Identification of a polycomb group-related gene signature for predicting prognosis and immunotherapy efficacy in lung adenocarcinoma
Highlight box
Key findings
• Report here about key findings of the study.
• The PcGScore is a good predictor of prognosis and immunotherapy response in LUAD.
What is known and what is new?
• Report here about what is known.
• 28 PcG-related genes had been reported in the previous literature.
• Report here about what does this manuscript adds.
• The model was constructed based on the 28 PcG-related genes for predicting prognosis and treatment response in LUAD for the first time.
What is the implication, and what should change now?
• Report here about implications and actions needed.
• This model had a good prediction of prognosis and treatment response for the LUAD, thus providing an ideal and stable predictor for the clinical treatment of the LUAD patients. However, the PcG-related genes need to be further validated by in vivo and in vitro studies.
Introduction
Lung cancer (LC) kills an estimated 1.6 million people per year, making it the leading cause of cancer-related deaths worldwide (1). LC can be divided into small cell lung carcinoma (SCLC) and non-small cell lung carcinoma (NSCLC), with NSCLC causing approximately 85% of the LC cases (2,3). Lung adenocarcinoma (LUAD) is the most common NSCLC, followed by lung squamous cell carcinoma (LUSC) (4). Since early symptoms of LC are frequently being missed, majority of the LC patients are in the advanced stages at the time of diagnosis (5). In clinical practice, patients with early-stage LC do not receive treatment, which makes it difficult to find prognostic markers through early screening. Furthermore, LC patients have a poor prognosis, with the 5-year relative survival rate of approximately 18% (6). Recently, immune checkpoint blockade (ICB) treatment has gained popularity for LC. Currently, immune checkpoint inhibitors (ICIs), such as programmed cell death-1/programmed death-ligand 1 (PD-1/PD-L1), are recommended for NSCLC treatment and have demonstrated significant benefits and improved the prognosis of advanced NCSLC patients (7). However, despite impressive advances, only a small percentage of cancer patients have benefited from immunotherapy, owing to immunotherapy resistance, limited response rates, and unpredictable clinical outcomes (8). Moreover, tumor heterogeneity impedes efficacy and immunotherapies are prohibitive expense and not being given as first-line treatments. Therefore, a novel biomarker is in urgent need to predict the prognosis and response of treatment for LUAD.
Polycomb group (PcG) proteins are transcriptional repressor that silences genes via histone post-translational modifications. The PcG proteins are involved in cell proliferation, differentiation, DNA damage and repair, and the progression of fatal diseases, such as cancer (9). The polycomb repressive complexes 1 and 2 (PRC1 and PRC2) (10) are the two major PRCs involved in tumorigenesis. Ring finger protein 1 (RING1) is a fundamental component of the PRC1, and its expression varies in different cancers, resulting in a wide range of outcomes (11). RING1 overexpression in liver cancer and NSCLC promotes tumor growth, while its downexpression in breast cancer causes adverse outcomes (12-14). In contrast, enhancer of zeste homolog 2 (EZH2) is an integral component of the PRC2, and its inhibition significantly slows tumor growth. For instance, EZH2 knock down in LC cell lines results in a significant reduction in tumor migration and invasive ability (15). Similarly, inhibition of EZH2 expression in prostate cancer significantly slows tumor growth and improves prognosis (16). In addition, EZH2 is involved in cancer immunity (17,18), metabolism (19,20), and resistance to treatments (21). Therefore, EZH2 has been studied extensively and several types of EZH2 inhibitors have been developed for cancer treatment (15,22).
In this study, PcG-related genes were integrated and two PcG-related patterns in LUAD were identified. The differentially expressed genes (DEGs) in the two groups was identified and the least absolute shrinkage and selection operator (LASSO) Cox regression analysis was used to develop a new scoring system called the PcGScore. Thereafter, the relationship between the PcGScore and prognosis, tumor mutational burden (TMB), immune cell infiltration, and treatment sensitivity was explored in the training and validation datasets. We present this article in accordance with the TRIPOD reporting checklist (available at https://jtd.amegroups.com/article/view/10.21037/jtd-22-1324/rc).
Methods
Preparation of the LC datasets
The study was conducted in accordance with the Declaration of Helsinki (as revised in 2013). The Cancer Genome Atlas (TCGA) database was used to collect transcriptome expression data of 598 samples (59 normal and 539 LUAD samples) and match clinical information of 522 samples. The transcripts per kilobase million (TPM) method was used to normalize the fragments per kilobase of transcript per million fragments sequenced (FPKM) values, which were then log2 transformed. The GSE13213, GSE30219, and GSE31210 datasets in the Gene Expression Omnibus (GEO) database were used to obtain the microarray expression profiles and clinical information of 117, 85, and 226 LUAD samples, respectively (https://www.ncbi.nlm.nih.gov/geo/). The “limma” and “sva” packages (23) were used for correction to reduce the possibility of batch effects due to non-biotechnical bias between different datasets.
Copy number variation (CNV) frequency of the PcG-related genes in LUAD samples
The UCSC Xena database (http://xena.ucsc.edu/) was used to obtain the CNV information for the LUAD samples. The variation frequencies of 28 PcG-related genes were then computed, and the results were presented as lollipop plots. The Rcircos package (24) was used to map the locations of these PcG-related genes on human chromosomes.
Consensus clustering analysis of the PcG-related genes in LUAD samples
Twenty-eight PcG-related genes were obtained for consensus clustering analysis, based on the previously published literature. The “ConsensusClusterPlus” package (25), with an hierarchical agglomerative consensus, was used to stratify the LUAD samples into two discrete subgroups. The stability evidence was used in this analysis to determine the cluster counts and membership, and this procedure was repeated 1,000 times to ensure clustering stability. The two PcG patterns were compared for overall survival (OS) using Kaplan-Meier (KM) curves. Lastly, the accuracy of this classification was verified using principal component analysis (PCA).
Gene set variation analysis (GSVA) of the two PcG patterns
Gene enrichment analysis was conducted using the “GSVA” R package (26) to explore the variations in the biological processes (BP) of the PcG patterns. The Kyoto Encyclopedia of Genes and Genomes (KEGG) gene set was downloaded from the Molecular Signatures Database (MSigDB) database (http://software.broadinstitute.org/gsea/msigdb/) to perform GSVA.
Evaluation of the immune cell infiltration of the two PcG patterns
The “GSVA” R package was used to perform single-sample gene-set enrichment analysis (ssGSEA), based on the expression levels of 23 immune-related genes, to assess and characterize immune cell infiltration in each sample of the PcG patterns. By estimating the enrichment fraction, ssGSEA can determine the relative abundance of each immune cell population. Lastly, the variations in immune cell infiltration levels between the two PcG patterns were investigated.
Identification and enrichment analysis of the DEGs in the two PcG patterns
Two PcG patterns were identified by using the consensus clustering algorithm, after which the “limma” R package was used to identify the DEGs in the two PcG patterns (27). DEGs with P value <0.01 and |log2FC| >1.5 were deemed significant and used in further analysis. Lastly, the DEGs were subjected to Gene Ontology (GO) and KEGG enrichment analysis using the “clusterProfiler” R package (28).
