Fractal structure in the volumetric contrast enhancement of malignant gliomas as a marker of oxidative metabolic pathway gene expression
Introduction
Fractals are structures that have the same general physical appearance when viewed over many scales of magnification (1). There are many examples of fractals in nature and biology; some famous ones are snowflakes, coastlines, retinal vasculature, and Romanesco Broccoli (2-4). Each fractal is associated with a fractal dimension, a statistical measure that quantifies how detail in a pattern changes with the spatial scale it is measured at (1). In general, a higher fractal dimension implies a more complex structure. Sophisticated fractal structures can emerge from iterations of simple growth rules. For example, the complex branching structure of snowflakes results from a simple tradeoff between surface tension and heat diffusion (5). This emergence of fractal structure may be of particular interest for those that study brain tumors—firstly because tumors emerge when a few genetic mutations change the rules for how cells behave with one another and grow, and secondly because blood vessels in the brain can develop in a fractal manner (6).
The term “radiogenomics” is used to describe the relationship between imaging findings and genomic changes, with the hope of inferring molecular properties without the need to obtain tissue surgically. These approaches have already established some direct links between imaging features and underlying molecular changes in brain tumors, particularly glioblastoma (7-11). Recent molecular studies have found that there are genomic subtypes of brain tumors that confer different prognoses and different susceptibility to surgical, radiation-based, and medicinal intervention. If one can accurately infer underlying tumor biology from imaging rather than surgical specimen, conservative therapies may be initiated with less associated morbidity and at lower cost. Existing glioblastoma radiogenomic studies have examined the gross volumes of the tumors, contrast enhancement volumes, and qualitative shape (11), but quantitative metrics to describe the internal structure of the tumor have not yet been developed as markers.
We have devised a new way to quantitatively describe volumetric brain imaging using the fractal dimensionality of contrast enhancement. In glioblastoma tumors, gadolinium enhancement reflects the diminished blood-brain barrier integrity from abnormally permeable endothelial cell layers. This increased permeability is both a property of newly-formed small blood vessels, and also a result of circulating endothelial growth factors that induce constant remodeling of the tumor vasculature (12,13). Therefore, quantifying the structure of enhancement in glioblastoma tumors may specifically inform us about a combination of both microvascular structure and angiogenic drive. The purpose of our study was to measure whether the large-scale structure of brain tumors exhibited fractal structure and to correlate these results with biological tumor characteristics.
Methods
Patients
After institutional review board approval (approval number 09-420), we retrospectively identified a cohort of 42 patients, who presented to care at the UMC Utrecht from February 2010 to November 2012. Each of these underwent open resection of intracranial lesions that were histologically confirmed to be de novo WHO grade 4 glioblastoma. All patients provided an informed consent for the (anonymized) genetical/biological analyses of their tumor samples and the use of the relevant clinical/radiological information. Patients with both treatment-naïve MR imaging and fresh-frozen surgical tissue specimens available for molecular analysis were included in this study. Survival data, documented in days from surgery, were obtained from hospital records, and analyzed with Kaplan-Meier curves and log-rank testing.
Imaging
All patients had pre-operative gadolinium contrasted T1 MRI images with voxel resolution of 1×1×1 mm3, and plainly visible contrast enhancing tumors. For each patient, a three-dimensional tumor boundary trace and contrast intensity threshold was manually identified by one investigator (Kai J. Miller), who was blinded to demographic, clinical, pathologic, and molecular data. For multifocal glioblastoma, all masses were traced, and the one with the largest volume was retained for further analysis.
