Abstract
Precise oil price forecasting is important and challenging. Long short term memory (LSTM) neural network is suitable to predict oil price, but the complexity of time series data limits the accuracy. Decomposition technique is an effective way to extract features from time series data. Variational mode decomposition (VMD) has been proved to be a good decomposition technique to generate discrete number of components. To reduce computation and improve prediction accuracy, relative entropy (RE) is used to criteria to recombine components. In this paper, based on VMD, RE and LSTM, we proposed a novel oil price forecasting model, named hybrid VMD-RE-LSTM model, considering the advantages of LSTM and VMD. The case results showed the forecasting ability of the hybrid VMD-RE-LSTM model is better than EEMD-LSTM model and LSTM for oil price forecasting.
Export citation and abstract BibTeX RIS
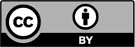
Content from this work may be used under the terms of the Creative Commons Attribution 3.0 licence. Any further distribution of this work must maintain attribution to the author(s) and the title of the work, journal citation and DOI.