Contrast between traditional and machine learning algorithms based on a urine culture predictive model: a multicenter retrospective study in patients with urinary calculi
Introduction
Over the past 10 years, numerous reports concerning disease prediction models based on urological diseases have been published (1-3). Most diagnostic prediction models are based on traditional algorithms such as logistic regression, which is one of the most widely used statistical predictive models in studies published in medical journals (4). However, the inherent limitations of logistic regression limit its accuracy as a predictive model (5). Machine learning algorithms have developed rapidly and can recognize important risk factors in nonlinear features and incorporate new data to continuously improve accuracy (6). In recent years, machine learning models are gradually being applied in the medical field, and they have provided better predictive model in cardiovascular disease, gastrointestinal cancer, and medical imaging (7-9).
A urinary tract infection is one of the common comorbidities in patients with urinary calculi. Urine culture tests can assist in guiding the use of antibiotics. Most urinary calculi patients with positive urine culture results are considered to have severe infections and require more aggressive anti-infection treatments. In the obstructive uropathy of urosepsis, every hour of delay in treatment with antibiotics reduces the mean survival rate by 7.6% (10). An accurate predictive model of urine culture based on routine examination information can help doctors make suitable judgments in a timely manner. However, existing reports concerning urine culture predictive models are based on logistic regression algorithms (11,12).
In this study, we used the data of urinary calculi patients from four clinical centers. Predictive models with different algorithms were constructed and verified through random data splitting with different ratios and number of times. The models included logistic regression and three machine learning models: random forest, adaboost, and gradient boosting decision tree (GBDT). We sought to compare the performances of different urine culture prediction models using traditional and machine learning algorithms.
We present the following article in accordance with the TRIPOD reporting checklist (13) (available at https://tau.amegroups.com/article/view/10.21037/tau-21-780/rc).
Methods
A flow diagram of the study is shown in Figure 1.
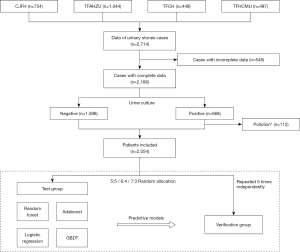
Patient population
Data from 2,714 urinary calculi patients from four clinical centers (China-Japan Friendship Hospital, The First Affiliated Hospital of Zhengzhou University, The First Affiliated Hospital of China Medical University and Tianjin First Central Hospital) between June 2016, and May 2019, were collected. The information collected included medical record information and auxiliary test results. The auxiliary examination information collected from different centers in this study were tested by the same series of instruments and were combined directly (blood biochemical test instruments: Beckman Coulter AU480, AU680, AU5800, or AU5811; urine routine instruments: Sysmex UF-500 or 1000i and Arkray Aution Max Ax-4280 or Ax-4030). Cases with incomplete data or contaminated urine culture results were excluded. For complex urinary tract infections (urinary tract stones), the colony count of clean mid-stage urine culture is >105 CFU/mL for females, and >104 CFU/mL for males, or the bacterial colony counts of urine specimens collected by the patient with catheterization >104 CFU/mL, it is considered that the urine culture result is positive. The mid-section urine culture with two or more bacteria or <104 CFU/mL is up to the physician to judge whether the urine culture result is contaminated.
A total of 2,054 cases were ultimately included in the study. Data were randomly split into test and verification sets in ratios of 5:5, 6:4, and 7:3, respectively, using random functions in Python. Each test/verification ratio was repeated five times independently for cross-validation. The outcome predicted by the prediction model is a positive or negative urine culture of the patient.
Logistic regression model
Logistic regression can quantify each independent predictor’s unique contribution to analyze the effect on a binary outcome and is a useful and established tool in medicine (14). Linear associations were assumed to exist between predictors and the outcome. Multiple regression analysis was used to identify collinearity among predictors. Variance inflation factors (VIF) <5.0 were considered to have little collinearity. We incorporated continuous predictors directly into the model’s construction to reduce the potential of poor predictive performance (15). Finally, the model was calibrated via the Hosmer-Lemeshow test (P values >0.05 were considered as an indicator of a well-calibrated model). A nomogram was constructed to predict the risk of positive urine culture based on risk factors, with a significance level of 5%.
