Investigation of the correlation between the change in the projected lung area and forced vital capacity using biphasic chest dynamic digital radiography: a cross-sectional study
Introduction
The routine pulmonary function test (PFT) is currently the gold standard for measuring pulmonary function (1). However, patients with severe myocardial infarction, unstable cardiac function, uncontrolled hypertension, an inability to cooperate, and other limiting factors, cannot undergo the PFT. Many studies have been conducted worldwide to examine the correlation between the quantitative pulmonary indicators of computed tomography (CT) scans and conventional pulmonary function indicators (2-4). However, these studies are still in the research and verification stage, and their findings have not been applied to clinical practice. In recent years, with the application of dynamic digital radiography (DDR) equipment, some researchers have conducted studies on diaphragmatic motion and pulmonary ventilation function using DDR and concluded that DDR chest radiographs can be used to quantitatively analyze diaphragmatic motion in chronic obstructive pulmonary disease (COPD) patients and to dynamically evaluate the diaphragmatic function in COPD patients simply and effectively (5-8).
Many studies have examined the correlation between certain pulmonary function parameters and DDR images. Ohkura et al. (9) examined the correlation between the change in the projected lung area (ΔPLA) on digital radiography (DR) images and residual volume/total lung capacity (RV/TLC) and forced expiratory volume in 1 second (FEV1) in patients with airflow limitation. Hino et al. (10) found that the ΔPLA measured by DR images in healthy participants was significantly correlated with their pulmonary function indicators [vital capacity (VC) and FEV1], highlighting its potential as an alternative testing method for those who are not suitable for the routine PFT. Ueyama et al. (11) also found that the VC (ΔPLA) measured by DR chest radiographs was correlated with forced vital capacity (FVC) in patients with interstitial lung disease (ILD) and attempted to develop a prediction model to predict other pulmonary function parameters of ILD. A preliminary study (12) reported that dynamic chest radiography (DCR) demonstrated significant improvements in hemidiaphragm excursion and PLA in people with cystic fibrosis who had commenced elexacaftor/tezacaftor/ivacaftor. The results observed by DCR are physiologically plausible and correlate well with spirometry.
In this study, biphasic chest DR images were obtained by DDR, one with deep inhalation and the other with deep exhalation. The participants in this study included both healthy individuals and patients with lung disease. By studying the correlation between the ΔPLA and FVC measured in two images, a DDR-FVC estimation model and a ΔPLA prediction value model were established. The FVC was estimated using the DDR-FVC estimation model, and the ratio of the measured ΔPLA value to the predicted ΔPLA value (ΔPLA%pred) was further applied to evaluate the FVC for anomalies. This study sought to provide a new and operable method for evaluating pulmonary function in patients who cannot undergo the conventional PFT, to simplify and enrich the FVC methods for assessing disease severity and efficacy, and to provide a possible supplementary method for the PFT. We present this article in accordance with the STROBE reporting checklist (available at https://qims.amegroups.com/article/view/10.21037/qims-23-1186/rc).
Methods
Participants
This prospective study was approved by the Research Ethics Review Committee of The First Affiliated Hospital of Guangzhou Medical University (No. 106 of 2022), and all the participants signed informed consent forms. The study was conducted in accordance with the Declaration of Helsinki (as revised in 2013). Between April 2022 and February 2023, 143 participants at The First Affiliated Hospital of Guangzhou Medical University were recruited to undergo PFT and DDR examinations for routine health care or clinical screenings of chest lesions. To be eligible for inclusion in this study, the patients had to meet the following inclusion criteria: (I) be aged 20–85 years; (II) not be pregnant or lactating; (III) have planned to undergo a routine chest DR physical examination; and (IV) have undergone the PFT within 1 week during the same period. Patients were excluded from the study if they met any of the following exclusion criteria: (I) were unable to understand and cooperate with the chest DDR examination; (II) did not undergo PFT or had no PFT report available; and/or (III) refused to sign the informed consent form.
Ultimately, 139 participants were enrolled in the study. According to the classification of respiratory diseases, there were 68 cases of normal pulmonary imaging, 36 cases of COPD, 8 cases of ILD, 6 cases of diaphragmatic paralysis, and 3 cases of space-occupying lesions in the lung. Additionally, there were another 10 cases of infectious pulmonary disease. Based on the PFT results, there were 50 cases of normal ventilation, 27 cases of small airway obstruction, 29 cases of obstructive ventilation, and 19 cases of restrictive ventilation. Further, mixed ventilatory dysfunction was observed in 14 patients. The FVC actual measured value was used as a grouping index. According to the guidelines for pulmonary function examination (13,14), the lung volume parameters and ventilation function parameters were reduced to abnormal, and the percentage of measured values in the normal range was ≥80%. The participants were allocated to the normal FVC group if the ratio of measured/predicted FVC values (FVC%pred) was ≥80% and to the abnormal FVC group if the FVC%pred was<80%.
Equipment
The following equipment was used: (I) X-ray imaging equipment: AeroDR C80 Digital Radiography X-Ray System (Konica Minolta, Tokyo, Japan); (II) image measurement equipment: Dynamic DR Equipment with IWS Dynamic Image Processing Workstation Software DI-X1 (Ver.1.2; Konica Minolta); and (III) PFT equipment: MasterScreen PFT System (Jaeger, Wurzburg, Germany).
