DNA damage repair molecular subtype derived immune signature applicable for the prognosis and immunotherapy response prediction in colon cancer
Introduction
Background
DNA is the most important genetic material in the human body, and its integrity affects the accuracy of genetic information transmission (1). In the human body, endogenous factors (such as replication errors, oxidative deamination and reactive oxygen species) and exogenous factors (such as UV light and radiation) could cause abnormalities in the chemical structure or coding characteristics of DNA (2,3), resulting in DNA damage, which in turn affects genome replication and transcription (4). If DNA damage is not repaired in time, cells will experience cell cycle arrest, aging or programmed cell death, which would pose a threat to the body and even lead to diseases (5,6). For example, DNA repair function is defective in the process of tumorigenesis and destroys the stability of the genome (7). Under normal circumstances, the human body guards the intracellular genomic DNA from a variety of physical and chemical factors through the DNA damage repair (DDR) pathway, thus maintaining the stability of genetic material. The functional gene sets that the DDR pathway mainly depends on including homologous recombination (HR), mismatch repair (MMR), base excision repair (BER), nucleotide excision repair (NER), and nonhomologous end-joining (NHEJ). There is evidence that functional imbalances or defects in DDR genes are related to tumor susceptibility (8).
Rationale and knowledge gap
Colon cancer (CC) is one of the most common malignant tumors in the digestive tract (9). It ranks fourth among the most common malignant tumors in the world; approximately 400,000 men and 380,000 women suffer from CC every year (10). In recent years, with the advancements in surgical concepts, the improvement in neoadjuvant therapy and the progress of laparoscopic techniques, the overall survival (OS) time of patients with CC has been significantly improved (11). However, there are still many patients being diagnosed with the advanced stage at the first diagnosis and missing the best opportunity for operation (12). At present, there are opposite conclusions in different studies on the relationship between the DDR and the prognosis of patients with CC (13,14). Therefore, in the face of one of the major threats to human health, it is particularly important to understand the molecular mechanism of the DDR: how cancer gradually changes the repair process of DNA and how to make use of these processes to kill cancer cells.
Objective
Prospective clinical trials conducted in recent years have shown that the DDR is critical to the immunotherapy response of cancer patients (15-17). However, the effect of the DDR on the immunophenotype of CC patients has not been clarified. This study analyzed the prognostic value of immune-related genes that are differentially expressed in CC patients with different DDR molecular subtypes to provide references for precise clinical assessment of the prognosis of CC patients. We present this article in accordance with the TRIPOD reporting checklist (available at https://tcr.amegroups.com/article/view/10.21037/tcr-23-747/rc).
Methods
Data acquisition
Four large-sample independent CC cohorts, namely, The Cancer Genome Atlas (TCGA)-colon adenocarcinoma (COAD) (n=430), GSE39582 (n=579), GSE17538 (n=232), and GSE38832 (n=122), were included in the research. The clinical and mRNA expression data are available from TCGA (https://portal.gdc.cancer.gov/) and Gene Expression Omnibus (GEO, https://www.ncbi.nlm.nih.gov/geo/). The transcripts per million (TPM) kilobase values were transformed from the fragments per kilobase of transcript per million (FPKM) data for data normalization in the different RNA-seq cohorts via the R package “limma”, and the ComBat function of the R “SVA” package was used to remove the batch effects in different datasets (18,19). The data analyzed in this research were obtained from public databases, and the approval of the local ethics committee was not required. This study was conducted in accordance with the Declaration of Helsinki (as revise in 2013). The flowchart of this work is presented in Figure S1.
DDR-related gene cluster analysis
The genes associated with DDR pathways were extracted from the molecular signatures database (MSigDB, http://www.gsea-msigdb.org/gsea) (tables available at https://cdn.amegroups.cn/static/public/tcr-23-747-1.xlsx). The immune-related gene list was obtained from the ImmPort database (https://immport.niaid.nih.gov) and the Innate database (https://www.innatedb.ca/) (tables available at https://cdn.amegroups.cn/static/public/tcr-23-747-2.xlsx). The training cohort (n=1,009), which was a combination of the TCGA and GSE39582 cohorts, was used to analyze the prognostic value of the DDR-related genes (DDRGs) for CC. The prognosis-related genes (PRGs) screened by univariate Cox regression analysis (P<0.05) were subjected to cluster analysis via the R package “ConsensusClusterPlus”. Gene set variation analysis (GSVA) was performed with the R package “GSVA” to evaluate immune-related pathway enrichment for different clusters (20). The tumor microenvironment (TME) scores were calculated by the R package “estimate” for comparison of the TME in different clusters. The OS difference between different clusters was analyzed by the Kaplan-Meier method. The Wilcoxon signed-rank test contained in the R package “limma” was used to identify the differentially expressed immune-related genes (DEIRGs) between different clusters [false discovery rate (FDR) <0.05] (21).
Construction and validation of the prognostic risk score
Univariate Cox regression analysis was performed in the training cohort (n=1,009), the PRGs were defined as the genes with a P value <0.001. The overfitting between the PRGs was removed by the least absolute shrinkage and selection operator (LASSO) algorithm with penalty parameter (λ) determined by the lowest partial likelihood deviance based on the R package “glmnet” (22). We performed 1,000 10-fold cross-validations of datasets and selected genes with more than 900 repetitions. The regression coefficient was shrunk with a penalty proportional to the size for determining a subset of genes. The genes with nonzero regression coefficients obtained from LASSO regression analysis were included in the multivariate Cox regression analysis (23). The risk score was equal to the sum of the product of the multivariate Cox regression coefficient of each gene multiplied by the expression level of each gene (24). The median risk score was the cutoff dividing patients into high- and low-risk groups (25). Internal (TCGA-COAD, n=430; GSE39582, n=579) and external (GSE17538, n=232; GSE38832, n=122) cohorts were used to validate the risk score’s performance in predicting prognosis, which was assessed by Kaplan-Meier survival analysis and time-dependent receiver operating characteristic (ROC) analysis. Univariate and multivariate Cox regression analyses were utilized to test whether the risk score could serve as an independent prognostic indicator. The chi-square test was used to compare clinical feature differences in different risk groups. The IMvigor210 cohort (http://research-pub.gene.com/IMvigor210CoreBiologies/) was enrolled to test the risk score’s predictive value for immunotherapy response.
