Abstract
Most disease associations detected by genome-wide association studies (GWAS) lie outside coding genes, but very few have been mapped to causal regulatory variants. Here, we present a method for detecting regulatory quantitative trait loci (QTLs) that does not require genotyping or whole-genome sequencing. The method combines deep, long-read chromatin immunoprecipitation–sequencing (ChIP-seq) with a statistical test that simultaneously scores peak height correlation and allelic imbalance: the genotype-independent signal correlation and imbalance (G-SCI) test. We performed histone acetylation ChIP-seq on 57 human lymphoblastoid cell lines and used the resulting reads to call 500,066 single-nucleotide polymorphisms de novo within regulatory elements. The G-SCI test annotated 8,764 of these as histone acetylation QTLs (haQTLs)—an order of magnitude larger than the set of candidates detected by expression QTL analysis. Lymphoblastoid haQTLs were highly predictive of autoimmune disease mechanisms. Thus, our method facilitates large-scale regulatory variant detection in any moderately sized cohort for which functional profiling data can be generated, thereby simplifying identification of causal variants within GWAS loci.
This is a preview of subscription content, access via your institution
Access options
Subscribe to this journal
Receive 12 print issues and online access
$259.00 per year
only $21.58 per issue
Buy this article
- Purchase on Springer Link
- Instant access to full article PDF
Prices may be subject to local taxes which are calculated during checkout






Similar content being viewed by others
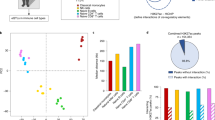
Accession codes
References
Boyle, A.P. et al. Annotation of functional variation in personal genomes using RegulomeDB. Genome Res. 22, 1790–1797 (2012).
Manolio, T.A. Genomewide association studies and assessment of the risk of disease. N. Engl. J. Med. 363, 166–176 (2010).
Maurano, M.T. et al. Systematic localization of common disease-associated variation in regulatory DNA. Science 337, 1190–1195 (2012).
Musunuru, K. et al. From noncoding variant to phenotype via SORT1 at the 1p13 cholesterol locus. Nature 466, 714–719 (2010).
Bauer, D.E. et al. An erythroid enhancer of BCL11A subject to genetic variation determines fetal hemoglobin level. Science 342, 253–257 (2013).
van den Boogaard, M. et al. Genetic variation in T-box binding element functionally affects SCN5A/SCN10A enhancer. J. Clin. Invest. 122, 2519–2530 (2012).
Smemo, S. et al. Obesity-associated variants within FTO form long-range functional connections with IRX3. Nature 507, 371–375 (2014).
Spieler, D. et al. Restless legs syndrome-associated intronic common variant in Meis1 alters enhancer function in the developing telencephalon. Genome Res. 24, 592–603 (2014).
Altshuler, D., Daly, M.J. & Lander, E.S. Genetic mapping in human disease. Science 322, 881–888 (2008).
Faye, L.L., Machiela, M.J., Kraft, P., Bull, S.B. & Sun, L. Re-ranking sequencing variants in the post-GWAS era for accurate causal variant identification. PLoS Genet. 9, e1003609 (2013).
The GTEx Consortium. The Genotype-Tissue Expression (GTEx) project. Nat. Genet. 45, 580–585 (2013).
Lappalainen, T. et al. Transcriptome and genome sequencing uncovers functional variation in humans. Nature 501, 506–511 (2013).
Montgomery, S.B. et al. Transcriptome genetics using second generation sequencing in a Caucasian population. Nature 464, 773–777 (2010).
Pickrell, J.K. et al. Understanding mechanisms underlying human gene expression variation with RNA sequencing. Nature 464, 768–772 (2010).
Veyrieras, J.B. et al. High-resolution mapping of expression-QTLs yields insight into human gene regulation. PLoS Genet. 4, e1000214 (2008).
Raj, T. et al. Polarization of the effects of autoimmune and neurodegenerative risk alleles in leukocytes. Science 344, 519–523 (2014).
Battle, A. et al. Characterizing the genetic basis of transcriptome diversity through RNA-sequencing of 922 individuals. Genome Res. 24, 14–24 (2014).
Degner, J.F. et al. DNase I sensitivity QTLs are a major determinant of human expression variation. Nature 482, 390–394 (2012).
Gaulton, K.J. et al. A map of open chromatin in human pancreatic islets. Nat. Genet. 42, 255–259 (2010).
Kasowski, M. et al. Variation in transcription factor binding among humans. Science 328, 232–235 (2010).
McDaniell, R. et al. Heritable individual-specific and allele-specific chromatin signatures in humans. Science 328, 235–239 (2010).
Ni, Y., Hall, A.W., Battenhouse, A. & Iyer, V.R. Simultaneous SNP identification and assessment of allele-specific bias from ChIP-seq data. BMC Genet. 13, 46 (2012).
Smith, A.J. et al. Use of allele-specific FAIRE to determine functional regulatory polymorphism using large-scale genotyping arrays. PLoS Genet. 8, e1002908 (2012).
