Identification of the ferroptosis regulator HELLS with prognostic value for adrenocortical carcinoma based on integrated analysis and experimental validation
Highlight box
Key findings
• A ferroptosis regulator signature showed promising power for predicting the prognosis of adrenocortical carcinoma (ACC), and HELLS was identified as a hub ferroptosis regulator in the initiation and progression of ACC.
What is known and what is new?
• Ferroptosis, a form of cell death, has been reported to be a promising therapeutic method for ACC; however, the core ferroptosis regulator with prognostic value in ACC remains unknown.
• We developed a 3-ferroptosis regulator prognostic signature that included HELLS, which may determine the biological and clinical progression of ACC.
What is the implication, and what should change now?
• Given the high recurrence rate of ACC after surgery and extremely limited drugs for therapy, this study provides the possibility of using ferroptosis agonists to treat ACC.
Introduction
Adrenocortical carcinoma (ACC), a malignant tumor originating from the adrenal cortex, has an annual incidence of 0.7–2.0 cases per million people and a low overall survival rate (1,2). Although ACC cells may secrete excess adrenocortical hormones to that cause some clinical features, most patients are diagnosed with local invasion or metastatic disease. Surgery is the most widely used method for treating ACC, and some adjuvant systemic therapies administered based on the tumor stage and grade may prolong the survival of patients with ACC. However, the local recurrence rate of ACC after surgery ranges from 19% to 34% (3). Meanwhile, adjuvant systemic therapies show limited therapeutic effectiveness (4). Predicting the overall survival and recurrence rate of ACC and choosing effective drug adjuvant therapies remain difficult. The prognostic factors for ACC include the tumor-node-metastasis (TNM) classification stage and tumor site (5); however, these factors are not adequate for accurate prognostic prediction. To improve prognostic prediction, many studies have focused on gene expression for the construction of a prognostic signature for ACC. Members of the gene signature for ACC mainly include immune-related genes (6), alternative splicing events (7), and m6A RNA methylation (8-11). However, the underlying mechanisms of ACC are complex and heterogenous; therefore, the biological process associated with ACC progression requires further analysis. Studies of the underlying biological mechanism of ACC are urgently needed for the development of more comprehensive guidelines for ACC treatment.
Ferroptosis, a form of regulated cell death, is an iron-dependent process characterized by extensive lipid peroxidation (12). Various agents that can trigger cell death by inducing ferroptosis have emerged as promising therapeutic alternatives for overcoming drug resistance (13). Mitotane, used as the standard treatment for ACC, has many undesirable side effects. A recent study showed that ACC is highly sensitive to treatment with inducers of ferroptosis, and thus, current standard therapy with mitotane may be examined with reference to ferroptosis inducers in the future (14). Another study reported that adrenal cortex cells are sensitive to ferroptosis owing to their active steroid synthetic pathways, which give rise to protective mechanisms against oxidative stress (15). As lipid hydroperoxides accumulate during ferroptosis and as ACC cells contain both enzymes and lipids that are crucial for ferroptosis execution, cells of the adrenal cortex are particularly sensitive to type II ferroptosis induction (15).
Immune checkpoint inhibitors have been used in the therapy of many cancer types resistant to chemotherapy; however, results of several clinical trials on immunotherapy in ACC are unsatisfactory, with median progression-free survival times ranging from 1.8 to 6.75 months (16-19). One potential reason for these underwhelming results may be excess tumorous glucocorticoid secretion, which is a well-known immunosuppressive that acts through the regulation of circulating and tumor-infiltrating immune cells (20-22). For instance, glucocorticoid secretion can inhibit T-helper-cell and cytotoxic T-lymphocyte activation, which are crucial for T-cell receptor–mediated activation of the adaptive immune system (23). One remarkable study reported that CD8+ T cells enhance ferroptosis by downregulating SLC3A2 and SLC7A11, and the induction of ferroptosis contributes to the antitumor efficacy of immunotherapy, suggesting that the immune system might, at least in part, function through ferroptosis (24,25). However, the roles of ferroptosis regulators in the progression and tumor microenvironment of ACC and whether these regulators can predict the survival of patients with ACC remain unknown.
Given the limitations of adjuvant treatment of ACC, we speculated that ferroptosis agonists might provide a novel direction for ACC treatment. Therefore, we aimed to identify hub ferroptosis genes by analyzing the differential expression of 113 reported ferroptosis regulators in ACC and explore their biological functions. The imbalanced expression of ferroptosis regulators between normal and ACC tissues was associated with prognostic values. Using a multivariate Cox regression model, we established a 3-gene signature to accurately predict the outcomes of patients with ACC. We validated the ferroptosis regulator signature by using 3 external validation datasets. Our data suggested that ferroptosis-related genes play pivotal roles in ACC progression and hold prognostic value for ACC. The underlying biological function between different risk groups was found to be associated with metabolism; E2F-, MYC-, and G2/M checkpoint-related pathways; and immune cell infiltration levels. Importantly, using integrated analysis and immunohistochemistry staining, we discovered HELLS to be a core ferroptosis regulator in ACC, which might be a promising object for future research into ACC treatment. Furthermore, we speculated that ferroptosis agonists might have application prospects in ACC. We present this article in accordance with the TRIPOD reporting checklist (available at https://gs.amegroups.com/article/view/10.21037/gs-22-736/rc).