Quantification of immune cell infiltration using the ssGSEA algorithm
The 23 types of immune cell infiltration were quantified by the normalized enrichment score (NES) based on the single sample gene set enrichment analysis (ssGSEA) algorithm (26). The NES differences between the high- and low-risk groups was compared by the independent-samples t-test, and P<0.05 was considered to be statistically significant.
Exploring the underlying molecular mechanisms of the prognostic signature
The R package ‘limma’ was used to identify the differentially expressed genes (DEGs) between the high- and low-risk groups (FDR <0.05), and the R package “clusterProfiler” was utilized to annotating the DEGs’ Gene Ontology (GO) term. Gene set enrichment analysis (GSEA) was used to determine the active molecular pathways in different risk groups (nom P<0.05, FDR <0.25) (19,27).
Statistical analysis
All statistical analyses were accomplished with R software (v3.6.3). The Student’s t-test was performed for continuous variables with a normal distribution, while the categorical variables were compared by the Pearson chi-square test. The survival outcome of patients between subgroups was compared by the Kaplan-Meier method with a two-sided log-rank test. Univariate and multivariate Cox regression models were used to verify the independent prognostic value of the risk model. The Wilcoxon rank-sum test was used to compare immune cell infiltration and immune pathway activation between different groups and clusters. A two-sided P<0.05 was considered statistically significant.
Results
DDR cluster analysis
Univariate Cox regression analysis suggested that there were 20 DDRGs associated with the OS of 1,009 CC patients in the training cohort (Figure 1A). According to the 20 DDRGs, the training cohort was clustered into C1 and C2 (Figure 1B,1C). The Kaplan-Meier survival curve showed that the OS of C2 was significantly reduced compared to that of C1 (Figure 1D). The immune score in C1 was significantly higher than that in C2, demonstrating that there were significant differences in the immune microenvironment between the two subtypes (Figure 1E).
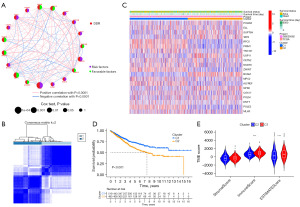
GSVA for different subtypes
The GSVA results showed that there were great differences between C1 and C2 in many biological processes related to the immune response, such as the immune response to tumor cells, diversification of immune receptors via somatic mutation, and positive regulation of the T helper type-1 (Th1) immune response (Figure 2). These results indicated that immune-related genes may play a critical role in the clinical outcomes of different DDR subtypes.
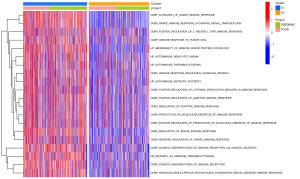
An 8 immune gene risk score predicts the prognosis of CC
On the basis of the above analysis results, we identified a total of 1,135 DEIRGs between C1 and C2 (Figure 3A,3B). As shown by the univariate Cox regression analysis, 24 DEIRGs exhibited a significant correlation with the OS of CC patients (P<0.001) (Figure 3C). Thirteen genes with nonzero LASSO regression coefficients were retained for multivariate Cox regression analysis (Figure 3D): risk score = CD36 * 0.153186265 − F2RL2 * 0.216884471 − IL17RB * 0.131787886 + INHBB * 0.228290017 + MID2 * 0.173778976 + PLEC * 0.245896502 + SEMA4C * 0.195763643 − TAPBPL * 0.283424794 (Figure 3E). The expression levels of eight genes were significantly different between C1 and C2 (Figure 4A). The Kaplan-Meier survival analysis also suggested that the expression levels of the eight genes were significantly associated with the OS of CC patients (Figure 4B). In the training cohort, the median risk score was 0.9854, which served as the unified cutoff for dividing patients equally into high- and low-risk groups. The OS of the high-risk patients was obviously lower than that of the low-risk patients (P<0.001, Figure 5A). The area under the curve (AUC) values for the risk score predicting the OS of patients at 1, 3 and 5 years were 0.691, 0.687, and 0.667, respectively (Figure 5B). The high- and low-risk groups were well separated into two clusters, as shown in the principal component analysis (PCA) and t-distributed stochastic neighbor embedding (t-SNE) (Figure 5C,5D). Low-risk patients were found to have lower death rates and longer survival times than high-risk patients (Figure 5E,5F). The training cohort was divided into six subgroups according to the clinical features presented in the heatmap (Figure 6A). Significant differences were found in OS between the high- and low-risk groups regardless of the patient’s sex, age and pathologic stage (Figure 6B).