Machine learning models
We constructed three machine learning models, including random forest, adaboost, and GBDT. Random forest is a supervised learning algorithm based on a decision tree. In short, the bootstrap method is randomly repeatedly used to select M training sets and construct a decision tree. Then the samples are trained and predicted by using multiple decision trees (16). Adaboost is an iterative algorithm. Its core purpose is to train different weak classifiers on the same training set. After each round of learner training, the weights on the incorrectly classified samples are increased. Finally, these weak classifiers are combined to form a strong classifier as a predictive model (17). GBDT is an iterative decision tree algorithm. The main concept of this algorithm is that weak classifiers are identified through multiple iterations. Then, each classifier is trained on the residuals of the previous classifier. The weak classifiers identified by each round of training are weighted and summed to obtain a total classifier (18).
We select and adapt the features of the machine learning model based on our clinical experience. To improve the ability to generalize and balance the two classes, SMOTE algorithmic was employed to counter the class imbalance. Then, we used grid search and adjusted the hyperparameter of the model to construct high-quality models.
Performances verification and comparison of models
Matthews correlation coefficient (MMC), F1-score, and receiver operating characteristic (ROC) curve and the area under curve (AUC) were used to evaluate the performance of each predictive model. Different models, built on the same batch of test sets, were validated using the same batch of validation datasets. The additive net reclassification index (NRI) and absolute NRI were used to assess the differences in predictive capabilities between models. Additive NRI and absolute NRI >0 were considered to have better predictive ability (19).
Statistical analysis
Continuous variables are expressed as mean ± standard deviation. Chi-square tests were conducted to analyze differences between groups. The R language (version 3.5.2) was used for conventional analyses and logistic regression. Python 3.8.1 for Windows was used for machine learning analysis. P<0.05 was considered statistically significant.
Ethical statement
The study was conducted in accordance with the Declaration of Helsinki (as revised in 2013). The study was approved by the ethics committees of China-Japan Friendship Hospital (No. 2019-187-K128), Tianjin the First Central Hospital (No. 2018N018KY), the First Affiliated Hospital of Zhengzhou University (No. 2018-ky-72), the First Affiliated Hospital of China Medical University (No. 2018-291-2). Informed consent was waived for this retrospective study, which does not involve any intervention treatment measures.
Results
Patients’ characteristics
A total of 2,054 cases from four clinical centers were ultimately included in the study. The patients had a mean age of 51.3±13.8 years and a positive urine culture rate of 22.3%. The association between urine culture results and main clinical characteristics showed that females with 60 years and above, hypertension, diabetes, coronary heart disease, and smoking habits had a significant association to positive outcomes of urine culture. Detailed results are summarized in Table 1 (20).
