Abstract
Tianshan Mountains are the headwater regions for the central Asia rivers, providing water resources for ecological protection and economic development in semiarid regions. Due to scarce observations, the hydroclimatic characteristics of the Tianshan Mountains Precipitation (TMP) measured over highland (>1500 m) regions remain to be revealed. Here, we show the TMP belongs to a monsoon-like climate regime, with a distinct annual range and a high ratio of summer-to-yearly rainfall, and exhibits six abrupt changes, dividing the annual cycle into six precipitation sub-seasons. Over the past 60 years, the yearly TMP has significantly increased by 17.3%, with a dramatic increase in winter (135.7%). The TMP displays a significant 40-day climatological intra-seasonal oscillation (CISO) in summer. The TMP CISO’s wet phase results from the confrontation of the eastward propagating mid-tropospheric Balkhash Lake Low and the southward migrating Mongolian High. The sudden changes in the two climatological circulation systems trigger TMP’s changes, shaping the 40-day CISO. Emerging scientific issues are also discussed.
Similar content being viewed by others
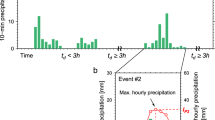
Introduction
The term “Tianshan Mountains” means “Heavenly Mountains” in Chinese, the largest mountain range in Central Asia (Fig. 1a), stretching about 2500 km and dividing Xinjiang, a province in northwest China, into the southern and northern basins (Fig. 1b). The precipitation over the Tianshan Mountains region is considerably larger than in other areas of Xinjiang (Fig. 1c, d). The annual precipitation is less than 100 mm over Southern Xinjiang but relatively wet (100–500 mm) over Northern Xinjiang1,2. Tianshan Mountains, located in central Eurasia, is the headwater region for rivers in Xinjiang and several Central Asian countries, including Kazakhstan, Kyrgyzstan, and Uzbekistan3,4 (Fig. 1a). Thus, the Tianshan Mountains are considered the Central Asian water tower.
a Topographic map of Central Asia with Xinjiang outlined in green lines. b Locations of 106 rain gauge observations. The red/purple lines denote the 1500 m contours. The red lines within the black box outline the Tianshan Mountains area studied here. The seven blue triangles indicate stations in the Tianshan Mountains above 1500 m. In comparison, the 11 green triangles refer to the stations in the Tianshan Mountains “foothills”. c, d show the spatial patterns of the annual mean precipitation (mm/day) derived from CN05.1 and ERA5 data, respectively.
This study focuses only on the Xinjiang Tianshan Mountains range due to data availability. Here, the Tianshan Mountains region is delineated by the area with an elevation over 1500 m in the box region shown in Fig. 1b. This work defines the precipitation averaged over the Xinjiang Tianshan Mountains region as Tianshan Mountains precipitation (TMP). Xinjiang is traditionally considered a dry and rainless region5,6,7, belonging to a westerlies-dominated dry climatic regime8,9. Heterogeneous terrain of mountains and basins intersect, creating a unique “mountain-oasis-desert” ecosystem pattern10,11. TMP and meltwater from glaciers determine the local hydrological changes12,13,14 and play a crucial role in agriculture, pasture management, disaster mitigation, ecosystem protection, and economic development in semiarid regions.
The shifts in Xinjiang and Tianshan Mountains hydroclimate have attracted significant attention15,16,17,18. Annual total precipitation in Xinjiang has exhibited an upward trend over the past decades, with the trend magnitude peaking at the Tianshan Mountains and decreasing from northwest to southeast19. Meanwhile, precipitation extremes become increasingly severe and frequent along the Tianshan Mountains12. However, previous findings on the seasonal precipitation trends are divergent. Some suggested the dry season is getting drier while the wet season is becoming wetter20. Others reported all four seasons exhibiting significant increases in precipitation, and the trend in the rainy season is more obvious21. The discrepancies in the trend estimations could be due to data quality and the different periods examined. Also, resolving the above differences in the precipitation trend22,23,24 requires a better understanding of the climatological precipitation seasonality and spatial heterogeneity due to topographic effects. An adequate classification of seasonal stages may offer a clearer picture of seasonal precipitation trends.
Although Xinjiang was classified as a westerly monsoon regime, no concrete evidence on the annual alternation of winter and summer monsoons has been provided25. Xinjiang was also considered a “westerlies-dominated climatic regime (WDCR)9. The WDCR concept describes the pattern of precipitation variations between westerlies-dominated arid Central Asia and mid-latitude monsoon-dominated Asia across time scales ranging from decadal to multi-millennial time scales8. The domain of WDCR stretches from the Caspian Sea in the west to the western Hexi Corridor in the east, with the northern and southern limits coinciding with the boundaries of arid Central Asia9. Tianshan Mountains is in the central WDCR region between arid Central Asia and dry mid-latitude monsoon Asia. Arid Central Asia has a peak wet season from February to April, while the midlatitude monsoon Asia shows a peak wet season in mid-summer26,27. A better understanding of the TMP climatology and the circulation systems controlling the annual precipitation variation is the prerequisite for understanding its climate variability and future change.
Owing to scarce observations in high mountain areas, fundamental characteristics of the TMP climatology still need to be revealed. While the Tianshan Mountains were traditionally regarded as a region outside the East Asian monsoon domain28, what climatic regime does the Tianshan Mountains climate belong to? How much do we know about the precipitation intensity and uncertainty over the Tianshan Mountains? What are the features of the TMP climatology compared with other monsoon and arid regions? We are curious whether sudden changes and climatological intra-seasonal oscillation (CISO)29,30,31,32 exist in the annual cycle of the TMP, which has yet to be unraveled. The study of CISO can be significant in understanding climatological variation and climate variability, subseasonal-to-seasonal prediction, and future projections.