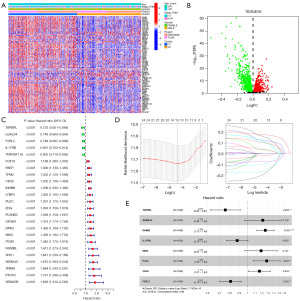
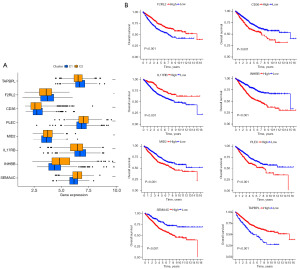
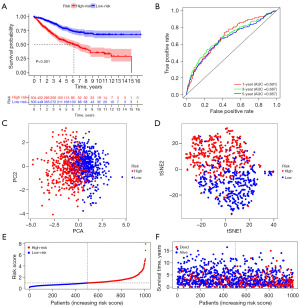
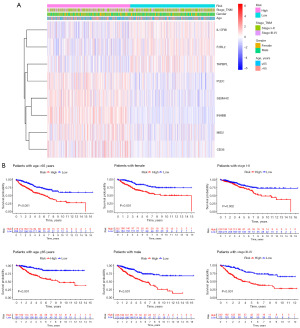
Internal validation of the 8 immune gene risk score in the TCGA and GSE39582 cohorts
Significant differences in OS, disease-specific survival (DSS), disease-free survival (DFS), and progression-free survival (PFS) were detected between the high- and low-risk groups in the TCGA cohort (Figure 7), and OS, DSS, DFS, and PFS in the high-risk group were all notably lower than those in the low-risk group (P<0.001, Figure 7A,7D,7G,7J). The AUC values of 1-, 3- and 5-year OS predicted by the risk score were 0.709, 0.671, and 0.630, respectively (Figure 7B). The AUC values of 1-, 3- and 5-year DSS predicted by the risk score were 0.741, 0.732, and 0.728, respectively (Figure 7E). The AUC values of 1-, 3- and 5-year DFS predicted by the risk score were 0.685, 0.731, and 0.735, respectively (Figure 7H). The AUC values of 1-, 3- and 5-year PFS predicted by the risk score were 0.694, 0.718, and 0.709, respectively (Figure 7K). The risk score was an independent indicator to predict OS, DSS, DFS, and PFS, as revealed by the univariate and multivariate Cox regression analyses (Figure 7C,7F,7I,7L). In the GSE14520 cohort (Figure 8), the OS and relapse-free survival (RFS) of CC patients in the high-risk group showed a notable decrease compared to the OS and RFS of CC patients in the low-risk group (P<0.001, Figure 8A,8D). The AUC values of 1-, 3- and 5-year OS predicted by the risk score were 0.675, 0.685, and 0.671, respectively (Figure 8B). The AUC values of 1-, 3- and 5-year RFS predicted by the risk score were 0.655, 0.653, and 0.642, respectively (Figure 8E). As revealed by the univariate and multivariate Cox regression analyses, the risk score was an independent predictor for OS and RFS (Figure 8C,8F).
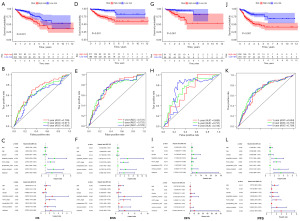
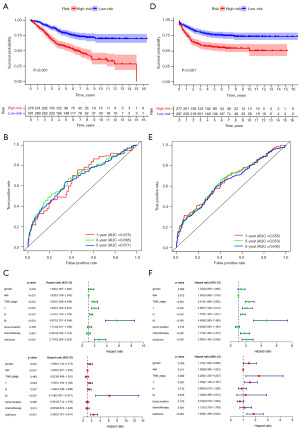
The potential molecular mechanism of the prognostic signature
To explore the potential molecular mechanisms of the prognostic signature, we identified the DEGs between different risk groups (Figure 9A). The GO function annotation of DEGs was mainly involved in extracellular structure organization, extracellular matrix organization, regulation of angiogenesis, and positive regulation of endothelial cell proliferation (Figure 9B). The Kyoto Encyclopedia of Genes and Genomes (KEGG) pathways related to cancer, such as colorectal cancer, pancreatic cancer, glioma, and melanoma, were positively enriched in the high-risk group (Figure 9C). The hallmarks correlated with epithelial-mesenchymal transition (EMT), angiogenesis, hypoxia, P53, TGF-β, KRAS signaling, etc., were upregulated in the high-risk group (Figure 9D). The DDR-related functional pathways, including HR, MMR, BER, and NER, were positively correlated with the low-risk group (Figure 9E).
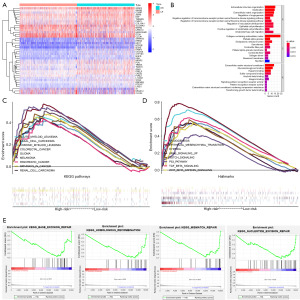
External validation of the prognostic signature in the GSE17538 cohort
Using the calculation formula constructed in the training cohort, we calculated the risk score of patients in the GSE17538 cohort (n=232), and the patients’ death rates gradually increased with increasing risk score (Figure 10A,10B). Based on the unified cutoff (0.9854), the patients were classified into two subgroups: high- and low-risk groups. Significantly reduced OS, DSS, and DFS were exhibited in the high-risk patients relative to the low-risk patients (Figure 10C-10E). Good predictive efficacy was displayed in the ROC curve for our signature, especially for DFS (AUC =0.630 for 1 year, 0.659 for 3 years, and 0.693 for 5 years) (Figure 10C-10E). Univariate and multivariate Cox regression analyses confirmed that the risk score capable of the ability to independently predict the OS, DSS, and DFS (Figure 10F-10H).
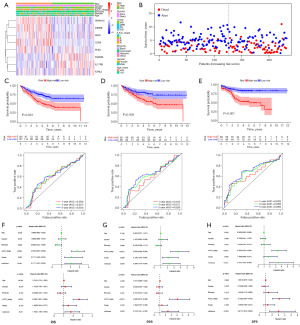
Clinical correlation analysis between different risk groups
On the basis of the clinical data of the three independent cohorts, we conducted the chi-square test between different risk groups. The results showed that the high- and low-risk groups differed significantly in lymphatic invasion, American Joint Committee on Cancer (AJCC) TNM stage, preoperative carcinoembryonic antigen (CEA) level, etc. (P<0.05, Tables 1-3).
Table 1
Clinical characteristics | High-risk (n=115), n (%) | Low-risk (n=110), n (%) | Chi-square | P value |
---|---|---|---|---|
MSI | 5.0067 | 0.0818 | ||
MSI-H | 17 (14.78) | 26 (23.64) | ||
MSI-L | 21 (18.26) | 11 (10.00) | ||
MSS | 77 (66.96) | 73 (66.36) | ||
Age | 0 | 1 | ||
≤65 years | 51 (44.35) | 48 (43.64) | ||
>65 years | 64 (55.65) | 62 (56.36) | ||
Gender | 6.1271 | 0.0133 | ||
Female | 66 (57.39) | 44 (40.00) | ||
Male | 49 (42.61) | 66 (60.00) | ||
Lymphatic invasion | 4.1149 | 0.0425 | ||
No | 60 (52.17) | 73 (66.36) | ||
Yes | 55 (47.83) | 37 (33.64) | ||
AJCC TNM stage | 18.8506 | 3.00E-04 | ||
Stage I | 13 (11.3) | 28 (25.45) | ||
Stage II | 36 (31.3) | 47 (42.73) | ||
Stage III | 38 (33.04) | 26 (23.64) | ||
Stage IV | 28 (24.35) | 9 (8.18) | ||
Preoperative CEA | 6.7255 | 0.0095 | ||
≤5 μg/L | 67 (58.26) | 83 (75.45) | ||
>5 μg/L | 48 (41.74) | 27 (24.55) | ||
Venous invasion | 0.5267 | 0.468 | ||
No | 83 (72.17) | 85 (77.27) | ||
Yes | 32 (27.83) | 25 (22.73) |
TCGA, The Cancer Genome Atlas; MSI, microsatellite instability; H, high; L, low; MSS, microsatellite stable; AJCC, American Joint Committee on Cancer; TNM, tumor, node, metastasis; CEA, carcinoembryonic antigen.