Table 1
Main clinical characteristic | Number of patients | Urine culture | P | |
---|---|---|---|---|
Positive (%) | Negative (%) | |||
Total | 2,054 | 456 (77.8%) | 1,598 (22.2%) | |
General information | ||||
Sex | ||||
Male vs. Female | 1,355 vs. 699 | 194 (14.3%) vs. 437 (62.5%) | 1,161 (85.7%) vs. 262 (37.5%) | <0.01* |
Age | ||||
<60 vs. ≥60 | 1,454 vs. 600 | 275 (18.9%) vs. 81(30.1%) | 1,179 (81.1%) vs. 419 (69.8%) | <0.01* |
BMI# | ||||
<23 vs. ≥23 | 454 vs. 1,599 | 87 (19.2%) vs. 369 (23.1%) | 367 (80.8%) vs. 1,230 (76.9%) | 0.08 |
Past history | ||||
Hypertension | ||||
Yes vs. No | 599 vs. 1,455 | 159 (26.5%) vs. 297 (20.4%) | 440 (73.5%) vs. 1,158 (79.6%) | <0.01* |
Diabetes | ||||
Yes vs. No | 300 vs. 1,754 | 81 (27.0%) vs. 375 (21.4%) | 219 (73.0%) vs. 1,379 (78.6%) | 0.03* |
Coronary heart disease | ||||
Yes vs. No | 98 vs. 1,956 | 34 (34.7%) vs. 422 (21.6%) | 64 (65.3%) vs. 1,534 (78.4%) | <0.01* |
History of abdominal/pelvic surgery | ||||
Yes vs. No | 260 vs. 1,794 | 69 (26.5%) vs. 387 (21.6%) | 191 (73.5%) vs. 1,407 (78.4%) | 0.07 |
History of cerebrovascular disease | ||||
Yes vs. No | 43 vs. 2,011 | 9 (21.0%) vs. 447 (22.2%) | 34 (79.1%) vs. 1,564 (77.8%) | 0.84 |
Malformation of urinary system | ||||
Yes vs. No | 120 vs. 1,934 | 30 (25.0%) vs. 426 (22.0%) | 90 (75.0%) vs. 1,508 (78.0%) | 0.45 |
Personal history | ||||
Smoking | ||||
Yes vs. No | 552 vs. 1,502 | 146 (26.5%) vs. 310 (20.6%) | 406 (73.6%) vs. 1,192 (79.4%) | <0.01* |
Drinking | ||||
Yes vs. No | 130 vs. 1,924 | 27 (20.8%) vs. 429 (22.3%) | 103 (79.2%) vs.1,495 (77.7%) | 0.69 |
*, P<0.05. BMI, body mass index; vs., versus; #, BMI was divided according to national surveys to fit Chinese actual situation (20). Chi-square tests.
Model construction
A logistic regression model was constructed repeatedly on each test set. Table S1 lists the effect-size estimates of predictors in association with the risk of positive urine culture in a typical example (Test No. 3 at a ratio of 6:4). A univariate analysis showed that sex, age, diabetes, symptom, smoking, urine potential of hydrogen potential of hydrogen (PH), nitrite (NIT), urine white blood cell (WBC), and urine bacteriuria (BACT) are the risk factors for positive urine culture. With the exception of diabetes and urine PH, other variables still have a statistically significant difference in the multivariate analysis. The multiple regression analysis suggested less collinearity among the predictors (all VIFs <5.0, range, 1.01–1.18). Finally, a nomogram model, used to predict the risk of positive urine culture, was constructed based on risk factors at a significance level of 5% (Figure 2A). The Hosmer-Lemeshow test showed that the model was well-calibrated (χ2=7.03, P=0.53).
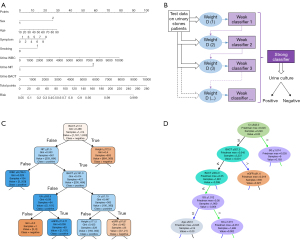
For the machine learning models, 42 features were selected to construct the models, including sex, age, and smoking habits (Table S2). The hyperparameter values of the final model are listed in Table S3. The visualization of three machine learning models is shown in Figure 2B-2D. It should be noted that the visual model only shows a part of the leaves or principles of the decision tree and does not represent the entire model. The 10 most important features of each machine learning model were identified and plotted (Figure 3A). Urine BACT was the most important feature among the three machine learning models.
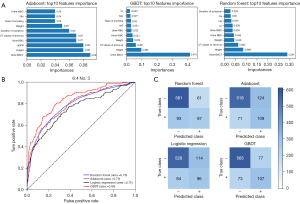
Model performance and comparison
Test No. 3, with a ratio of 6:4, is a typical example of model performance. Compared to logistic regression model, the MMC (0.53 vs. 0.38) and F1 score (0.65 vs. 0.54) of GBDT model were improved. The AUCs of the logistic regression, random forest, adaboost, and GBDT models were 0.75, 0.78, 0.79, and 0.84, respectively (Figure 3B). The GBDT model had an AUC that was 0.09 higher than that of the logistic regression model. The confusion matrix was plotted and also suggested that the GBDT model had the best predictive performance among all models (Figure 3C). The additive NRI and absolute NRI for the GBDT and logistic regression models were estimated at 0.119 and 0.058, respectively.
