Abstract
Incorporating Battery Energy Storage Systems (BESS) into renewable energy configurations offers numerous apparent advantages. Nonetheless, to fully capitalize on these advantages, it is imperative to implement management strategies that facilitate optimal system performance. Various approaches and methods can be employed to optimize the functionality of BESS within renewable energy systems (RES), encompassing specific dispatch goals as well as financial, technical, or hybrid objectives. These optimization methods are categorized into three primary groups: directed search-based (DSB), probabilistic, and rule-based strategies. Historically, research has heavily focused on tailoring systems based on the renewable energy sources for specific purposes, such as distributed generation (DG). This investigation not only offers a comprehensive overview of battery management measures but also assesses these endeavors in terms of their alignment with application objectives and the chosen optimization strategy. This approach unveils connections between distinct optimization goals and preferred strategies. The findings reveal that DSB approaches and control strategies, commonly employed for technical objectives, are more likely to succeed in addressing financial goals. Moreover, the extent to which a problem can be analytically defined emerges as a critical consideration. Upon comparing the merits and demerits of different reported optimization methodologies, it becomes evident that hybrid approaches, amalgamating the strengths of various optimization techniques, will increasingly shape future operational procedures. This study not only equips researchers with valuable insights into viable optimization strategies for forthcoming generation applications but also provides a cutting-edge overview of battery applications and optimization techniques.
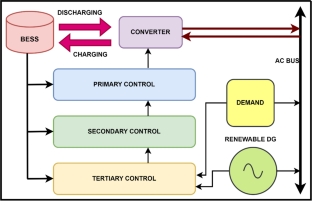







Similar content being viewed by others
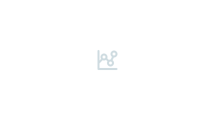
Abbreviations
- ADALINE:
-
Adaptive Linear Neuron
- ADP:
-
Approximate Dynamic Programming
- AI:
-
Artificial Intelligence
- BEMS:
-
Battery Energy Management Systems
- BMS:
-
Battery Management Systems
- CAES:
-
Compressed Air Energy Storage
- DR:
-
Demand Response
- ESS:
-
Energy Storage Systems
- EV:
-
Electric Vehicle
- FLC:
-
Fuzzy Logic Controller
- GA:
-
Genetic Algorithms
- GAMS:
-
General Algebraic Modelling System
- HESS:
-
Hybrid ESS
- HH:
-
Hyper-Heuristic
- HRES:
-
Hybrid Renewable Energy System
- IP:
-
Integer Programming
- LP:
-
Linear Programming
- MILP:
-
Mixed-Integer Linear Programming
- ML:
-
Machine Learning
- MPC:
-
Model Predictive Control
- NaS:
-
Sodium-Sulfur
- NLP:
-
Nonlinear Programming
- NSGA:
-
Non-Dominated Sorting Genetic Algorithm
- OLTC:
-
On-Load Tap Changers
- PSO:
-
Particle Swarm Optimization
- PV:
-
Photovoltaic
- QP:
-
Quadratic Programming
- RC:
-
Resistor–Capacitor
- SDP:
-
Stochastic Dynamic Programming
References
Suberu YM, Mustafa MW, Bashir N (2014) Energy storage systems for renewable energy power sector integration and mitigation of intermittency. Renew Sustain Energy Rev 35:499–514. https://doi.org/10.1016/j.rser.2014.04.009
Luo X, Wang J, Dooner M, Clarke J (2015) Overview of current development in electrical energy storage technologies and the application potential in power system operation. Appl Energy 137:511–536. https://doi.org/10.1016/j.apenergy.2014.09.081
Toledo OM, Oliveira Filho D, Diniz ASAC (2010) Distributed photovoltaic generation and energy storage systems: a review. Renew Sustain Energy Rev 14:506–511. https://doi.org/10.1016/j.rser.2009.08.007
Tan X, Li Q, Wang H (2013) Advances and trends of energy storage technology in Microgrid. Int J Electr Power Energy Syst 44:179–191. https://doi.org/10.1016/j.ijepes.2012.07.015
Mahto T, Mukherjee V (2015) Energy storage systems for mitigating the variability of isolated hybrid power system. Renew Sustain Energy Rev 51:1564–1577. https://doi.org/10.1016/j.rser.2015.07.012
Berrada A, Loudiyi K (2016) Operation, sizing, and economic evaluation of storage for solar and wind power plants. Renew Sustain Energy Rev 59:1117–1129
Divya KC, Østergaard J (2009) Battery energy storage technology for power systems—an overview. Electr Power Syst Res 79(3):511–520. https://doi.org/10.1016/j.epsr.2008.09.017