This study elaborates on the features of the Tianshan Mountains climate regime from a hydrometeorological perspective. We first validate the ability of the ERA5 and CN05.1 to capture the TMP and explore the sub-season-dependent trends over the past 60 years. Then, we explore the annual precipitation cycle, focusing on its climate regime, sudden changes, rainy season characteristics, and the precipitation CISO’s features and origin. The last section presents a summary and discussion.
Results
How much does it precipitate over the Tianshan Mountains?
The short answer is we do not precisely know because the rain gauge stations are scarce over the Tianshan mountains. For clarity, we define Tianshan Mountains as the highland regions above 1500 m and their surrounding areas with heights between 1500 m and 1000 m as foothills. There are only seven rain gauge observations in the Tianshan Mountains (blue triangles in Fig. 1b) and 11 stations in their foothills (green triangles in Fig. 1b). To validate the ERA5 and CN05.1 data against the rain gauge observations, we selected the grid cells in ERA5 and CN05.1 that cover the rain gauge stations. The climatological pentad mean precipitation was made for 30 years (1987–2016).
Figure 2a shows that the annual variations of CN05.1 and ERA5 are highly consistent with the rain gauge observations. However, significant discrepancies exist in rainfall intensity. The CN05.1 data (1.18 mm/day or 431 mm/year) underestimated the rain gauge observation (1.25 mm/day or 455 mm/year), whereas the ERA5 data (1.72 mm/day, 629 mm/year) overestimated the precipitation, especially during fall. However, given the scarce and uneven distribution of the rain gauges over the Tianshan Mountains highland, it still needs to be determined whether ERA5 overestimates and CN05.1 underestimates the precipitation over the entire Tianshan Mountains.
a Comparison of observed (the mean of seven rain gauge stations, blue) and the corresponding ERA5 (green) and CN05.1 (purple) precipitation measured at the grids nearest to the seven stations. b Comparison of the observed precipitation at the Tianshan Mountains (the mean of the seven stations above 1500 m, blue) and Tianshan Mountains foothill (the mean of 11 stations with altitudes between 1000 m and 1500 m, pink).
Notably, the Tianshan Mountains’ precipitation almost triples their foothills’ precipitation (Fig. 2b). That means the precipitation varies remarkably with altitudes. The heights of the Tianshan Mountains range from 1500 m to over 7000 m. These topographic height fluctuations add additional difficulties in estimating the total amount of TMP.
The season-dependent Tianshan Mountains precipitation trends over the past six decades
We then explored the observed linear trends from 1961 to 2019 based on the rain gauge data. Figure 3 shows that the annual mean precipitation in the Tianshan Mountains has increased by 17.3% (p < 0.01) over the past 60 years. However, the trends are season-dependent: 7.7% (p > 0.1) in the wet season (May through September, MJJAS) and 135.7% (p < 0.01) in the dry winter (November through March, NDJFM). The salient increase in dry winter snowfall implies a substantial increasing trend in the winter snowpack. The increasing wet season rainfall is statistically insignificant. Thus, the Tianshan Mountains’ climate is getting wetter, and the dry season is becoming remarkably wetter.
a, b are precipitation time series (solid line) and the corresponding linear trend (dashed line) for the annual (black), summer (MJJAS, red), and winter (NDJFM, blue) over the Tianshan Mountains (a) and Tianshan Mountains foothill (b), respectively. The Tianshan Mountains precipitation is measured by six rain gauge stations above 1500 m. The foothill precipitation is measured by 11 rain gauge stations with elevations between 1000 m to 1500 m. The percentage values on the right side of the panels indicate the percentage changes (relative to 1961) of the precipitation trends from 1961 to 2019.
Notably, the precipitation in the foothills of the Tianshan Mountains shows a more prominent trend than the TMP, especially during the wet season. The annual total precipitation in the Tianshan foothills has significantly increased by 42.2%, with remarkably enhanced precipitation both in the wet season (29.3%, p < 0.01) and dry season (109.4%, p < 0.01). The results here differ from previous studies that suggested the dry season is getting drier while the wet season is becoming wetter20 or the trend in the rainy season is more obvious21.
Tianshan Mountains belongs to a monsoon-like precipitation regime
Our interest is to study the Tianshan Mountains’ average precipitation. Tianshan Mountains occupy approximately 14.4% of the Xinjiang province, roughly 23,4300 square kilometers. For clarity, we define the precipitation averaged over the entire Xinjiang Tianshan Mountains region (Fig. 1b) as “Tianshan Mountains Precipitation” or TMP. To estimate TMP, we use ERA5 precipitation data because the seven rain gauge data might not fully represent the mean precipitation over the entire Tianshan Mountains region. An additional advantage to using ERA5 data is their dynamical consistency with the circulation data used later.
To understand to what extent the seven-station average can represent the TMP, we compare the ERA5 TMP with the ERA5 seven-station precipitation. As shown in Fig. 4a, the ERA5 TMP generally coincides with the ERA5 seven-station precipitation in the dry season. However, from June to September (Pentad 31 to Pentad 55), the TMP is higher than the seven-station mean precipitations. As such, the June-July-August mean TMP exceeds the corresponding seven-station precipitation by about 11%, suggesting that the seven-station observation may underestimate the TMP.
a Climatological pentad-mean precipitation and the corresponding smoothed annual cycle (thin solid lines). The green line represents the ERA5 precipitation averaged over the entire Tianshan Mountains area (above 1500 m). The blue lines denote the ERA5 precipitation averaged over the vicinity of the seven rain gauge stations above 1500 m. The black dashed lines indicate the baselines of 0.7 mm/day, 2.3 mm/day, and 3.3 mm/day, respectively. b Detection of the sudden change pentads (SCPs) in the TMP. The t value is computed by using a two-tailed t test. The red dashed lines represent the significance at the 99% confidence level. Details are in Method. In (a) and (b), the red dots denote the diagnosed significant SCPs, and the cyan vertical lines indicate the six SCPs dividing the six substages.
