2014;176:126-31. [Crossref] [PubMed]
- Ambalavanan N, Carlo WA. Comparison of the prediction of extremely low birth weight neonatal mortality by regression analysis and by neural networks. Early Hum Dev 2001;65:123-37. [Crossref] [PubMed]
- Ambalavanan N, Carlo WA, Bobashev G, et al. Prediction of death for extremely low birth weight neonates. Pediatrics 2005;116:1367-73. [Crossref] [PubMed]
- Sheikhtaheri A, Zarkesh MR, Moradi R, et al. Prediction of neonatal deaths in NICUs: development and validation of machine learning models. BMC Med Inform Decis Mak 2021;21:131. [Crossref] [PubMed]
- Jensen EA, DeMauro SB, Kornhauser M, et al. Effects of Multiple Ventilation Courses and Duration of Mechanical Ventilation on Respiratory Outcomes in Extremely Low-Birth-Weight Infants. JAMA Pediatr 2015;169:1011-7. [Crossref] [PubMed]
- Klingenberg C, Wheeler KI, McCallion N, et al. Volume-targeted versus pressure-limited ventilation in neonates. Cochrane Database Syst Rev 2017;10:CD003666. [Crossref] [PubMed]
- Sweet DG, Carnielli VP, Greisen G, et al. European Consensus Guidelines on the Management of Respiratory Distress Syndrome: 2022 Update. Neonatology 2023;120:3-23. [Crossref] [PubMed]
- Buzzella B, Claure N, D'Ugard C, et al. A randomized controlled trial of two nasal continuous positive airway pressure levels after extubation in preterm infants. J Pediatr 2014;164:46-51. [Crossref] [PubMed]
- Kusuda S, Hirano S, Nakamura T. Creating experiences from active treatment towards extremely preterm infants born at less than 25 weeks in Japan. Semin Perinatol 2022;46:151537. [Crossref] [PubMed]
- Amer R, Moddemann D, Seshia M, et al. Neurodevelopmental outcomes of infants born at <29 weeks of gestation admitted to Canadian neonatal intensive care units based on location of birth. J Pediatr 2018;196:31-37.e1. [Crossref] [PubMed]
- Isayama T. The clinical management and outcomes of extremely preterm infants in Japan: past, present, and future. Transl Pediatr 2019;8:199-211. [Crossref] [PubMed]
- Cho IY, Lee HM, Kim SY, et al. Impact of Outborn/Inborn Birth Status of Infants Born at <29 Weeks of Gestation on Neurodevelopmental Impairment: A Nationwide Cohort Study in Korea. Int J Environ Res Public Health 2022;19:11718. [Crossref] [PubMed]
- Fischer N, Soraisham A, Shah PS, et al. Extensive cardiopulmonary resuscitation of preterm neonates at birth and mortality and developmental outcomes. Resuscitation 2019;135:57-65. [Crossref] [PubMed]
- Wyckoff MH, Salhab WA, Heyne RJ, et al. Outcome of extremely low birth weight infants who received delivery room cardiopulmonary resuscitation. J Pediatr 2012;160:239-244.e2. [Crossref] [PubMed]
- Shah PS. Extensive cardiopulmonary resuscitation for VLBW and ELBW infants: a systematic review and meta-analyses. J Perinatol 2009;29:655-61. [Crossref] [PubMed]
- Patel RM, Kandefer S, Walsh MC, et al. Causes and timing of death in extremely premature infants from 2000 through 2011. N Engl J Med 2015;372:331-40. [Crossref] [PubMed]
- Hailemeskel HS, Tiruneh SA. Development of a Nomogram for Clinical Risk Prediction of Preterm Neonate Death in Ethiopia. Front Pediatr 2022;10:877200. [Crossref] [PubMed]
- Romero TE, Friedman WF. Limited left ventricular response to volume overload in the neonatal period: a comparative study with the adult animal. Pediatr Res 1979;13:910-5. [Crossref] [PubMed]
- Toyoshima K, Kawataki M, Ohyama M, et al. Tailor-made circulatory management based on the stress-velocity relationship in preterm infants. J Formos Med Assoc 2013;112:510-7. [Crossref] [PubMed]
- Scholl JE, Yanowitz TD. Pulmonary hemorrhage in very low birth weight infants: a case-control analysis. J Pediatr 2015;166:1083-4. [Crossref] [PubMed]
- Basu S, Rathore P, Bhatia BD. Predictors of mortality in very low birth weight neonates in India. Singapore Med J 2008;49:556-60.