Tumor enhancement fractal structure (illustrated in Figure 1)
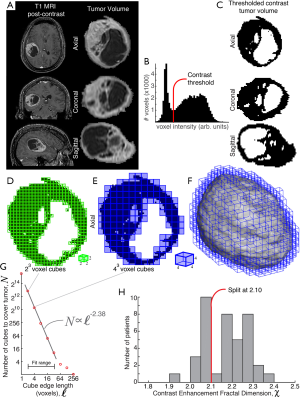
For each tumor, a fractal dimension (Minkowski-Bouligand dimension/scaling exponent) using was calculated a 3D box-counting (cubic) approach (14,15). Each T1 post-contrast tumor volume was thresholded at an intensity determined based upon a histogram of voxel contrast intensities of the isolated tumor as well as visual inspection of the MRI. The thresholded tumor volume was then parsed by cube-counting using a fixed grid scan, which determines the number of cubes, N, of edge length , needed to cover the thresholded volume. The fractal dimension, χ, is estimated from the relation , by performing a linear least-squares fit of log2N versus over a set fit range. The fit range was theoretically constrained on the lower end by voxel resolution, and on the upper end by tumor size. For stable fits, we chose the range =2 voxels to < middle dimension of tumor bounding region, with interval steps of =2n (see supplemental comment on fitting). Prior to further examination, 3 patients were excluded from study because their tumor size was too small to estimate an acceptable fit range. One examiner (Kai J. Miller) determined natural split value of χ =2.10 from the histogram of fractal dimension values for the 39 patients included in the cohort, and a separate examiner (Pierre A. Robe) independently performed the molecular analyses. This natural split was confirmed appropriate by applying a Gaussian mixture model to the set of fit fractal dimensions, and drawing the fit threshold such that all values below threshold have >90% chance of belonging to the lower fractal dimension group (this results in χ =2.099 before rounding as the tumor with the highest fractal dimension to belong to the lower group). Future studies, with larger cohorts, may further refine this threshold.
Fresh-frozen tissue samples and mRNA expression analysis
Fresh-frozen surgical samples of glioblastoma patients were prospectively collected between February 2010 and November 2012. RNA was extracted with the Nucleospin® TriPrep kit (Macherey-Nagel, Bethlehem, PA, USA) to the manufacturer’s instructions. Affymetrix HG U133 plus 2.0 array preparation and scanning were performed according to the manufacturer’s protocol and as reported previously (16). Exploratory gene set enrichment analyses (GSEA) were performed after RMA-normalization (17) and correction for batch effects, with the Partek Genomics suite platform (Partek® Genomic Suite 6.6). Analyses were performed with the Broad Institute MySig libraries of curated gene sets C2&C4, version 5.0 (17), 1,000 permutations and default additional parameters. A false discovery rate (FDR) threshold of 0.15 was chosen to be more stringent than the recommended threshold level of 0.25 for GSEA studies (17).
Vascular endothelial growth factor (VEGF) expression assessment (illustrated in Figure 2)
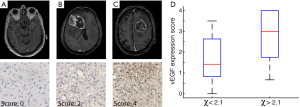
Based upon initial findings with the GSEA analysis that showed an association between fractal dimension of contrast enhancement and oxidative metabolism (Table 1), we decided to test the samples for VEGF expression. Formalin fixed, paraffin embedded tissue samples of 35 of the patients in our cohort were included in tissue microarrays. Construction and processing of tissue microarrays and methods on immunohistochemical staining have been reported in a previous study (18). Rabbit polyclonal VEGF antibody was used for staining (1:100, Thermo Scientific, Waltham, MA, USA). Assessment of cytoplasmic VEGF expression was performed blinded to the clinical and imaging data. The VEGF expression was quantified as percentage of cytoplasmic staining, and scored by visual slide inspection: 0, no staining; 1, 1–25% of cells stain; 2, 26–50%; 3, 51–75%; 4, 76–100%. Images were obtained at 40× magnification using a Nanozoomer 2.0HT digital slide scanner (Hamamatsu Photonics, Hamamatsu City, Japan).
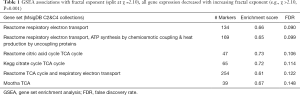
Full table
Results
Note that in addition to these results, there is also a supplement, which contains a comment on the fitting, three supplemental figures (Figures S1-S3), and two supplemental table (Tables S1).