Table 2
Clinical characteristics | High-risk (n=248), n (%) | Low-risk (n=280), n (%) | Chi-square | P value |
---|---|---|---|---|
Gender | 1.1959 | 0.2741 | ||
Female | 119 (47.98) | 120 (42.86) | ||
Male | 129 (52.02) | 160 (57.14) | ||
Age | 0.0205 | 0.886 | ||
≤65 years | 99 (39.92) | 109 (38.93) | ||
>65 years | 149 (60.08) | 171 (61.07) | ||
AJCC TNM stage | 9.1005 | 0.028 | ||
I | 10 (4.03) | 26 (9.29) | ||
II | 116 (46.77) | 139 (49.64) | ||
III | 95 (38.31) | 97 (34.64) | ||
IV | 27 (10.89) | 18 (6.43) | ||
T | 17.36 | 6.00E−04 | ||
T1 | 3 (1.21) | 9 (3.21) | ||
T2 | 12 (4.84) | 34 (12.14) | ||
T3 | 167 (67.34) | 192 (68.57) | ||
T4 | 66 (26.61) | 45 (16.07) | ||
N | 5.8305 | 0.1202 | ||
N0 | 132 (53.23) | 170 (60.71) | ||
N1 | 62 (25.00) | 68 (24.29) | ||
N2 | 50 (20.16) | 41 (14.64) | ||
N3 | 4 (1.61) | 1 (0.36) | ||
M | 5.0973 | 0.0782 | ||
M0 | 219 (88.31) | 262 (93.57) | ||
M1 | 28 (11.29) | 18 (6.43) | ||
MX | 1 (0.4) | 0 (0) | ||
Tumor location | 2.7967 | 0.0945 | ||
Distal | 139 (56.05) | 178 (63.57) | ||
Proximal | 109 (43.95) | 102 (36.43) | ||
Chemotherapy | 0.0565 | 0.8122 | ||
No | 140 (56.45) | 162 (57.86) | ||
Yes | 108 (43.55) | 118 (42.14) |
AJCC, American Joint Committee on Cancer; TNM, tumor, node, metastasis.
Table 3
Clinical characteristics | High-risk (n=97), n (%) | Low-risk (n=116), n (%) | Chi-square | P value |
---|---|---|---|---|
Age | 0.0267 | 0.8701 | ||
≤65 years | 48 (49.48) | 55 (47.41) | ||
>65 years | 49 (50.52) | 61 (52.59) | ||
Gender | 0.2886 | 0.5911 | ||
Female | 44 (45.36) | 58 (50.00) | ||
Male | 53 (54.64) | 58 (50.00) | ||
Ethnicity | 4.599 | 0.2036 | ||
Black | 8 (8.25) | 4 (3.45) | ||
Caucasian | 79 (81.44) | 105 (90.52) | ||
Hispanic | 1 (1.03) | 0 (0) | ||
Other | 9 (9.28) | 7 (6.03) | ||
AJCC TNM stage | 8.1981 | 0.0421 | ||
I | 6 (6.19) | 21 (18.10) | ||
II | 28 (28.87) | 37 (31.90) | ||
III | 37 (38.14) | 33 (28.45) | ||
IV | 26 (26.80) | 25 (21.55) | ||
Grade | 3.493 | 0.3217 | ||
MD | 70 (72.16) | 89 (76.72) | ||
MPD | 5 (5.15) | 2 (1.72) | ||
PD | 16 (16.49) | 14 (12.07) | ||
WD | 6 (6.19) | 11 (9.48) |
AJCC, American Joint Committee on Cancer; TNM, tumor, node, metastasis; MD, moderately differentiated; MPD, moderate to poorly differentiated; PD, poorly differentiated; WD, well differentiated.
External validation of the prognostic signature in the GSE38832 cohort
With the same calculation formula and the unified cutoff (0.9854) obtained in the training cohort, the patients in the GSE38832 cohort (n=122) were classified into high- and low-risk groups (Figure 11A). The high-risk patients’ DSS was reduced with statistical significance relative to that of the low-risk patients (Figure 11B), and the AUC was 0.816 for 1-year, 0.768 for 3-year, and 0.667 for 5-year DSS (Figure 11C). The percent weight of AJCC stage III–IV in the high-risk group was significantly higher than that in the low-risk group (66% vs. 44%) (Figure 11D), and patients’ risk score increased with the progression of AJCC stage (Figure 11E).
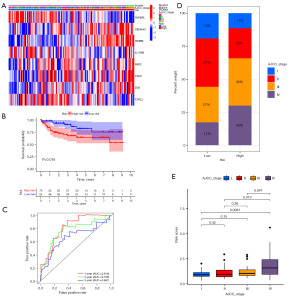
Significance of the risk score in immunotherapy
The immunophenoscore (IPS) of the TCGA dataset was acquired from The Cancer Immunome Atlas (https://tcia.at/home) (28); the higher the IPS was, the more sensitive to immune checkpoint inhibitors (ICIs), such as anti-PD-1 and CTLA4. By comparing the IPS, we found that the response of the low-risk group to ICIs was significantly stronger than that of the high-risk group (Figure 12A), indicating that the likelihood of the low-risk patients become to the responder who received immunotherapy was larger. Through the comprehensive analysis of the expression profile by Tumor Immune Dysfunction and Exclusion (TIDE) (http://tide.dfci.harvard.edu/), we found that patients with high risk had a higher potential for TIDE, which was statistically significant (Figure 12B), further confirming that high-risk patients were less likely to benefit from ICIs. To verify the above results, we applied the calculation formula and the unified cutoff (0.9854) on the patients in the IMvigor210 cohort (n=348) (Figure 13A). The high-risk patients’ OS was obviously lower than that of the low-risk group (Figure 13B). The risk score of patients who achieved objective response [complete response (CR) + partial response (PR)] after receiving immunotherapy was significantly reduced (Figure 13C), the risk score of patients with immunophenotype inflammation was significantly reduced compared to patients with immunophenotype desert and excluded (Figure 13D), and the risk score of patients with immunotherapy response was significantly lower than that of the patients with immunotherapy nonresponse (Figure 13E). The above evidence suggests that immunotherapy is an effective treatment option for patients in the low-risk group.
