Abstract
Recent development in space-time data forecasting includes a hybrid model. In this study, we propose a hybrid spatio-temporal model by combining Generalized Space-Time Autoregressive with exogenous variable and Recurrent Neural Network (GSTARX-RNN) for space-time data forecasting with calendar variation effect. The GSTARX model as a linear model is used to modeling space-time data with exogenous variables while the RNN model as nonlinear model is used to modeling the nonlinear patterns of the data. In particular, we employ two variants of RNNs, i.e. Elman RNN and Jordan RNN. We apply our methods on the simulation study. The results show that the proposed methods yielded more accurate forecast especially in the simulated data containing nonlinear patterns. Moreover, the GSTARX-Elman RNN as a more complex model tends to give more accurate forecast than the GSTARX-Jordan RNN.
Export citation and abstract BibTeX RIS
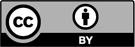
Content from this work may be used under the terms of the Creative Commons Attribution 3.0 licence. Any further distribution of this work must maintain attribution to the author(s) and the title of the work, journal citation and DOI.