The annual cycle of the ERA5 TMP comprises a contrasting summer rainy season and a dry winter, with a total amount of annual rainfall of 673 mm (Fig. 4a). On the seasonal time scale, the total amount of precipitation from May through September is 496 mm, while precipitation from November through March is 88 mm only. Thus, the annual range of rainfall, defined as the mean MJJAS precipitation minus that in NDJFM28, is 407 mm. The TMP’s summer-to-yearly rainfall ratio is thus over 70%, as high as in the typical monsoon region over India and northern China. Generally speaking, a monsoonal precipitation regime should satisfy two criteria: (1) The annual range of precipitation exceeds 300 mm, and (2) the local summer precipitation exceeds 55% of the annual rainfall33. The TMP meets these criteria. So is the precipitation averaged over the Tianshan Mountains seven rain gauge stations. Therefore, we propose that the Tianshan Mountains belong to the monsoon-like regime from a hydroclimate perspective.
The TMP displays different features than that in northern China (30–45°N, 105–120°E). First, the TMP peaks in pentad 40 (hereafter P40) around mid-July (Fig. 4a), which is earlier than the counterparts (mid-August) in Northern China34. Secondly, in northern China, the monsoon precipitation features a concentrated, short, rainy summer but a prolonged dry winter (a “wet-dry asymmetry” in duration). However, the TMP tends to be more “symmetric” in terms of wet-dry season duration (Fig. 4a): The precipitation rate exceeds the annual average (1.84 mm/day) from P24 (April 26–30) to P53 (September 18–22), lasting 30 pentads, slightly shorter than the dry winter below the annual average. Thirdly, the TMP shows a “spring-fall asymmetry”– the April–May precipitation rate is 2.20 mm/day while the September–October mean precipitation rate is only 1.72 mm/day. The relatively wet spring could result from some weather systems (e.g., mid-tropospheric troughs) coming from the west35, as adjacent Central Asia has a wet season peaking in March and April (figure not shown). Additionally, stronger moisture recycling from the enhanced springtime evapotranspiration (due to snowmelt and rising temperature) compared to the fall may also explain the origin of the TMP’s spring-fall asymmetry35.
Abrupt changes and sub-seasonal stages in the Tianshan Mountains hydroclimate
Precipitation is the hydrological cycle’s central component and the hydroclimate’s archetypal representation. Defining the rainy season of the Tianshan Mountains with an absolute value of the precipitation rate36 is difficult because of its relatively low precipitation intensity. However, we found the annual variation of TMP exhibits prominent abrupt changes. Using the Student’s t test, we identified ten SCPs significant at the 99% confidence level: P8, P14, P19, P24, P28, P33, P46, P51, P58, and P67 (Fig. 4b). We propose to use six sudden change pentads (SCPs, see “Methods”) to demarcate TMP’s seasonal progression (Fig. 4b and Table 1).
Precipitation rate higher than 3.3 mm/day occurs between a pair of SCPs, P33 and P46 (Fig. 4b). We consider the period from P33 to P45 (June 10th to August 13th) as the summer rain stage (Table 1). The second pair of SCPs, P24 and P51, roughly corresponds to a 2.3 mm/day precipitation rate, defining two sub-seasonal stages adjacent to the summer rain. One is pre-summer rain from P24 to P32, and the other is post-summer rain from P46 to P51. The third pair of SCPs, P67 and P14, define dry winter, corresponding to a precipitation rate of less than 0.7 mm/day. The remaining periods, P15-P23 and P52-P66, are the spring and fall transitional substages, respectively. The average precipitation intensity and the ratio to annual mean precipitation amount for each substage are listed in Table 1.
The SCPs over the Tianshan Mountains tend to echo the sudden changes in the seasonal progression of the East Asian monsoon. The surge of TMP at P24 (end of April) concurs with the increased rainfall in central southern China37. The rapidly increasing TMP at P28 corresponds to the climatological onset of the South China Sea summer monsoon38. The sudden onset of TMP summer rain at P33 is nearly concurrent with the Meiyu onset at P34 over the Yangtze River basin26. The period from P28 to P33 is the so-called pre-Meiyu stage39, also known as the Dragon Boat rain in southern China40, which falls within the TMP’s pre-summer rain stage. The sudden change at P46 (mid-August) roughly corresponds to the summit of the northern China rainfall. The sudden change at P51 (mid-September) nearly concurs with western China’s autumn rain41. These linkages suggest a synergistic change of monsoon stages in both the Tianshan Mountains and East Asia, which bespeaks a common origin from the abrupt adjustment of the large-scale circulation.
TMP shows a 40-day CISO coupled with Eurasian circulation systems
The TMP seasonal progression is not a smoothed annual cycle (Fig. 4). The smoothed annual cycle in response to solar forcing can be represented by the first four Fourier harmonics of the daily climatology42. Figure 5a shows the climatological daily departure from the smoothed annual cycle. The pentad-mean departures derived from the ERA5 and station data are coherent with their correlation coefficient of 0.69 after a 5-day running mean (with an effective degree of freedom of 72). The high correlation justifies the use of ERA5 data in the following diagnoses.
a The 5-day running mean climatological daily TMP departure from the base annual cycle derived from ERA5 and the seven Tianshan Mountains stations. b The panel on the left shows the wavelet analysis of the climatological daily TMP anomaly derived from ERA5. The areas with a white slant are significant at the 95% confidence level, and the cross-hatched regions on either end indicate the “cone of influence,” where edge effects become important. The panel on the right shows the global wavelet spectrum.