Radiographic methods
The participants were radiographed in DDR ventilation mode in the standing position, and the forced breathing mode captured, under a state of deep inspiration, the process of expiring at the fastest rate and then immediately inspiring deeply. The following radiographic parameters were established based on previous research findings (9,10): 180 cm source-image distance, 110 kV tube voltage, 100 mA tube current, 5 ms pulse X-ray duration, an additional filter of 1 mm aluminum + 0.5 mm copper, and dynamic image data captured at 15 frames/second synchronized with the pulse X-rays. Images were sent to the dynamic image processing workstation after the radiography was completed.
Area measuring methods
On the dynamic image processing workstation provided with the DDR equipment, the horizontal axis represents the time (unit: frame, 1 frame =1/15 second), and the vertical axis represents the amplitude of diaphragmatic motion (unit: mm) according to the diaphragmatic motion curve. The maximum PLA (Figure 1A) was determined by finding the frame with the lowest position of the diaphragm at the end of deep inspiration (Figure 1B), and the minimum PLA (Figure 1C) was determined by finding the frame with the highest position of the diaphragm at the end of deep expiration (Figure 1D). The PLA (enclosed by the medial edge of the ribs, the diaphragm, and the mediastinal margin) was automatically outlined. Due to the precise measurement requirements, the outlined range was determined, any necessary adjustments were made, and the outlined range was confirmed through consultation with two senior technicians with 15 years of work experience, and the corresponding ΔPLA was finally automatically calculated by the software (Figure 1).
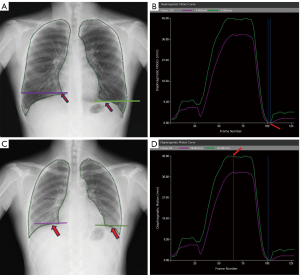
PFT methods
All the participants underwent the PFT in the same week as the chest DDR, which met the requirements of the American Thoracic Society 2019 guidelines for pulmonary ventilation function tests (15), and their measured FVC, predicted FVC, FVC%pred, forced expiratory volume in one second (FEV1), predFEV1, FEV1%pred, maximum vital capacity (VC MAX), predVC MAX, VC MAX%pred, forced inspiratory vital capacity (FVC IN), predFVC IN, FVC IN%pred, forced expiratory volume in the sixth second (FEV6), peak expiratory flow (PEF), predPEF, PEF%pred were recorded.
Statistical methods
The statistical analysis was performed using SPSS 22.0 statistical software (IBM Corp., Armonk, NY, USA). A correlation analysis was performed on the data of the 139 participants, of whom 91 were in the normal FVC group and 48 were in the abnormal FVC group. A regression analysis was performed using the data of the normal participants for the ΔPLA and the data of all the participants for the FVC. The ΔPLA results and the measured and predicted FVC results were analyzed by non-parametric tests (the Kruskal-Wallis test) and pairwise comparisons of multiple independent samples. The ΔPLA and the mean FVC were determined. Correlation and regression analyses were performed on the measured data, and the correlation coefficients were tested for significance. A linear correlation was established if the P value was <0.05. Using a stepwise regression model, the explained variables [i.e., height, weight, age, and gender (male =1, female =0)] were indicators that were related to the PLA. The value in parentheses below the estimated regression equation was the t-statistic for the significance test of the regression coefficients, and the F-statistic was used to test whether the equation as a whole was significant. The results were considered statistically significant when the one-sided P value was <0.05 in the F-test and Kruskal-Wallis test, and the two-sided P value was <0.05 in the correlation and regression analyses. A regression analysis was performed to evaluate the correlations using the following criteria: strong (r>0.8), moderate (r=0.5–0.8), and poor (r<0.5).
Results
Statistical characteristics of variables in various sample conditions
In total, 139 participants were included in this study. The patients had an average age of 52.8±13.3 years, and 66 were male and 73 were female. A detailed description of the participant selection process can be found in Figure 2, in which FVC%pred was ≥80% for the normal pulmonary function group and <80% for the abnormal pulmonary function group, with 91 and 48 patients in each group, respectively. According to the normality test: full sample (n=139), normal FVC group (n=91), abnormal FVC group (n=48). For predFEV1, FEV1, FEV1%pred, predVC MAX, VC MAX, VC MAX%pred, predFVC IN, FVC IN, FVC IN%pred, FEV6, predPEF, PEF, PEF%pred, a P value >0.05 was considered not statistically significant (Table 1).