Das T, Krishnan V, McCalley JD (2015) Assessing the benefits and economics of bulk energy storage technologies in the power grid. Appl Energy 139:104–118. https://doi.org/10.1016/j.apenergy.2014.11.017
Del Rosso AD, Eckroad SW (2014) Energy storage for relief of transmission congestion. IEEE Trans Smart Grid 5(3):1138–1146. https://doi.org/10.1109/TSG.2013.2277411
Stephan A, Battke B, Beuse MD, Clausdeinken JH, Schmidt TS (2016) Limiting the public cost of stationary battery deployment by combining applications. Nat Energy 1:16079. https://doi.org/10.1038/nenergy.2016.79
Saxena V, Kumar N, Nangia U (2022) recent trends in the optimization of renewable distributed generation: a review. Ingen Invest 42(3):e97702. https://doi.org/10.15446/ing.investig.97702
Poullikkas A (2013) A comparative overview of large-scale battery systems for electricity storage. Renew Sustain Energy Rev 27:778–788. https://doi.org/10.1016/j.rser.2013.07.017
Lawder MT, Suthar B, Northrop PWC, De S, Hoff CM, Leitermann O et al (2014) Battery energy storage system (BESS) and battery management system (BMS) for grid-scale applications. Proc IEEE 102(6):1014–1030. https://doi.org/10.1109/JPROC.2014.2317451
Dai H, Jiang B, Hu X, Lin X, Wei X, Pecht M (2020) Advanced battery management strategies for a sustainable energy future: Multilayer design concepts and research trends. Renew Sustain Energy Rev. https://doi.org/10.1016/j.rser.2020.110480
Zakeri B, Syri S (2015) Electrical energy storage systems: a comparative life cycle cost analysis. Renew Sustain Energy Rev 42:569–596. https://doi.org/10.1016/j.rser.2014.10.011
Duffner F, Wentker M, Greenwood M, Leker J (2020) Battery cost modeling: a review and directions for future research. Renew Sustain Energy Rev 127:109872. https://doi.org/10.1016/j.rser.2020.109872
Sani SB, Celvakumaran P, Ramachandaramurthy VK, Walker S, Alrazi B, Jia Y et al (2020) Energy storage system policies: way forward and opportunities for emerging economies. J Energy Storage 32:101902. https://doi.org/10.1016/j.est.2020.101902
Díaz-González F, Sumper A, Gomis-Bellmunt O, Villafáfila-Robles R (2012) A review of energy storage technologies for wind power applications. Renew Sustain Energy Rev 16(4):2154–2171. https://doi.org/10.1016/j.rser.2012.01.029
Zhao H, Wu Q, Hu S, Xu H, Rasmussen CN (2015) Review of energy storage system for wind power integration support. Appl Energy 137:545–553. https://doi.org/10.1016/j.apenergy.2014.04.103
de Siqueira LMS, Peng W (2021) Control strategy to smooth wind power output using battery energy storage system: a review. J Energy Storage 35:102252. https://doi.org/10.1016/j.est.2021.102252
Barra PHA, de Carvalho WC, Menezes TS, Fernandes RAS, Coury DV (2021) A review on wind power smoothing using high-power energy storage systems. Renew Sustain Energy Rev 137:110455. https://doi.org/10.1016/j.rser.2020.110455
Lamsal D, Sreeram V, Mishra Y, Kumar D (2019) Output power smoothing control approaches for wind and photovoltaic generation systems: a review. Renew Sustain Energy Rev 113:109245. https://doi.org/10.1016/j.rser.2019.109245
Akram U, Nadarajah M, Shah R, Milano F (2020) A review on rapid responsive energy storage technologies for frequency regulation in modern power systems. Renew Sustain Energy Rev 120:109626. https://doi.org/10.1016/j.rser.2019.109626
Dai H, Jiang B, Hu X, Lin X, Wei X, Pecht M (2021) Advanced battery management strategies for a sustainable energy future: multilayer design concepts and research trends. Renew Sustain Energy Rev 138:110480. https://doi.org/10.1016/j.rser.2020.110480
Hoppmann J, Volland J, Schmidt TS, Hoffmann VH (2014) The economic viability of battery storage for residential solar photovoltaic systems—a review and a simulation model. Renew Sustain Energy Rev 39:1101–1118. https://doi.org/10.1016/j.rser.2014.07.068
Coppez G, Chowdhury S, Chowdhury SP (2010) Review of battery storage optimisation in distributed generation. In: 2010 Joint international conference on power electronics, drives and energy systems & 2010 power, India, pp 1–6. https://doi.org/10.1109/PEDES.2010.5712406
Hajiaghasi S, Salemnia A, Hamzeh M (2019) Hybrid energy storage system for microgrids applications: a review. J Energy Storage 21:543–570. https://doi.org/10.1016/j.est.2018.12.017
Zhang L, Hu X, Wang Z, Ruan J, Ma C, Song Z et al (2021) Hybrid electrochemical energy storage systems: an overview for smart grid and electrified vehicle applications. Renew Sustain Energy Rev 139:110581. https://doi.org/10.1016/j.rser.2020.110581