Prognostic model based on the PcG-related genes
Univariate Cox regression was used to identify the PcG-related genes in the training dataset that were correlated with OS in LUAD patients. LUAD prognostic features in the training dataset were examined by the “glmnet” R package (29) for the OS associated PcG-related genes with the LASSO analysis. The PcGScore was determined as follows:
where i is the number of variables in the model, Coef denotes the regression coefficient, and Exp denotes the mRNA expression levels of the variables in the LUAD samples. The LUAD patients in the training and validation datasets were divided into high-risk and low-risk groups, based on the median risk score. Furthermore, KM curves were generated using the “survival” and “survminer” R packages, to examine the survival differences between the high- and low-risk groups to further evaluate the viability of the model. The “timeROC” and “survivor” R packages were used to plot time-dependent receiver operating characteristic (ROC) curves to evaluate the predictive performance of the risk scores on 1-, 3-, and 5-year survival rates of the LUAD patients. Thereafter, the survival statuses of the two groups were plotted. The “pheatmap” package was used to create a visual representation of the mRNA expression patterns of each variable in the prognostic model. Lastly, the “Rtsne” and “ggplot2” packages were used to conduct PCA and t-distributed stochastic neighborhood embedding (t-SNE) analysis of the LUAD patients to further validate the risk score prediction model.
Comprehensive assessment of the PcGScore and the clinical parameters of the LUAD patients
A boxplot was created using the “ggpubr” package to compare the differences between the PcGScore and other clinically relevant parameters of LUAD. Additionally, KM curves were plotted for various clinical parameters, including age, gender, sex, and the stages of tumor progression, to determine whether the PcGScore is applicable in different clinical situations.
Prediction of the PcGScore and development of a prognostic nomogram
Univariate and multivariate Cox regression analyses of the clinical information (age, gender, and stage) were conducted for the training and testing datasets to determine whether the PcGScore is an independent prognostic predictor of LUAD. Subsequently, a nomogram was created using the “rms” package for both the training and testing datasets to predict the 1-, 3-, and 5-year survival rates of the LUAD patients. Calibration curves were used to assess the prediction ability of the nomogram.
Assessment of the TMB in the two risk groups
TMB was calculated using the somatic alteration data of the LUAD patients, which was downloaded from TCGA. The relationship between the PcGScore and TMB was investigated using Spearman correlation analysis, while KM analysis was used to compare TMB and prognostic variations between the high- and low-risk groups. The non-synonymous point mutations in the somatic cells were counted using the “maftools” R package (30).
PcGScore correlation with LUAD immune status
The Estimation of Stromal and Immune Cells in Malignant Tumors Using the Expression Data (ESTIMATE) algorithm (31) was used to calculate the immune and stromal fractions in the samples, predict the level of infiltrating immune and stromal cells, and determine the purity of each tumor sample. The abundance of 23 tumor-infiltrating immune cell types was then calculated for each LUAD sample in the high- and low-risk groups in the training dataset using the ssGSEA algorithm, and the results were visualized using a boxplot.
Correlation analysis between the PcGScore and treatment sensitivity
Wilcoxon and Spearman tests were used to compare the differences between the PcGScore and the six key ICB genes, and the results were visualized using boxplots. The clinical response to ICIs in LUAD patients was measured using the Tumor Immune Dysfunction and Exclusion (TIDE) algorithm (32). A systemic search was conducted for ICB gene expression profiles, to further determine the accuracy of the PcGScore as a prognostic factor for immunotherapy. A single immunotherapy cohort (IMvigor210: http://research-pub.gene.com/IMvigor210CoreBiologies/), focusing on metastatic urothelial tumors, was included in the study (33,34). The “edgeR” R package was used to filter and normalize the raw data. Lastly, the relationship between PcGscore and four commonly used chemotherapeutic agents was assessed, and the “pRRophetic” R package (35) was used to calculate the half maximal inhibitory concentration (IC50) values of the chemotherapeutic drugs. The Wilcoxon test was used to compare the IC50 values between the high- and low-risk groups.
Statistical analysis
The Wilcoxon test was used to compare two groups, and the Kruskal-Wallis test was used to compare multiple groups. The “survival” software package was used for the KM analysis, and the log-rank test was used to determine statistically significant differences. The Spearman test was used for correlation analysis and correlation coefficient calculation. The R software (version 4.1.3) was used for all statistical analyses and P<0.05 was considered statistically significant.
Results
After excluding patients with no information on OS, the TCGA and GEO databases yielded a total of 935 LC patients for the follow-up study. Table 1 summarizes the main demographic and clinical characteristics of the LUAD sample in the aforementioned dataset.
Table 1
Characteristics | TCGA | GSE13213 | GSE30219 | GSE31210 |
---|---|---|---|---|
Number | 522 | 117 | 85 | 226 |
Age, median [range] | 66 [33–88] | 61 [32–84] | 60 [44–84] | 61 [30–76] |
Gender, n (%) | ||||
Female | 280 (53.6) | 57 (48.7) | 19 (22.4) | 121 (53.5) |
Male | 242 (46.4) | 60 (51.3) | 66 (77.6) | 105 (46.5) |
OS days (median) | 665 | 2,019 | 2,040 | 1,744.5 |
Survival status, n (%) | ||||
Alive | 334 (64.0) | 68 (58.1) | 40 (47.1) | 191 (84.5) |
Dead | 188 (36.0) | 49 (41.9) | 45 (52.9) | 35 (15.5) |
TNM stage, n (%) | ||||
I | 279 (53.4) | 79 (67.5) | 81 (95.3) | 168 (74.3) |
II | 124 (23.8) | 13 (11.1) | 4 (4.7) | 58 (25.7) |
III | 85 (16.3) | 25 (21.4) | 0 | 0 |
IV | 26 (5.0) | 0 | 0 | 0 |
LUAD, lung adenocarcinoma; TCGA, The Cancer Genome Atlas; OS, overall survival.
