Abstract
At present, as the knowledge graph continues to increase, problems such as incomplete information in the graph also emerge, mainly reflected in the lack of links between entities [1], and the current commonly solution is to learn their representations that can represent more semantic information for the entities and relations in the graph. This article focuses on the temporal knowledge graph (TKG), consistent with the goal in the static knowledge graph, this paper tries to propose a better representation learning method to solve the link prediction problem. According to the time information in the temporal knowledge graph, the paper constructs a bag of words about time, uses the bag of words model to decompose the time in the temporal knowledge graph into sequences, and learns its semantic information together with relations and entities. The embeddings obtained in this way can learn more semantic information. In addition, proving the effectiveness of the model through experiments on the currently commonly used temporal knowledge graphs.
Export citation and abstract BibTeX RIS
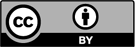
Content from this work may be used under the terms of the Creative Commons Attribution 3.0 licence. Any further distribution of this work must maintain attribution to the author(s) and the title of the work, journal citation and DOI.