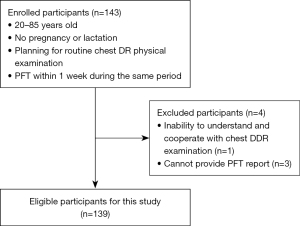
Table 1
Variables | All participants (n=139) | Participants with normal FVC (n=91) | Participants with abnormal FVC (n=48) |
---|---|---|---|
Age (years) | 52.8±13.3 [18.0–84.0] | 50.0 (5.0) [18.0–84.0] | 63.0 (13.3) [31.0–79.0] |
Female | 73 (52.5) | 59 (64.8) | 14 (29.2) |
Male | 66 (47.5) | 32 (35.2) | 34 (70.8) |
Height (cm) | 160.3±8.4 [143.5–178.0] | 158.5±8.3 [143.5–178.0] | 163.9±7.7 [148.0–178.0] |
Weight (kg) | 58.7 (15.0) [34.0–119.0] | 58.7 (14.4) [34.0–119.0] | 57.4±12.4 [35.0–89.9] |
ΔPLA (mm2) | 12,662.9±4,518.5 [4,525.0–25,310.0] |
14,498.5±3,914.4 [5,141.0–25,310.0] |
91,82.8±3,422.4 [4,525.0–18,5470.0] |
FVC (L) | 2.8±0.9 [1.0–5.1] | 3.2±0.8 [1.5–5.1] | 2.1±0.7 [1.0–3.8] |
predFVC (L) | 3.2±0.8 [1.7–5.0] | 3.1±0.8 [1.7–5.0] | 3.4±0.7 [1.9–5.0] |
FVC%pred | 0.9±0.2 [0.3–1.4] | 1.1±0.1 [0.8–1.4] | 0.6 (0.2) [0.3–0.8] |
FEV1%FVC | 0.8 (0.3) [0.2–1.8] | 0.8 (0.1) [0.3–1.0] | 0.6 (0.5) [0.2–1.8] |
predFEV1 | 2.6 (0.8) [1.4–4.2] | 2.4 (0.8) [1.4–4.2] | 2.8 (0.7) [1.6–4.2] |
FEV1 | 2.0±0.9 [0.3–4.2] | 2.4±0.8 [0.7–4.2] | 1.3±0.7 [0.3–2.7] |
FEV1%pred | 85.7 (45.8) [10.0–126.6] | 96.8 (22.5) [31.0–126.6] | 46.8±22.4 [10.0–108.4] |
predVC MAX | 3.1 (1.1) [2.1–5.3] | 2.9 (1.0) [2.1–5.1] | 3.5±0.7 [2.2–5.3] |
VC MAX | 2.9±0.9 [1.0–5.1] | 3.2±0.8 [1.6–5.1] | 2.2±0.7 [1.0–3.8] |
VC MAX%pred | 94.0 (36.3) [32.5–138.3] | 103.6±14.9 [57.7–138.3] | 62.0±13.8 [32.5–101.8] |
predFVC IN | 2.9 (1.0) [2.0–5.3] | 2.8 (0.9) [2.1–5.2] | 3.4±0.9 [2.0–5.3] |
FVC IN | 2.7±0.9 [0.8–5.0] | 3.0±0.8 [1.4–5.0] | 1.9±0.8 [0.8–3.8] |
FVC IN%pred | 88.2±23.1 [32.5–133.7] | 97.7±15.6 [49.8–133.7] | 57.7±15.8 [32.5–96.9] |
FEV6 | 2.8±1.0 [0.8–5.1] | 3.1±0.8 [1.6–5.1] | 1.9±0.7 [0.8–3.6] |
predPEF | 6.5 (1.8) [4.8–9.7] | 6.1 (1.6) [4.8–9.7] | 7.2±1.1 [5.0–9.7] |
PEF | 5.7±2.3 [0.8–10.9] | 6.4±2.0 [1.6–10.9] | 3.9±2.1 [0.8–7.5] |
PEF%pred | 91.5 (33.1) [11.0–135.5] | 98.6 (21.9) [24.7–135.5] | 55.1±29.3 [11.0–111.5] |
Data are presented as the mean ± SD [range], median (interquartile) [range], or number (percentage). FVC, forced vital capacity; ΔPLA, change in the projected lung area; FEV1, forced expiratory volume in one second; VC MAX, maximum vital capacity; FVC IN, forced inspiratory vital capacity; FEV6, forced expiratory volume in the sixth second; PEF, peak expiratory flow; %pred, % predicted; SD, standard deviation.
Correlation and regression analysis between the ΔPLA and the measured FVC under forced breathing
According to the results of the one-sample t-test, the ΔPLA and FVC were normally distributed. Thus, the Pearson simple correlation coefficient was used to measure the linear correlation between them. The Pearson simple correlation coefficients were 0.81, 0.74, and 0.68 with 95% confidence intervals (CIs) of 0.75–0.86, 0.64–0.81, and 0.53–0.81 in the full sample, normal FVC group, and abnormal FVC group, respectively (all P<0.001). The correlation coefficient was >0.8 in the full sample, which indicated a strong correlation (Table 2).
Table 2
Sample | r | 95% CI | P value |
---|---|---|---|
All participants | 0.81 | 0.75, 0.86 | <0.001 |
Participants with normal FVC | 0.74 | 0.64, 0.81 | <0.001 |
Participants with abnormal FVC | 0.68 | 0.53, 0.81 | <0.001 |
ΔPLA, change in the projected lung area; FVC, forced vital capacity; CI, confidence interval.
For further significance testing, the null hypothesis was that the population correlation coefficient was 0. The P values of the t-tests were <0.001, and the null hypothesis was rejected, which indicated that there was a statistically significant linear correlation between the ΔPLA and FVC in all three sample conditions. The scatter diagrams of the ΔPLA and FVC in the full sample, normal FVC group, and abnormal FVC group showed that the scatter points were all distributed in the oblique upward oval area, indicating a positive covariant relationship between the two variables in these sample conditions (Figure 3).