Genetic variation of the PcG-related genes in LUAD
Based on the previously reported literature, the 28 PcG-related genes were obtained in LUAD samples, which are summarized in the Table S1. The expression levels of the 28 PcG-related genes were determined in 59 normal tissues and 539 LUAD samples. The results revealed that MBTD1, PCGF2, PCGF3, PCGF6, PHC2, SCML2, SFMBT1, PCGF1, MTF2, EZH2 and SUZ12 were up-regulated, while EPC1, PCGF5, PHC1, RYBP, SCMH1, SCML4, PHF1 and EZH1 were significantly down-regulated in the LUAD samples, compared with their expression in the adjacent non-tumor tissues (Figure 1A). Analysis of the somatic mutation profiles revealed that the PcG-related genes were mutated in 110 samples of the 561 LUAD samples, with a mutation frequency of 19.61%. Furthermore, BCOR showed the highest mutation rate, followed by SFMBT2, ASXL1, and SCML2 (Figure 1B). The interaction networks of these genes were obtained from the Search Tool for the Retrieval of Interacting Genes/Proteins (STRING) database and visualized them with the “ggraph” R package, to further explore the relationships among the 28 PcG-related genes. As shown in Figure 1C, ASXL1, BMI1, EED, EZH1, and EZH2 showed higher interactions with the other genes. In addition, the CNV incidence of the 28 PcG-related genes were assessed in the TCGA cohort and CNV alterations were found to be widespread in the PcG-related genes, and different PcG-related genes exhibited unique deletions or amplifications. PHC3, MBTD1, EED, SCMH1, ASXL1, EZH2, etc., showed high copy number amplification frequencies, while MTF2, PHC1, SCML4, etc., showed high deletion frequencies (Figure 1D). Figure 1E demonstrates the location of the PcG-related genes on the chromosome. These results indicate that the PcG-related genes are highly heterogeneous in expression and genetic mutations in normal and tumor tissues, suggesting that the PcG-related genes are critical in the development of LUAD.
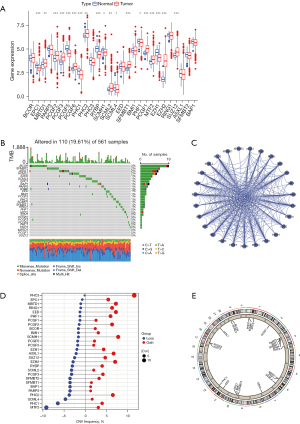
PcG patterns in LUAD
The training dataset consisted of 633 LUAD samples from the TCGA and GSE13213 datasets, which were subjected to consensus clustering analysis based on the expression of the PcG-related genes. The LUAD samples showed excellent clustering stability in the consensus matrix when the number of groups (K) was 2. Furthermore, the cumulative distribution function (CDF) reached its approximate maximum value when K was 2 (Figure 2A-2C). Therefore, the LUAD patients were divided into two PcG-related patterns: PcG cluster A and B (317 and 316 samples, respectively). Prognostic analysis of the two PcG patterns revealed that the PcG cluster A had a significantly lower survival advantage than PcG cluster B (P=0.004; Figure 2D). Additionally, PCA analysis revealed a substantial difference between the two cluster patterns (Figure 2E). Moreover, the heatmap in Figure 2F clearly demonstrates the variations in the expressions of PcG-related genes between the two clusters. Subsequent, immune cell infiltration analysis using ssGSEA revealed that the two PcG patterns differed significantly in immune cell infiltration characteristics (Figure 2G). Further examination revealed that the PcG cluster B was significantly infiltrated with innate immune cells, including eosinophils, myeloid-derived suppressor cells (MDSCs), macrophages, mast cells, monocytes, neutrophils, natural killer (NK) cell, etc. In contrast, the PcG cluster A showed significantly lower immune cell infiltration, indicating an immune-desert phenotype (36). These results strongly suggest that immune cell infiltration is important in the clustering, stratification, and progression of LUAD, thereby supporting the prognosis of the two PcG patterns described above. GSVA enrichment analysis of the two PcG patterns revealed that the PcG cluster A was primarily associated with DNA damage repair, including base excision repair, homologous recombination, mismatch repair, DNA replication, nucleotide excision repair, spliceosome, and RNA degradation. In contrast, the PcG cluster B was primarily associated with fatty acid metabolism, including arachidonic acid metabolism, linoleic acid metabolism, primary bile acid biosynthesis, and the peroxisome proliferator-activated receptor (PPAR) signaling pathway. Additionally, the PcG cluster B was significantly enriched in aldosterone-regulated sodium reabsorption; complement and coagulation cascades; calcium signaling pathways; neuroactive ligand receptor interactions; drug metabolism; and cytochrome P450 pathways (Figure 2H).
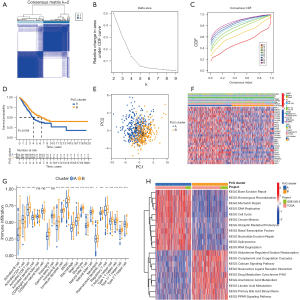
Development of the LUAD prognostic risk model
The 18 DEGs (FDR <0.01 and |log2FC| >1.5) were identified between the two PcG patterns, which were then subjected to GO and KEGG enrichment analyses. GO enrichment analysis showed that the DEGs were significantly enriched in nuclear division, as well as mitosis, in BP and chromatin condensation in cellular constituents (CC; Figure S1A,S1B). The KEGG enrichment analysis showed that the DEGs were enriched in platinum resistance (Figure S1C,S1D). After eliminating 13 samples that lacked complete OS temporal information, the univariate Cox regression analysis was performed and discovered 16 PcG-related genes associated with LUAD prognosis (P<0.01). Among these, nine genes were considered to be risk factors according to the hazard ratio (HR), while the remaining seven genes were considered to be protective factors (Figure 3A). Overfitting was avoided by performing LASSO Cox regression analysis. Finally, four core PcG-related genes including NIMA-related kinase 2 (NEK2), Anillin (ANLN), the Polymeric Immunoglobulin Receptor (PIGR), and Surfactant Protein C (SFTPC) were selected to develop a novel prognostic risk score model known as the PcGScore (Figure 3B,3C). The risk score of this model was calculated using the following formula: PcGScore = 0.0656816627702443 × mRNA expression of NEK2 + 0.186081340526048 × mRNA expression of ANLN + (−0.012442499748831) × mRNA expression of PIGR + (−0.0183563668042736) × mRNA expression of SFTPC. All the LUAD patients in the training dataset were categorized into high- and low-risk subgroups, based on the median risk score, and KM survival analysis revealed that the prognosis was higher for the low-risk group than for the high-risk group (P<0.001; Figure 3D). Furthermore, the ability of the PcGScore to accurately predict 1-, 3-, and 5-year survival of LUAD patients had an AUC of 0.692, 0.674, and 0.643, respectively (Figure 3E). The distribution of the PcGScore for LUAD patients can be seen in Figure 3F. The survival status of the LUAD patients is shown in Figure 3G, which demonstrates that the high-risk group showed higher death rate compared to the low-risk group. Furthermore, as seen in the heatmap (Figure 3H), NEK2 and ANLN were highly expressed in the PcGScore high-risk groups than in the PcGScore low-risk groups. In addition, the PCA and t-SNE algorithms verified that the samples in the two risk categories were distributed independently (Figure 3I-3J).