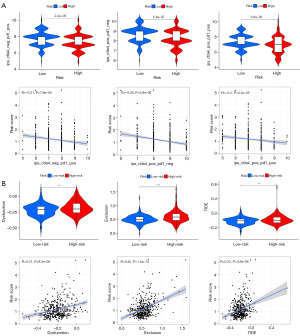
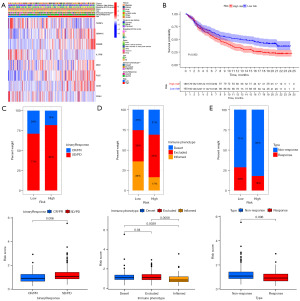
Comparison of the immune cell infiltration abundance between different risk groups
We quantified the infiltration of 23 types of immune cells in the 1,241 CC samples included in the research using the ssGSEA algorithm (Figure 14A). Through Kaplan-Meier survival analysis, four kinds of immune cells’ infiltration degree were observed to influence the OS of patients significantly, and the higher infiltration levels of gamma delta T cells (γδ T cells), immature dendritic cells, natural killer T cells, and T follicular helper (Tfh) cells resulted in adverse clinical outcomes (Figure 14B). A positive correlation was found between the risk score and the infiltration level of the above four kinds of immune cells (Figure 14C). The infiltration levels of γδ T cells, immature dendritic cells, tumor killer T cells, and Tfh cells in the high-risk group were significantly higher than those in the low-risk group (Figure 14D).
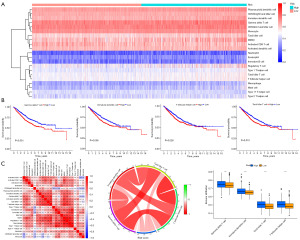
Discussion
CC is mainly caused by malignant transformation of benign lesions of the colon mucosa; its incidence closely follows those of gastric and esophageal cancers, and it is the third highest incidence among digestive tract malignant tumors (29). The number of patients dying from CC is increasing every year, and the main reason for the poor prognosis of patients with CC is that it has the characteristics of concealment, slow progression, lack of characteristic clinical manifestations, and early lymph node metastasis, among others (30). Growing evidence suggests that the process of DNA repair and the occurrence and development of cancer are inextricably linked (31-33), and its impact on the immunotherapy response of cancer patients cannot be ignored (34). However, relevant research on CC is still very scarce. To enrich the treatment strategy of CC, we carried out this study.
Key findings
In our research, the immune microenvironment (represented by the immune score) and immune pathway activity among different prognostic DDR molecular subtypes were existed significant differences were found. The OS of C2 was significantly reduced compared with that of C1, corresponding to its immune score, and the immune response was significantly lower than that of C1. Based on the above results, we preliminarily speculated that immune-related genes may be a potential factor causing the difference in prognosis of different DDR molecular subtypes of CC. Taking this clue into consideration, we identified the DEIRGs between C1 and C2, and univariate Cox regression analysis showed that 24 of 1,135 DEIRGs were significantly correlated with the OS of CC patients. After further screening by LASSO and multivariate Cox regression analysis, an 8-gene risk score was built in our training cohort. Clinically, OS, DSS, DFS, PFS and RFS are important indicators to evaluate tumor prognosis.
Strengths and limitations
Both internal and external validation proved that the risk score was an independent indicator for the prediction of OS, DSS, DFS, PFS and RFS in CC patients and that it had high precision. For patients with different clinical features, such as age, sex, and pathologic stage, the prognostic model was also applicable. But whether this model can be applied to clinical practice still needs prospective cohort study.
Comparison with similar researches
Research on these eight genes in the field of cancer is not rare; for example, Li et al. (35) found that CD36 promotes the proliferation and metastasis of hepatocellular carcinoma by activating the MEK/ERK and PI3K/Akt signaling pathways. Lupu et al. (36) found that the inhibition of F2RL2 was related to the development of mouse precancerous liver lesions. In pancreatic cancer, Song et al. (37) found that low expression of IL-17RB is associated with longer OS and DFS; however, in thyroid cancer, Ren et al. (38) found that IL-17RB could activate the expression of MMP-9 through the ERK1/2 pathway and promote the invasion and metastasis of thyroid cancer cells. Zou et al. (39) found that INHBB inhibits anoikis resistance and migration of nasopharyngeal carcinoma cells through the TGF-β signaling pathway. Wang et al. (40) found that overexpression of MID2 could promote proliferation of breast cancer cells. Hu et al. (41) found that the increase in PLEC germline copy number resulted in an increased risk of esophageal squamous cell carcinoma in Southwest China. Hou et al. (42) found that high expression of SEMA4C could promote EMT of colorectal cancer and predict poor prognosis, and it has also been reported that overexpression of SEMA4C can promote the proliferation of breast cancer and pancreatic cancer (43,44). Lin et al. (45) found that an anti-TAPBPL monoclonal antibody can neutralize the inhibitory activity of TAPBPL-Ig on T cells, enhance antitumor immunity, and inhibit tumor growth in animal models.