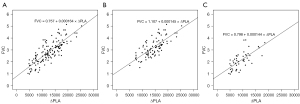
With the measured FVC as the explained variable, and the ΔPLA, height, age, gender, and weight as the explaining variables, the variables affecting the measured FVC were screened using the stepwise regression method to establish the DDR-FVC estimation model. Ultimately, four explaining variables were included in the stepwise regression; that is, the ΔPLA, height, age, and gender, which had respective regression coefficients of 1.35×10−4, 0.017, −0.014, and 0.249 with 95% CIs of 1.14×10−4 to 1.56×10−4, 0.003 to 0.031, –0.022 to –0.006, and –0.019 to 0.498 (P<0.001, P=0.015, P<0.001, and P=0.041), respectively (Table 3). Therefore, the results showed that the ΔPLA had a positive effect on the measured FVC and could be used to estimate the FVC measurement results. In addition, for the measured FVC, the effect of height was positive, the effect of age was negative, and the mean FVC was greater for males. The adjusted R2 (adj. R2) of the stepwise regression coefficient was 0.731, which indicated that 73.1% of the FVC information could be explained by the four variables. The F-statistic was used to test whether the equation as a whole was significant. The calculated results showed a F-value of 94.615 with a P value <0.001; thus, it can be inferred that this linear regression relationship also exists in the population. The specific regression equation was expressed as follows:
Table 3
Explaining variable | Coefficient | 95% CI | t | P value |
---|---|---|---|---|
C | −0.997 | –3.413, 1.212 | −0.869 | 0.386 |
Lung area change | 1.35×10−4 | 1.14×10−4, 1.56×10−4 | 12.935 | <0.001 |
Height | 0.017 | 0.003, 0.031 | 2.463 | 0.015 |
Age | −0.014 | –0.022, –0.006 | −3.704 | <0.001 |
Gender | 0.249 | –0.019, 0.498 | 2.059 | 0.041 |
DDR, dynamic digital radiography; FVC, forced vital capacity; CI, confidence interval; C, constant.
The comparison of the ΔPLA under forced breathing between the normal FVC group and the abnormal FVC group
An independent samples t-test was used to examine whether there was a significant difference in the ΔPLA between the normal and abnormal FVC groups. First, using the Levene F-test, no significant difference in variance was found between the normal and abnormal groups in the population. Accordingly, with a t-statistic of 7.940 and a P value <0.001, the t-test indicated that there was no significant difference in the ΔPLA between the two groups. Therefore, the null hypothesis was rejected and the ΔPLA between the normal and abnormal FVC groups was considered statistically significantly different.
Prediction of the ΔPLA under forced breathing in the normal FVC group
With the ΔPLA as the explained variable, and age, gender, height, and weight as the explaining variables, the variables affecting the ΔPLA were screened using the stepwise regression method. Ultimately, three explaining variables were included in the stepwise regression; that is, height, age, and weight (weight was retained because the significance level was 0.055, which was close to 0.05, and significant at a 0.10 level), which had respective regression coefficients of 173.185, −84.933, and 62.971 with 95% CIs of 73.161 to 277.341, –136.408 to –28.729, and 6.063 to 117.762 (P=0.001, P=0.002, and P=0.055), respectively (Table 4). The greater the height and weight, the greater the ΔPLA on average; however, the opposite was true for age. The adj. R2 of the stepwise regression was 0.393, which indicated that 39.3% of the FVC information could be explained by the three variables. The F-statistic was used to test whether the equation as a whole was significant. The calculated results showed an F-value of 20.453 with a P value <0.001; thus, it can be inferred that this linear regression relationship also exists in the population. The regression equation was expressed as follows:
Table 4
Explaining variable | Coefficient | 95% CI | t | P value |
---|---|---|---|---|
C | −12,504.287 | –27,736.282, 1,898.602 | −1.725 | 0.088 |
Height | 173.185 | 73.161, 277.341 | 3.373 | 0.001 |
Age | −84.933 | –136.408, –28.729 | −3.212 | 0.002 |
Weight | 62.971 | 6.063, 117.762 | 1.948 | 0.055 |
ΔPLA, change in the projected lung area; CI, confidence interval; C, constant.
Characteristics of indicators in the FVC normal group and FVC abnormal group
Based on the sample data, multiple index values with respect to FVC were calculated and compared (using the independent sample t-test) in the normal and abnormal groups (Table 5). The mean values of FVC were 3.2 and 2.1 (95% CI of mean difference: 0.827–1.360, P<0.001), the FVC%pred values were 1.051 and 0.622 (95% CI of mean difference: 0.380–0.477, P<0.001), the ΔPLA values were 14,498.550 and 9,182.750 (95% CI of mean difference: 3,991.966–6,639.633, P<0.001), and the ΔPLA%pred values were 0.999 and 0.628 (95% CI of mean difference: 0.300–0.443, P<0.001), in the normal group and the abnormal group, respectively. The results indicated that all these indicators were significantly different when the two groups were divided according to the following standard: an FVC%pred of 80%.
Table 5
Variables | Participants with normal FVC (n=91), mean value | Participants with abnormal FVC (n=48), mean value | 95% CI of mean difference | t | P |
---|---|---|---|---|---|
FVC | 3.210 | 2.117 | 0.827, 1.360 | 8.11 | <0.001 |
FVC%pred | 1.051 | 0.622 | 0.380, 0.477 | 17.35 | <0.001 |
ΔPLA | 14,498.550 | 9,182.750 | 3,991.966, 6,639.633 | 7.94 | <0.001 |
ΔPLA%pred | 0.999 | 0.628 | 0.300, 0.443 | 10.26 | <0.001 |
In this article, the predictive value of the ΔPLA was calculated using the original regression model. FVC, forced vital capacity; CI, confidence interval; ΔPLA, change in the projected lung area; %pred, % predicted.