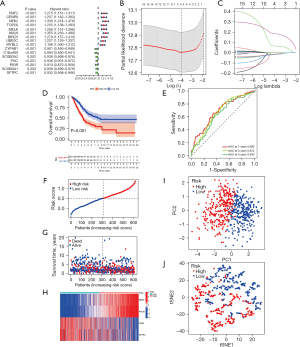
Correlation analysis of the PcGScore and clinical characteristics of LUAD
The PcGScore was further evaluated for its ability to predict the LUAD clinical parameters, such as the age, gender, T stage, N stage, and TNM stage. The PcGScore was found to vary significantly with age, gender, T stage, N stage, and TNM stage. Therefore, the long-term survival of the PcGScore and LUAD clinical characteristics were investigated further. The study showed that patients in the low-risk group had a greater survival advantage in age and gender than patients in the high-risk group. Furthermore, the early-stage LC patients in the low-risk group showed a better prognosis, while there was no significant difference in the survival of the advanced LC patients in the high- and low-risk groups (Figure 4A-4E). These results indicate that the PcGScore model is more accurate and applicable for assessing the clinical characteristics and survival status of the early-stage LC. However, due to the lack of similar samples distribution between cancer and healthy samples, there may be a lack of consistency between TNM staging within the TCGA dataset and of relevant clinicopathological data.
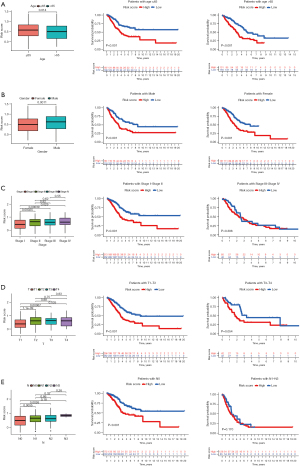
Construction of a prognostic nomogram for LUAD
Univariate and multivariate Cox regression analyses were conducted to determine whether the PcGScore prognostic model is an independent indicator for LUAD in the training dataset. The univariate Cox regression analysis revealed that the TNM stage and risk score were significantly associated with OS in LUAD patients (P<0.001), while the multivariate Cox analysis revealed that the age, TNM stage, and risk score were independent prognostic factors for LUAD patients (P<0.01; Figure 5A,5B). Thereafter, a nomogram was constructed based on these findings by combining the risk score with the two clinical characteristics: age and TNM stage (Figure 5C). The calibration plots revealed a high degree of agreement between the nomogram-predicted and actual 1-, 3-, and 5-year OS of the LUAD patients (Figure 5D). The 1-, 3-, and 5-year (AUC =0.758, 0.717, and 0.713, respectively) survival rates of the LUAD patients were also examined using ROC curves (Figure 5E), which revealed that the nomogram, combining the signature and clinical variables, outperformed a single clinical variable in terms of predictive accuracy.
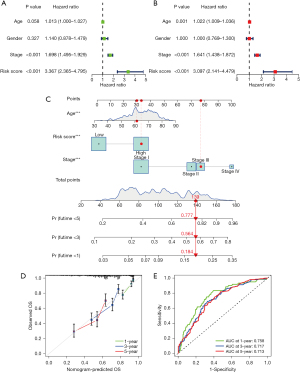
Validation of the signatures and the nomogram using external validation cohort
The validation dataset consisted of 311 LUAD samples from the GSE30219 and GSE31210 datasets. The validity and feasibility of the signatures and the nomogram were verified using the validation dataset, as described earlier. A risk score was calculated for each LUAD sample using the same formula, after which the samples were divided into high- and low-risk groups based on the median risk score. As shown in Figure 6A, the significant difference was discovered in the OS between the high- and low-risk groups in the validation dataset (P<0.01). The low-risk group showed a better chance of survival compared to the high-risk group, which was consistent with results of the training dataset. Furthermore, ROC curves from the validation dataset showed that the PcGScore model was extremely accurate in predicting the outcomes of the LUAD patients. According to the ROC curves, survival at 1-, 3-, and 5-year had an AUC of 0.705, 0.715, and 0.739, respectively (Figure 6B). Figure 6C shows the risk curve for the high- and low-risk groups. Figure 6D depicts the survival status of the LUAD patients, while the heatmap in Figure 6E demonstrates the differences in gene expression between the high- and low-risk groups. Furthermore, the PCA and t-SNE analyses revealed that the LUAD patients in the validation dataset can be divided into two groups (Figure 6F,6G). Moreover, univariate and multivariate Cox regression analyses of the validation dataset revealed that the risk score was an independent prognostic factor in LUAD (Figure 7A,7B). Furthermore, the nomogram based on the age, TNM stage, and risk score (Figure 7C) showed an excellent correlation between predicted and observed values, as observed by the calibration curves (Figure 7D). According to the nomogram, the AUCs of 1-, 3-, and 5-year OS were 0.677, 0.741, and 0.756, respectively (Figure 7E). Altogether, these results demonstrate the excellent predictive ability and reproducibility of the nomogram in predicting the OS of LUAD patients.
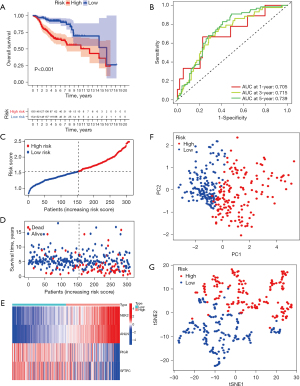
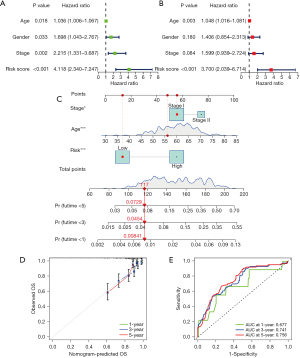
Correlation analysis of the PcGScore and TMB
Studies show that infiltrating CD8+ T cells are linked to TMB. For instance, infiltrating CD8+ T cells reduce TGF-β signaling in tumor stromal cells, following anti-PD-L1treatment, to facilitate T cell infiltration, resulting in intense tumor killing effects (33). In addition, patients with a high TMB showed better prognosis with anti-PD-L1 therapy (37). Therefore, we speculated that TMB might serve as a biomarker for ICB efficacy prediction. Considering that somatic mutation rates are high in LC (38), the possible interaction between the PcGScore and TMB is investigated. The study showed TMB was higher in the PcGScore high-risk group (P<2.22e-16; Figure 8A) and correlation analysis revealed that TMB was positively and significantly correlated with the PcGScore (R=0.43, P<2.2e-16; Figure 8B). In addition, the stratified survival analysis was performed to examine the potential synergistic effect of the PcGScore and TMB in the prognosis prediction of LUAD. Patients in the PcGscore low-risk and high TMB group showed the best prognosis, while those in a PcGScore high-risk and low TMB showed the worst prognosis (Figure 8C). Furthermore, the distribution of LUAD gene mutations in the PcGScore high- and low-risk groups was investigated, and the top 20 highly mutated genes in each group have been displayed in Figure 8D and Figure 8E. TP53 (62%) and MUC16 (29%) were the most frequently mutated genes in the high- and low-risk groups, respectively.