The statistical information of all models is summarized in Table 2. The mean AUCs of the logistic regression, random forest, adaboost, and GBDT models were 0.761 (95% CI: 0.753–0.770), 0.790 (95% CI: 0.782–0.798), 0.779 (95% CI: 0.766–0.791), and 0.831 (95% CI: 0.823–0.840), respectively. The GBDT model had the highest average AUC among all models, 0.07 higher than that of the logistic regression model. Moreover, the average MMC and F1-score of GBDT model was 0.460 and 0.588, which was improved compared to logistic regression model of 0.335 and 0.501. The additive NRI and absolute NRI of the GBDT and logistic regression models were 0.124 (95% CI: 0.106–0.142) and 0.065 (95% CI: 0.060–0.069), respectively.
Table 2
Models | AUC | MMC | F1-core | Additive NRI* | Absolute NRI* | |||||||||
---|---|---|---|---|---|---|---|---|---|---|---|---|---|---|
Average | 95% CI | Average | 95% CI | Average | 95% CI | Average | 95% CI | Average | 95% CI | |||||
Logistic regression | 0.761 | 0.753–0.770 | 0.335 | 0.322–0.348 | 0.501 | 0.490–0.512 | – | – | – | – | ||||
Random forest | 0.790 | 0.782–0.798 | 0.406 | 0.395–0.417 | 0.530 | 0.521–0.540 | 0.020 | 0.004–0.035 | 0.065 | 0.057–0.065 | ||||
Adaboost | 0.779 | 0.766–0.791 | 0.385 | 0.369–0.400 | 0.536 | 0.523–0.549 | 0.051 | 0.036–0.159 | 0.023 | 0.016–0.030 | ||||
GBDT | 0.831 | 0.823–0.840 | 0.460 | 0.446–0.475 | 0.588 | 0.575–0.601 | 0.124 | 0.106–0.142 | 0.065 | 0.060–0.069 |
*, additive NRI and absolute NRI were calculated by comparing machine learning models to the logistic regression model. AUC, area under curve; 95% CI, 95% confidence interval; MMC, the Matthews correlation coefficient; NRI, net reclassification index; GBDT, gradient boosting decision tree.
Discussion
The most obvious finding that emerged from this study is that the machine learning algorithms performed better than the traditional algorithm, based on a urine culture predictive model in patients with urinary calculi. The predictive accuracy of the GBDT model was, on average, 7% higher than that of the logistic regression model. Meaning while, the average MMC of GBDT model was more improved compared to logistic regression model. To the best of our knowledge, this is the first research conducted with data from multiple centers to evaluate the performance of machine learning algorithms for diagnostic predictive models of urine culture.