The resultant daily departure displays an oscillatory behavior with a considerable amplitude from April to September. The wavelet analysis reveals that the departure time series contains two bands of intraseasonal signals with high variability at 8–16 days and about 40 days, in addition to high-frequency (<7 days) noises (Fig. 5b). The 8–16 days signal prevails in April, September, and October. In contrast, the 40-day signal remains prominent from May through August. Here we refer to the 6th to 13th harmonics (30–60 days) as CISO42. Thus, the TMP CISO features a 40-day oscillation from May through August.
To depict the coherent 40-day CISO structure and propagation in precipitation and circulation anomalies, we computed the 30–60 day filtered MJJAS mean daily precipitation, the vertically integrated (1000 hPa to 100 hPa) water vapor transport, and 500-hPa geopotential height. Figure 6 presents their lead-lag regression maps with reference to the 40-day TMP CISO index from -15 day to +10 day from May through September. Day 0 means the peak rain day in TMP. An anomalous 500-hPa low-pressure system in Europe extends to the Ural Mountains (60°N, 60°E) on Day -20 (Figure omitted). From Day −15 to Day −5, the Low moves eastward slowly with a trough between the Caspian Sea and the Balkhash Lake. For convenience, we coin it “Balkhash Lake Low”. The associated rainy region is located southeast of the Low. After Day −5, the Low decays and becomes a deep trough near Balkhash Lake on Day 0. The movement of the Balkhash Lake Low appears to be blocked by a high-pressure system, which originates from western Siberia and moves to central Siberia on Day −15. From Day −15 to Day 0, the High migrates southward slowly with a prominent ridge extending from Mongolia southwestward to northern Xinjiang. For this reason, we name it “Mongolian High”. Low-level easterlies prevail south of the Mongolian High. The water vapor was continuously transported from East Asia toward Xinjiang from Day −10 to Day +5 shown by the vertically integrated vapor transport vectors. The TMP increased from Day −5 and peaked on Day 0. The CISO decaying phase is almost a mirror image of the developing phase.
Shown are lead-lag regression maps of the precipitation (mm/day, shading), 500-hPa geopotential height (gpm, contours with solid (dashed) red (blue) denting the positive (negative) anomalies), and the vertically integrated (1000 hPa to 100 hPa) water vapor transport (kg m−1 s−1, vector) anomalies with reference to the TMP CISO index from −15 day to +10 day after 30–60 day filtering. Data are derived from the ERA5 dataset during summer (MJJAS). Black lines mark the 1500 m elevation contours of the Xinjiang Tianshan Mountains. The black rectangles represent the defining domains for the Mongolia High and the Balkhash Lake Low indices. The letters “H” and “L” stand for the center of the high and low pressures, respectively.
The two large-scale mid-tropospheric circulation systems, the Mongolian High and Balkhash Lake Low dominate the evolution of the Tianshan Mountains CISO. To disclose further the links between TMP and the large-scale circulation systems, we use the 500-hPa geopotential height averaged over (90–120°E, 45–65°N) to define the Mongolian High CISO index and the 500-hPa geopotential height averaged over (55–75°E, 45–65°N) to define the Balkhash Lake low CISO index (Fig. 7). We find a significant 40-day peak in the power spectra of the Mongolian High and Balkhash Lake Low CISO indices (Fig. 7b, c). These spectral peaks were derived from a daily climatological time series during MJJAS without filtering. The Mongolian High CISO index exhibits a single significant quasi-40-day periodicity without high-frequency variance, suggesting that the high-frequency variability of TMP may be related to local variabilities. Likewise, the Balkhash Lake Low CISO index shows a 40-day peak significant at the 90% confidence level and a more significant quasi-20-day peak.
a Standardized time series of three CISO indices represented by the sum of the 6th to 13th annual harmonics from April 1st to November 1st. The purple dots represent the statistically significant pentad in the TMP CISO at a 95% confidence level. b, c show the power spectrum of the Mongolian High and Balkhash Lake Low CISO indices.
The TMP, Mongolian High, and Balkhash Lake Low CISO indices tend to vary coherently in general, except that phase differences exist between the two circulation indices and the TMP CISO (Fig. 7a). The Mongolian High CISO has a relatively large amplitude in summer, generally coinciding with the TMP CISO from May to July, but leading the TMP CISO by about ten days in August to October. The Balkhash Lake Low CISO has a relatively small amplitude in May and October and leads TMP by about ten days from April to July and covaries with it from mid-August to the end of September.
The large-scale circulation origin of the TMP 40-day CISO
CISO has been interpreted as a result of the phase-locking of transient intra-seasonal oscillation (ISO) to the annual cycle42. However, this appears not the case for the TMP CISO, as we could only find significant transitory 40-day ISO in less than one-third of the 30 years (Figure omitted). Thus, we propose an alternative explanation. We hypothesize that the TMP CISO may arise from the sudden changes in the annual cycles of the large-scale circulation systems. To test the hypothesis, we investigated the relationships among the TMP CISO peaks (or valleys), the TMP SCPs, and the Mongolian High and Balkhash Lake Low indices (Table 2). The SCPs in TMP tend to lead one to two pentads or concur with its CISO peaks or valleys. Additionally, the TMP SCPs generally occur before the corresponding CISO peaks during the rising precipitation season while before the CISO valleys during the precipitation decay season, suggesting that the TMP CISO arises from the abrupt changes in its annual variation. The question is: How are the abrupt changes in TMP created?