Naval N, Yusta JM (2021) Virtual power plant models and electricity markets—a review. Renew Sustain Energy Rev 149:111393. https://doi.org/10.1016/j.rser.2021.111393
Lee JW, Haram MHSM, Ramasamy G, Thiagarajah SP, Ngu EE, Lee YH (2021) Technical feasibility and economics of repurposed electric vehicles batteries for power peak shaving. J Energy Storage 40:102752. https://doi.org/10.1016/j.est.2021.102752
Zhang Z, Ding T, Zhou Q, Sun Y, Qu M, Zeng Z et al (2021) A review of technologies and applications on versatile energy storage systems. Renew Sustain Energy Rev 148:111263. https://doi.org/10.1016/j.rser.2021.111263
Tan KM, Babu TS, Ramachandaramurthy VK, Kasinathan P, Solanki SG, Raveendran SK (2021) Empowering smart grid: a comprehensive review of energy storage technology and application with renewable energy integration. J Energy Storage 39:102591. https://doi.org/10.1016/j.est.2021.102591
Bidram A, Davoudi A (2012) Hierarchical structure of microgrids control system. IEEE Trans Smart Grid 3:1963–1976. https://doi.org/10.1109/TSG.2012.2197425
Morstyn T, Hredzak B, Agelidis VG (2016) Control strategies for microgrids with distributed energy storage systems: an overview. IEEE Trans Smart Grid. https://doi.org/10.1109/TSG.2016.2637958
Dunn B, Kamath H, Tarascon J-M (2011) Electrical energy storage for the grid: a battery of choices. Science 334(80):928–935. https://doi.org/10.1126/science.1212741
Ferreira HL, Garde R, Fulli G, Kling W, Lopes JP (2013) Characterisation of electrical energy storage technologies. Energy 53:288–298. https://doi.org/10.1016/j.energy.2013.02.037
Jenkins DP, Fletcher J, Kane D (2008) Lifetime prediction and sizing of lead–acid batteries for microgeneration storage applications. IET Renew Power Gener 2:191–200. https://doi.org/10.1049/iet-rpg:20080021
Dogger JD, Roossien B, Nieuwenhout FDJ (2011) Characterization of Li-ion batteries for intelligent management of distributed grid-connected storage. IEEE Trans Energy Convers 26:256–263. https://doi.org/10.1109/TEC.2009.2032579
Hatta T (2012) Applications of sodium-sulfur batteries. In: Transmission and distribution conference and exposition (T&D), 2012 IEEE PES, pp 1–3. https://doi.org/10.1109/TDC.2012.6281442
Stan AI, Swierczynski M, Stroe DI, Teodorescu R, Andreasen SJ (2014) Lithium ion battery chemistries from renewable energy storage to automotive and back-up power applications: an overview. In: 2014 International conference on optimization of electrical and electronic equipment (OPTIM), pp 713–720. https://doi.org/10.1109/OPTIM.2014.6850936
Baccino F, Marinelli M, Nørgård P, Silvestro F (2014) Experimental testing procedures and dynamic model validation for vanadium redox flow battery storage system. J Power Sources 254:277–286. https://doi.org/10.1016/j.jpowsour.2013.12.078
Schreiber M, Harrer M, Whitehead A, Bucsich H, Dragschitz M, Seifert E et al (2012) Practical and commercial issues in the design and manufacture of vanadium flow batteries. J Power Sources 206:483–489. https://doi.org/10.1016/j.jpowsour.2010.12.032
Li Y, Sun L, Cao L, Bao J, Skyllas-Kazacos M (2021) Dynamic model based membrane permeability estimation for online SOC imbalances monitoring of vanadium redox flow batteries. J Energy Storage 39:102688. https://doi.org/10.1016/j.est.2021.102688
Liu W, Placke T, Chau KT (2022) Overview of batteries and battery management for electric vehicles. Energy Rep 8:4058–4084. https://doi.org/10.1016/j.egyr.2022.03.016
Zou C, Zhang L, Hu X, Wang Z, Wik T, Pecht M (2018) A review of fractional-order techniques applied to lithium-ion batteries, lead-acid batteries, and supercapacitors. J Power Sources 390:286–296. https://doi.org/10.1016/j.jpowsour.2018.04.033
Wang Y, Tian J, Sun Z, Wang L, Xu R, Li M et al (2020) A comprehensive review of battery modeling and state estimation approaches for advanced battery management systems. Renew Sustain Energy Rev 131:110015. https://doi.org/10.1016/j.rser.2020.110015
Shahjalal M, Shams T, Islam ME, Alam W, Modak M, Hossain SB et al (2021) A review of thermal management for Li-ion batteries: prospects, challenges, and issues. J Energy Storage 39:102518. https://doi.org/10.1016/j.est.2021.102518
Nguyen TA, Crow ML, Elmore AC (2015) Optimal sizing of a vanadium redox battery system for microgrid systems. IEEE Trans Sustain Energy 6:729–737. https://doi.org/10.1109/TSTE.2015.2404780