Methods
Data collection and processing
RNA sequencing data and clinical information of patients with ACC in The Cancer Genome Atlas (TCGA) database were downloaded from the XENA database (https://xenabrowser.net/datapages/). The gene expression profiles and clinical information of patients with ACC in the Gene Expression Omnibus (GEO) database were directly downloaded from the GEO database (https://www.ncbi.nlm.nih.gov/geo/) (Table S1). Due to detection in the same GPL570 platform, the expression profiles of GSE10927 and GSE19750 were normalized with the removal of batch effects to a single dataset for further study. The matrix profiles of the datasets GSE76019 and GSE76021 detected by GPL13158 and GPL96, respectively, were directly downloaded from the GEO database. Transcriptomic data and clinical data for normal adrenal glands listed in the Genotype-Tissue Expression Project (GTEx) were also downloaded from the XENA database. We normalized and merged the transcriptome data of ACC and normal adrenal tissues from TCGA and GTEx into a single dataset using the fragments per kilobase of transcript per million fragments mapped value for further analysis. A total of 113 ferroptosis regulators were extracted from the online website FerrDb (http://www.zhounan.org/ferrdb/), including 49 suppressors, 61 drivers, and 3 markers, with validated confidence levels in the Homo sapiens experiment; specific information on these genes is shown in Table S2.
Identification of differentially expressed ferroptosis regulators
For differentially expressed gene analysis, we defined 2 groups: group 1, consisting of 127 normal adrenal tissues from GTEx and 79 ACC tissues from TCGA; and group 2, consisting of 14 normal adrenal tissues and 77 ACC tissues from GSE10927 and GSE19750. A false discovery rate adjusted to P<0.05 was defined as the cutoff criterion for differentially expressed ferroptosis regulators between the normal and ACC tissues. The corresponding heatmap and box plot were depicted in R software (The R Foundation for Statistical Computing) using the “complexheatmap” and “ggplot2” packages.
Construction of the ferroptosis regulator signature
Based on the gene expression of ferroptosis regulators in TCGA and patient survival, the cutoff points for each regulator were determined using the “survminer” R package. The best separation cutoff points for each regulator were found using the “surv-cutpoint” function. Samples were divided into the high- and low-risk groups based on the maximally selected log-rank statistics under the condition that the number of each group was not less than 40% of the total. Candidate ferroptosis regulators with prognostic value were subjected to multivariate Cox regression analysis to construct a gene signature for predicting the overall survival probability of patients. Based on the coefficient value, the risk score was computed as follows (26,27): risk score = ∑ βi × expRNAi, where expRNA is the expression of the gene and β is the coefficient value. The sensitivity and specificity of the receiver operating characteristic (ROC) curve were determined to evaluate the prognostic accuracy of the signature. The threshold values of area under the curve (AUC) for assessing the predictive accuracy in both the training and validation datasets were set to 0.7. A nomogram was built using the R package “rms” to predict the survival probability, and a calibration plot was used to test the predictive accuracy. To validate the prognostic prediction value of the signature, we chose 3 external independent datasets to calculate the risk scores with the same coefficient value of each gene in the signature.
Gene set variation analysis (GSVA)
To investigate the biological process differences between the high- and low-risk groups, we performed GSVA, a nonparametric and unsupervised method for estimating variation levels in pathways, using the “GSVA” R package. The gene sets of hallmark genes downloaded from the Molecular Signatures Database (MSigDB) database were employed for GSVA. An adjusted P value <0.05 was considered to indicate a statistically significant result.
The infiltration level analysis of immune cells
To assess the immune cell infiltration level of a single sample, we implemented the single-sample gene set enrichment analysis (ssGSEA) program through the R package “GSVA” to derive the relative enrichment levels of previously experimentally validated immune cell gene signatures (28). The prognostic value of immune cells for ACC was also determined using the “surv-cutpoint” function. Through Tumor Immune Estimation Resource (TIMER; http://timer.comp-genomics.org/), a web server to estimate tumor immune infiltrate populations (29), we determined the association between the expression levels of HELLS and the infiltration levels of immune cells.
Biological function enrichment analysis
To predict the biological function of the hub ferroptosis regulator, HELLS, correlation analysis was conducted between the expression of HELLS and other genes. Genes with |correlation coefficients| ≥0.4 were regarded as genes correlated with HELLS. These genes were input into the Database for Annotation, Visualization and Integrated Discovery (DAVID) database (http://david.ncifcrf.gov/summary.jsp) to conduct Gene Ontology (GO) and Kyoto Encyclopedia of Genes and Genomes (KEGG) analyses. The visualization of biological process terms of GO analysis and KEGG pathways was completed via imageGP (http://www.ehbio.com/ImageGP/index.php/home/index/upsetview.html).
Tissue specimens and immunohistochemistry
A total of 4 ACC specimens from the Urology Department of West China Hospital were retrospectively examined. The study was conducted in accordance with the Declaration of Helsinki (as revised in 2013). This experiment was approved by the Ethics Committee of West China Hospital (No. 20210276). Informed consent was not required as the specimens were collected retrospectively. Immunohistochemistry (IHC) staining was performed in formalin-fixed paraffin-embedded tumor tissue sections. The details for the primary antibodies are listed in Table S3. The sections were stained with standard procedures and examined under a microscope.
Statistical analysis
The IHC images of HELLS in normal adrenal glands were downloaded from the Human Protein Atlas (https://www.proteinatlas.org). The survival curves for prognostic analysis were generated via the Kaplan-Meier method, and log-rank tests were used to identify any significant of differences. Correlation coefficients were calculated by Spearman correlation analyses, and the Wilcoxon test was used to compare differences between 2 groups. All statistical analyses were 2-sided, with P<0.05 being considered statistically significant.