We find that the SCPs in the Mongolian High occur systematically ahead of the TMP sudden changes by one to two pentads from spring to autumn (Table 2). The sudden changes in the Balkhash Lake Low index also tend to be ahead of the TMP CISO’s peaks and valleys by two to three pentads. The Balkhash Lake Low closely interacts with the westerly jet. We find that the latitudinal location of the westerly jet axis at 200 hPa averaged over 50–80°E (slightly upstream of the Tianshan Mountains) exhibits a sudden jump, which occurs nearly the same SCPs as the Balkhash Lake Low index (figure not shown). Therefore, we argue that the abrupt seasonal changes in the large-scale Eurasian circulations may trigger the sudden changes in the TMP, which in turn causes the significant 40-day TMP CISO.
Discussion
Our significant findings concerning the characteristic TMP climatology are summarized as follows.
-
Over the past 60 years (1961–2019), the annual total TMP has significantly increased by 17.3% with season-dependence: 7.7% in the wet season and 135.7% in the dry winter, indicating that the dry season has become remarkably wetter. In contrast, precipitation in the Tianshan Mountains foothill exhibits a more robust increasing trend in the annual (42.2% increase), primarily due to a more robust wet season rainfall increase.
-
The TMP within Xinjiang belongs to a monsoon-like regime with a significant annual range of 407 mm and a high ratio (over 70%) of summer to yearly rainfall.
-
The TMP annual cycle displays significant sudden changes that can divide the annual variation into six subseasonal stages. The wet season includes pre-summer rain from P24 to P32 (April 26 to June 9) summer rain from P33 to P45 (June 10 to August 13), and post-summer rain from P46 to P51 (August 14 to September 12). The dry season includes winter from P67 to P14 (November 27 to March 11) and the spring and fall transition substages.
-
The TMP displays a significant 40-day CISO from May through August, coupled with the mid-tropospheric Mongolian High and Balkhash Lake Low. The TMP CISO’s peak wet phase results from the confrontation of the eastward propagating Balkhash Lake low and the southward propagating Mongolian high. The latter is crucial in transporting water vapor from East Asia to the Tianshan Mountains region.
-
The sudden changes in the two climatological circulation systems triggered the TMP’s sudden changes, leading to the 40-day CISO.
Our finding has significant implications for seasonal prediction and future projection. The results here lay a foundation for predicting the onset dates and the amounts of precipitation in each subseasonal stage. However, such predictions require a deep understanding of the factors that control the interannual variability of the precipitation subseasonal stages. Our results also paved the way to investigate future changes in the precipitation trends, hydroclimate singularities, and sub-seasonality at the Central Asian water tower, a keen concern of the Central Asian countries. The monsoon-like regime in the Tianshan Mountains, as revealed here, could be understood as follows. During summer, the surface sensible heating in the sloped terrain could produce thermally-driven upslope flows, enhancing orographic precipitation and mesoscale convective system, much like in the daytime situation in the diurnal cycle43,44. The westerly jet over the Tianshan Mountains may further boost orographic disturbance45.
The TMP CISO is delineated based on the climatology from 1987 to 2016. We have tested each 30-year climatology from 1961 through 2020 and found the 40-day peak varies over time, with an enhancement after the 1980s (figure not shown). Using a 227-year daily precipitation record gathered in Seoul, South Korea, Wang et al. 46 demonstrated that the rainy season characteristics (the dates of onset, retreat, and summit) show significant centennial variations, indicating that the monsoon singularities detected by using a 30-year climatology may change with time. This is no surprise because the climatology defined by a 30-year mean state generally changes with time due to changes in solar irradiance cycles and other external forcings and internal feedbacks within the coupled climate system, such as multidecadal variability in the Atlantic and Pacific oceans. Alternatively, one may delineate the interannual and interdecadal variabilities by applying Fourier analysis on the full time series of pentad-day precipitation over the entire study period35, though a much greater computational burden could be incurred. In any case, the study of the secular changes in CISO and monsoon singularity will help better understand the cause of climate change.
An outstanding issue we are facing in the study of TMP is its intensity. There is a large discrepancy between CN05.1 and ERA5 data. The CN05.1 data are based on station rain gauge data, and the rainfall in the high mountain area of Tianshan Mountains is, to a large extent, extrapolated. Although the elevation has been considered the covariate in the interpolation method, the total precipitation is likely underestimated in the mountain region47. Presently, only seven meteorological stations are in the Tianshan Mountains. Thus, we see an urgent need to increase ground-based observations in the highland area of the Tianshan Mountains, which is indispensable for accurately estimating the water resources in the Xinjiang region. On the other hand, ERA5 reanalysis, based on the Cy41r2 with a native horizontal resolution of 31 km, has an advantage in capturing part of the topographic effects on precipitation, which is critical over complex terrains. Although observations do not directly constrain the precipitation, the ERA5 reanalysis becomes more reliable and benefits from decade-long improvements in model physics and data assimilation. However, ERA5 is not perfect as it appeared to exhibit a systematic wet bias in precipitation over the Tibetan Plateau48. Comprehensive assessment and integration of various sources of data (e.g., gauge-, satellite-, radar- and reanalysis-based products) will be highly demanded for a more accurate understanding of the TMP climatology and variability.
Tropical and subtropical CISO is traditionally thought to originate from the phase lock of transient ISO42. However, we find that the midlatitude TMP CISO could result from the abrupt adjustment of the large-scale circulation systems, the Mongolia High and Balkhash Lake Low, throughout the annual march. Understanding the causes of the abrupt changes in the midlatitude circulation system in their annual variations warrants future studies. Additionally, we conjectured that the circulation associated with the high-latitude Mongolian High may convey water vapor from East Asia to the Tianshan Mountains, but various mechanisms involving the Xinjiang precipitation have been proposed49,50,51,52, and further studies based on oxygen isotopes53 or Lagrangian tracking techniques35,54,55 are demanded to understand the origin of the water vapor in the extreme synoptic events over the Tianshan Mountains.