Fractal structure of contrast enhancement in glioblastoma
We found robust evidence for fractal structure in the contrast enhancement pattern, with a fractal dimension of 2.17±0.10 (Figure 1 and supplemental Figure S1—significantly different from 2.00, P<10–9 by unpaired t-test). There was a significant correlation between fractal dimension and tumor-volume (r=0.35, P=0.03, t-test), contrast-enhancement-volume (r=0.54, P=4×10–4, t-test), and volumetric-fraction-of-tumor-enhancement (r=0.35, P=0.03, t-test). However, there was no correlation between survival and fractal dimension (P=0.75, log-rank test, supplemental Figure S3). When comparing patients with tumors of fractal dimension above and below 2.1, prognosis did not vary between both groups, both in univariable and Cox multivariable analyses (taking age and KPS into account). Age, KPS and post-operative treatment do not vary between both groups (Table S1). All assessed tumors were IDH1 WT (25% of IDH data missing, evenly distributed between both groups. Tumor volumes were similar in both groups, and all tumors had a gross total resection.
Fractal dimension correlates with specific gene set expression pattern
Exploratory GSEA analysis showed a strong association between fractal dimension and mitochondrial respiration/ATP production pathways (Table 1: all associations significant with P<0.001, at FDR <0.15). The gene set enrichment assay assesses correlations of a split parameter (in this case our measure of fractal dimension, split at visually-apparent and fit-confirmed threshold χ =2.10, as illustrated in Figure 1H) with a large library of RNA expression gene clusters [MsigDB v5.0 C2&C4 curated gene set collections (17)]. This assessment was performed over 4,080 potential gene set pathways (Figure S2), and only 6 gene sets met significance threshold. All of these showed decreased gene expression for tumors with higher fractal exponent (e.g., χ >2.10). The full set of 4,080 gene sets tested for is listed in supplemental http://tcr.amegroups.com/public/addition/tcr/supp-tcr.2017.10.15.pdf.
Higher fractal dimension is correlated with increased VEGF expression
Increased fractal dimension was correlated with increased VEGF expression (r=0.45, P=0.006, t-test). The split in fractal dimension at χ =2.10 is associated with significant increase in VEGF expression (illustrated in the box-plot of Figure 2D, P=0.01, t-test).
Discussion
The fractal dimension of approximately 2.15 that we observe in glioblastoma enhancement quantitatively describes a structure that is significantly more complex than a simple surface, such as a hollow sphere (also fractal, but with dimension 2), but less complex than some other volumetric structures with extensive branching, such as a broccoli (fractal with dimension 2.66) (19). Interestingly, there is variation in the complexity of enhancement across tumors from different patients. Being able to quantitatively describe the internal structure of tumors in this way may be an important new tool. However, there is no a priori reason why glioblastoma tumors should have fractal structure at all—one can easily imagine other tumors, with plainly observable structural complexity at one particular level of magnification, which would be non-fractal. For example, metastatic adenocarcinomas would not be expected to have self-similarity at different spatial scales. Instead, they would have well-defined, non-fractal, structure reflecting the glandular anatomy of the tissue of origin.
The fractal structures generated by mathematical simulation have infinite resolution, and the fractal fit can extend over an arbitrarily large range. However, real world phenomena have structure that can only be measured over a limited range—e.g., snowflakes only grow to a few millimeters and coastlines can’t be measured in centimeters. Our measurements are no exception, and we were spatially limited in quantification of fractal structure on the on the upper end by the finite width of tumors at ~3–5 cm, and the lower end by MRI voxel edge length of 1 mm. In future studies, one might attempt to work around this lower limit by obtaining smaller voxel sizes with higher field magnets (e.g., 7T).