Discussion
Exploration of the correlation between the ΔPLA measured by biphasic DDR and the FVC, and the establishment of a DDR-FVC estimation model and a predictive value model of the ΔPLA
The diaphragm is the main muscle that maintains human respiration. When the diaphragm actively contracts and moves downward, it creates negative pressure in the thoracic cavity, which promotes the entry of air into the chest cavity, and the PLA becomes larger. When the diaphragm relaxes, the air is exhaled with the elastic retraction of the lung, and the PLA becomes smaller. This is the well-known physiological process of breathing. Many relevant imaging studies have evaluated the morphology or function of the diaphragm (16-18). The common chest X-ray is the primary screening method for evaluating morphological changes in the diaphragm (19). However, few studies have examined the correlation between the ΔPLA and FVC. Therefore, we conducted a preliminary study on the correlation between the ΔPLA and FVC, and the results revealed a statistically significant linear correlation between the ΔPLA measured by chest DDR and FVC.
A previous study reported that lung air content is strongly correlated with the FVC and other PFT parameters in patients with pulmonary dysfunction, which helped to establish a multi-linear model of PFT parameters with high predictive ability (11). Based on the ΔPLA, we established a DDR-FVC estimation model to estimate a patient’s FVC through the PLA change, and we also established a predictive value model for the ΔPLA. The DDR examination is characterized by its user-friendly operation, low radiation dose, and capability for vertical photography. Compared to CT, magnetic resonance imaging (MRI), and other functional examinations, DDR depicts the normal pathological status of patients more effectively.
The PFT has a high level of difficulty due to factors such as poor tester cooperation, which often results in inconsistencies between the examination results and the patient’s condition. By using DDR and collecting maximum and minimum areas through deep inspirations and exhalations, the area difference can be calculated. Patients can be diagnosed as ILD if the percentage of vital capacity (%VC) is <80% (10). The ΔPLA is strongly correlated with the FVC, which enables ΔPLA%pred. Additionally, the DDR-FVC estimation model can be employed to estimate a patient’s FVC while calculating the ratio between the estimated DDR-FVC value and preFVC; if this ratio is <80%, an abnormality in FVC may also be considered. These two models serve as preliminary evaluations for assessing a patient’s FVC while providing an imaging basis for clinical decision making.
Correlation between the ΔPLA and FVC under forced breathing
The PLA, as measured by biphasic chest DR, changes with respiratory movements. The PLA increases as the lung air content increases during inspiration, and the PLA decreases as the lung air content decreases during expiration. In this study, patients with abnormal vital capacity primarily presented with COPD and restrictive lung diseases, such as interstitial disease and diaphragmatic paralysis. These patients exhibited distinct ΔPLA values due to varying pathogeneses. For instance, COPD patients displayed limited airway stenosis and reduced alveolar retraction function, resulting in increased alveolar compensation and residual volume. Consequently, the minimum area increased while the maximum area remained relatively unchanged, leading to a smaller ΔPLA value. Conversely, patients with restrictive lung diseases experienced restricted respiratory function. Interstitial disease was characterized by low residual lung volume accompanied by significant reductions in both the minimum and maximum areas. Diaphragmatic paralysis, a neuromuscular disorder associated with high residual lung volume, caused an opposite movement of the diaphragm during expiration compared to normal individuals, and as a result, the minimum area expanded. During inspiration when the diaphragm ascended, the maximum area decreased significantly leading to a smaller ΔPLA value. Therefore, a decreasing ΔPLA and a ΔPLA%pred ratio <80% may indicate diseases related to a decreased FVC.
The FVC, which is the gold standard of the PFT, reflects the amount (volume) of inspired air into the human body, which corresponds to the change in the volume of human lung tissue (thorax). The quantitative analysis of pulmonary function in patients with COPD by CT and its post-processing techniques has great advantages. It provides an early quantitative diagnosis, and condition and prognosis evaluations of COPD by indirectly analyzing pathological and physiological changes of lung tissue based on factors such as the lung volume, lung density, or pixel index (20). DDR uses a lower dose of radiation than conventional X-ray fluoroscopy and CT, and it provides continuous chest radiographs during respiration (21). The utility of MRI is limited due to its high costs, low usability, and low throughput. Conversely, despite being two-dimensional (2D) imaging, DCR can be performed in seconds with a radiation dose comparable to conventional posteroanterior and lateral chest radiography (22). These images reflect neodynamics parameters that can effectively aid in evaluating pulmonary function in patients with lung diseases (23), providing a simple and convenient new method for assessing pulmonary function with 2D imaging examinations.
With following advances in DDR equipment in recent years, some researchers have conducted studies on pulmonary ventilation function using DDR. It has been proposed that the rate of change in the lung area from maximum inspiration to maximum expiration (the Rs ratio) is correlated with the RV/TLC ratio and the percentage of the predicted FEV, and the rate of change in the lung area during the respiratory cycle reflects the severity of the airflow limitation (3). It has also been suggested that the lung area at the time of the maximum inspiration is correlated with the VC (functional residual capacity, RV, and TLC) (9).
In this article, we focused on the correlation between the ΔPLA and lung capacity. However, only PLA (rather than lung tissue volume) could be measured in the DR 2D images. In the lung tissue volume change during the respiratory cycle, the main influencing factors were the up-and-down motion of the diaphragm, and changes in the transverse diameter and anterior-posterior diameter of the thorax. Diaphragmatic motion and changes in the transverse thoracic diameter directly affected the ΔPLA, which can be easily measured in routine posterior-anterior chest images. However, changes in the anterior-posterior thoracic diameter have relatively low effects on the lung volume, and the main factor contributing to lung tissue volume changes is diaphragmatic motion. This study showed that the PLA had a significant and strong correlation with the lung volume. Therefore, changes in the PLA could indirectly reflect changes in the lung volume. Additionally, changes in lung volume are closely related to the VC. The results of this study on the correlation between the ΔPLA and FVC under forced breathing showed that the correlation coefficient between the ΔPLA and the measured FVC in the PFT was 0.81 (P<0.001).