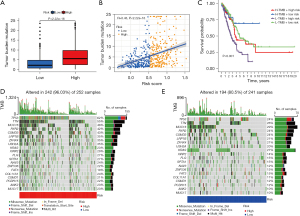
PcGScore correlation with LUAD immune status
In recent years, tumor microenvironment (TME) has emerged as a critical factor in tumorigenesis and progression (39,40). Thus, the potential relationship between the PcGScore and TME was investigated. The ESTIMATE algorithm revealed that immune, stromal, and estimated scores were higher in the PcGScore low-risk group compared to the PcGScore high-risk group, while tumor purity was higher in the PcGScore high-risk group (Figure 9A-9D). After that,the relationship between the PcGScore and immune infiltration was analyzed. The boxplot depicted the distribution of tumor-infiltrating cells in the high- and low-risk groups as predicted by the ssGSEA algorithm (Figure 9E). A large number of immune cells, including activated B cells, activated dendritic cells, eosinophils, immature B cells, immature dendritic cells, macrophages, mast cells, monocytes, NK cells, plasmacytoid dendritic cells, T follicular helper cells, type 17 helper cells, and type 1 helper cells, were found in the PcGscore low-risk group. In contrast, immune cell infiltration was significantly decreased in the PcGScore high-risk group. Moreover, the correlation analysis confirmed that the majority of the infiltrating cells were negatively correlated with the PcGScore (Figure 9F). According to a previous study, immune infiltration can be classified into immune-inflamed, immune-excluded, and immune-desert phenotypes. The immune cells accumulate in the stroma surrounding the tumors, instead of the tumor parenchyma, resulting in the immune-excluded phenotypic state. In contrast, the immune-desert phenotype is characterized by a low immune cell infiltration (36,41). The results of the current study have demonstrated that different PcGScores indicate different immune cell infiltration characteristics. The PcGScore low-risk group exhibited a characteristic immune-excluded phenotype (massive immune cell infiltration and stromal activation), while the PcGScore high-risk group exhibited an immune-desert phenotype (a weakened immune cell infiltration). These results indicate that the PcGScore may play a significant role in the TME of the LUAD patients.
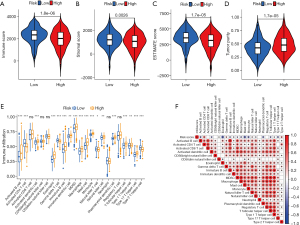
PcGScore shows great potential in evaluating therapeutic sensitivity in LUAD
Immunotherapy is expected to become the preferred mode of treatment for cancer, owing to the successful use of ICIs, such as anti-CTLA4 and anti-PD-1/PD-L1, in several tumors (42-44). Thus, six main immune checkpoint genes were examined in LUAD patients to determine whether the PcGScore could be used to predict the prognosis of immunotherapy. The findings revealed that PD-L1, PDCD1, PDCD1LG2, LAG3, and IDO1 had higher expression in the high-risk group compared to the low-risk group, whereas CTLA4 expression did not differ significantly between the two groups (Figure 10A-10F). A correlation analysis revealed that the six main immune checkpoint genes were positively correlated with the PcGScore (Figure 10G), suggesting that immunotherapy may be more effective for the high-risk group. Thereafter, the accuracy of the PcGScore was tested in predicting immunotherapy response by using TIDE and IMvigor210 cohort. TIDE is a computational approach that models two major mechanisms of tumor immune evasion: inducing T-cell dysfunction in tumors with high cytotoxic T-lymphocyte (CTL) infiltration and blocking T-cell infiltration in tumors with low levels of CTL infiltration (32). Prediction of the therapeutic efficacy of ICI by TIDE revealed that the TIDE score was higher in the PcGScore low-risk group and there was a significant negative correlation between PcGScore and TIDE score (R=−0.45, P<2.2e-16; Figure 10H,10I). These results indicate that immune evasion is more common in immunotherapy patients in the low-risk group compared to those in the high-risk group. Patients in the IMvigor210 cohort who received the ICI therapy were analyzed to determine if the PcGScore could predict their response to the ICI treatment. The results of this study indicate that a higher percentage of patients responded to the ICI therapy in the high-risk group compared to the low-risk group (Figure 10J,10K). Therefore, the PcGScore was shown to have a great potential in predicting the prognosis of immunotherapy for patients in the high-risk group. Lastly, the correlation was analyzed between the PcGScore and the four commonly used chemotherapeutic drugs: cisplatin, paclitaxel, docetaxel, and gemcitabine. There was a significant difference in the estimated IC50 values of the chemotherapeutic drugs between the two risk groups, with the IC50 values being lower in the high-risk group (P<0.001; Figure 10L-10O), suggesting that chemotherapy may be more effective for patients in the high-risk group. Altogether, these results suggest that the PcGScore has a great potential for predicting the sensitivity of immunotherapy and chemotherapeutic treatments.
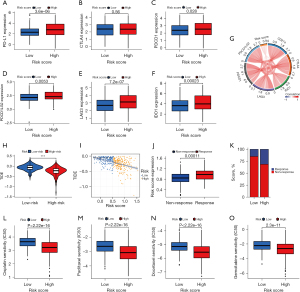
Discussion
It is estimated that more than one million people die each year from LC, making it one of the most common cancers worldwide (45). However, since early symptoms of LC are difficult to detect, approximately 75% of the patients are found to have advanced LC at the time of diagnosis (46). According to the previous studies, the 5-year OS rate for advanced LC is approximately 6%, whereas the OS rate for early-stage LC is approximately 82% (47). Although non-invasive techniques have increased the chances of early detection of LC, only 16% of the patients are detected in the early stages (48). Therefore, there is an urgent need for novel and more effective methods for early diagnosis and treatment of LC. The PcG is a family of chromatin regulators that is overexpressed in several tumors, including melanoma, chronic myelogenous leukemia, prostate cancer, breast cancer, ovarian cancer, LC, etc. and has been linked to poor prognosis in many cases (49-55). In addition, PcG proteins play a critical role in cell proliferation (56), apoptosis (57), and senescence (58) as well as tumor progression, depending on the cellular environment (59). However, the role of PcG proteins in LUAD has not yet been established. Therefore, we developed a novel independent predictive model based on the PcG-related genes to predict the prognosis and treatment of LUAD patients.
Based on the expression levels of 28 PcG-related genes, two distinct PcG patterns were identified in LUAD, which differed significantly in the survival and immune infiltration of LUAD patients. Subsequently, evaluation of the immune cell infiltration in TME using the ssGSEA method revealed that immune cells, especially innate immune cells, including eosinophils, MDSCs, macrophages, mast cells, monocytes, neutrophils, NK cells, etc., were highly infiltrated in the PcG cluster B. In contrast, PcG cluster A showed an immune-desert phenotype, which is characterized by a significantly reduced infiltration of immune cells. Furthermore, consistent with the previously observed results, PcG cluster A showed the worst prognostic survival, possibly due to the low infiltration of the protective immune cells. GSVA enrichment analysis of the PcG patterns showed that the PcG cluster A was predominantly enriched in DNA damage repair, while the PcG cluster B was significantly enriched in various metabolic pathways, including fatty acid metabolism. It has been demonstrated that genomic instability, a risk factor for cancer, is closely associated with the accumulation of DNA damage over time (60), which forms the basis for radiation therapy and chemotherapy in cancer treatment (61). Mutations in some key genes, including oncogenes, tumor-suppressor genes, and cell cycleregulatory genes, may produce clonal cell populations with a significant proliferative advantage, leading to tumorigenesis (62). Furthermore, cellular metabolism is another characteristic feature of cancer , since limiting the use of fatty acids, which are required for the synthesis of membranes and signaling molecules, has been shown to inhibit cancer cell proliferation (63). These findings suggest that PcG-related genes play a key role in tumorigenesis and progression and should be explored further for their use in the diagnosis and treatment of LUAD.