Because contrast enhancement reflects fragile neovascularization within the glioblastoma tumor, the fractal measurement may directly reflect microvascular structure and angiogenic drive, and indirectly reflect genetic expression profiles of the tumor. Indeed, a correlation between fractal structure and underlying tumor biology was found; increases in the fractal dimension of contrast enhancement correlate with decreased oxidative pathway gene set expression (a shift to glycolytic metabolism), with increased VEGF expression (Figure 2). We propose two potential explanations for this, one anaerobic and one aerobic. In the anaerobic case, one might presuppose that some of these glioblastoma tumors outgrow their blood supply, forcing them to undergo an ischemia driven glycolytic shift, while simultaneously releasing angiogenic factors like VEGF to induce rapid neovascularization. The correlation between increasing fractal dimension and increased tumor volume may suggest that larger tumors produce more ischemic drive for a glycolytic shift while releasing VEGF in an attempt to improve oxygen delivery. Conversely, there may be an aerobic explanation for our findings, independent of ischemic drive: many tumors show a distinct shift from mitochondrial oxidative phosphorylation to glycolysis for energy production, known as the “Warburg effect” (aerobic glycolysis) (20,21). This involves a number of changes in genetic expression, such as stabilization of HIF-1α, and increased expression of VEGF, GLUT1, and pyruvate dehydrogenase kinase. These changes encourage tumor growth by reducing reactive oxygen species levels, increasing ATP supply, and providing structural elements for new cell growth (20,21). With this connection in mind, future studies may measure HIF-1α and markers of metabolism using an acute frozen preparation of tumor tissue, before it is affected by ischemia at time of resection.
Predictive radiogenomic features in MRI that reflect molecular aberrations and subtypes of tumors would serve as a preliminary surrogate prior to invasive and expensive genomic tissue testing, and facilitate real-time translation/integration of genomic-based studies into the clinic. If our finding hints at a possible metabolic drive for vascularization during tumor development, in response to the Warburg effect, it begs the question: does metabolic change drive neovascularization independent of ischemic drive to do so? Administration of an anti-VEGF agent for glioblastoma results in decreased volume of tumor enhancement, but increased glycolysis and increased tumor invasion (22). This suggests that perhaps increased VEGF expression comes as a downstream effect of other glycolysis-associated changes that promote tumor growth, but that VEGF is not itself of direct benefit to the developing tumor. Conversely, inhibitors of pyruvate dehydrogenase kinase like dichloroacetate reverse the Warburg effect and potentiate formation of oxidating free radicals (23). Dichloroacetate decreases tumor invasion (24), is safe for glioblastoma patients (based upon phase 1 FDA trial) (25), and is rapidly gaining popularity as an adjunct agent in glioblastoma therapy (24,26-28). HIF-1α is a transcription factor that increases transcription of VEGF, enzymes of glycolysis, and glucose transporters; many tumors exhibiting the Warburg effect have loss-of-function mutations in enzymes that degrade HIF-1α (21). Initiation of HIF-1α translation is blocked the agent auraptene, which has been shown to inhibit glycolytic and mitochondrial metabolism, decrease cell motility, and inhibit VEGF-induced neovascularization (29). Tumors with higher fractal dimension in enhancement structure may be selectively susceptible to administration of dichloroacetate, auraptene, and other emerging agents. Pending further exploration, this fractal-dimension measurement might help to non-surgically identify which patients would benefit from these agents.
Conclusions
Quantification of fractal structure in tumor enhancement is a new type of methodology for the emerging radiogenomic toolbox, and may be of direct clinical benefit if it can guide therapies without need for tissue sample. We observed fractal structure in the volumetric contrast enhancement pattern of glioblastoma tumors, with an average fractal dimension of approximately 2.15, but variation from ~2.0–2.3. Higher fractal dimension correlated specifically with decreased gene expression for oxidative metabolic pathways on GSEA analysis, and also correlated with increased VEGF expression by immunostaining. Together, these measurements suggest that increased fractal dimension of glioblastoma enhancement reflects a glycolytic shift in tumor, and may indicate which patients would benefit from emerging anti-glycolysis agents like auraptene and dichloroacetate.