Estimation of FVC by the ΔPLA under forced breathing and the establishment of the DDR-FVC estimation model
Research in other populations has shown that the PLA measured by chest DDR can be used to predict FVC (11). In this study, the data of 139 participants were statistically analyzed to examine the influence of age, gender, height, weight, the PLA, and the ΔPLA on the FVC. We further compared the FVC, FVC%pred change in peak left atrial pressure ΔPLA, ΔPLA%pred, and the differences between the two groups (Table 5). Our analysis revealed a significant disparity between the two groups, confirming that participants with normal FVC and abnormal FVC exhibited distinct correlations. However, when applying the criterion of FVC%pred ≥80% to divide the participants into two groups, we found that the DDR-FVC estimation model remained statistically significant for evaluating FVC. The estimated result reflects the actual and examiner results and differs from the normal predicted value of the FVC. Based on the statistical analysis, the estimation model was expressed as DDR-FVC = –0.997 + 1.35×10−4 × ΔPLA + 0.017 × height −0.014 × age + 0.249 × gender (male: 1, female: 0; adj. R2=0.731; F=94.615; P<0.001). This indicates that regardless of whether a patient’s FVC is normal or abnormal, it is closely aligned with the estimated value derived from our DDR-FVC estimation model, thus demonstrating its ability to accurately reflect the examiner’s actual measurement values.
Further, our findings suggest that FVC can be effectively estimated using the ΔPLA in conjunction with age, sex, and height to construct a reliable DDR-FVC estimation model. By comparing the actual measured FVC values with those predicted by our model, we were able to identify potential abnormalities in FVC levels. The Global Initiative for Chronic Obstructive Lung Disease (GOLD) 2020 states that COPD is characterized by respiratory symptoms and airflow limitation, and persistent airflow limitation can be confirmed with FEV1/FVC <0.7 after the use of bronchodilators (24). A larger PLA during expiration may reflect a higher degree of lung inflation at the end of expiration. Therefore, an increased PLA during expiration may suggest greater RV and obstruction due to air trapping, which may be observed in functional CT (25). Therefore, FVC estimation by the PLA has practical clinical significance for patients who cannot undergo PFTs. In patients with pulmonary fibrosis, the measurement of the PLA by chest DDR can be used as an auxiliary indicator to assess the deterioration of pulmonary function (26). DDR is a potential tool for assessing respiratory disorders in patients with respiratory diseases (27).
Establishment of a predictive value model for the ΔPLA under forced breathing
In routine spirometry, measurements are assessed by comparison with reference values based on age, height, gender, and race (28,29). In this study, age, gender, height, weight, the PLA, and the ΔPLA were statistically analyzed in the samples. The statistical results showed that the predictive value of the ΔPLA could be calculated as follows: ΔPLA = −12,504.287 + 173.185 × height + 62.971 × weight − 84.933 × age (adj. R2=0.393, F=20.453, P<0.001). Thus, the ΔPLA could be predicted by age, height, and weight, and the ΔPLA was significantly correlated with the FVC. In other words, a prediction model for the ΔPLA established based on age, height, and weight, and a DDR-FVC estimation model for estimating FVC was established based on the ΔPLA by age, gender, and height. These models provide a theoretical basis for evaluating pulmonary ventilation function in related diseases by biphasic chest DR. However, we also note that the correlation between the ΔPLA and FVC in participants with abnormal FVC was 0.68, which was slightly lower than that in participants with normal FVC (0.74), which may be related to different types of diseases, diverse mechanisms of change, or different degrees of cooperation of patients with diseases.
We performed a characteristics analysis of the normal and abnormal FVC groups (Table 5), and found significant differences. Based on the FVC%pred of 80% criterion, the participants were divided into two groups. There was a statistically significant clear difference in the performance of each index between the two groups that could be used to evaluate whether the FVC was abnormal. The specific disease classification needs to be further explored. The directions of our continued research include increasing the evaluation of the resting breathing state and seeking to identify disease types based on the changes in the maximum and minimum lung field area.
Limitations
The qualitative results of this study are clear; however, the quantitative data needs to be further improved due to a number of factors. First, the sample size was small, and there were biases in the prediction model; thus, a study with a large sample size needs to be conducted to improve the quantitative analysis. Second, it was a single-center study with limited coverage. Third, the radiography process, patient cooperation, and test specifications need to be further improved. Fourth, chest DDR is a new technique, and the captured images only contain anterior-posterior information, and lateral information is lacking. This needs to be further improved to provide more objective data for clinical research. Fifth, this study only focused on the correlation between the ΔPLA and FVC under forced breathing during deep breathing, and did not include the eupnea state; thus, further correlation studies need to be conducted under this state.
Conclusions
In summary, there was a linear correlation between the ΔPLA measured by biphasic chest DR and the FVC. A model for estimating the FVC was established based on the ΔPLA, which allows the FVC to be assessed by the ΔPLA measured by biphasic chest DDR. A predictive value model for the ΔPLA was also established to provide reference values of the PLA changes for assessment and comparison.
