Abstract
Crop water demand prediction is an important part of Precision agriculture. Due to the nonlinear relationship between input variables (weather data, soil moisture, and crop type) and output variables (crop water demand), it is difficult to accurately predict crop water demand. This article proposes a method for predicting crop water demand based on the EEMD Attention LSTM model. The model combines the ensemble empirical mode decomposition (EEMD), attention mechanism (Attention), and Long short-term memory (LSTM) neural networks to capture the changes in different scales of input variables. It selectively focuses on the relevant parts of the input sequence when making predictions. The performance of the EEMD attention LSTM model was evaluated on the historical crop water demand data set and compared with other prediction models such as RNN, LSTM, and EMD-LSTM. It shows that the model is superior to other methods in terms of Mean squared error and mean absolute error. When the relationship between input variables and output variables is nonlinear, the EEMD-attention-LSTM model is a powerful tool for crop water demand prediction.
Export citation and abstract BibTeX RIS
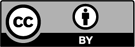
Content from this work may be used under the terms of the Creative Commons Attribution 3.0 licence. Any further distribution of this work must maintain attribution to the author(s) and the title of the work, journal citation and DOI.