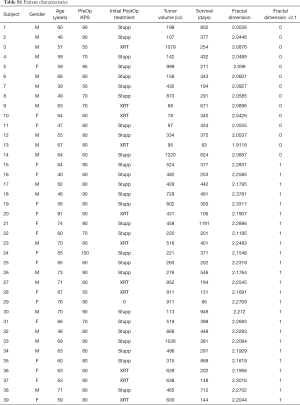
Full table
Acknowledgments
Funding: This study was supported by grants from the Stanford Society of Physician Scholars to KJ Miller; the Belgian Ministry of Health (grant number PNC 29-006, to PA Robe); the National Research Fund of Belgium (grant number 1.5.162.10, to PA Robe); and the Belgian Foundation against Cancer to PA Robe.
Footnote
Conflicts of Interest: All authors have completed the ICMJE uniform disclosure form (available at http://dx.doi.org/10.21037/tcr.2017.10.15). The authors have no conflicts of interest to declare.
Ethical Statement: The authors are accountable for all aspects of the work in ensuring that questions related to the accuracy or integrity of any part of the work are appropriately investigated and resolved. The study was conducted in accordance with the Declaration of Helsinki (as revised in 2013). The study was approved by institutional review board (approval number 09-420). All patients provided an informed consent for the (anonymized) genetical/biological analyses of their tumor samples and the use of the relevant clinical/radiological information.
Open Access Statement: This is an Open Access article distributed in accordance with the Creative Commons Attribution-NonCommercial-NoDerivs 4.0 International License (CC BY-NC-ND 4.0), which permits the non-commercial replication and distribution of the article with the strict proviso that no changes or edits are made and the original work is properly cited (including links to both the formal publication through the relevant DOI and the license). See: https://creativecommons.org/licenses/by-nc-nd/4.0/.
References
- Mandelbrot BB. Self-affine fractals and fractal dimension. Physica Scripta 1985;32:257. [Crossref]
- Mandelbrot BB. How long is the coast of Britain. Science 1967;156:636-8. [Crossref] [PubMed]
- Green DG. Fractals and scale. 1993, self published.
- Family F, Masters BR, Platt DE. Fractal pattern formation in human retinal vessels. Physica D 1989;38:98-103. [Crossref]
- Ben-Jacob E, Goldenfeld N, Langer J, et al. Dynamics of interfacial pattern formation. Phys Rev Lett 1983;51:1930. [Crossref]
- Risser L, Plouraboué F, Steyer A, et al. From homogeneous to fractal normal and tumorous microvascular networks in the brain. J Cereb Blood Flow Metab 2007;27:293-303. [Crossref] [PubMed]
- Zinn PO, Mahajan B, Sathyan P, et al. Radiogenomic mapping of edema/cellular invasion MRI-phenotypes in glioblastoma multiforme. PLoS One 2011;6:e25451 [Crossref] [PubMed]
- Gutman DA, Cooper LA, Hwang SN, et al. MR imaging predictors of molecular profile and survival: multi-institutional study of the TCGA glioblastoma data set. Radiology 2013;267:560-9. [Crossref] [PubMed]
- Diehn M, Nardini C, Wang DS, et al. Identification of noninvasive imaging surrogates for brain tumor gene-expression modules. Proc Natl Acad Sci U S A 2008;105:5213-8. [Crossref] [PubMed]
- Naeini KM, Pope WB, Cloughesy TF, et al. Identifying the mesenchymal molecular subtype of glioblastoma using quantitative volumetric analysis of anatomic magnetic resonance images. Neuro Oncol 2013;15:626-34. [Crossref] [PubMed]
- Itakura H, Achrol AS, Mitchell LA, et al. Magnetic resonance image features identify glioblastoma phenotypic subtypes with distinct molecular pathway activities. Sci Transl Med 2015;7:303ra138 [Crossref] [PubMed]