Subsequently, 18 DEGs were identified between the two PcG patterns, which were significantly enriched in cell proliferation and division. Moreover, existing studies have found a strong correlation between normal stem cell division and cancer incidence (64), reaffirming the existence of a strong correlation between the PcG-related genes and tumorigenesis. Furthermore, the prognostic model was developed to evaluate the prognosis and sensitivity of LUAD patients with regard to different types of treatments. The LUAD patients from the training (TCGA and GSE13213) and validation (GSE30219 and GSE31210) datasets were divided into high- and low-risk groups based on the median risk score, and patients in the high-risk group showed a poorer prognosis. The PcGScore was also found to be an independent predictor in both univariate and multifactorial Cox regression analyses. Furthermore, the prognostic nomogram model, constructed by combining the PcGScore and multifactorial Cox analysis results, outperformed clinical characteristics in predicting 1-, 3-, and 5-year OS of LUAD patients, which was further validated using the validation dataset. Therefore, the study results provide a new direction and strategy for clinical diagnosis and treatment of LUAD.
Furthermore, TMB can be used to predict immunotherapy response (65), and patients treated with ICI show a better prognosis with elevated TMB (66). Therefore, the potential association of the PcGScore with TMB was explored and TMB was found to increase significantly with the PcGScore. Patients with a low PcGScore and a high TMB showed the best prognosis, as shown by the stratified survival analysis, which is consistent with the previously reported literature (66). Additionally, the study showed that TP53 and MUC16 were the most frequently mutated genes in the high- and low-risk groups. Previous studies have shown that in NSCLC, TP53 mutations significantly increase the expression of immune checkpoints, activated T-cell effectors, and γ-interferon signatures, as well as TMB, implying that patients with TP53 mutations may benefit more from ICI therapy (67). Additionally, patients with high MUC16 mutation rates have higher TMB, better ICI response, and a better prognosis (68). These findings imply that differences in the distribution of the PcGScore-related somatic mutation driver genes are significantly associated with antitumor immunity and that the complex regulatory mechanism of their interaction may provide a new direction for immunotherapy in LUAD.
Subsequently, the ESTIMATE and ssGSEA algorithms were employed to compare the differences between PcGScore high- and low-risk groups, to further understand the underlying mechanisms associated with the PcGScore and TME. The study revealed that almost all immune cell infiltrations and immune scores were negatively-correlated with the PcGScore significantly, suggesting that the PcGScore may serve as an indicator of immunosuppression. The PcGScore high- and low-risk groups demonstrated immune-desert and immune-excluded phenotypes, respectively, which is consistent with the better prognosis of the PcGScore low-risk group and the worse prognosis of the PcGscore high-risk group. Therefore, the PCGScore has a significant impact on the TME in LUAD.
During the last decade, there was a significant improvement in the long-term survival rates of advanced NSCLC patients, due to the use of PD-1/PD-L1 blocking antibodies (69,70). Thus, the correlation analysis between the PcGScore and immune checkpoints was investigated, and five common immune checkpoint genes were found to be significantly expressed in the high-risk group. Thereafter, the study showed that the PcGScore can accurately predict response to ICI therapy. The TIDE scores were higher in the low-risk group compared to those in the high-risk group. Additionally, the ICI therapy was more effective for patients in the high-risk group. Lastly, analysis of the association between the PcGScore and common clinical chemotherapeutic drugs revealed that the IC50 values were lower in the high-risk group, suggesting that the high-risk group may benefit more from chemotherapy. Altogether, these findings suggest that patients in the high-risk group respond better to immunotherapy and chemotherapy, and that PcGScoer may serve as a marker for predicting response to immunotherapy and chemotherapy in LUAD.
Conclusions
In summary, the PcGScore model was constructed using four PcG-related genes to predict prognosis and treatment responsiveness in LUAD. The predictive ability of the model was further validated, thus providing an ideal and stable predictor for the clinical treatment of the LUAD patients. However, this study has a few limitations. Firstly, the stability of the PcGScore stability was only tested and validated by two independent cohorts in this paper. Secondly, prospective cohort studies are required to demonstrate the validity of the PcG-related genetic signature. Moreover, the additional unrelated immunotherapy cohorts are required to confirm the stability and reliability of the PcGScore in predicting the outcome of immunotherapy in LUAD. Lastly, to fully comprehend the unique role of the PcG-related genes in the development of LUAD and their regulatory mechanisms, it is necessary that the identified DEGs are further validated by in vivo and in vitro studies.
Acknowledgments
We thank the Gene Expression Omnibus (GEO) database and The Cancer Genome Atlas (TCGA) for sharing data. We thank Bullet Edits Limited for the linguistic editing and proofreading of the manuscript.
Funding: This work was supported by the National Natural Science Foundation of China (No. 81770266).
Footnote
Reporting Checklist: The authors have completed the TRIPOD reporting checklist. Available at https://jtd.amegroups.com/article/view/10.21037/jtd-22-1324/rc
Conflicts of Interest: All authors have completed the ICMJE uniform disclosure form (available at https://jtd.amegroups.com/article/view/10.21037/jtd-22-1324/coif). The authors have no conflicts of interest to declare.
Ethical Statement: The
Open Access Statement: This is an Open Access article distributed in accordance with the Creative Commons Attribution-NonCommercial-NoDerivs 4.0 International License (CC BY-NC-ND 4.0), which permits the non-commercial replication and distribution of the article with the strict proviso that no changes or edits are made and the original work is properly cited (including links to both the formal publication through the relevant DOI and the license). See: https://creativecommons.org/licenses/by-nc-nd/4.0/.