Quick and accurate identification of urinary calculi patients with positive urinary cultures, who sometimes require aggressive antibiotic management in a timely manner, is a major challenge for urologists. As a gold standard, urine culture testing has high requirements for operation and is time-consuming. A predictive model based on conventional clinical information is one of the better solutions. However, current reports concerning urine culture predictive models are based on logistic regression algorithms, and their results are insufficiently accurate as a consequence of the algorithm’s inherent limitations (11,21). One of the prerequisites to determine the accuracy of the logistic regression algorithm is the existence of a linear relationship between predictors and the outcome, but a typical linear relationship is uncommon in clinical practice. Meanwhile, some models do not consider the points of collinearity among predictors, which also affects the performance of the model. In addition, in the performance evaluation of the model, although the AUC area of GBDT is slightly larger than that of logistic regression model in our study, we are more concerned that the MMC has been significantly improved. MMC, which is an important adapted metrics, can better measure the model prediction ability of unbalanced data sets. In this study, we found that the MMC scores of machine learning models represented by GBDT are better than Logistic regression model, suggesting that machine learning models are more suitable to apply in unbalancing dataset. Unbalanced data sets are very common in medical databases. While paying attention to the accuracy of the model, we should pay more attention to the imbalance of data sets to avoid arrived at imbalanced models. Therefore, we believe that our urine culture predictive model based on GBDT model has better performance and has high clinical application value clinical application value. Notably, new technologies for urine culture outcome prediction with more sensitivity and speed have been reported in recent years, such as specific reverse transcriptase amplification, plasmonic nanosensors, and molecular chip-based diagnostic systems, but these technologies still require significantly more development before application in clinical practice (22-24).
The characteristics of machine learning algorithms are that they allow the use of as many features as desired from all available data to construct individual predictive models in a nonlinear pattern, and they do not need to consider the collinearity among features. These characteristics are the main differences from the logistic regression algorithm, which is “hypothesis-driven”. Machine learning models can also identify the importance of different features in the modeling process. Additionally, by continuously merging and learning new data, machine learning models can autonomously optimize and improve predictive performance.
Machine learning algorithms are widely used in many fields, such as financial management and environmental prediction, and are gradually becoming utilized in medicine. Articles published in recent years show that machine learning models have excellent performance when used in cardiovascular disease detection, medical imaging identification, and other areas (7-9), and some studies have applied these models in urology. It has been reported that an artificial neural network can enhance the accuracy of predicting posterior urethral obstruction with lower urinary tract symptoms, but only 201 patients from one center were included (25). However, an artificial neural network usually requires substantial amounts of data, at least thousands of samples, to build a stable model. Another study reported that random forest models can assist in enhancing the accuracy of predicting the success of shock wave lithotripsy (26). The study included 51 patients but did not compare the performance between different models. In short, lack of samples limits the generalizability of the results. Therefore, as the research sample size increases and models improve, we believe that the application of machine learning models has a high potential for impact in the field of urology.
However, there are different opinions concerning the values between logistic regression algorithm and machine learning model. A meta-analysis including 71 articles published between 1/2016 and 7/2018 stated that no significant difference was discovered between the machine learning model and the logistic regression algorithm (27). The algorithms used in prior studies in the literature were mainly classification trees (42%) and random forests (28.39%). The GBDT algorithm, which has achieved excellent results in this study, was under-evaluated. Choosing a suitable model and adjusting the model hyperparameters appropriately are important factors in building a high-quality model. The feature selection and hyperparameter values used in model construction in our study were optimized based on the authors’ clinical experiences, leading to great performance from the machine learning models compared to traditional algorithms.
The improvement in predictive accuracy is of great significance. For example, compared with laparoscopic technique, the accuracy of Da Vinci robot surgery is higher, so the benefit to patients is immense. It is clear that this method can serve as the basis for further research and may alter clinical practice in the near future. By connecting directly to the electronic medical record system, urologists can obtain predictions of urine cultures from machine learning models in a timely manner as a treatment reference.
There are some limitations to this study. First, the samples for model training are still insufficient compared with machine learning models in other fields. Second, models should be trained regularly to avoid degradation in accuracy caused by the so-called concept drift. Additionally, machine learning models is called black-box models. It is hard to explain its specific statistical mode (28), thus limiting the promotion of these models in the medical field.
Conclusions
Machine learning algorithms may be useful tools for urine culture outcome prediction in patients with urinary calculi because they exhibit superior performance compared with the logistic regression model. Prospective studies with larger sample sizes are required for further verification.
Acknowledgments
We wish to thank Dr. Li Wenliang, who was one of the first doctors to warn of the new infectious disease epidemic and eventually died of COVID-19 infection on February 7, 2020. His deed will always remind us that being a doctor, no matter what you encounter, never forget your original aspiration. We sincerely thank Zeng Fan, a hard-working and down-to-earth programmer, for providing technical support for this study.
