Abstract
Oil palm is one of the important sources of vegetable oil that is mostly consumed by the Malaysian citizen. Because the demand of vegetable oil is high in Malaysia, the expansion of oil palm tree plantation has been increasing rapidly. Remote sensing application in monitoring and detecting oil palm trees has become a very useful tool to help minimize the human energy to monitor in large plantations. The aim of this study is to evaluate the accuracy of oil palm tree detection using deep learning and support vector machine (SVM) approaches using high-resolution remote sensing images. Deep learning is one of the base frameworks for oil palm tree detection using high-resolution remote sensing images. Deep learning also is a counting tool provided in newest technology software such as ArcGIS Pro, where the tools use the pattern recognition concept as a template in detecting objects in a high-resolution image. In machine learning, support vector machines are supervised learning models with associated machine learning algorithms that analyse data for classification analysis. Based on this study, 91% of the oil palm trees detected using deep learning approach gained higher accuracy than SVM classifier with 86%. The accuracy of oil palm tree detection using deep learning is higher than the accuracy of support vector machine classifier. Based on the findings, deep learning approach create better object interpretation than SVM. The oil palm tree detection using both classification approaches had also been displayed by using a spatial map distribution for easier analysed and observation.
Export citation and abstract BibTeX RIS
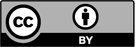
Content from this work may be used under the terms of the Creative Commons Attribution 3.0 licence. Any further distribution of this work must maintain attribution to the author(s) and the title of the work, journal citation and DOI.