Cervone A, Carbone G, Santini E, Teodori S (2016) Optimization of the battery size for PV systems under regulatory rules using a Markov-Chains approach. Renew Energy 85:657–665. https://doi.org/10.1016/j.renene.2015.07.007
Rodrigues EMG, Osório GJ, Godina R, Bizuayehu AW, Lujano-Rojas JM, Matias JCO et al (2015) Modelling and sizing of NaS (sodium sulfur) battery energy storage system for extending wind power performance in Crete Island. Energy 90:1606–1617. https://doi.org/10.1016/j.energy.2015.06.116
Aaslid P, Geth F, Korpås M, Belsnes MM, Fosso OB (2020) Non-linear charge-based battery storage optimization model with bi-variate cubic spline constraints. J Energy Storage 32:101979. https://doi.org/10.1016/j.est.2020.101979
Li X (2012) Fuzzy adaptive Kalman filter for wind power output smoothing with battery energy storage system. IET Renew Power Gener 6:340–347. https://doi.org/10.1049/iet-rpg.2011.0177
Eghtedarpour N, Farjah E (2012) Control strategy for distributed integration of photovoltaic and energy storage systems in DC micro-grids. Renew Energy 45:96–110. https://doi.org/10.1016/j.renene.2012.02.017
Fares RL, Webber ME (2014) A flexible model for economic operational management of grid battery energy storage. Energy 78:768–776. https://doi.org/10.1016/j.energy.2014.10.072
Lee SJ, Kim JH, Kim CH, Kim SK, Kim ES, Kim DU et al (2016) Coordinated control algorithm for distributed battery energy storage systems for mitigating voltage and frequency deviations. IEEE Trans Smart Grid 7:1713–1722. https://doi.org/10.1109/TSG.2015.2429919
Sebastián R (2016) Application of a battery energy storage for frequency regulation and peak shaving in a wind diesel power system. IET Gener Transm Distrib 10:764–770. https://doi.org/10.1049/iet-gtd.2015.0435
Abdeltawab HH, Mohamed YARI (2015) Market-oriented energy management of a hybrid wind-battery energy storage system via model predictive control with constraint optimizer. IEEE Trans Ind Electron 62:6658–6670. https://doi.org/10.1109/TIE.2015.2435694
Tao L, Ma J, Cheng Y, Noktehdan A, Chong J, Lu C (2017) A review of stochastic battery models and health management. Renew Sustain Energy Rev 80:716–732. https://doi.org/10.1016/j.rser.2017.05.127
Xu B, Oudalov A, Ulbig A, Andersson G, Kirschen D (2016) Modeling of lithium-ion battery degradation for cell life assessment. IEEE Trans Smart Grid. https://doi.org/10.1109/TSG.2016.2578950
IRENA (2015) Battery storage for renewables: market status and technology outlook. http://www.irena.org/documentdownloads/publications/irena_battery_storage_report_2015.pdf
Sullivan JP, Fenton KR, Gabaly FEG, Harris TC, Hayden CC et al (2015) The science of battery degradation. Unlimited Release. Sandia National Laboratories, Albuquerque
Krieger EM, Cannarella J, Arnold CB (2013) A comparison of lead-acid and lithium-based battery behavior and capacity fade in off-grid renewable charging applications. Energy 60:492–500. https://doi.org/10.1016/j.energy.2013.08.029
Nuhic A, Terzimehic T, Soczka-Guth T, Buchholz M, Dietmayer K (2013) Health diagnosis and remaining useful life prognostics of lithium-ion batteries using data-driven methods. J Power Sources 239:680–688. https://doi.org/10.1016/j.jpowsour.2012.11.146
Cotton B (2012) VRLA battery lifetime fingerprints—Part 1. In: Intelec 2012. IEEE. https://doi.org/10.1109/INTLEC.2012.6374495
Ru Y, Kleissl J, Martinez S (2013) Storage size determination for grid-connected photovoltaic systems. IEEE Trans Sustain Energy 4:68–81. https://doi.org/10.1109/TSTE.2012.2199339
Stroe DI, Knap V, Swierczynski M, Stroe AI, Teodorescu R (2017) Operation of a grid-connected lithium-ion battery energy storage system for primary frequency regulation: a battery lifetime perspective. IEEE Trans Ind Appl 53:430–438. https://doi.org/10.1109/TIA.2016.2616319
Dragicevic T, Pandzic H, Skrlec D, Kuzle I, Guerrero JM, Kirschen DS (2014) Capacity optimization of renewable energy sources and battery storage in an autonomous telecommunication facility. IEEE Trans Sustain Energy 5:1367–1378. https://doi.org/10.1109/TSTE.2014.2316480
Li S, He H, Su C, Zhao P (2020) Data driven battery modeling and management method with aging. Appl Energy 275:115340. https://doi.org/10.1016/j.apenergy.2020.115340
Wang S, Guo D, Han X, Lu L, Sun K, Li W et al (2020) Impact of battery degradation models on energy management of a grid-connected DC microgrid. Energy 207:118228. https://doi.org/10.1016/j.energy.2020.118228