Acknowledgments
The authors would like to appreciate The First Affiliated Hospital of Guangzhou Medical University providing examinations for the participants. The images produced by this study would not have been possible without the expertise of the clinicians and radiology technicians at The First Affiliated Hospital of Guangzhou Medical University, who have shown outstanding dedication in their work.
Funding: This study was funded by
Footnote
Reporting Checklist: The authors have completed the STROBE reporting checklist. Available at https://qims.amegroups.com/article/view/10.21037/qims-23-1186/rc
Conflicts of Interest: All authors have completed the ICMJE uniform disclosure form (available at https://qims.amegroups.com/article/view/10.21037/qims-23-1186/coif). The authors have no conflicts of interest to declare.
Ethical Statement: The authors are accountable for all aspects of the work in ensuring that questions related to the accuracy or integrity of any part of the work are appropriately investigated and resolved. The study was conducted in accordance with the Declaration of Helsinki (as revised in 2013). The study was approved by the Research Ethics Review Committee of The First Affiliated Hospital of Guangzhou Medical University (No. 106 of 2022). The patients/participants provided their written informed consent to participate in this study.
Open Access Statement: This is an Open Access article distributed in accordance with the Creative Commons Attribution-NonCommercial-NoDerivs 4.0 International License (CC BY-NC-ND 4.0), which permits the non-commercial replication and distribution of the article with the strict proviso that no changes or edits are made and the original work is properly cited (including links to both the formal publication through the relevant DOI and the license). See: https://creativecommons.org/licenses/by-nc-nd/4.0/.
References
- Tao LI, Liang WH, Respiratory DO. Value of lung function to evaluate chronic obstructive pulmonary disease. Clin Med 2018;23:274-7.
- Matsuoka S, Kurihara Y, Yagihashi K, Nakajima Y. Quantitative assessment of peripheral airway obstruction on paired expiratory/inspiratory thin-section computed tomography in chronic obstructive pulmonary disease with emphysema. J Comput Assist Tomogr 2007;31:384-9. [Crossref] [PubMed]
- Zou LG, Sun QR, Qi YY, Dai SH, Chen K. Feasibility of low dose scanning of multi-slice CT in quantitative analysis of lung volume and density. Chinese Journal of Medical Imaging Technology 2007;23:1491-4.
- Zhang WH, Mou WB, Qi B, Zhang YQ, Wang Y, Ma Y, Wang FY, Wang JL, Cai BQ, Lin YG, Yan HZ, Jin ZY, Liu YQ. Parameters of CT pulmonary function imaging in emphysema and obstructive ventilatory defect. Chinese Journal of Medical Imaging Technology 2005;21:906-9.
- Chen J, Zhong Z, Wang W, Yu G, Zhang T, Wang Z. Quantitative evaluation of diaphragmatic motion during forced breathing in chronic obstructive pulmonary disease patients using dynamic chest radiography. Front Integr Neurosci 2022;16:842404. [Crossref] [PubMed]
- Hida T, Yamada Y, Ueyama M, Araki T, Nishino M, Kurosaki A, Jinzaki M, Honda H, Hatabu H, Kudoh S. Decreased and slower diaphragmatic motion during forced breathing in severe COPD patients: Time-resolved quantitative analysis using dynamic chest radiography with a flat panel detector system. Eur J Radiol 2019;112:28-36. [Crossref] [PubMed]
- Yu GG, Wang W, Chen JH, Cui RX, Zhong ZH, Xu B, Wang HY. Application of dynamic chest digital radiography in evaluation of diaphragm motor function in patients with chronic obstructive pulmonary disease. International Journal of Respiration 2021;41:1500-5.
- Shen HY, Zhu XX, Chen Q, Zhao LL, Zhong LJ. Comparison of different examination methods in evaluating diaphragmatic motion in patients with COPD. Journal of China Clinic Medical Imaging 2019;30:698-702.
- Ohkura N, Kasahara K, Watanabe S, Hara J, Abo M, Sone T, Kimura H, Takata M, Tamura M, Matsumoto I, Nakade Y, Sanada S, Tanaka R. Dynamic-Ventilatory Digital Radiography in Air Flow Limitation: A Change in Lung Area Reflects Air Trapping. Respiration 2020;99:382-8. [Crossref] [PubMed]
- Hino T, Hata A, Hida T, Yamada Y, Ueyama M, Araki T, Kamitani T, Nishino M, Kurosaki A, Jinzaki M, Ishigami K, Honda H, Hatabu H, Kudoh S. Projected lung areas using dynamic X-ray (DXR). Eur J Radiol Open 2020;7:100263. [Crossref] [PubMed]
- Ueyama M, Hashimoto S, Takeda A, Maruguchi N, Yamamoto R, Matsumura K, Nakamura S, Terada S, Inao T, Kaji Y, Yasuda T, Hajiro T, Tanaka E, Taguchi Y, Noma S. Prediction of forced vital capacity with dynamic chest radiography in interstitial lung disease. Eur J Radiol 2021;142:109866. [Crossref] [PubMed]
- FitzMaurice TS, McCann C, Nazareth D, Shaw M, McNamara PS, Walshaw MJ. Measuring the effect of elexacaftor/tezacaftor/ivacaftor combination therapy on the respiratory pump in people with CF using dynamic chest radiography. J Cyst Fibros 2022;21:1036-41. [Crossref] [PubMed]
- Pulmonary Function Professional Group, Respiratory Diseases Branch of Chinese Medical Association. Guidelines for pulmonary function examination (part 2)-spirometer examination. Chin J Tuberc Respir 2014;37:481-6.