Based on precipitation characteristics, we propose that the Tianshan Mountains belong to a monsoon-like climate regime. However, the overall near-surface circulations do not show a typical monsoon-like annual reversal. Geographically, the Tianshan Mountains are separated from the South Asian monsoon by the Tibetan Plateau and the East Asian monsoon by drylands. On the other hand, the Xinjiang Tianshan Mountains are distinguished from the Mediterranean climate regime to the west (central Asia), albeit they are both categorized by WDCR. Thus, its climate regime remains an unsettled issue, inviting further studies.
Methods
Data
The primary datasets, including the daily precipitation and atmospheric circulation fields are obtained from ERA5 reanalysis56, the fifth generation of atmospheric reanalysis, provided by the European Centre for Medium‐Range Weather Forecasts. ERA5 combines model data derived from the Integrated Forecasting System Cy41r2 with observations worldwide into a globally complete and consistent dataset31. ERA5 has been widely used in atmospheric sciences as it provides abundant variables and a high horizontal resolution of 31 km. High-resolution data are particularly relevant to studying precipitation variability in complex topographic regions.
We also used the daily precipitation data from CN05.147, a gridded dataset with a high resolution of 0.25° × 0.25° during 1961–2019. The CN05.1 precipitation is interpolated from a total of 2416 rain gauge observations in China. The “anomaly approximation” is applied in the interpolation. The climatology is first interpolated by thin-plate smoothing splines47. Then a gridded daily anomaly derived from the angular distance weighting method is added to the climatology to obtain the final dataset.
The 106 rain gauge data in the Xinjiang region were provided by the Chinese Meteorological Administration. In which, seven stations above 1500 m are chosen to depict the TMP, and 11 stations with an altitude of 1000–1500 m are selected to represent the Tianshan Mountains foothill precipitation. Note that, only six stations are used to explore the linear precipitation trend in Fig. 3 limited by the length of the data. The pentad mean data were computed from the daily data.
Detecting Sudden change pentads of the annual variation of precipitation
We detect the SCPs in the climatological annual cycles using the two-tailed Student’s t test. The null hypothesis is that the sample means are from the same population, and the rejection of the null hypothesis means that the sample means are from two different populations. The t test statistic for a variable \(x\) with a range \(r\),
follows a Student’s t distribution. \({t}_{r}\left(i\right)\) is the t value on the fixed pentad \(i\), and \({x}_{i,i+r-1}\) represents the climatological precipitation from \({{\rm{P}}}_{i}\) to \({{\rm{P}}}_{i+r-1}\). Taking P6 and \(r\) = 5 for example, the t value at P6 is estimated by the statistical significance of the difference between the P1-P5 and P6-P10. Additionally, the significant pentad is determined by two criteria: (1) the t value is above the 99% confidence level, and (2) the t value must be a local maximum (minimum), which means higher (lower) than the t value in the adjacent two pentads on both sides.
Figure 4b displays the detected SCPs in TMP derived from the ERA5 following the foregoing methodology for the t test range is five pentads. Note that we also computed the t values using different test ranges from 3 to 5, the detected sudden change pentads are consistent among three different ranges.
Defining CISO and testing its statistical significance
The climatological precipitation contains two major components, a “smoothed” base annual cycle and a CISO42. Following Wang and Xu42, the smoothed annual cycle is calculated with the annual mean plus the first four annual Fourier harmonics, and the sum of the 6th to 13th Fourier harmonics comprises the 30–60-day CISO. In the significance test, the null hypothesis is that the CISO amplitude at a fixed day is not significantly different from zero. Using the two-tailed t test and given a sample size \(n\) of 30, the test statistic,
follows the Student’s t distribution with the degree of freedom \(n\)-1, where \(A\) denotes the amplitude of CISO and \(S\) is the sample standard deviation that results from the year-to-year variation. If \(t\) > 2.756 (2.045), the null hypothesis would be rejected at the 99% (95%) confidence level, implying that the climatological pentad mean departure should not be viewed as an ordinary sampling fluctuation.
Data availability
Data related to this paper can be downloaded from the following. The meteorological data is retrieved from the ERA5 by the European Center for Medium-Range Weather Forecast (ECMWF) at https://www.ecmwf.int/en/forecasts/datasets/reanalysis-datasets/era5, Chinese Meteorological Station data at http://cdc.cma.gov.cn, river and lake data at https://www.webmap.cn and https://www.hydrosheds.org. DEM data is co-produced by the General Bathymetric Chart of the Oceans and Nippon Foundation at https://www.gebco.net. The CN05.1 data is available by applying to the data producer.
Code availability
All codes used for analyses of the data are available from the corresponding author upon reasonable request.
References
Wu, Z., Zhang, H., Krause, C. M. & Cobb, N. S. Climate change and human activities: a case study in Xinjiang, China. Clim. Change 99, 457–472 (2010).
Lu, X. et al. Correcting GPM IMERG precipitation data over the Tianshan Mountains in China. J. Hydrol. 575, 1239–1252 (2019).
Sorg, A., Bolch, T., Stoffel, M., Solomina, O. & Beniston, M. Climate change impacts on glaciers and runoff in Tien Shan (Central Asia). Nat. Clim. Chang. 2, 725–731 (2012).
Li, X. et al. Improvement of the multi-source weighted-ensemble precipitation dataset and application in the arid area of Tianshan Mountains, central Asia. Adv. Space Res. 72, 327–348 (2023).
Ma, Q. et al. How do multiscale interactions affect extreme precipitation in Eastern Central Asia? J. Clim. 34, 7475–7491 (2021).
Chen, H. & Sun, J. Changes in drought characteristics over China using the standardized precipitation evapotranspiration index. J. Clim. 28, 5430–5447 (2015).