Lujano-Rojas JM, Dufo-López R, Atencio-Guerra JL, Rodrigues EMG, Bernal- Agustín JL, Catalão JPS (2016) Operating conditions of lead-acid batteries in the optimization of hybrid energy systems and microgrids. Appl Energy 179:590–600. https://doi.org/10.1016/j.apenergy.2016.07.018
Luo F, Meng K, Dong ZY, Zheng Y, Chen Y, Wong KP (2015) Coordinated operational planning for wind farm with battery energy storage system. IEEE Trans Sustain Energy 6:253–262. https://doi.org/10.1109/TSTE.2014.2367550
Yao DL, Choi SS, Tseng KJ, Lie TT (2012) Determination of short-term power dispatch schedule for a wind farm incorporated with dual-battery energy storage scheme. IEEE Trans Sustain Energy 3:74–84. https://doi.org/10.1109/TSTE.2011.2163092
Zhang X, Yuan Y, Hua L, Cao Y, Qian K (2017) On generation schedule tracking of wind farms with battery energy storage systems. IEEE Trans Sustain Energy 8:341–353. https://doi.org/10.1109/TSTE.2016.2598823
Barnes AK, Balda JC, Escobar-Mejia A (2015) A semi-markov model for control of energy storage in utility grids and microgrids with PV generation. IEEE Trans Sustain Energy 6:546–556. https://doi.org/10.1109/TSTE.2015.2393353
Wang G, Ciobotaru M, Agelidis VG (2014) Power smoothing of large solar PV plant using hybrid energy storage. IEEE Trans Sustain Energy 5:834–842. https://doi.org/10.1109/TSTE.2014.2305433
Zhang Z, Wang J, Wang X (2015) An improved charging/discharging strategy of lithium batteries considering depreciation cost in day-ahead microgrid scheduling. Energy Convers Manag 105:675–684. https://doi.org/10.1016/j.enconman.2015.07.079
Feng X, Gooi HB, Chen S (2015) Capacity fade-based energy management for lithium-ion batteries used in PV systems. Electr Power Syst Res 129:150–159. https://doi.org/10.1016/j.epsr.2015.08.011
Mazzola S, Vergara C, Astolfi M, Li V, Perez-Arriaga I, Macchi E (2017) Assessing the value of forecast-based dispatch in the operation of off-grid rural microgrids. Renew Energy 108:116–125. https://doi.org/10.1016/j.renene.2017.02.040
Comodi G, Giantomassi A, Severini M, Squartini S, Ferracuti F, Fonti A et al (2015) Multi-apartment residential microgrid with electrical and thermal storage devices: experimental analysis and simulation of energy management strategies. Appl Energy 137:854–866. https://doi.org/10.1016/j.apenergy.2014.07.068
Hanna R, Kleissl J, Nottrott A, Ferry M (2014) Energy dispatch schedule optimization for demand charge reduction using a photovoltaic-battery storage system with solar forecasting. Sol Energy 103:269–287. https://doi.org/10.1016/j.solener.2014.02.020
Teleke S, Baran ME, Bhattacharya S, Huang AQ (2010) Optimal control of battery energy storage for wind farm dispatching. IEEE Trans Energy Convers 25:787–794. https://doi.org/10.1109/TEC.2010.2041550
Yuan Y, Zhang X, Ju P, Qian K, Fu Z (2012) Applications of battery energy storage system for wind power dispatchability purpose. Electr Power Syst Res 93:54–60. https://doi.org/10.1016/j.epsr.2012.07.008
Jiang Q, Gong Y, Wang H (2013) A battery energy storage system dual-layer control strategy for mitigating wind farm fluctuations. IEEE Trans Power Syst 28:3263–3273. https://doi.org/10.1109/TPWRS.2013.2244925
Baziar A, Kavousi-Fard A (2013) Considering uncertainty in the optimal energy management of renewable micro-grids including storage devices. Renew Energy 59:158–166. https://doi.org/10.1016/j.renene.2013.03.026
Lei Z, Yaoyu L (2013) Optimal energy management of wind-battery hybrid power system with two-scale dynamic programming. IEEE Trans Sustain Energy 4:765–773. https://doi.org/10.1109/TSTE.2013.2246875
Abdulla K, De Hoog J, Muenzel V, Suits F, Steer K, Wirth A et al (2016) Optimal operation of energy storage systems considering forecasts and battery degradation. IEEE Trans Smart Grid. https://doi.org/10.1109/TSG.2016.2606490
Wang Y, Dvorkin Y, Fernandez-Blanco R, Xu B, Qiu T, Kirschen D (2017) Look-ahead bidding strategy for energy storage. IEEE Trans Sustain Energy. https://doi.org/10.1109/TSTE.2017.2656800
Li T, Dong M (2016) Real-time residential-side joint energy storage management and load scheduling with renewable integration. IEEE Trans Smart Grid. https://doi.org/10.1109/TSG.2016.2550500
Sigrist L, Lobato E, Rouco L (2013) Energy storage systems providing primary reserve and peak shaving in small isolated power systems: an economic assessment. Int J Electr Power Energy Syst 53:675–683. https://doi.org/10.1016/j.ijepes.2013.05.046