Results
Identification of differentially expressed ferroptosis regulators
The flowchart of the main steps in this study is shown in Figure 1A. To predict the ferroptosis regulators involved in ACC progression, differentially expressed genes were analyzed in groups 1 and 2 between normal and cancer tissues (Figure 1B). To improve the accuracy of screening, we determined the intersection of the 2 groups: 9 ferroptosis regulators showed higher expression in ACC tissues than in normal tissues, whereas 14 were both expressed at lower levels in ACC tissues than in normal tissues (Figure 1C). Among the regulators highly expressed in ACC, ABCC1, CDKN2A, ELAVL1, KEAP1, PRKAA1, and TP53 were ferroptosis driver genes, and HELLS, RB1, and SESN2 were ferroptosis suppressor genes. Among the regulators showing low expression in ACC, ACSF2, ALOX15B, ALOX5, MAPK3, SAT1, and SLC1A5 were ferroptosis driver genes; FTH1 and PTGS2 were ferroptosis marker genes; and ARNTL, ATF4, MT1G, SLC40A1, SRC, and STAT3 were ferroptosis suppressor genes. All these genes were considered as hub ferroptosis regulators and were further analyzed. These genes were considered as potentially playing vital roles in ACC by influencing the biological function of ferroptosis.
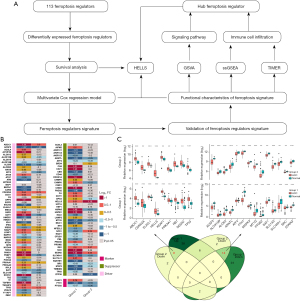
Identification of prognostic ferroptosis regulators
To further determine the value of ferroptosis regulators for predicting the prognosis of ACC, survival analysis was performed, which showed that 50 ferroptosis regulators were prognostic factors for ACC in TCGA dataset (Figure 2A, Table S4). Among these, there were 26 ferroptosis suppressors and 24 ferroptosis drivers. Combined with the above analysis, 13 ferroptosis regulators were differentially expressed and could be used as prognostic factors in ACC (Figure 2B), including 8 protective factors and 5 risk factors.
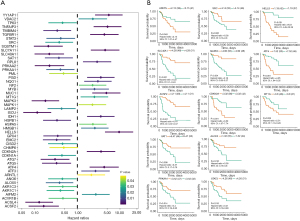
Construction of the ferroptosis regulator signature
Using the multivariate Cox regression model and the above 13 prognostic ferroptosis regulators showing differential expression, a 3-gene signature model was established containing ATF4, HELLS, and MT1G in TCGA dataset (Figure 3A, Table S5). The risk score for each patient was calculated using the following equation: risk score = (0.682258 × ATF4 expression) + (1.606768 × HELLS expression) + (0.186928 × MT1G expression). Survival analysis showed that overall survival was lower in the high-risk group than in the low-risk group (Figure 3B). In ROC analysis, the AUC values for the signature were 0.924, 0.906, and 0.866 for 3-, 5-, and 8-year survival, respectively (Figure 3C). Notably, the number of deaths increased and the number of surviving patients decreased with increasing risk scores. The expression value of each candidate gene in the signature associated with the risk score was visualized in a heatmap (Figure 3D-3F).
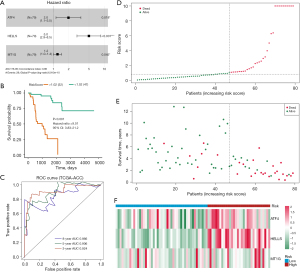
Validation of the ferroptosis regulator signature
A nomogram was constructed to predict the overall survival rates for 3 and 5 years (Figure 4A). In contrast to the ideal model, the calibration plot predicting overall survival (OS) outcomes for 3 and 5 years had relatively good results (Figure 4B). To validate the prognostic value of this ferroptosis regulator signature, 3 external independent datasets were employed, GSE76019, GSE76021, and GSE10927 + GSE19750. The risk score was calculated using the coefficient value obtained by multivariate Cox regression analysis. Patients showing low-risk scores had longer survival times than did those in the higher risk score group in the 3 validation datasets (Figure 4C-4E). The AUC values of ROC analysis for the prognostic signature in the 3 validation datasets are shown in Figure 4F-4H. The ferroptosis regulator signature accurately predicted the overall survival of patients with ACC.
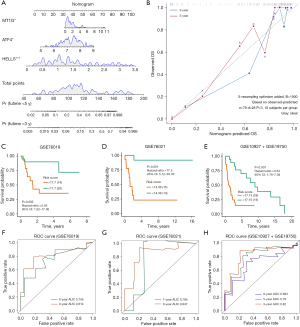
Functional characteristics of the ferroptosis regulator signature
To explore the underlying mechanisms of the ferroptosis regulator signature, GSVA was used to investigate pathway variations that may explain the prediction of survival probability of ACC using the signature. Indeed, we found that the bile acid metabolism, heme metabolism, protein secretion, and interferon-α response signaling pathways were highly enriched in the high-risk group, whereas the E2F targets, G2/M checkpoint, MYC targets v1, and MYC targets v2 signaling pathways were enriched in the low-risk group (Figure 5A). To verify the pathway aberrations in ACC, Spearman correlation analysis was conducted in 4 datasets between pathway GSVA scores and risk scores (Figure 5B). We found that the risk score was significantly positively correlated with E2F targets, G2/M checkpoint, MYC targets v1, MYC targets v2, DNA repair, and unfolded protein response signaling pathways and negatively correlated with bile acid metabolism and myogenesis signaling pathways. Correlation analysis was also performed between the abovementioned important pathways and the expression levels of the 3 signature genes, MT1G, HELLS, and ATF4 (Figure 5C). The expression of HELLS was the main factor correlated with aberrations in these pathways. We found that the expression of HELLS in ACC was highly positive correlated with the enrichment scores of the E2F targets, G2/M checkpoint, MYC targets, MTORC1 signaling, and DNA repair pathways, while it was negative correlated with bile acid metabolism and unfolded protein response pathways. In contrast, MT1G and ATF4 showed only a very weak correlation with enrichment scores of these pathways. Therefore, we inferred that HELLS might be a more crucial gene among these 3 genes in ACC.
