Abstract
Visual Odometry (VO) is one of the important components of Visual SLAM system. Some impressive work on the end-to-end deep neural networks for 6-DoF VO has appeared. We propose two-part cascade network structure to learn depth from binocular image and to infer ego-motion from consecutive frames. We propose depth warp constraints to make the Network learning more geometrically information. A lot of experiments on KITTI data set show that our model is superior to previous unsupervised methods and has comparable results with the supervised method, verifying that such a depth warp constraints perform successfully in the unsupervised deep method which is an important complement to the geometric method.
Export citation and abstract BibTeX RIS
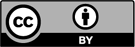
Content from this work may be used under the terms of the Creative Commons Attribution 3.0 licence. Any further distribution of this work must maintain attribution to the author(s) and the title of the work, journal citation and DOI.