Miranda I, Silva N, Leite H (2016) A holistic approach to the integration of battery energy storage systems in island electric grids with high wind penetration. IEEE Trans Sustain Energy 7:775–785. https://doi.org/10.1109/TSTE.2015.2497003
Kazemi M, Zareipour H, Amjady N, Rosehart WD, Ehsan M (2017) Operation scheduling of battery storage systems in joint energy and ancillary services markets. IEEE Trans Sustain Energy. https://doi.org/10.1109/TSTE.2017.2706563
Kazemi M, Zareipour H (2017) Long-term scheduling of battery storage systems in energy and regulation markets considering battery’s lifespan. IEEE Trans Smart Grid. https://doi.org/10.1109/TSG.2017.2724919
Riffonneau Y, Bacha S, Barruel F, Delaille A (2009) Energy flow management in grid connected PV systems with storage - a deterministic approach. In: 2009 IEEE international conference on industrial technology. IEEE, pp 1–6. https://doi.org/10.1109/ICIT.2009.4939609
Mariaud A, Acha S, Ekins-Daukes N, Shah N, Markides CN (2017) Integrated optimisation of photovoltaic and battery storage systems for UK commercial buildings. Appl Energy 199:466–478. https://doi.org/10.1016/j.apenergy.2017.04.067
Zhou B, Liu X, Cao Y, Li C, Chung CY, Chan KW (2016) Optimal scheduling of virtual power plant with battery degradation cost. IET Gener Transm Distrib 10:712–725. https://doi.org/10.1049/iet-gtd.2015.0103
Mallol-Poyato R, Salcedo-Sanz S, Jiménez-Fernández S, Díaz-Villar P (2015) Optimal discharge scheduling of energy storage systems in MicroGrids based on hyper-heuristics. Renew Energy 83:13–24. https://doi.org/10.1016/j.renene.2015.04.009
Jen-Hao T, Shang-Wen L, Dong-Jing L, Yong-Qing H (2013) Optimal charging/discharging scheduling of battery storage systems for distribution systems interconnected with sizeable PV generation systems. IEEE Trans Power Syst 28:1425–1433. https://doi.org/10.1109/TPWRS.2012.2230276
Zheng M, Meinrenken CJ, Lackner KS (2015) Smart households: dispatch strategies and economic analysis of distributed energy storage for residential peak shaving. Appl Energy 147:246–257. https://doi.org/10.1016/j.apenergy.2015.02.039
Brenna M, Foiadelli F, Longo M, Zaninelli D (2016) Energy storage control for dispatching photovoltaic power. IEEE Trans Smart Grid. https://doi.org/10.1109/TSG.2016.2611999
Alam MJE, Muttaqi KM, Sutanto D (2014) A novel approach for ramp-rate control of solar PV using energy storage to mitigate output fluctuations caused by cloud passing. IEEE Trans Energy Convers 29:507–518. https://doi.org/10.1109/TEC.2014.2304951
Abedlrazek SA, Kamalasadan S (2016) A weather based optimal storage management algorithm for PV capacity firming. IEEE Trans Ind Appl. https://doi.org/10.1109/TIA.2016.2598139
Hansang L, Byoung Yoon S, Sangchul H, Seyong J, Byungjun P, Gilsoo J (2012) Compensation for the power fluctuation of the large scale wind farm using hybrid energy storage applications. IEEE Trans Appl Supercond 22:5701904. https://doi.org/10.1109/TASC.2011.2180881
Teleke S, Baran ME, Bhattacharya S, Huang AQ (2010) Rule-based control of battery energy storage for dispatching intermittent renewable sources. IEEE Trans Sustain Energy 1(2):117–124. https://doi.org/10.1109/TSTE.2010.2061880
Bo L, Shahidehpour M (2005) Short-term scheduling of battery in a grid-connected PV/battery system. IEEE Trans Power Syst 20:1053–1061. https://doi.org/10.1109/TPWRS.2005.846060
Osório GJ, Rodrigues EMG, Lujano-Rojas JM, Matias JCO, Catalão JPS (2015) New control strategy for the weekly scheduling of insular power systems with a battery energy storage system. Appl Energy 154:459–470. https://doi.org/10.1016/j.apenergy.2015.05.048
Shuai H, Fang J, Ai X, Wen J, He H (2019) Optimal real-time operation strategy for microgrid: an ADP-based stochastic nonlinear optimization approach. IEEE Trans Sustain Energy 10:931–942. https://doi.org/10.1109/TSTE.2018.2855039
Wang B, Zhang C, Dong ZY (2020) Interval optimization based coordination of demand response and battery energy storage system considering SOC management in a microgrid. IEEE Trans Sustain Energy 11:2922–2931. https://doi.org/10.1109/TSTE.2020.2982205
Abu Abdullah M, Muttaqi KM, Sutanto D, Agalgaonkar AP (2015) An effective power dispatch control strategy to improve generation schedulability and supply reliability of a wind farm using a battery energy storage system. IEEE Trans Sustain Energy 6:1093–1102. https://doi.org/10.1109/TSTE.2014.2350980