Kasowski, M. et al. Extensive variation in chromatin states across humans. Science 342, 750–752 (2013).
Kilpinen, H. et al. Coordinated effects of sequence variation on DNA binding, chromatin structure, and transcription. Science 342, 744–747 (2013).
McVicker, G. et al. Identification of genetic variants that affect histone modifications in human cells. Science 342, 747–749 (2013).
Altshuler, D.M. et al. Integrating common and rare genetic variation in diverse human populations. Nature 467, 52–58 (2010).
The 1000 Genomes Project Consortium. An integrated map of genetic variation from 1,092 human genomes. Nature 491, 56–65 (2012).
Wang, Z. et al. Combinatorial patterns of histone acetylations and methylations in the human genome. Nat. Genet. 40, 897–903 (2008).
Heintzman, N.D. et al. Histone modifications at human enhancers reflect global cell-type-specific gene expression. Nature 459, 108–112 (2009).
Kumar, V. et al. Uniform, optimal signal processing of mapped deep-sequencing data. Nat. Biotechnol. 31, 615–622 (2013).
Gerstein, M.B. et al. Architecture of the human regulatory network derived from ENCODE data. Nature 489, 91–100 (2012).
Neph, S. et al. An expansive human regulatory lexicon encoded in transcription factor footprints. Nature 489, 83–90 (2012).
DePristo, M.A. et al. A framework for variation discovery and genotyping using next-generation DNA sequencing data. Nat. Genet. 43, 491–498 (2011).
Bamshad, M.J. et al. Exome sequencing as a tool for Mendelian disease gene discovery. Nat. Rev. Genet. 12, 745–755 (2011).
Benjamini, Y. & Hochberg, Y. Controlling the false discovery rate: a practical and powerful approach to multiple testing. J. R. Stat. Soc. Series B Stat. Methodol. 57, 289–300 (1995).
Potapova, A. et al. Systematic cross-validation of 454 sequencing and pyrosequencing for the exact quantification of DNA methylation patterns with single CpG resolution. BMC Biotechnol. 11, 6 (2011).
Hou, S. et al. Identification of a susceptibility locus in STAT4 for Behcet's disease in Han Chinese in a genome-wide association study. Arthritis Rheum. 64, 4104–4113 (2012).
Kirino, Y. et al. Genome-wide association analysis identifies new susceptibility loci for Behcet's disease and epistasis between HLA-B*51 and ERAP1. Nat. Genet. 45, 202–207 (2013).
Pai, A.A. et al. The contribution of RNA decay quantitative trait loci to inter-individual variation in steady-state gene expression levels. PLoS Genet. 8, e1003000 (2012).
Li, H. & Durbin, R. Fast and accurate short read alignment with Burrows-Wheeler transform. Bioinformatics 25, 1754–1760 (2009).
Kent, W.J. et al. The human genome browser at UCSC. Genome Res. 12, 996–1006 (2002).
Jurinke, C., van den Boom, D., Cantor, C.R. & Koster, H. The use of MassARRAY technology for high throughput genotyping. Adv. Biochem. Eng. Biotechnol. 77, 57–74 (2002).
Skotte, L., Korneliussen, T.S. & Albrechtsen, A. Association testing for next-generation sequencing data using score statistics. Genet. Epidemiol. 36, 430–437 (2012).
ONeill, R. Algorithm AS 47: function minimization using a simplex procedure. J. R. Stat. Soc. Ser. C Appl. Stat. 20, 338–345 (1971).
Nelder, J.A. & Mead, R. A simplex method for function minimization. Comput. J. 7, 308–313 (1965).
Benjamini, Y. & Yekutieli, D. The control of the false discovery rate in multiple testing under dependency. Ann. Stat. 29, 1165–1188 (2001).
Degner, J.F. et al. Effect of read-mapping biases on detecting allele-specific expression from RNA-sequencing data. Bioinformatics 25, 3207–3212 (2009).
Kheradpour, P. & Kellis, M. Systematic discovery and characterization of regulatory motifs in ENCODE TF binding experiments. Nucleic Acids Res. 42, 2976–2987 (2014).
Welter, D. et al. The NHGRI GWAS Catalog, a curated resource of SNP-trait associations. Nucleic Acids Res. 42, D1001–D1006 (2014).
Beecham, A.H. et al. Analysis of immune-related loci identifies 48 new susceptibility variants for multiple sclerosis. Nat. Genet. 45, 1353–1360 (2013).
Tsoi, L.C. et al. Identification of 15 new psoriasis susceptibility loci highlights the role of innate immunity. Nat. Genet. 44, 1341–1348 (2012).
Eyre, S. et al. High-density genetic mapping identifies new susceptibility loci for rheumatoid arthritis. Nat. Genet. 44, 1336–1340 (2012).
Cortes, A. et al. Identification of multiple risk variants for ankylosing spondylitis through high-density genotyping of immune-related loci. Nat. Genet. 45, 730–738 (2013).