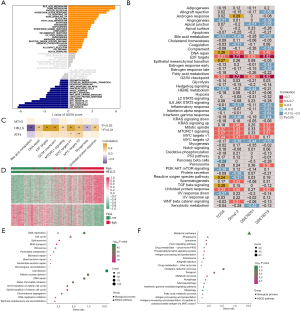
Identification of the hub ferroptosis regulator HELLS in ACC
Given that HELLS was the functional regulator with the most potential among the ferroptosis regulator signatures, we aimed to further predict the biological function of HELLS in ACC. From the above analysis, we screened HELLS as a hub ferroptosis regulator in ACC and a candidate novel biomarker for ACC. To further investigate the biological function of HELLS in ACC, we employed correlation analysis between HELLS and other genes in TCGA dataset. We found that 969 genes were significantly positively correlated with HELLS, with a correlation coefficient ≥0.4, while 732 genes were significantly negatively correlated with HELLS, with a correlation coefficient ≤−0.4 (Figure 5D and table available at https://cdn.amegroups.cn/static/public/gs-22-736-1.xlsx). Subsequently, we performed GO and KEGG analyses of these correlated genes to predict the biological function of HELLS. We found that the positively correlated genes were mainly enriched in DNA replication, cell cycle, cell division, and other cell proliferation–related pathways (Figure 5E). The negatively correlated genes were highly associated with metabolic pathways and immune-related pathways, such as interferon-γ–related pathways, antigen processing, and MHC II–related pathways (Figure 5F). Using the Human Protein Atlas, we confirmed that HELLS displayed low expression levels in normal adrenal gland tissues (Figure 6A). Moreover, we collected 4 ACC tissue samples verified with hematoxylin and eosin (HE) staining to test the expression level of HELLS, and the IHC results of our samples validated that HELLS expression was markedly more abundant in ACC tissues than in normal adrenal gland tissues (Figure 6B). Furthermore, correlation analysis was conducted between the expression of HELLS and GSVA enrichment scores (Figure 7A). We found that HELLS was significantly positively correlated with E2F targets, the G2/M checkpoint and MYC target pathways (Figure 7B), which are highly associated with cell proliferation and progression. Thus, we tested Ki-67 and proliferating cell nuclear antigen (PCNA) levels in 4 ACC samples. IHC results showed that Ki-67 and HELLS were highly positive in patients no. 1 and no. 2 and that the expression level of HELLS was also higher in patients no. 1 and no. 2 (Figure 7C). This result suggested that HELLS might be highly associated with cell proliferation activity in ACC. Interestingly, we verified that the expression level of HELLS gradually increased with the progression staging of the samples, and the expression of HELLS was significantly higher in advanced and metastatic samples (Figure 7D).
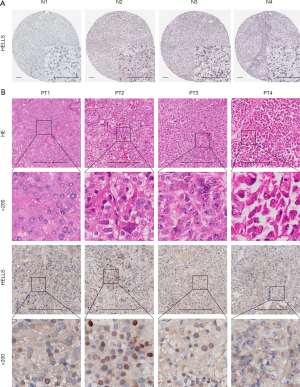
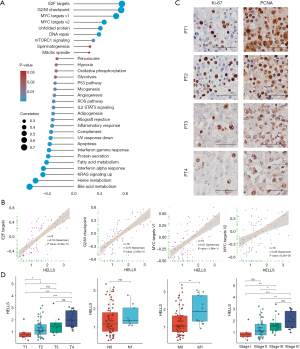
Relationship between immune cell infiltration level and HELLS
From the above analysis of pathway variation between the high- and low-risk groups, immune-related pathways were also changed, such as the inflammatory response and interferon-α response pathways. To verify whether the progression of ACC was correlated with tumor microenvironment variation, we conducted immune cell infiltration analysis using ssGSEA. First, we assessed 24 types of immune cell infiltration in each ACC sample. We performed survival analysis to determine which kinds of immune cells were prognosis-associated cell types. The results showed that 11 immune cells were protective factors, while only 2 immune cells were risk factors, including T helper 2 (Th2) cells and regulatory T (Treg) cells (Figure 8A,8B). To further investigate the infiltration levels of immune cells between the high- and low-risk groups, a heatmap (Figure 9A) and violin plot (Figure 9B) were used to display the abundance of immune cells. We found that the integral immune cell infiltration levels of the low-risk group were significantly higher than those of the high-risk group. In particular, the infiltration levels of B cells, cytotoxic cells, eosinophils, interstitial dendritic cells, macrophages, mast cells, neutrophils, natural killer CD56 bright cells, T follicular helper cells, and Th17 cells in the low-risk group were all higher than those in the high-risk group. In contrast, only the Th2 cell infiltration level was higher in the high-risk group than in the low-risk group. Correlation analysis showed that the hub ferroptosis regulator HELLS was significantly positively associated with the Th2 cell infiltration level and negatively associated with many protective immune cells (Figure 9C). To further verify the relationship between HELLS and immune cell infiltration, we used TIMER to analyze the association between the expression of HELLS and the infiltration levels of immune cells (Figure 10). We found that HELLS was significantly negatively associated with CD8+ T cells, CD4+ effector memory T cells, B cells, monocytes, myeloid dendritic cells, and mast cells, while HELLS was significantly positively associated with Th2 cells, cancer-associated fibroblast cells, and myeloid-derived suppressor cells (MDSCs). Unfortunately, IHC results showed that CD20, CD3, programmed cell death protein 1 (PD-1), and programmed death ligand 1 (PD-L1) were mostly negative in 4 ACC samples (Figure S1), indicating that these 4 samples might be “cold tumors”.