Explanations of findings
The underlying molecular mechanism of the signature may help to clarify the poor prognosis of the high-risk group. The GSEA results showed that the pathways involved in EMT, hypoxia and angiogenesis were abnormally active in the high-risk group, while the DDR related pathways were obviously suppressed. Current in vivo and in vitro experimental evidence suggests that EMT plays an important role in primary invasion and secondary metastasis of CC. Due to the occurrence of EMT, the cells show loss of polarity, decreased adhesion and enhanced migration ability (46,47). Tumor angiogenesis is subject to fine and complex regulation, which involves the degradation of the extracellular matrix, the proliferation and migration of vascular endothelial cells, and the formation of vascular structures and networks (48,49). The rapid proliferation of tumor cells leads to local ischemia and hypoxia, directly stimulates angiogenesis, promotes the secretion of angiogenic factors by many kinds of tissue cells, especially tumor cells, promotes the proliferation of endothelial cells, and promotes chemotaxis and the migration of endothelial cells (50,51). Thus, EMT, hypoxia and angiogenesis complement each other in the progression of CC. Although the relationship between DNA repair status and the prognosis of CC has not been determined (17), we found that DDR defects are an important molecular feature of the high-risk group. These findings may contribute to a better understanding of the pathogenesis of CC. Interestingly, through mechanistic research, we found that this signature was also related to the occurrence and development of other cancers, such as colorectal cancer, pancreatic cancer, glioma, and melanoma, which may provide new insights for the diagnosis and treatment of other malignant tumors.
Immunotherapy has become an effective means to improve the prognosis of CC patients; however, considering the side effects of immunotherapy, it is also critical to screen patients who can truly benefit from immunotherapy (52). Based on our evidence, the possibility of low-risk patients receiving a benefit from immunotherapy was obviously higher than that of the high-risk group in terms of mechanism, which is inseparable from the inflamed immunophenotype of the low-risk group (53). The higher infiltration levels of γδ T cells, immature dendritic cells, tumor killer T cells, and Tfh cells were found to have a significant adverse impact on the prognosis of CC patients. These four kinds of immune cells were all highly infiltrated in the high-risk group. A new study found that the massive infiltration of γδ T cells in pancreatic tumor tissue makes it difficult for CD4+ and CD8+ T cells to recognize and attack tumor cells (54). Immature DCs can further produce immune tolerance by inducing the body to produce regulatory T cells, anergic T cells or tolerogenic T cells (55). Tumor-infiltrating Tfh cells are related to the increase in Th1, CD8+ T and B cells producing IFN-γ in tumors and to the improvement in tumor outcome. Effective and sustainable antitumor immunity depends on the interaction between Tfh B cell response and T cell response (56). However, Tfh-like cell-mediated B cell maturation helps to create conditions for the polarization of tumor-promoting M2b macrophages in tumors (57). NK cells are inherent lymphocytes and have strong cytotoxicity in the innate immune system, accounting for 15% of all circulating lymphocytes (58). The immunosuppressive TME could damage the function, phenotype, activation and persistence of NK cells and even lead to abnormal function or failure of NK cells (59,60). The significantly positive correlation between the risk score and the infiltration level of γδ T cells, immature dendritic cells, natural killer T cells, and Tfh cells meant that we could estimate the infiltration abundance of the four kinds of immune cells by calculating the risk score. This discovery may provide new clues for immunotherapy of CC.
Implications and actions needed
In this study, we proposed an immune gene risk score for predicting the prognosis of CC based on DDR molecular subtypes. A total of 1,363 CC samples from three independent cohorts confirmed the stability of the prognostic model. Importantly, we discovered the biological characteristics of CC patients with poor prognosis by exploring the potential mechanism of this prognostic signature: activation of EMT and angiogenesis, tumor hypoxic microenvironment, defects in DDR-related pathways, and desert and excluded immunophenotypes, among others. Interestingly, the risk score is also applicable for the estimation of immunotherapy response and immune cell infiltration. These evidences may provide new insights into the comprehensive management of CC patients, but the specific function of the eight genes in CC is still not fully clarified and needs to be experimentally validated in the future.
Conclusions
An immune risk score associated with the DDR molecular subtype was built and verified in research applicable for prognosis and immune cell infiltration prediction in CC.
Acknowledgments
Funding: None.
Footnote
Reporting Checklist: The authors have completed the TRIPOD reporting checklist. Available at https://tcr.amegroups.com/article/view/10.21037/tcr-23-747/rc
Peer Review File: Available at https://tcr.amegroups.com/article/view/10.21037/tcr-23-747/prf
Conflicts of Interest: All authors have completed the ICMJE uniform disclosure form (available at https://tcr.amegroups.com/article/view/10.21037/tcr-23-747/coif). The authors have no conflicts of interest to declare.
Ethical Statement: The authors are accountable for all aspects of the work in ensuring that questions related to the accuracy or integrity of any part of the work are appropriately investigated and resolved. This study was conducted in accordance with the Declaration of Helsinki (as revise in 2013).
Open Access Statement: This is an Open Access article distributed in accordance with the Creative Commons Attribution-NonCommercial-NoDerivs 4.0 International License (CC BY-NC-ND 4.0), which permits the non-commercial replication and distribution of the article with the strict proviso that no changes or edits are made and the original work is properly cited (including links to both the formal publication through the relevant DOI and the license). See: https://creativecommons.org/licenses/by-nc-nd/4.0/.
