Abstract
The current standardization and legalization of commercial housing price assessment is low. In addition, computer technology is less used in this field. Therefore, the evaluation of commercial housing prices can no longer meet the requirements of today’s economy and society. Traditional real estate assessment methods such as market comparison method, cost estimation method, income reduction method, etc., pay attention to market information, but tend to emphasize the total value of real estate in evaluation practice, and they ignore the impact of various constraints on real estate prices, so it is impossible to scientifically understand the influencing factors of real estate prices and their mechanisms of action, resulting in a lack of scientific basis for decision-making by governments, developers or property users. Therefore, this paper introduces the improved algorithm of BP neural network to establish the housing price evaluation model, which reduces the subjectivity and randomness in the evaluation process. This has certain reference for the development of China’s real estate assessment method. The evaluation results show that the real estate evaluation price of the network output is close to the actual price, with maximum error of 3.04%. This shows that the application of improved BP neural network model in real estate price evaluation is not only technically feasible, but also credible.







Similar content being viewed by others
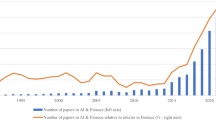
References
Amirazodi N, Saghiri AM, Meybodi M (2018) An adaptive algorithm for super-peer selection considering peer’s capacity in mobile peer-to-peer networks based on learning automata. Peer-to-Peer Netw Appl 11:74–89
Assaf A (2015) Long memory and level shifts in REITs returns and volatility. Int Rev Financ Anal 42:172–182
Bilbao A, Bilbao C, Labeaga JM (2007) A method for evaluating the excess burden associated with characteristics of the goods. Econ Lett 96:209–214
Bouhouras AS, Labridis DP, Bakirtzis AG (2010) Cost/worth assessment of reliability improvement in distribution networks by means of artificial intelligence. Int J Electr Power Energy Syst 32(5):530–538
Cavalcanti DV, Tiago V, Mohaddes K, Raissi M (2015) Commodity price volatility and the sources of growth. J Appl Econom 30(6):857–873
Cervero R, Landis J (1993) Assessing the impacts of urban rail transit on local real estate markets using quasi-experimental comparisons. Transp Res A 27(1):13–22
Chen C, Wang Y, Li L, Hu J, Zhang Z (2012) The retrieval of intra-day trend and its influence on traffic prediction. Transp Res Part C Emerg Technol 22:103–118
Cockburn J, Decaluwe B, Robichoud V (2008) Trade liberalization and poverty: lessons from Asia and Africa. In: Trade liberalization and poverty: a CGE analysis of the 1990s experience in Africa and Asia. Poverty and economic policy (PEP) research network. http://hdl.handle.net/10625/46822. Accessed 24 Dec 2019
Compton J, Wiggins S, Keats S (2010) Impact of the global food crisis on the poor: What is the evidence? Research report. Overseas Development Institute, London
Dávila OG (2010) Food security and poverty in Mexico: the impact of higher global food prices. Food Secur 2:383–393
Decaluwe B, Dumont JC, Savard L (1999) Measuring poverty and inequality in a computable general equilibrium model. Working Paper 99-20 CREFA, Laval University, Quebec
Dong GP, Zhang WZ, Wu WJ, Guo TY (2011) Spatial heterogeneity in determinants of residential land price: simulation and prediction. Acta Geogr Sin 66(6):750–760 (in Chinese)
Dorward A (2012) The short- and medium- term impacts of rises in staple food prices. Food Secur 4(4):633–645
Karfakis P, Velazco J, Moreno E, Covarrubias K (2011) Impact of increasing prices of agricultural commodities on poverty. Agricultural Development Economics Division, Food and Agriculture Organization of the United Nations, ESA Working Paper No. 11–14
Mitra S, Josling T (2009) Agricultural export restrictions: welfare implications and trade disciplines. IPC Position Paper Agricultural and Rural Development Policy Series. International Food and Agricultural Trade Policy Council
Plevin R, Beckman J, Golub A, Witcover J, O’Hare M (2015) Carbon accounting and economic model uncertainty of emissions from biofuels-induced land use change. Environ Sci Technol 49:2656–2664
Qu Z, Perron P (2013) A stochastic volatility model with random level shifts and its applications to S&P 500 and NASDAQ return indices. Econom J 16(3):309–339
Valenzuela E, Hertel T, Keeney R, Reimer J (2007) Assessing global computable general equilibrium model validity using agricultural price volatility. Am J Agric Econ 89(2):383–397
Author information
Authors and Affiliations
Corresponding author
Additional information
Publisher's Note
Springer Nature remains neutral with regard to jurisdictional claims in published maps and institutional affiliations.
Rights and permissions
About this article
Cite this article
Zhou, X. The usage of artificial intelligence in the commodity house price evaluation model. J Ambient Intell Human Comput (2020). https://doi.org/10.1007/s12652-019-01616-4
Received:
Accepted:
Published:
DOI: https://doi.org/10.1007/s12652-019-01616-4