Acknowledgements
This work was funded by the Agency for Science, Technology and Research (A*STAR), Singapore, including grant no. GIS/11-IAF300-4.
Author information
Authors and Affiliations
Contributions
R.C.-H.d.R. performed the computational and statistical analyses; J.P. performed ChIP-seq experiments and PyroMark validation with assistance from E.P.; R.C.-H.d.R. and S.L.R. programmed the G-SCI test; C.C.K. performed Sequenom validation; S.P., R.C.-H.d.R., J.P. and M.L.H. designed the study; S.P. provided overall supervision; S.P., R.C.-H.d.R. and J.P. drafted the manuscript with assistance from M.L.H. and C.C.K.
Corresponding author
Ethics declarations
Competing interests
The authors declare no competing financial interests.
Integrated supplementary information
Supplementary Figure 1 H3K27ac peak-height correlation across data sets.
Peak-heights were input corrected, normalized by the total number of reads in the library and then Pearson correlations were computed between pairs of data sets. GIS: samples sequenced in this study, KAS: samples sequenced by Kasowski et al. (2013)24, MCV: samples sequenced by McVicker et al. (2013)26, ENC: samples sequenced by ENCODE.
Supplementary Figure 2 Histogram of H3K27ac peak-height correlations for all pairs in the 57-sample set.
Correlation values are the same as in Supplementary Fig. 1. median R=0.87.
Supplementary Figure 3 Quantile-quantile plot of observed versus expected haQTL P values for the 500,066 called SNPs.
The dashed black line is the expected null distribution.
Supplementary Figure 4 Functional enrichment of low-frequency haQTLs.
(a) Enrichment of low frequency (minor allele frequency below 5%) haQTLs in GM12878 TF ChIP-seq peak regions. Only low frequency called SNPs within histone acetylation peaks were used as the negative control. (b) Low-frequency haQTL enrichment in GM12878 TF binding sites. Binding sites were defined as motif matches within the corresponding ChIP-seq peaks and only haQTLs that overlapped the binding site were considered in the analysis. The P-values and enrichment scores were calculated as in Fig. 4b,c.
Supplementary Figure 5 Low-frequency haQTL distribution at promoter and nonpromoter H3K27ac peaks.
Similar to Fig. 5 except that only low frequency (minor allele frequency below 5%) haQTLs and SNPs were used in the analysis. For both promoter and non-promoter peaks, the low frequency haQTLs show an enrichment at the hypersensitive region of the peak (right panels).
Supplementary Figure 7 LD of haQTL SNPs with autoimmune GWAS SNPs.
The upper two bars are identical to Fig. 6b – they represent the GWAS statistics of the set of 8,764 haQTLs regulome-wide by our G-SCI pipeline, which includes an effect-size filter. When that filter was removed, the haQTL set expanded to 17,936 SNPs. Both haQTL sets show enrichment for LD with GWAS SNPs, with the latter having a stronger P-value due to the larger number of tested SNPs. See also Supplementary Table 6.
Supplementary Figure 8 Functional enrichment of haQTLs with and without the effect-size filter.
(a) haQTLs in GM12878 TF ChIP-seq peak regions (b) haQTLs in GM12878 TF binding sites (motifs in peak regions). The P-values and enrichment scores were calculated as in Fig. 4b,c. While haQTL sets were enriched for evidence of transcription factor binding, the filtered set showed stronger enrichment.
Supplementary information
Supplementary Text and Figures
Supplementary Figures 1–8 and Supplementary Tables 1, 2, 4, 6 and 7 (PDF 1154 kb)
Supplementary Table 3
List of haQTLs (XLSX 1403 kb)
Supplementary Table 5
List of autoimmune GWAS SNPs (XLSX 57 kb)
Rights and permissions
About this article
Cite this article
del Rosario, RH., Poschmann, J., Rouam, S. et al. Sensitive detection of chromatin-altering polymorphisms reveals autoimmune disease mechanisms. Nat Methods 12, 458–464 (2015). https://doi.org/10.1038/nmeth.3326
Received:
Accepted:
Published:
Issue Date:
DOI: https://doi.org/10.1038/nmeth.3326
This article is cited by
-
Revisiting genetic artifacts on DNA methylation microarrays exposes novel biological implications
Genome Biology (2021)
-
Integrative epigenomic and high-throughput functional enhancer profiling reveals determinants of enhancer heterogeneity in gastric cancer
Genome Medicine (2021)
-
IMAGE: high-powered detection of genetic effects on DNA methylation using integrated methylation QTL mapping and allele-specific analysis
Genome Biology (2019)
-
Resistin expression in human monocytes is controlled by two linked promoter SNPs mediating NFKB p50/p50 binding and C-methylation
Scientific Reports (2019)
-
Natural genetic variation of the cardiac transcriptome in non-diseased donors and patients with dilated cardiomyopathy
Genome Biology (2017)