References
- Friedberg EC. DNA damage and repair. Nature 2003;421:436-40. [Crossref] [PubMed]
- Karran P, Brem R. Protein oxidation, UVA and human DNA repair. DNA Repair (Amst) 2016;44:178-185. [Crossref] [PubMed]
- Paz-Elizur T, Sevilya Z, Leitner-Dagan Y, et al. DNA repair of oxidative DNA damage in human carcinogenesis: potential application for cancer risk assessment and prevention. Cancer Lett 2008;266:60-72. [Crossref] [PubMed]
- Lombard DB, Chua KF, Mostoslavsky R, et al. DNA repair, genome stability, and aging. Cell 2005;120:497-512. [Crossref] [PubMed]
- Sancar A, Lindsey-Boltz LA, Unsal-Kaçmaz K, et al. Molecular mechanisms of mammalian DNA repair and the DNA damage checkpoints. Annu Rev Biochem 2004;73:39-85. [Crossref] [PubMed]
- Thompson LH, Schild D. Recombinational DNA repair and human disease. Mutat Res 2002;509:49-78. [Crossref] [PubMed]
- Jeggo PA, Pearl LH, Carr AM. DNA repair, genome stability and cancer: a historical perspective. Nat Rev Cancer 2016;16:35-42. [Crossref] [PubMed]
- Helleday T, Petermann E, Lundin C, et al. DNA repair pathways as targets for cancer therapy. Nat Rev Cancer 2008;8:193-204. [Crossref] [PubMed]
- Ahmed M. Colon Cancer: A Clinician's Perspective in 2019. Gastroenterology Res 2020;13:1-10. [Crossref] [PubMed]
- Benson AB, Venook AP, Al-Hawary MM, et al. NCCN Guidelines Insights: Colon Cancer, Version 2.2018. J Natl Compr Canc Netw 2018;16:359-69. [Crossref] [PubMed]
- Vogel JD, Eskicioglu C, Weiser MR, et al. The American Society of Colon and Rectal Surgeons Clinical Practice Guidelines for the Treatment of Colon Cancer. Dis Colon Rectum 2017;60:999-1017. [Crossref] [PubMed]
- Klaver CEL, Wisselink DD, Punt CJA, et al. Adjuvant hyperthermic intraperitoneal chemotherapy in patients with locally advanced colon cancer (COLOPEC): a multicentre, open-label, randomised trial. Lancet Gastroenterol Hepatol 2019;4:761-70. [Crossref] [PubMed]
- Joost P, Bendahl PO, Halvarsson B, et al. Efficient and reproducible identification of mismatch repair deficient colon cancer: validation of the MMR index and comparison with other predictive models. BMC Clin Pathol 2013;13:33. [Crossref] [PubMed]
- Qin Q, Ying J, Lyu N, et al. Correlations between DNA mismatch repair (MMR) and prognosis and prediction of treatment efficacy in stage II/II colon cancer. Zhonghua Zhong Liu Za Zhi 2014;36:844-8. [PubMed]
- Wang Z, Zhao J, Wang G, et al. Comutations in DNA Damage Response Pathways Serve as Potential Biomarkers for Immune Checkpoint Blockade. Cancer Res 2018;78:6486-96. [Crossref] [PubMed]
- Teo MY, Seier K, Ostrovnaya I, et al. Alterations in DNA Damage Response and Repair Genes as Potential Marker of Clinical Benefit From PD-1/PD-L1 Blockade in Advanced Urothelial Cancers. J Clin Oncol 2018;36:1685-94. [Crossref] [PubMed]
- Zaanan A, Shi Q, Taieb J, et al. Role of Deficient DNA Mismatch Repair Status in Patients With Stage III Colon Cancer Treated With FOLFOX Adjuvant Chemotherapy: A Pooled Analysis From 2 Randomized Clinical Trials. JAMA Oncol 2018;4:379-83. [Crossref] [PubMed]
- Huo J, Wu L, Zang Y. Development and Validation of a Metabolic-related Prognostic Model for Hepatocellular Carcinoma. J Clin Transl Hepatol 2021;9:169-79. [Crossref] [PubMed]
- Huo J, Wu L, Zang Y. Development and validation of a CTNNB1-associated metabolic prognostic model for hepatocellular carcinoma. J Cell Mol Med 2021;25:1151-65. [Crossref] [PubMed]
- Hänzelmann S, Castelo R, Guinney J. GSVA: gene set variation analysis for microarray and RNA-seq data. BMC Bioinformatics 2013;14:7. [Crossref] [PubMed]
- Huo J, Wu L, Zang Y. Construction and Validation of a Universal Applicable Prognostic Signature for Gastric Cancer Based on Seven Immune-Related Gene Correlated With Tumor Associated Macrophages. Front Oncol 2021;11:635324. [Crossref] [PubMed]
- Huo J, Wu L, Zang Y. Construction and Validation of a Reliable Six-Gene Prognostic Signature Based on the TP53 Alteration for Hepatocellular Carcinoma. Front Oncol 2021;11:618976. [Crossref] [PubMed]
- Huo J, Wu L, Zang Y. Eleven immune-gene pairs signature associated with TP53 predicting the overall survival of gastric cancer: a retrospective analysis of large sample and multicenter from public database. J Transl Med 2021;19:183. [Crossref] [PubMed]
- Huo J, Wu L, Zang Y. Development and Validation of a Robust Immune-Related Prognostic Signature for Gastric Cancer. J Immunol Res 2021;2021:5554342. [Crossref] [PubMed]
- Huo J, Wu L, Zang Y. Eight-gene prognostic signature associated with hypoxia and ferroptosis for gastric cancer with general applicability. Epigenomics 2021;13:875-90. [Crossref] [PubMed]
- Barbie DA, Tamayo P, Boehm JS, et al. Systematic RNA interference reveals that oncogenic KRAS-driven cancers require TBK1. Nature 2009;462:108-12. [Crossref] [PubMed]
- Subramanian A, Tamayo P, Mootha VK, et al. Gene set enrichment analysis: a knowledge-based approach for interpreting genome-wide expression profiles. Proc Natl Acad Sci U S A 2005;102:15545-50. [Crossref] [PubMed]
- Charoentong P, Finotello F, Angelova M, et al. Pan-cancer Immunogenomic Analyses Reveal Genotype-Immunophenotype Relationships and Predictors of Response to Checkpoint Blockade. Cell Rep 2017;18:248-62. [Crossref] [PubMed]
- Newcomb PA, Baron J, Cotterchio M, et al. Colon Cancer Family Registry: an international resource for studies of the genetic epidemiology of colon cancer. Cancer Epidemiol Biomarkers Prev 2007;16:2331-43. [Crossref] [PubMed]