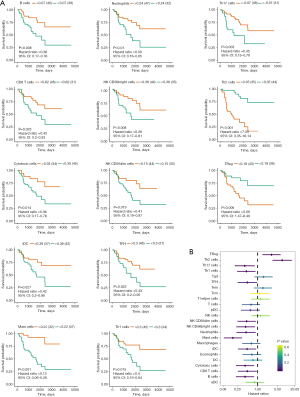
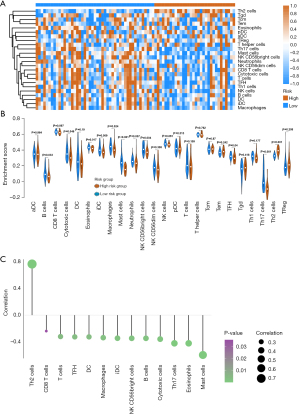
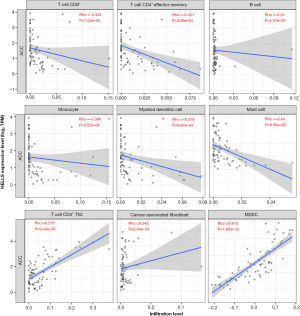
Discussion
ACC, as an endocrine-related malignant tumor, is characterized by a complex pathogenesis and poor prognosis, but has a low incidence (30). In recent years, ferroptosis induction has emerged as a promising therapeutic alternative to trigger cancer cell death, particularly for malignancies that are resistant to traditional treatments (31). Multiple studies based on the expression of ferroptosis regulators have led to the construction of prognostic signatures for predicting the overall survival of patients with many cancer subtypes, including hepatocellular carcinoma (32,33), glioma (34,35), and lung adenocarcinoma (36). However, few studies have focused on the function of ferroptosis regulators in ACC, nor has a related prognostic signature for ACC been constructed. In this study, based on public datasets in the, GTEx, TCGA, and GEO databases, we identified differentially expressed ferroptosis regulators between normal and tumor tissues. Combining these findings with survival information and using a multivariate Cox regression model, we identified prognostic ferroptosis regulators and constructed a 3-gene prognostic signature for ACC. Based on 3 external datasets, the prognostic prediction value of the ferroptosis regulator signature was verified. We also investigated the differences in underlying biological pathways and immune cell infiltration levels between the different risk groups of ACC.
Although ferroptosis genes were extracted from a specialized database, these regulators have been identified in multiple types of cancer, and their biological functions are not limited to ferroptosis. For example, CDKN2A was reported to induce ferroptosis in p53-independent tumor suppression (37); it has also been widely studied in ACC, but its function in driving ferroptosis has not been thoroughly evaluated. We identified ferroptosis regulators showing significantly differential expression between normal and ACC tumor tissues, 13 of which had prognostic value. Among them, only MAPK3 (38), CDKN2A, and STAT3 (39) have been studied for their prognostic value in ACC and may function in regulating ferroptosis in ACC to affect disease progression.
Three important ferroptosis regulators were used to construct a prognostic signature to predict the overall survival of patients with ACC. This signature accurately predicted the survival probability of patients with ACC in multiple independent datasets. Further pathway GSVA revealed that the risk score calculated by the signature was highly correlated with aberrations in multiple biological pathways. The high-risk group showed enrichment in cell progression- and metastasis-related pathways, such as the E2F, MYC, and G2/M checkpoint signaling pathways, which have been verified to be associated with poor prognosis and malignant tumor characteristics (40), explaining the poor prognosis of the high-risk group. Activation of these pathways may be associated with the suppression of ferroptosis; for instance, it has been reported that EGLN1/c-Myc induces lymphoid-specific helicase to inhibit ferroptosis by altering the expression of lipid metabolism-related genes (41). Activation of ferroptosis or suppression of the above pathways may be helpful for improving the prognosis of patients with high-risk scores. In contrast, metabolism-related pathways and myogenesis were highly enriched in the low-risk group; however, the relationship of these pathways with ACC remains unknown. Ferroptosis is a form of regulated cell death acting as the nexus between metabolism, redox biology, and human health (31).
Furthermore, immune cell infiltration analysis showed that multiple immune cells were protective factors for survival. Most of them, including cytotoxic cells (42), B cells (43), and dendritic cells (44), have been reported to be associated with better prognosis and response to immunotherapy in other types of tumors. Although only Th2 cells and Treg cells were found to be risk factors for ACC, both have been reported to be immune-suppressive cells (45-48). However, the variation in immune cell infiltration levels in ACC has not been systematically investigated. From our perspective, the integral immune cell infiltration level differences between the high- and low-risk groups were not coincident. The tumor microenvironment might be vital in the initiation and progression of ACC, and research into this area might represent a new chapter in the treatment of ACC.