Yang, F. et al. Desert environment and climate observation network over the Taklimakan Desert. Bull. Am. Meteorol. Soc. 102, E1172–E1191 (2020).
Chen, F. et al. Westerlies Asia and monsoonal Asia: spatiotemporal differences in climate change and possible mechanisms on decadal to sub-orbital timescales. Earth-Sci. Rev. 192, 337–354 (2019).
Huang, W., Chen, J., Zhang, X., Feng, S. & Chen, F. Definition of the core zone of the “westerlies-dominated climatic regime”, and its controlling factors during the instrumental period. Sci. China Earth Sci. 58, 676–684 (2015).
Ranghui, W., Huizhi, Z. & Qing, H. Characteristics and laws of MODS coupling relation in arid zone under global change.Chin. Sci. Bull. 51, 75–81 (2006).
Wei, H. et al. Evaluation on dynamic change and interrelations of ecosystem services in a typical mountain-oasis-desert region. Ecol. Indic. 93, 917–929 (2018).
Yao, J. et al. Intensification of extreme precipitation in arid Central Asia. J. Hydrol. 598, 125760 (2021).
Shen, Y.-J. et al. Review of historical and projected future climatic and hydrological changes in mountainous semiarid Xinjiang (northwestern China), central Asia. CATENA 187, 104343 (2020).
Zhao, W. Y., Chen, Y. N., Li, J. L. & Jia, G. S. Periodicity of plant yield and its response to precipitation in the steppe desert of the Tianshan Mountains region. J. Arid Environ. 74, 445–449 (2010).
Chen, Y., Li, Z., Fan, Y., Wang, H. & Deng, H. Progress and prospects of climate change impacts on hydrology in the arid region of northwest China. Environ. Res. 139, 11–19 (2015).
Shi, Y. & Chen, Y. Signal, impact and outlook of climate shift from warm-dry to warm-humid in Northwest China (in Chinese). Sci. Technol. Rev. 000, 54–57 (2003).
Yao, J. et al. Climatic and associated atmospheric water cycle changes over the Xinjiang, China. J. Hydrol. 585, 124823 (2020).
Zhang, Q., Singh, V. P., Li, J., Jiang, F. & Bai, Y. Spatio-temporal variations of precipitation extremes in Xinjiang, China. J. Hydrol. 434–435, 7–18 (2012).
Yao, J. et al. Recent climate and hydrological changes in a mountain–basin system in Xinjiang, China. Earth-Sci. Rev. 226, 103957 (2022).
Ren, Y. et al. Attribution of dry and wet climatic changes over Central Asia. J. Clim. 35, 1399–1421 (2022).
Wu, P., Ding, Y., Liu, Y. & Li, X. The characteristics of moisture recycling and its impact on regional precipitation against the background of climate warming over Northwest China. Int. J. Climatol. 39, 5241–5255 (2019).
Dai, A. Drought under global warming: a review. Wileys Interdiscip. Rev. Clim. Chang. 2, 45–65 (2011).
Yang, T., Ding, J., Liu, D., Wang, X. & Wang, T. Combined use of multiple drought indices for global assessment of dry gets drier and wet gets wetter paradigm. J. Clim. 32, 737–748 (2019).
Greve, P. et al. Global assessment of trends in wetting and drying over land. Nat. Geosci. 7, 716–721 (2014).
Kao, Y.-H. Some problems about the East Asian summer monsoon (in Chinese), (Sci. Press,1962).
Yihui, D. & Chan, J. C. L. The East Asian summer monsoon: an overview. Meteorol. Atmos. Phys. 89, 117–142 (2005).
Zhou, T., Gong, D., Li, J. & Li, B. Detecting and understanding the multi-decadal variability of the East Asian summer monsoon–recent progress and state of affairs. Meteorol. Z. 18, 455–467 (2009).
Wang, B. & Ding, Q. Global monsoon: dominant mode of annual variation in the tropics.Dyn. Atmos. Ocean. 44, 165–183 (2008).
Song, Z., Zhu, C., Su, J. & Liu, B. Coupling modes of climatological intraseasonal oscillation in the East Asian summer monsoon. J. Clim. 29, 6363–6382 (2016).
LinHo & Wang, B. The time–space structure of the Asian–Pacific summer monsoon: a fast annual cycle view. J. Clim. 15, 2001–2019 (2002).
Wang, H., Liu, F. & Dong, W. Features of climatological intraseasonal oscillation during Asian summer monsoon onset and their simulations in CMIP6 models. Clim. Dyn. 59, 3153–3166 (2022).
Suhas, E. & Goswami, B. N. Regime shift in Indian summer monsoon climatological intraseasonal oscillations. Geophys. Res. Lett. 35, L20703 (2008).
Wang, B., Liu, J., Kim, H.-J., Webster, P. J. & Yim, S.-Y. Recent change of the global monsoon precipitation (1979–2008). Clim. Dyn. 39, 1123–1135 (2012).
Ding, Y. Summer monsoon rainfalls in China. J. Meteorol. Soc. Jpn. Ser. II 70, 373–396 (1992).
Cheng, T. F. & Lu, M. Global Lagrangian tracking of continental precipitation recycling footprint and Cascades.J. Clim. 36, 1923–1941 (2023).
Wang, B. & LinHo Rainy season of the Asian–Pacific summer monsoon. J. Clim. 15, 386–398 (2002).
Wang, Z.-Y. & Ding, Y. Climatic characteristics of rainy seasons in china.Chin. J. Atmos. Sci. 32, 1–13 (2008).
Wang, B., LinHo, Zhang, Y. & Lu, M.-M. Definition of South China sea monsoon onset and commencement of the East Asia summer monsoon. J. Clim. 17, 699–710 (2004).
