Abstract
Purpose: This paper presents a CNN-RNN neural network approach towards electromyography. Here the neural network is employed to identify different gestures from the reading of signals from human's hand muscles. The identified gestures are then utilized to control a drone. Methodology: Implementation is made by using Myo, an 8-channel Electromyography (EMG) data acquisition device. 14000 datasets of 9 different gestures are collected and used to train the CNN-RNN models. The trained models are then tested on a drone using dronekit to translate the gestures to drone commands and send them from Python to the drone. Results: The results show that a CNN-RNN approach is very effective in identifying gestures from raw muscle data, resulting in an average of 96.60% positive identification for each gesture. The identified gesture shows an effective drone control from the simulated drone. Applications/Originality/Value: The high rate of positive identification opens the possibility to use a wearable device that is able to give the current state of the hand's muscle tensions, translating them into a specific command to control devices or machines. With a rich information and real-time human control, it is expected that humans will experience a more intuitive approach towards devices or machines control.
Export citation and abstract BibTeX RIS
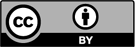
Content from this work may be used under the terms of the Creative Commons Attribution 3.0 licence. Any further distribution of this work must maintain attribution to the author(s) and the title of the work, journal citation and DOI.