Correlation analysis between the expression of 3 members of the signature and the GSVA score of the pathways revealed that HELLS may be the most important regulator, as it was associated with pathways that were significantly altered between the risk groups. HELLS, a chromatin remodeler, is involved in the progression of many types of tumors and regulates glioma stem cells via E2F3 and MYC (49), as well as chromatin remodeling and the epigenetic splicing of multiple tumor suppressors in hepatocellular carcinoma (50). Other studies have reported that HELLS is critical for retinoblastoma tumor initiation and progression (51), and it may serve as a poor prognostic biomarker contributing to the malignant phenotype in pancreatic cancer (52). Additionally, HELLS is highly expressed in and is a risk factor for ACC, having been positively correlated with multiple tumor progression–related pathways. Through IHC tests, we found that patients with ACC and higher expression of HELLS also had higher expressions of PCNA and Ki-67, meaning the expression level of HELLS might reflect the proliferative activity index of the lesions. Furthermore, the expression of HELLS showed a strong association with immune cell infiltration levels, especially that of Th2 cells, which is a prognostic risk factor for ACC. Moreover, the relative infiltration levels of mast cells, eosinophils, Th17 cells, cytotoxic cells, and B cells were negative correlated with the expression of HELLS in ACC. The results of TIMER also verified that HELLS was significantly associated with Th2 cells, MDSCs, and cancer-associated fibroblasts, which are widely known to be related to tumor progression and metastasis. Meanwhile, the infiltration levels of CD8+ T cells, CD4+ effector memory T cells, B cells, dendritic cells, and mast cells were all significantly negatively correlated with HELLS, inferring that HELLS might be associated with an immunosuppressive phenotype and a poor immunotherapy response rate. To date, the precise function of HELLS in the initiation of ACC or in the process of the tumor microenvironment remains unknown. Thus, targeting HELLS in ACC may be a reasonable treatment approach.
However, we verified our results using multiple independent datasets and further validated them with IHC. Additional basic studies of the ferroptosis regulators involved in ACC development and progression are needed to develop new therapies and identify diagnostic targets.
Conclusions
We comprehensively identified and systematically investigated the gene signatures of ferroptosis regulators in ACC. The differentially expressed ferroptosis regulators between normal adrenal gland and ACC tissues were identified, and their prognostic value was explored. We developed a 3-ferroptosis regulator prognostic signature that included HELLS, which may predict the biological and clinical progression of ACC. Moreover, the underlying mechanisms between different risk groups may be associated with metabolism; E2F, MYC, and G2/M checkpoint signaling pathways; and immune cell infiltration levels. Use of ferroptosis regulators is promising for ACC prognosis prediction. Given the high recurrence rate of ACC after surgery and extremely limited options for drug therapy, this study provides promising results concerning the possibility of using ferroptosis agonists to treat ACC. However, more clinical studies and experimental research are needed to verify this point.
Acknowledgments
Funding: This work was supported by National Natural Science Foundation of China (No. 81902723).
Footnote
Reporting Checklist: The authors have completed the TRIPOD reporting checklist. Available at https://gs.amegroups.com/article/view/10.21037/gs-22-736/rc
Data Sharing Statement: Available at https://gs.amegroups.com/article/view/10.21037/gs-22-736/dss
Peer Review File: Available at https://gs.amegroups.com/article/view/10.21037/gs-22-736/prf
Conflicts of Interest: All authors have completed the ICMJE uniform disclosure form (available at https://gs.amegroups.com/article/view/10.21037/gs-22-736/coif). The authors have no conflicts of interest to declare.
Ethical Statement: The authors are accountable for all aspects of the work in ensuring that questions related to the accuracy or integrity of any part of the work are appropriately investigated and resolved. The study was conducted in accordance with the Declaration of Helsinki (as revised in 2013). This experiment was approved by the Ethics Committee of West China Hospital (No. 20210276). Informed consent was not required as the specimens were collected retrospectively.
Open Access Statement: This is an Open Access article distributed in accordance with the Creative Commons Attribution-NonCommercial-NoDerivs 4.0 International License (CC BY-NC-ND 4.0), which permits the non-commercial replication and distribution of the article with the strict proviso that no changes or edits are made and the original work is properly cited (including links to both the formal publication through the relevant DOI and the license). See: https://creativecommons.org/licenses/by-nc-nd/4.0/.
References
- Kebebew E, Reiff E, Duh QY, et al. Extent of disease at presentation and outcome for adrenocortical carcinoma: have we made progress? World J Surg 2006;30:872-8. [Crossref] [PubMed]
- Allolio B, Fassnacht M. Clinical review: Adrenocortical carcinoma: clinical update. J Clin Endocrinol Metab 2006;91:2027-37. [Crossref] [PubMed]
- Gonzalez RJ, Tamm EP, Ng C, et al. Response to mitotane predicts outcome in patients with recurrent adrenal cortical carcinoma. Surgery 2007;142:867-75; discussion 867-75. [Crossref] [PubMed]
- Icard P, Chapuis Y, Andreassian B, et al. Adrenocortical carcinoma in surgically treated patients: a retrospective study on 156 cases by the French Association of Endocrine Surgery. Surgery 1992;112:972-9; discussion 979-80.