Dai, L., Cheng, T. F. & Lu, M. Define East Asian monsoon annual cycle via a self-organizing map-based approach. Geophys. Res. Lett. 48, e2020GL089542 (2021).
Sheng, B., Wang, H., Li, H., Wu, K. & Li, Q. Thermodynamic and dynamic effects of anomalous dragon boat water over South China in 2022. Weather Clim. Extrem. 40, 100560 (2023).
Kao, Y.-H. On the high Autumn clear weather in China. Acta Meteorol. Sin. (in Chinese) 83–92 https://doi.org/10.11676/qxxb1958.010 (1958).
Wang, B. & Xu, X. Northern hemisphere summer monsoon singularities and climatological intraseasonal oscillation. J. Clim. 10, 1071–1085 (1997).
Rampanelli, G., Zardi, D. & Rotunno, R. Mechanisms of up-valley winds. J. Atmos. Sci. 61, 3097–3111 (2004).
Barros, A. P. & Lettenmaier, D. P. Dynamic modeling of orographically induced precipitation. Rev. Geophys. 32, 265–284 (1994).
Schiemann, R., Lüthi, D. & Schär, C. Seasonality and interannual variability of the Westerly jet in the Tibetan plateau region. J. Clim. 22, 2940–2957 (2009).
Wang, B., Jhun, J.-G. & Moon, B.-K. Variability and singularity of Seoul, South Korea, rainy season (1778–2004). J. Clim. 20, 2572–2580 (2007).
Wu, J. & Gao, X. A gridded daily observation dataset over China region and comparison with the other datasets. Chin. J. Geophys. 56, 1102–1111 (2013).
Ou, T. et al. Wet bias of summer precipitation in the northwestern Tibetan Plateau in ERA5 is linked to overestimated lower-level southerly wind over the plateau. Clim. Dyn. 61, 2139–2153 (2023).
Chen, C. et al. Increasing summer precipitation in arid Central Asia linked to the weakening of the East Asian summer monsoon in the recent decades. Int. J. Climatol. 41, 1024–1038 (2021).
Wu, P., Ding, Y. & Liu, Y. A new study of El Niño impacts on summertime water vapor transport and rainfall in China. Acta Meteorol. Sin. 75, 13 (2017).
Jiang, J., Zhou, T., Chen, X. & Wu, B. Central Asian precipitation shaped by the tropical pacific decadal variability and the atlantic multidecadal variability. J. Clim. 34, 7541–7553 (2021).
Zhang, J., Wang, S., He, Y., Ren, Y. & Huang, J. Contribution of the precipitation-recycling process to the wetting trend in Xinjiang, China. J. Geophys. Res. Atmos. 127, e2021JD036407 (2022).
Liu, X. et al. Variations in the oxygen isotopic composition of precipitation in the Tianshan Mountains region and their significance for the westerly Circulation.J. Geogr. Sci. 25, 801–816 (2015).
Cheng, T. F. & Lu, M. Moisture source–receptor network of the East Asian summer monsoon land regions and the associated atmospheric steerings. J. Clim. 33, 9213–9231 (2020).
Cheng, T. F., Lu, M. & Dai, L. Moisture channels and pre-existing weather systems for East Asian rain belts. npj Clim. Atmos. Sci. 4, 32 (2021).
Hersbach, H. et al. The ERA5 global reanalysis. Q. J. R. Meteorol. Soc. 146, 1999–2049 (2020).
Acknowledgements
This work is jointly supported by the Natural Science Foundation of Xinjiang Uygur Autonomous Region (Grant No. 2022D01C78), the Basic Resource Investigate Project of the Ministry of Science and Technology: Land Resource Carrying Capacity and Ecological Agriculture Investigation and assessment of Turpan-Hami Basin (2022xjkk1103), the Basic Research Projects for Universities in the Autonomous Region (XJEDU2023P018), and the National Natural Science Foundation of China (Grant Nos. 42205055). B.W. acknowledges support from NSF/Climate dynamics Award #2025057. This is publication No. 11758 of the School of Ocean and Earth Science and Technology, publication No. 1615 of the International Pacific Research Center, and publication No. 417 of the Earth System Modeling Center.
Author information
Authors and Affiliations
Contributions
C.J. initialized and wrote the original drafts. B.W. sharpened up major points and revised the draft manuscript. C.J. and L.D. conducted analyses and visualization. L.D., T.F.C. and T.W. participated in discussions. All authors read and approved the final manuscript.
Corresponding author
Ethics declarations
Competing interests
The authors declare no competing interests.
Additional information
Publisher’s note Springer Nature remains neutral with regard to jurisdictional claims in published maps and institutional affiliations.
Rights and permissions
Open Access This article is licensed under a Creative Commons Attribution 4.0 International License, which permits use, sharing, adaptation, distribution and reproduction in any medium or format, as long as you give appropriate credit to the original author(s) and the source, provide a link to the Creative Commons license, and indicate if changes were made. The images or other third party material in this article are included in the article’s Creative Commons license, unless indicated otherwise in a credit line to the material. If material is not included in the article’s Creative Commons license and your intended use is not permitted by statutory regulation or exceeds the permitted use, you will need to obtain permission directly from the copyright holder. To view a copy of this license, visit http://creativecommons.org/licenses/by/4.0/.
About this article
Cite this article
Jin, C., Wang, B., Cheng, T.F. et al. How much we know about precipitation climatology over Tianshan Mountains––the Central Asian water tower. npj Clim Atmos Sci 7, 21 (2024). https://doi.org/10.1038/s41612-024-00572-x
Received:
Accepted:
Published:
DOI: https://doi.org/10.1038/s41612-024-00572-x