References
- Didkowska J, Wojciechowska U, Mańczuk M, et al. Lung cancer epidemiology: contemporary and future challenges worldwide. Ann Transl Med 2016;4:150. [Crossref] [PubMed]
- Li MY, Liu LZ, Dong M. Progress on pivotal role and application of exosome in lung cancer carcinogenesis, diagnosis, therapy and prognosis. Mol Cancer 2021;20:22. [Crossref] [PubMed]
- Zappa C, Mousa SA. Non-small cell lung cancer: current treatment and future advances. Transl Lung Cancer Res 2016;5:288-300. [Crossref] [PubMed]
- Osmani L, Askin F, Gabrielson E, et al. Current WHO guidelines and the critical role of immunohistochemical markers in the subclassification of non-small cell lung carcinoma (NSCLC): Moving from targeted therapy to immunotherapy. Semin Cancer Biol 2018;52:103-9. [Crossref] [PubMed]
- Jin JO, Puranik N, Bui QT, et al. The Ubiquitin System: An Emerging Therapeutic Target for Lung Cancer. Int J Mol Sci 2021;22:9629. [Crossref] [PubMed]
- Siegel RL, Miller KD, Jemal A. Cancer statistics, 2016. CA Cancer J Clin 2016;66:7-30. [Crossref] [PubMed]
- Borghaei H, Paz-Ares L, Horn L, et al. Nivolumab versus Docetaxel in Advanced Nonsquamous Non-Small-Cell Lung Cancer. N Engl J Med 2015;373:1627-39. [Crossref] [PubMed]
- Zhang Y, Zhang Z. The history and advances in cancer immunotherapy: understanding the characteristics of tumor-infiltrating immune cells and their therapeutic implications. Cell Mol Immunol 2020;17:807-21. [Crossref] [PubMed]
- Fitieh A, Locke AJ, Motamedi M, et al. The Role of Polycomb Group Protein BMI1 in DNA Repair and Genomic Stability. Int J Mol Sci 2021;22:2976. [Crossref] [PubMed]
- Au SL, Ng IO, Wong CM. Epigenetic dysregulation in hepatocellular carcinoma: focus on polycomb group proteins. Front Med 2013;7:231-41. [Crossref] [PubMed]
- Zhao X, Wu X. Polycomb-group proteins in the initiation and progression of cancer. J Genet Genomics 2021;48:433-43. [Crossref] [PubMed]
- Gao S, Wang SY, Zhang XD, et al. Low Expression of the Polycomb Protein RING1 Predicts Poor Prognosis in Human Breast Cancer. Front Oncol 2020;10:618768. [Crossref] [PubMed]
- Zhou Y, Wan C, Liu Y, et al. Polycomb group oncogene RING1 is over-expressed in non-small cell lung cancer. Pathol Oncol Res 2014;20:549-56. [Crossref] [PubMed]
- Zhu K, Li J, Li J, et al. Ring1 promotes the transformation of hepatic progenitor cells into cancer stem cells through the Wnt/β-catenin signaling pathway. J Cell Biochem 2020;121:3941-51. [Crossref] [PubMed]
- Duan R, Du W, Guo W. EZH2: a novel target for cancer treatment. J Hematol Oncol 2020;13:104. [Crossref] [PubMed]
- Kong Y, Zhang Y, Mao F, et al. Inhibition of EZH2 Enhances the Antitumor Efficacy of Metformin in Prostate Cancer. Mol Cancer Ther 2020;19:2490-501. [Crossref] [PubMed]
- Peng D, Kryczek I, Nagarsheth N, et al. Epigenetic silencing of TH1-type chemokines shapes tumour immunity and immunotherapy. Nature 2015;527:249-53. [Crossref] [PubMed]
- Dangaj D, Bruand M, Grimm AJ, et al. Cooperation between Constitutive and Inducible Chemokines Enables T Cell Engraftment and Immune Attack in Solid Tumors. Cancer Cell 2019;35:885-900.e10. [Crossref] [PubMed]
- Tao T, Chen M, Jiang R, et al. Involvement of EZH2 in aerobic glycolysis of prostate cancer through miR-181b/HK2 axis. Oncol Rep 2017;37:1430-6. [Crossref] [PubMed]
- Yiew NKH, Greenway C, Zarzour A, et al. Enhancer of zeste homolog 2 (EZH2) regulates adipocyte lipid metabolism independent of adipogenic differentiation: Role of apolipoprotein E. J Biol Chem 2019;294:8577-91. [Crossref] [PubMed]
- Liu X, Lu X, Zhen F, et al. LINC00665 Induces Acquired Resistance to Gefitinib through Recruiting EZH2 and Activating PI3K/AKT Pathway in NSCLC. Mol Ther Nucleic Acids 2019;16:155-61. [Crossref] [PubMed]
- Kim KH, Roberts CW. Targeting EZH2 in cancer. Nat Med 2016;22:128-34. [Crossref] [PubMed]
- Gibbons SM, Duvallet C, Alm EJ. Correcting for batch effects in case-control microbiome studies. PLoS Comput Biol 2018;14:e1006102. [Crossref] [PubMed]
- Zhang H, Meltzer P, Davis S. RCircos: an R package for Circos 2D track plots. BMC Bioinformatics 2013;14:244. [Crossref] [PubMed]
- Wilkerson MD, Hayes DN. ConsensusClusterPlus: a class discovery tool with confidence assessments and item tracking. Bioinformatics 2010;26:1572-3. [Crossref] [PubMed]
- Hänzelmann S, Castelo R, Guinney J. GSVA: gene set variation analysis for microarray and RNA-seq data. BMC Bioinformatics 2013;14:7. [Crossref] [PubMed]
- Ritchie ME, Phipson B, Wu D, et al. limma powers differential expression analyses for RNA-sequencing and microarray studies. Nucleic Acids Res 2015;43:e47. [Crossref] [PubMed]
- Yu G, Wang LG, Han Y, et al. clusterProfiler: an R package for comparing biological themes among gene clusters. OMICS 2012;16:284-7. [Crossref] [PubMed]
- Engebretsen S, Bohlin J. Statistical predictions with glmnet. Clin Epigenetics 2019;11:123. [Crossref] [PubMed]
- Mayakonda A, Lin DC, Assenov Y, et al. Maftools: efficient and comprehensive analysis of somatic variants in cancer. Genome Res 2018;28:1747-56. [Crossref] [PubMed]
- Yoshihara K, Shahmoradgoli M, Martínez E, et al. Inferring tumour purity and stromal and immune cell admixture from expression data. Nat Commun 2013;4:2612. [Crossref] [PubMed]
- Jiang P, Gu S, Pan D, et al. Signatures of T cell dysfunction and exclusion predict cancer immunotherapy response. Nat Med 2018;24:1550-8. [Crossref] [PubMed]
- Mariathasan S, Turley SJ, Nickles D, et al. TGFβ attenuates tumour response to PD-L1 blockade by contributing to exclusion of T cells. Nature 2018;554:544-8. [Crossref] [PubMed]
- Balar AV, Galsky MD, Rosenberg JE, et al. Atezolizumab as first-line treatment in cisplatin-ineligible patients with locally advanced and metastatic urothelial carcinoma: a single-arm, multicentre, phase 2 trial. Lancet 2017;389:67-76. [Crossref] [PubMed]