Zhang X, Bao J, Wang R, Zheng C, Skyllas-Kazacos M (2016) Dissipativity based distributed economic model predictive control for residential microgrids with renewable energy generation and battery energy storage. Renew Energy. https://doi.org/10.1016/j.renene.2016.05.006
Sandgani MR, Sirouspour S (2017) Coordinated optimal dispatch of energy storage in a network of grid-connected microgrids. IEEE Trans Sustain Energy 8:1166–1176. https://doi.org/10.1109/TSTE.2017.2664666
Lujano-Rojas JM, Dufo-López R, Bernal-Agustín JL, Catalão JPS (2017) Optimizing daily operation of battery energy storage systems under real-time pricing schemes. IEEE Trans Smart Grid 8:316–330. https://doi.org/10.1109/TSG.2016.2602268
Al Essa MJM (2018) Management of charging cycles for grid-connected energy storage batteries. J Energy Storage 18:380–388. https://doi.org/10.1016/j.est.2018.05.019
Zhu Y, Zhao D, Li X, Wang D (2019) Control-limited adaptive dynamic programming for multi-battery energy storage systems. IEEE Trans Smart Grid 10:4235–4244. https://doi.org/10.1109/TSG.2018.2854300
Zeynali S, Rostami N, Ahmadian A, Elkamel A (2020) Two-stage stochastic home energy management strategy considering electric vehicle and battery energy storage system: an ANN-based scenario generation methodology. Sustain Energy Technol Assess 39:100722. https://doi.org/10.1016/j.seta.2020.100722
Nguyen S, Peng W, Sokolowski P, Alahakoon D, Yu X (2020) Optimizing rooftop photovoltaic distributed generation with battery storage for peer-to-peer energy trading. Appl Energy 228:2567–2580. https://doi.org/10.1016/j.apenergy.2018.07.042
Zhang N, Leibowicz BD, Hanasusanto GA (2020) Optimal residential battery storage operations using robust data-driven dynamic programming. IEEE Trans Smart Grid 11:1771–1780. https://doi.org/10.1109/TSG.2019.2942932
Nath S, Wu J (2020) Online battery scheduling for grid-connected photo-voltaic systems. J Energy Storage 31:101713. https://doi.org/10.1016/j.est.2020.101713
Riffonneau Y, Bacha S, Barruel F, Ploix S (2011) Optimal power flow management for grid connected PV systems with batteries. IEEE Trans Sustain Energy 2:309–320. https://doi.org/10.1109/TSTE.2011.2114901
Sani Hassan A, Cipcigan L, Jenkins N (2017) Optimal battery storage operation for PV systems with tariff incentives. Appl Energy 203:422–441. https://doi.org/10.1016/j.apenergy.2017.06.043
Khalilpour R, Vassallo A (2016) Planning and operation scheduling of PV-battery systems: a novel methodology. Renew Sustain Energy Rev 53:194–208. https://doi.org/10.1016/j.rser.2015.08.015
Li T, Dong M (2015) Real-time energy storage management with renewable integration: finite-time horizon approach. IEEE J Sel Areas Commun 33:2524–2539. https://doi.org/10.1109/JSAC.2015.2481212
Yuan Y, Zhang X, Ju P, Li Q, Qian K, Fu Z (2014) Determination of economic dispatch of wind farm-battery energy storage system using Genetic Algorithm. Int Trans Electr Energy Syst 24:264–280. https://doi.org/10.1002/etep.1696
Correa-florez CA, Gerossier A, Michiorri A, Kariniotakis G (2018) Stochastic operation of home energy management systems including battery cycling. Appl Energy 225:1205–1218. https://doi.org/10.1016/j.apenergy.2018.04.130
Hossain A, Roy H, Squartini S, Zaman F (2019) Energy scheduling of community microgrid with battery cost using particle swarm optimization. Appl Energy 254:113723. https://doi.org/10.1016/j.apenergy.2019.113723
Cao J, Harrold D, Fan Z, Morstyn T, Healey D, Li K (2020) Deep reinforcement learning-based energy storage arbitrage with accurate lithium-ion battery degradation model. IEEE Trans Smart Grid 11:4513–4521. https://doi.org/10.1109/TSG.2020.2986333
Kim JS, Yang H, Choi SG (2017) Distributed real-time stochastic optimization based ESS management strategy for residential customers. In: 2017 19th International conference on advanced communication technology 2017, pp 321–325. https://doi.org/10.23919/ICACT.2017.7890107
Maheshwari A, Paterakis NG, Santarelli M, Gibescu M (2020) Optimizing the operation of energy storage using a non-linear lithium-ion battery degradation model. Appl Energy 261:114360. https://doi.org/10.1016/j.apenergy.2019.114360
Arteaga J, Zareipour H (2019) A price-maker/price-taker model for the operation of battery storage systems in electricity markets. IEEE Trans Smart Grid 10:6912–6920. https://doi.org/10.1109/TSG.2019.2913818