- Brasch R, Pham C, Shames D, et al. Assessing tumor angiogenesis using macromolecular MR imaging contrast media. J Magn Reson Imaging 1997;7:68-74. [Crossref] [PubMed]
- Roberts HC, Roberts TP, Brasch RC, et al. Quantitative measurement of microvascular permeability in human brain tumors achieved using dynamic contrast-enhanced MR imaging: correlation with histologic grade. AJNR Am J Neuroradiol 2000;21:891-9. [PubMed]
- Liebovitch LS, Toth T. A fast algorithm to determine fractal dimensions by box counting. Physics Letters A 1989;141:386-90. [Crossref]
- Perret J, Prasher S, Kacimov A. Mass fractal dimension of soil macropores using computed tomography: from the box-counting to the cube-counting algorithm. Eur J Soil Sci 2003;54:569-79. [Crossref]
- Artesi M, Kroonen J, Bredel M, et al. Connexin 30 expression inhibits growth of human malignant gliomas but protects them against radiation therapy. Neuro Oncol 2015;17:392-406. [Crossref] [PubMed]
- Subramanian A, Tamayo P, Mootha VK, et al. Gene set enrichment analysis: a knowledge-based approach for interpreting genome-wide expression profiles. Proc Natl Acad Sci U S A 2005;102:15545-50. [Crossref] [PubMed]
- Berendsen S, Varkila M, Kroonen J, et al. Prognostic relevance of epilepsy at presentation in glioblastoma patients. Neuro Oncol 2016;18:700-6. [Crossref] [PubMed]
- Kapelner A, Schupack V, Golomshtok M, et al. Fractal Dimension of Broccoli. The Physics Factbook. 2002. Available online: https://hypertextbook.com/facts/2002/broccoli.shtml
- Vander Heiden MG, Cantley LC, Thompson CB. Understanding the Warburg effect: the metabolic requirements of cell proliferation. Science 2009;324:1029-33. [Crossref] [PubMed]
- Linehan WM, Rouault TA. Molecular pathways: fumarate hydratase-deficient kidney cancer—targeting the Warburg effect in cancer. Clin Cancer Res 2013;19:3345-52. [Crossref] [PubMed]
- Keunen O, Johansson M, Oudin A, et al. Anti-VEGF treatment reduces blood supply and increases tumor cell invasion in glioblastoma. Proc Natl Acad Sci U S A 2011;108:3749-54. [Crossref] [PubMed]
- Velpula KK, Bhasin A, Asuthkar S, et al. Combined targeting of PDK1 and EGFR triggers regression of glioblastoma by reversing the Warburg effect. Cancer Res 2013;73:7277-89. [Crossref] [PubMed]
- Kumar K, Wigfield S, Gee HE, et al. Dichloroacetate reverses the hypoxic adaptation to bevacizumab and enhances its antitumor effects in mouse xenografts. J Mol Med (Berl) 2013;91:749-58. [Crossref] [PubMed]
- Dunbar E, Coats B, Shroads A, et al. Phase 1 trial of dichloroacetate (DCA) in adults with recurrent malignant brain tumors. Invest New Drugs 2014;32:452-64. [Crossref] [PubMed]
- Shen H, Hau E, Joshi S, et al. Sensitization of Glioblastoma Cells to Irradiation by Modulating the Glucose Metabolism. Mol Cancer Ther 2015;14:1794-804. [Crossref] [PubMed]
- Shen H, Decollogne S, Dilda PJ, et al. Dual-targeting of aberrant glucose metabolism in glioblastoma. J Exp Clin Cancer Res 2015;34:14. [Crossref] [PubMed]
- Michelakis ED, Sutendra G, Dromparis P, et al. Metabolic modulation of glioblastoma with dichloroacetate. Sci Transl Med 2010;2:31ra34 [Crossref] [PubMed]
- Jang Y, Han J, Kim SJ, et al. Suppression of mitochondrial respiration with auraptene inhibits the progression of renal cell carcinoma: involvement of HIF-1α degradation. Oncotarget 2015;6:38127. [Crossref] [PubMed]
- Clauset A, Shalizi CR, Newman ME. Power-law distributions in empirical data. SIAM Review 2009;51:661-703. [Crossref]