- Geeleher P, Cox N, Huang RS. pRRophetic: an R package for prediction of clinical chemotherapeutic response from tumor gene expression levels. PLoS One 2014;9:e107468. [Crossref] [PubMed]
- Chen DS, Mellman I. Elements of cancer immunity and the cancer-immune set point. Nature 2017;541:321-30. [Crossref] [PubMed]
- Rizvi NA, Hellmann MD, Snyder A, et al. Cancer immunology. Mutational landscape determines sensitivity to PD-1 blockade in non-small cell lung cancer. Science 2015;348:124-8. [Crossref] [PubMed]
- Alexandrov LB, Nik-Zainal S, Wedge DC, et al. Signatures of mutational processes in human cancer. Nature 2013;500:415-21. [Crossref] [PubMed]
- Jing X, Yang F, Shao C, et al. Role of hypoxia in cancer therapy by regulating the tumor microenvironment. Mol Cancer 2019;18:157. [Crossref] [PubMed]
- Zeng D, Li M, Zhou R, et al. Tumor Microenvironment Characterization in Gastric Cancer Identifies Prognostic and Immunotherapeutically Relevant Gene Signatures. Cancer Immunol Res 2019;7:737-50. [Crossref] [PubMed]
- Xu Q, Chen S, Hu Y, et al. Landscape of Immune Microenvironment Under Immune Cell Infiltration Pattern in Breast Cancer. Front Immunol 2021;12:711433. [Crossref] [PubMed]
- Callahan MK, Postow MA, Wolchok JD. CTLA-4 and PD-1 Pathway Blockade: Combinations in the Clinic. Front Oncol 2014;4:385. [PubMed]
- Goodman A, Patel SP, Kurzrock R. PD-1-PD-L1 immune-checkpoint blockade in B-cell lymphomas. Nat Rev Clin Oncol 2017;14:203-20. [Crossref] [PubMed]
- Postow MA, Callahan MK, Wolchok JD. Immune Checkpoint Blockade in Cancer Therapy. J Clin Oncol 2015;33:1974-82. [Crossref] [PubMed]
- Rahal Z, El Nemr S, Sinjab A, et al. Smoking and Lung Cancer: A Geo-Regional Perspective. Front Oncol 2017;7:194. [Crossref] [PubMed]
- Wadowska K, Bil-Lula I, Trembecki Ł, et al. Genetic Markers in Lung Cancer Diagnosis: A Review. Int J Mol Sci 2020;21:4569. [Crossref] [PubMed]
- Xi KX, Zhang XW, Yu XY, et al. The role of plasma miRNAs in the diagnosis of pulmonary nodules. J Thorac Dis 2018;10:4032-41. [Crossref] [PubMed]
- Hirsch FR, Scagliotti GV, Mulshine JL, et al. Lung cancer: current therapies and new targeted treatments. Lancet 2017;389:299-311. [Crossref] [PubMed]
- Parreno V, Martinez AM, Cavalli G. Mechanisms of Polycomb group protein function in cancer. Cell Res 2022;32:231-53. [Crossref] [PubMed]
- Zhang H, Qi J, Reyes JM, et al. Oncogenic Deregulation of EZH2 as an Opportunity for Targeted Therapy in Lung Cancer. Cancer Discov 2016;6:1006-21. [Crossref] [PubMed]
- Li H, Cai Q, Godwin AK, et al. Enhancer of zeste homolog 2 promotes the proliferation and invasion of epithelial ovarian cancer cells. Mol Cancer Res 2010;8:1610-8. [Crossref] [PubMed]
- Anwar T, Arellano-Garcia C, Ropa J, et al. p38-mediated phosphorylation at T367 induces EZH2 cytoplasmic localization to promote breast cancer metastasis. Nat Commun 2018;9:2801. [Crossref] [PubMed]
- Morel KL, Sheahan AV, Burkhart DL, et al. EZH2 inhibition activates a dsRNA-STING-interferon stress axis that potentiates response to PD-1 checkpoint blockade in prostate cancer. Nat Cancer 2021;2:444-56. [Crossref] [PubMed]
- Xie H, Peng C, Huang J, et al. Chronic Myelogenous Leukemia- Initiating Cells Require Polycomb Group Protein EZH2. Cancer Discov 2016;6:1237-47. [Crossref] [PubMed]
- Jackson PK. EZH2 Inactivates Primary Cilia to Activate Wnt and Drive Melanoma. Cancer Cell 2018;34:3-5. [Crossref] [PubMed]
- Nutt SL, Keenan C, Chopin M, et al. EZH2 function in immune cell development. Biol Chem 2020;401:933-43. [Crossref] [PubMed]
- Yao Y, Hu H, Yang Y, et al. Downregulation of Enhancer of Zeste Homolog 2 (EZH2) is essential for the Induction of Autophagy and Apoptosis in Colorectal Cancer Cells. Genes (Basel) 2016;7:83. [Crossref] [PubMed]
- Ito T, Teo YV, Evans SA, et al. Regulation of Cellular Senescence by Polycomb Chromatin Modifiers through Distinct DNA Damage- and Histone Methylation-Dependent Pathways. Cell Rep 2018;22:3480-92. [Crossref] [PubMed]
- Anwar T, Gonzalez ME, Kleer CG. Noncanonical Functions of the Polycomb Group Protein EZH2 in Breast Cancer. Am J Pathol 2021;191:774-83. [Crossref] [PubMed]
- O'Connor MJ. Targeting the DNA Damage Response in Cancer. Mol Cell 2015;60:547-60. [Crossref] [PubMed]
- Huang R, Zhou PK. DNA damage repair: historical perspectives, mechanistic pathways and clinical translation for targeted cancer therapy. Signal Transduct Target Ther 2021;6:254. [Crossref] [PubMed]
- Basu AK. DNA Damage, Mutagenesis and Cancer. Int J Mol Sci 2018;19:970. [Crossref] [PubMed]
- Currie E, Schulze A, Zechner R, et al. Cellular fatty acid metabolism and cancer. Cell Metab 2013;18:153-61. [Crossref] [PubMed]
- Tomasetti C, Li L, Vogelstein B. Stem cell divisions, somatic mutations, cancer etiology, and cancer prevention. Science 2017;355:1330-4. [Crossref] [PubMed]
- Liu L, Bai X, Wang J, et al. Combination of TMB and CNA Stratifies Prognostic and Predictive Responses to Immunotherapy Across Metastatic Cancer. Clin Cancer Res 2019;25:7413-23. [Crossref] [PubMed]
- Samstein RM, Lee CH, Shoushtari AN, et al. Tumor mutational load predicts survival after immunotherapy across multiple cancer types. Nat Genet 2019;51:202-6. [Crossref] [PubMed]
- Dong ZY, Zhong WZ, Zhang XC, et al. Potential Predictive Value of TP53 and KRAS Mutation Status for Response to PD-1 Blockade Immunotherapy in Lung Adenocarcinoma. Clin Cancer Res 2017;23:3012-24. [Crossref] [PubMed]
- Zhang L, Han X, Shi Y. Association of MUC16 Mutation With Response to Immune Checkpoint Inhibitors in Solid Tumors. JAMA Netw Open 2020;3:e2013201. [Crossref] [PubMed]
- Huang MY, Jiang XM, Wang BL, et al. Combination therapy with PD-1/PD-L1 blockade in non-small cell lung cancer: strategies and mechanisms. Pharmacol Ther 2021;219:107694. [Crossref] [PubMed]
- Xia L, Liu Y, Wang Y. PD-1/PD-L1 Blockade Therapy in Advanced Non-Small-Cell Lung Cancer: Current Status and Future Directions. Oncologist 2019;24:S31-41. [Crossref] [PubMed]