Abstract
Monocular depth estimation is a classical computer vision task. At present, most CNN methods cannot effectively combine high-level and low-level features, leading to the loss of details and blurring of boundaries. To solve the problem, we propose a Multi-Scale Context Enhanced Network (MCEN) to learn more abundant context and expand its receptive field for high-accuracy estimation. Our method employs CRE-HRNet (Context and Receptive Enhanced High-Resolution Network) with four branches ranging from low-dimension to high-dimension features to obtain richer contextual information and extract multi-scale features. It then uses RM (Refinement Module) adopting the residual dilated convolution to retains detailed information and improve the receptive field. Finally, non-local block enables our network to capture the longdistance context through its special non-local operation. Experiments with the NYU Depth V2 dataset show its outstanding performance.
Export citation and abstract BibTeX RIS
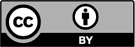
Content from this work may be used under the terms of the Creative Commons Attribution 3.0 licence. Any further distribution of this work must maintain attribution to the author(s) and the title of the work, journal citation and DOI.