- Zhu L, Chen RC. Chinese experts’ consensus on the standardization of adult lung function diagnosis. Journal of Clinical Pulmonary Medicine 2022;27:973-981.
- Graham BL, Steenbruggen I, Miller MR, Barjaktarevic IZ, Cooper BG, Hall GL, Hallstrand TS, Kaminsky DA, McCarthy K, McCormack MC, Oropez CE, Rosenfeld M, Stanojevic S, Swanney MP, Thompson BR. Standardization of Spirometry 2019 Update. An Official American Thoracic Society and European Respiratory Society Technical Statement. Am J Respir Crit Care Med 2019;200:e70-88. [Crossref] [PubMed]
- do Nascimento IB, Fleig R. Mobility impact and methods of diaphragm monitoring in patients with chronic obstructive pulmonary disease: a systematic review. Clinics (Sao Paulo) 2020;75:e1428. [Crossref] [PubMed]
- Zhou L, Li Q, Huang S. Progress of research on composite evaluation methods of diaphragmatic dysfunction. Zhonghua Jie He He Hu Xi Za Zhi 2014;37:115-8.
- Koo CW, Johnson TF, Gierada DS, White DB, Blackmon S, Matsumoto JM, Choe J, Allen MS, Levin DL, Kuzo RS. The breadth of the diaphragm: updates in embryogenesis and role of imaging. Br J Radiol 2018;91:20170600. [Crossref] [PubMed]
- Chetta A, Rehman AK, Moxham J, Carr DH, Polkey MI. Chest radiography cannot predict diaphragm function. Respir Med 2005;99:39-44. [Crossref] [PubMed]
- Chen H, Chen RC, Guan YB, Li W, Liu Q, Zeng QS. Correlation of pulmonary function indexes determined by low-dose MDCT with spirometric pulmonary function tests in patients with chronic obstructive pulmonary disease. AJR Am J Roentgenol 2014;202:711-8. [Crossref] [PubMed]
- Tanaka R, Sanada S, Kobayashi T, Suzuki M, Matsui T, Matsui O. Computerized methods for determining respiratory phase on dynamic chest radiographs obtained by a dynamic flat-panel detector (FPD) system. J Digit Imaging 2006;19:41-51. [Crossref] [PubMed]
- Bennett GF. International basic safety standards for protection against ionizing radiation and for the safety of radiation sources. International Atomic Energy Agency, Vienna, Safety Series No. 115, 1996, 353 pp. ISBN: 92-0-104295-7. J Hazard Mater 1997;54:134-5.
- Tanaka R, Sanada S, Okazaki N, Kobayashi T, Fujimura M, Yasui M, Matsui T, Nakayama K, Nanbu Y, Matsui O. Evaluation of pulmonary function using breathing chest radiography with a dynamic flat panel detector: primary results in pulmonary diseases. Invest Radiol 2006;41:735-45. [Crossref] [PubMed]
- Rabe KF, Hurd S, Anzueto A, Barnes PJ, Buist SA, Calverley P, Fukuchi Y, Jenkins C, Rodriguez-Roisin R, van Weel C, Zielinski JGlobal Initiative for Chronic Obstructive Lung Disease. Global strategy for the diagnosis, management, and prevention of chronic obstructive pulmonary disease: GOLD executive summary. Am J Respir Crit Care Med 2007;176:532-55. [Crossref] [PubMed]
- Lauwers E, Snoeckx A, Ides K, Van Hoorenbeeck K, Lanclus M, De Backer W, De Backer J, Verhulst S. Functional respiratory imaging in relation to classical outcome measures in cystic fibrosis: a cross-sectional study. BMC Pulm Med 2021;21:256. [Crossref] [PubMed]
- FitzMaurice TS, McCann C, Nazareth DS, McNamara PS, Walshaw MJ. Use of Dynamic Chest Radiography to Assess Treatment of Pulmonary Exacerbations in Cystic Fibrosis. Radiology 2022;303:675-81. [Crossref] [PubMed]
- Ohkura N, Tanaka R, Watanabe S, Hara J, Abo M, Nakade Y, Horii J, Matsuura Y, Inoue D, Takata M, Tamura M, Matsumoto I, Sanada S, Kasahara K. Chest Dynamic-Ventilatory Digital Radiography in Chronic Obstructive or Restrictive Lung Disease. Int J Chron Obstruct Pulmon Dis 2021;16:1393-9. [Crossref] [PubMed]
- Pellegrino R, Viegi G, Brusasco V, Crapo RO, Burgos F, Casaburi R, Coates A, van der Grinten CP, Gustafsson P, Hankinson J, Jensen R, Johnson DC, MacIntyre N, McKay R, Miller MR, Navajas D, Pedersen OF, Wanger J. Interpretative strategies for lung function tests. Eur Respir J 2005;26:948-68. [Crossref] [PubMed]
- Quanjer PH, Stanojevic S, Cole TJ, Baur X, Hall GL, Culver BH, Enright PL, Hankinson JL, Ip MS, Zheng J, Stocks JERS Global Lung Function Initiative. Multi-ethnic reference values for spirometry for the 3-95-yr age range: the global lung function 2012 equations. Eur Respir J 2012;40:1324-43. [Crossref] [PubMed]