- Cazejust J, De Baère T, Auperin A, et al. Transcatheter arterial chemoembolization for liver metastases in patients with adrenocortical carcinoma. J Vasc Interv Radiol 2010;21:1527-32. [Crossref] [PubMed]
- Fu Y, Sun S, Bi J, et al. Construction of a risk signature for adrenocortical carcinoma using immune-related genes. Transl Androl Urol 2020;9:1920-30. [Crossref] [PubMed]
- Xu N, Ke ZB, Lin XD, et al. Identification of survival-associated alternative splicing events and signatures in adrenocortical carcinoma based on TCGA SpliceSeq data. Aging (Albany NY) 2020;12:4996-5009. [Crossref] [PubMed]
- Shen C, Liu J, Yang X, et al. Development and Validation of an m6A RNA Methylation Regulators-Based Signature for Predicting the Prognosis of Adrenocortical Carcinoma. Front Endocrinol (Lausanne) 2021;12:568397. [Crossref] [PubMed]
- Xu F, Guan Y, Ma Y, et al. Bioinformatic analyses and experimental validation of the role of m6A RNA methylation regulators in progression and prognosis of adrenocortical carcinoma. Aging (Albany NY) 2021;13:11919-41. [Crossref] [PubMed]
- Fu Y, Sun S, Bi J, et al. Expression patterns and prognostic value of m6A RNA methylation regulators in adrenocortical carcinoma. Medicine (Baltimore) 2021;100:e25031. [Crossref] [PubMed]
- Jin Y, Wang Z, He D, et al. Analysis of m6A-Related Signatures in the Tumor Immune Microenvironment and Identification of Clinical Prognostic Regulators in Adrenocortical Carcinoma. Front Immunol 2021;12:637933. [Crossref] [PubMed]
- Dixon SJ, Lemberg KM, Lamprecht MR, et al. Ferroptosis: an iron-dependent form of nonapoptotic cell death. Cell 2012;149:1060-72. [Crossref] [PubMed]
- Hassannia B, Vandenabeele P, Vanden Berghe T. Targeting Ferroptosis to Iron Out Cancer. Cancer Cell 2019;35:830-49. [Crossref] [PubMed]
- Belavgeni A, Bornstein SR, von Mässenhausen A, et al. Exquisite sensitivity of adrenocortical carcinomas to induction of ferroptosis. Proc Natl Acad Sci U S A 2019;116:22269-74. [Crossref] [PubMed]
- Weigand I, Schreiner J, Röhrig F, et al. Active steroid hormone synthesis renders adrenocortical cells highly susceptible to type II ferroptosis induction. Cell Death Dis 2020;11:192. [Crossref] [PubMed]
- Carneiro BA, Konda B, Costa RB, et al. Nivolumab in Metastatic Adrenocortical Carcinoma: Results of a Phase 2 Trial. J Clin Endocrinol Metab 2019;104:6193-200. [Crossref] [PubMed]
- Raj N, Zheng Y, Kelly V, et al. PD-1 Blockade in Advanced Adrenocortical Carcinoma. J Clin Oncol 2020;38:71-80. [Crossref] [PubMed]
- Le Tourneau C, Hoimes C, Zarwan C, et al. Avelumab in patients with previously treated metastatic adrenocortical carcinoma: phase 1b results from the JAVELIN solid tumor trial. J Immunother Cancer 2018;6:111. [Crossref] [PubMed]
- Habra MA, Stephen B, Campbell M, et al. Phase II clinical trial of pembrolizumab efficacy and safety in advanced adrenocortical carcinoma. J Immunother Cancer 2019;7:253. [Crossref] [PubMed]
- Berruti A, Fassnacht M, Haak H, et al. Prognostic role of overt hypercortisolism in completely operated patients with adrenocortical cancer. Eur Urol 2014;65:832-8. [Crossref] [PubMed]
- Fassnacht M, Kroiss M, Allolio B. Update in adrenocortical carcinoma. J Clin Endocrinol Metab 2013;98:4551-64. [Crossref] [PubMed]
- Vanbrabant T, Fassnacht M, Assie G, et al. Influence of hormonal functional status on survival in adrenocortical carcinoma: systematic review and meta-analysis. Eur J Endocrinol 2018;179:429-36. [Crossref] [PubMed]
- Coutinho AE, Chapman KE. The anti-inflammatory and immunosuppressive effects of glucocorticoids, recent developments and mechanistic insights. Mol Cell Endocrinol 2011;335:2-13. [Crossref] [PubMed]
- Wang W, Green M, Choi JE, et al. CD8(+) T cells regulate tumour ferroptosis during cancer immunotherapy. Nature 2019;569:270-4. [Crossref] [PubMed]
- Stockwell BR, Jiang X. A Physiological Function for Ferroptosis in Tumor Suppression by the Immune System. Cell Metab 2019;30:14-5. [Crossref] [PubMed]
- Liu N, Chen NY, Cui RX, et al. Prognostic value of a microRNA signature in nasopharyngeal carcinoma: a microRNA expression analysis. Lancet Oncol 2012;13:633-41. [Crossref] [PubMed]
- Liu N, Cui RX, Sun Y, et al. A four-miRNA signature identified from genome-wide serum miRNA profiling predicts survival in patients with nasopharyngeal carcinoma. Int J Cancer 2014;134:1359-68. [Crossref] [PubMed]