- Cappell MS. Pathophysiology, clinical presentation, and management of colon cancer. Gastroenterol Clin North Am 2008;37:1-24. v. [Crossref] [PubMed]
- Perera D, Poulos RC, Shah A, et al. Differential DNA repair underlies mutation hotspots at active promoters in cancer genomes. Nature 2016;532:259-63. [Crossref] [PubMed]
- Sabarinathan R, Mularoni L, Deu-Pons J, et al. Nucleotide excision repair is impaired by binding of transcription factors to DNA. Nature 2016;532:264-7. [Crossref] [PubMed]
- Khurana E. Cancer genomics: Hard-to-reach repairs. Nature 2016;532:181-2. [Crossref] [PubMed]
- Bever KM, Le DT. DNA repair defects and implications for immunotherapy. J Clin Invest 2018;128:4236-42. [Crossref] [PubMed]
- Li Q, Wang C, Wang Y, et al. HSCs-derived COMP drives hepatocellular carcinoma progression by activating MEK/ERK and PI3K/AKT signaling pathways. J Exp Clin Cancer Res 2018;37:231. [Crossref] [PubMed]
- Lupu DS, Orozco LD, Wang Y, et al. Altered methylation of specific DNA loci in the liver of Bhmt-null mice results in repression of Iqgap2 and F2rl2 and is associated with development of preneoplastic foci. FASEB J 2017;31:2090-103. [Crossref] [PubMed]
- Song Y, Ji B, Jiang CX, et al. IL17RB expression might predict prognosis and benefit from gemcitabine in patients with resectable pancreatic cancer. Pathol Res Pract 2019;215:152650. [Crossref] [PubMed]
- Ren L, Xu Y, Liu C, et al. IL-17RB enhances thyroid cancer cell invasion and metastasis via ERK1/2 pathway-mediated MMP-9 expression. Mol Immunol 2017;90:126-35. [Crossref] [PubMed]
- Zou G, Ren B, Liu Y, et al. Inhibin B suppresses anoikis resistance and migration through the transforming growth factor-β signaling pathway in nasopharyngeal carcinoma. Cancer Sci 2018;109:3416-27. [Crossref] [PubMed]
- Wang L, Wu J, Yuan J, et al. Midline2 is overexpressed and a prognostic indicator in human breast cancer and promotes breast cancer cell proliferation in vitro and in vivo. Front Med 2016;10:41-51. [Crossref] [PubMed]
- Hu L, Wu Y, Guan X, et al. Germline copy number loss of UGT2B28 and gain of PLEC contribute to increased human esophageal squamous cell carcinoma risk in Southwest China. Am J Cancer Res 2015;5:3056-71. [PubMed]
- Hou Y, Wang W, Zeng Z, et al. High SEMA4C expression promotes the epithelial-mesenchymal transition and predicts poor prognosis in colorectal carcinoma. Aging (Albany NY) 2020;12:21992-2018. [Crossref] [PubMed]
- Gurrapu S, Pupo E, Franzolin G, et al. Sema4C/PlexinB2 signaling controls breast cancer cell growth, hormonal dependence and tumorigenic potential. Cell Death Differ 2018;25:1259-75. [Crossref] [PubMed]
- Fei X, Jin HY, Gao Y, et al. Hsa-miR-10a-5p promotes pancreatic cancer growth by BDNF/SEMA4C pathway. J Biol Regul Homeost Agents 2020;34:927-34. [PubMed]
- Lin Y, Cui C, Su M, Silbart LK, Liu H, Zhao J, He L, Huang Y, Xu D, Wei X, Du Q, Lai L. Identification of TAPBPL as a novel negative regulator of T-cell function. EMBO Mol Med 2021;13:e13404. [Crossref] [PubMed]
- Loboda A, Nebozhyn MV, Watters JW, et al. EMT is the dominant program in human colon cancer. BMC Med Genomics 2011;4:9. [Crossref] [PubMed]
- Bates RC, Mercurio AM. The epithelial-mesenchymal transition (EMT) and colorectal cancer progression. Cancer Biol Ther 2005;4:365-70. [Crossref] [PubMed]
- Weis SM, Cheresh DA. Tumor angiogenesis: molecular pathways and therapeutic targets. Nat Med 2011;17:1359-70. [Crossref] [PubMed]
- Kerbel RS. Tumor angiogenesis. N Engl J Med 2008;358:2039-49. [Crossref] [PubMed]
- Mizukami Y, Kohgo Y, Chung DC. Hypoxia inducible factor-1 independent pathways in tumor angiogenesis. Clin Cancer Res 2007;13:5670-4. [Crossref] [PubMed]
- Jiang X, Wang J, Deng X, et al. The role of microenvironment in tumor angiogenesis. J Exp Clin Cancer Res 2020;39:204. [Crossref] [PubMed]
- Xiang B, Snook AE, Magee MS, et al. Colorectal cancer immunotherapy. Discov Med 2013;15:301-8. [PubMed]
- Chen DS, Mellman I. Elements of cancer immunity and the cancer-immune set point. Nature 2017;541:321-30. [Crossref] [PubMed]
- Daley D, Zambirinis CP, Seifert L, et al. γδ T Cells Support Pancreatic Oncogenesis by Restraining αβ T Cell Activation. Cell 2016;166:1485-1499.e15. [Crossref] [PubMed]
- Roncarolo MG, Levings MK, Traversari C. Differentiation of T regulatory cells by immature dendritic cells. J Exp Med 2001;193:F5-9. [Crossref] [PubMed]
- Gu-Trantien C, Willard-Gallo K. Tumor-infiltrating follicular helper T cells: The new kids on the block. Oncoimmunology 2013;2:e26066. [Crossref] [PubMed]
- Chen MM, Xiao X, Lao XM, et al. Polarization of Tissue-Resident TFH-Like Cells in Human Hepatoma Bridges Innate Monocyte Inflammation and M2b Macrophage Polarization. Cancer Discov 2016;6:1182-95. [Crossref] [PubMed]
- Baginska J, Viry E, Paggetti J, et al. The critical role of the tumor microenvironment in shaping natural killer cell-mediated anti-tumor immunity. Front Immunol 2013;4:490. [Crossref] [PubMed]
- Roma-Rodrigues C, Mendes R, Baptista PV, et al. Targeting Tumor Microenvironment for Cancer Therapy. Int J Mol Sci 2019;20:840. [Crossref] [PubMed]
- Hasmim M, Messai Y, Ziani L, et al. Critical Role of Tumor Microenvironment in Shaping NK Cell Functions: Implication of Hypoxic Stress. Front Immunol 2015;6:482. [Crossref] [PubMed]