Funding: This research received a grant from “Taihu Talents Program”, Medical and Health High-level Talents Project in Wuxi, China.
Footnote
Reporting Checklist: The authors have completed the TRIPOD reporting checklist. Available at https://tau.amegroups.com/article/view/10.21037/tau-21-780/rc
Data Sharing Statement: Available at https://tau.amegroups.com/article/view/10.21037/tau-21-780/dss
Conflicts of Interest: All authors have completed the ICMJE uniform disclosure form (available at https://tau.amegroups.com/article/view/10.21037/tau-21-780/coif). Xuesong Li and Liqun Zhou both serve as the unpaid editorial board members of Translational Andrology and Urology. The other authors have no conflicts of interest to declare.
Ethical Statement: The authors are accountable for all aspects of the work in ensuring that questions related to the accuracy or integrity of any part of the work are appropriately investigated and resolved. The study was conducted in accordance with the Declaration of Helsinki (as revised in 2013). The study was approved by the ethics committees of China-Japan Friendship Hospital (No. 2019-187-K128), Tianjin the First Central Hospital (No. 2018N018KY), the First Affiliated Hospital of Zhengzhou University (No. 2018-ky-72), the First Affiliated Hospital of China Medical University (No. 2018-291-2). Informed consent was waived for this retrospective study, which did not involve any intervention treatment measures.
Open Access Statement: This is an Open Access article distributed in accordance with the Creative Commons Attribution-NonCommercial-NoDerivs 4.0 International License (CC BY-NC-ND 4.0), which permits the non-commercial replication and distribution of the article with the strict proviso that no changes or edits are made and the original work is properly cited (including links to both the formal publication through the relevant DOI and the license). See: https://creativecommons.org/licenses/by-nc-nd/4.0/.
References
- Fujii Y. Prediction models for progression of non-muscle-invasive bladder cancer: A review. Int J Urol 2018;25:212-8. [Crossref] [PubMed]
- Osses DF, Roobol MJ, Schoots IG. Prediction Medicine: Biomarkers, Risk Calculators and Magnetic Resonance Imaging as Risk Stratification Tools in Prostate Cancer Diagnosis. Int J Mol Sci 2019;20:1637. [Crossref] [PubMed]
- Klatte T, Rossi SH, Stewart GD. Prognostic factors and prognostic models for renal cell carcinoma: a literature review. World J Urol 2018;36:1943-52. [Crossref] [PubMed]
- Nick TG, Campbell KM. Logistic regression. Methods Mol Biol 2007;404:273-301. [Crossref] [PubMed]
- Gutiérrez-Pérez GL, Mesones-Tello JP, Medina-Conde BB, et al. About conditions of application of logistic regression. Rev Psiquiatr Salud Ment 2017;10:216. [PubMed]
- Waljee AK, Higgins PD. Machine learning in medicine: a primer for physicians. Am J Gastroenterol 2010;105:1224-6. [Crossref] [PubMed]
- Motwani M, Dey D, Berman DS, et al. Machine learning for prediction of all-cause mortality in patients with suspected coronary artery disease: a 5-year multicentre prospective registry analysis. Eur Heart J 2017;38:500-7. [PubMed]
- Singal AG, Mukherjee A, Elmunzer BJ, et al. Machine learning algorithms outperform conventional regression models in predicting development of hepatocellular carcinoma. Am J Gastroenterol 2013;108:1723-30. [Crossref] [PubMed]
- Yasaka K, Akai H, Kunimatsu A, et al. Deep learning with convolutional neural network in radiology. Jpn J Radiol 2018;36:257-72. [Crossref] [PubMed]