- Bindea G, Mlecnik B, Tosolini M, et al. Spatiotemporal dynamics of intratumoral immune cells reveal the immune landscape in human cancer. Immunity 2013;39:782-95. [Crossref] [PubMed]
- Li T, Fu J, Zeng Z, et al. TIMER2.0 for analysis of tumor-infiltrating immune cells. Nucleic Acids Res 2020;48:W509. [Crossref] [PubMed]
- Assié G, Letouzé E, Fassnacht M, et al. Integrated genomic characterization of adrenocortical carcinoma. Nat Genet 2014;46:607-12. [Crossref] [PubMed]
- Liang C, Zhang X, Yang M, et al. Recent Progress in Ferroptosis Inducers for Cancer Therapy. Adv Mater 2019;31:e1904197. [Crossref] [PubMed]
- Liang JY, Wang DS, Lin HC, et al. A Novel Ferroptosis-related Gene Signature for Overall Survival Prediction in Patients with Hepatocellular Carcinoma. Int J Biol Sci 2020;16:2430-41. [Crossref] [PubMed]
- Tang B, Zhu J, Li J, et al. The ferroptosis and iron-metabolism signature robustly predicts clinical diagnosis, prognosis and immune microenvironment for hepatocellular carcinoma. Cell Commun Signal 2020;18:174. [Crossref] [PubMed]
- Zhuo S, Chen Z, Yang Y, et al. Clinical and Biological Significances of a Ferroptosis-Related Gene Signature in Glioma. Front Oncol 2020;10:590861. [Crossref] [PubMed]
- Liu HJ, Hu HM, Li GZ, et al. Ferroptosis-Related Gene Signature Predicts Glioma Cell Death and Glioma Patient Progression. Front Cell Dev Biol 2020;8:538. [Crossref] [PubMed]
- Gao X, Tang M, Tian S, et al. A ferroptosis-related gene signature predicts overall survival in patients with lung adenocarcinoma. Future Oncol 2021;17:1533-44. [Crossref] [PubMed]
- Chen D, Tavana O, Chu B, et al. NRF2 Is a Major Target of ARF in p53-Independent Tumor Suppression. Mol Cell 2017;68:224-232.e4. [Crossref] [PubMed]
- Pereira SS, Monteiro MP, Costa MM, et al. MAPK/ERK pathway inhibition is a promising treatment target for adrenocortical tumors. J Cell Biochem 2019;120:894-906. [Crossref] [PubMed]
- Zhu Y, Xu Y, Chen D, et al. Expression of STAT3 and IGF2 in adrenocortical carcinoma and its relationship with angiogenesis. Clin Transl Oncol 2014;16:644-9. [Crossref] [PubMed]
- Liberzon A, Birger C, Thorvaldsdóttir H, et al. The Molecular Signatures Database (MSigDB) hallmark gene set collection. Cell Syst 2015;1:417-25. [Crossref] [PubMed]
- Jiang Y, Mao C, Yang R, et al. EGLN1/c-Myc Induced Lymphoid-Specific Helicase Inhibits Ferroptosis through Lipid Metabolic Gene Expression Changes. Theranostics 2017;7:3293-305. [Crossref] [PubMed]
- Hofland T, Eldering E, Kater AP, et al. Engaging Cytotoxic T and NK Cells for Immunotherapy in Chronic Lymphocytic Leukemia. Int J Mol Sci 2019;20:4315. [Crossref] [PubMed]
- Wang SS, Liu W, Ly D, et al. Tumor-infiltrating B cells: their role and application in anti-tumor immunity in lung cancer. Cell Mol Immunol 2019;16:6-18. [Crossref] [PubMed]
- Wculek SK, Cueto FJ, Mujal AM, et al. Dendritic cells in cancer immunology and immunotherapy. Nat Rev Immunol 2020;20:7-24. [Crossref] [PubMed]
- Kidd P. Th1/Th2 balance: the hypothesis, its limitations, and implications for health and disease. Altern Med Rev 2003;8:223-46.
- Abdel-Gadir A, Schneider L, Casini A, et al. Oral immunotherapy with omalizumab reverses the Th2 cell-like programme of regulatory T cells and restores their function. Clin Exp Allergy 2018;48:825-36. [Crossref] [PubMed]
- Tanaka A, Sakaguchi S. Targeting Treg cells in cancer immunotherapy. Eur J Immunol 2019;49:1140-6. [Crossref] [PubMed]
- Tanaka A, Sakaguchi S. Regulatory T cells in cancer immunotherapy. Cell Res 2017;27:109-18. [Crossref] [PubMed]
- Zhang G, Dong Z, Prager BC, et al. Chromatin remodeler HELLS maintains glioma stem cells through E2F3 and MYC. JCI Insight 2019;4:e126140. [Crossref] [PubMed]
- Law CT, Wei L, Tsang FH, et al. HELLS Regulates Chromatin Remodeling and Epigenetic Silencing of Multiple Tumor Suppressor Genes in Human Hepatocellular Carcinoma. Hepatology 2019;69:2013-30. [Crossref] [PubMed]
- Zocchi L, Mehta A, Wu SC, et al. Chromatin remodeling protein HELLS is critical for retinoblastoma tumor initiation and progression. Oncogenesis 2020;9:25. [Crossref] [PubMed]
- Wang FJ, Jing YH, Cheng CS, et al. HELLS serves as a poor prognostic biomarker and its downregulation reserves the malignant phenotype in pancreatic cancer. BMC Med Genomics 2021;14:189. [Crossref] [PubMed]