- Dreger NM, Degener S, Ahmad-Nejad P, et al. Urosepsis-etiology, diagnosis, and treatment. Dtsch Arztebl Int 2015;112:837-47. [PubMed]
- Jones CW, Culbreath KD, Mehrotra A, et al. Reflect urine culture cancellation in the emergency department. J Emerg Med 2014;46:71-6. [Crossref] [PubMed]
- Richards KA, Cesario S, Best SL, et al. Reflex urine culture testing in an ambulatory urology clinic: Implications for antibiotic stewardship in urology. Int J Urol 2019;26:69-74. [Crossref] [PubMed]
- Collins GS, Reitsma JB, Altman DG, et al. Transparent reporting of a multivariable prediction model for individual prognosis or diagnosis (TRIPOD): the TRIPOD statement. BMC Med 2015;13:1. [Crossref] [PubMed]
- Stoltzfus JC. Logistic regression: a brief primer. Acad Emerg Med 2011;18:1099-104. [Crossref] [PubMed]
- Collins GS, Ogundimu EO, Cook JA, et al. Quantifying the impact of different approaches for handling continuous predictors on the performance of a prognostic model. Stat Med 2016;35:4124-35. [Crossref] [PubMed]
- Breiman L. Random Forests. Mach Learn 2001;45:5-32. [Crossref]
- Freund Y, Schapire RE. A decision-theoretic generalization of on-line learning and an application to boosting. Journal of Computer and System Sciences 1997;55:119-39. [Crossref]
- Friedman JH. Greedy function approximation: a gradient boosting machine. Ann Statist 2001;29:1189-232. [Crossref]
- Pencina MJ, D'Agostino RB Sr, D'Agostino RB Jr, et al. Evaluating the added predictive ability of a new marker: from area under the ROC curve to reclassification and beyond. Stat Med 2008;27:157-72; discussion 207-12. [Crossref] [PubMed]
- Tian Y, Jiang C, Wang M, et al. BMI, leisure-time physical activity, and physical fitness in adults in China: results from a series of national surveys, 2000-14. Lancet Diabetes Endocrinol 2016;4:487-97. [Crossref] [PubMed]
- Munigala S, Rojek R, Wood H, et al. Effect of changing urine testing orderables and clinician order sets on inpatient urine culture testing: Analysis from a large academic medical center. Infect Control Hosp Epidemiol 2019;40:281-6. [Crossref] [PubMed]
- Chen J, Xu Y, Yan H, et al. Sensitive and rapid detection of pathogenic bacteria from urine samples using multiplex recombinase polymerase amplification. Lab Chip 2018;18:2441-52. [Crossref] [PubMed]
- Santopolo G, Doménech-Sánchez A, Russell SM, et al. Ultrafast and Ultrasensitive Naked-Eye Detection of Urease-Positive Bacteria with Plasmonic Nanosensors. ACS Sens 2019;4:961-7. [Crossref] [PubMed]
- Li N, Lu Y, Cheng J, et al. A self-contained and fully integrated fluidic cassette system for multiplex nucleic acid detection of bacteriuria. Lab Chip 2020;20:384-93. [Crossref] [PubMed]
- Abdovic S, Cuk M, Cekada N, et al. Predicting posterior urethral obstruction in boys with lower urinary tract symptoms using deep artificial neural network. World J Urol 2019;37:1973-9. [Crossref] [PubMed]
- Mannil M, von Spiczak J, Hermanns T, et al. Three-Dimensional Texture Analysis with Machine Learning Provides Incremental Predictive Information for Successful Shock Wave Lithotripsy in Patients with Kidney Stones. J Urol 2018;200:829-36. [Crossref] [PubMed]
- Christodoulou E, Ma J, Collins GS, et al. A systematic review shows no performance benefit of machine learning over logistic regression for clinical prediction models. J Clin Epidemiol 2019;110:12-22. [Crossref] [PubMed]
- Rajkomar A, Dean J, Kohane I. Machine Learning in Medicine. N Engl J Med 2019;380:1347-58. [Crossref] [PubMed]