Zhang YJ, Zhao C, Tang W, Low SH (2016) Profit maximizing planning and control of battery energy storage systems for primary frequency control. IEEE Trans Smart Grid. https://doi.org/10.1109/TSG.2016.2562672
Bitaraf H, Rahman S (2017) Reducing curtailed wind energy through energy storage and demand response. IEEE Trans Sustain Energy. https://doi.org/10.1109/TSTE.2017.2724546
Zhang C, Xu Y, Dong ZY, Ma J (2016) Robust operation of microgrids via two-stage coordinated energy storage and direct load control. IEEE Trans Power Syst. https://doi.org/10.1109/TPWRS.2016.2627583
Barsali S, Giglioli R, Lutzemberger G, Poli D, Valenti G (2017) Optimised operation of storage systems integrated with MV photovoltaic plants, considering the impact on the battery lifetime. J Energy Storage 12:178–185. https://doi.org/10.1016/j.est.2017.05.003
He G, Chen Q, Kang C, Xia Q, Poolla K (2017) Cooperation of wind power and battery storage to provide frequency regulation in power markets. IEEE Trans Power Syst 32:3559–3568. https://doi.org/10.1109/TPWRS.2016.2644642
Zafar R, Ravishankar J, Fletcher JE, Pota HR (2020) Multi-timescale voltage stability-constrained Volt/VAR optimization with battery storage system in distribution grids. IEEE Trans Sustain Energy 11:868–878. https://doi.org/10.1109/TSTE.2019.2910726
Darcovich K, Kenney B, MacNeil DD, Armstrong MM (2015) Control strategies and cycling demands for Li-ion storage batteries in residential micro-cogeneration systems. Appl Energy 141:32–41. https://doi.org/10.1016/j.apenergy.2014.11.079
Leadbetter J, Swan L (2012) Battery storage system for residential electricity peak demand shaving. Energy Build 55:685–692. https://doi.org/10.1016/j.enbuild.2012.09.035
Teki VK, Maharana MK, Panigrahi CK (2020) Study on home energy management system with battery storage for peak load shaving. Mater Today Proc. https://doi.org/10.1016/j.matpr.2020.08.377
Uddin M, Romlie MF, Abdullah MF, Tan C, Sha GM, Bakar AHA (2020) A novel peak shaving algorithm for islanded microgrid using battery energy storage system. Energy 196:1–13. https://doi.org/10.1016/j.energy.2020.117084
Samanta H, Bhattacharjee A, Pramanik M, Das A (2020) Internet of Things based smart energy management in a vanadium redox flow battery storage integrated bio-solar microgrid. J Energy Storage 32:101967. https://doi.org/10.1016/j.est.2020.101967
Taylor Z, Akhavan-Hejazi H, Cortez E, Alvarez L, Ula S, Barth M et al (2019) Customer-side SCADA-assisted large battery operation optimization for distribution feeder peak load shaving. IEEE Trans Smart Grid 10:992–1004. https://doi.org/10.1109/TSG.2017.2757007
Cai Z, Bussar C, Stöcker P, Moraes L, Magnor D, Leuthold M et al (2015) Application of battery storage for compensation of forecast errors of wind power generation in 2050. Energy Proc 73:208–17. https://doi.org/10.1016/j.egypro.2015.07.673
Reihani E, Sepasi S, Roose LR, Matsuura M (2016) Energy management at the distribution grid using a battery energy storage system (BESS). Int J Electr Power Energy Syst 77:337–344. https://doi.org/10.1016/j.ijepes.2015.11.035
Kou P, Gao F, Guan X (2015) Stochastic predictive control of battery energy storage for wind farm dispatching: using probabilistic wind power forecasts. Renew Energy 80:286–300. https://doi.org/10.1016/j.renene.2015.02.001
Abbey C, Strunz K, Joos G (2009) A knowledge-based approach for control of two-level energy storage for wind energy systems. IEEE Trans Energy Convers 24:539–547. https://doi.org/10.1109/TEC.2008.2001453
Dan W, Shaoyun G, Hongjie J, Chengshan W, Yue Z, Ning L et al (2014) A demand response and battery storage coordination algorithm for providing microgrid tie-line smoothing services. IEEE Trans Sustain Energy 5:476–486. https://doi.org/10.1109/TSTE.2013.2293772
Jae Woong S, Youngho C, Seog-Joo K, Sang Won M, Kyeon H (2013) Synergistic control of SMES and battery energy storage for enabling dispatchability of renewable energy sources. IEEE Trans Appl Supercond 23:5701205. https://doi.org/10.1109/TASC.2013.2241385
Jannati M, Hosseinian SH, Vahidi B, Li G (2016) ADALINE (ADAptive Linear NEuron)-based coordinated control for wind power fluctuations smoothing with reduced BESS (battery energy storage system) capacity. Energy 101:1–8. https://doi.org/10.1016/j.energy.2016.01.100
Dicorato M, Forte G, Pisani M, Trovato M (2012) Planning and operating combined wind-storage system in electricity market. IEEE Trans Sustain Energy 3:209–217. https://doi.org/10.1109/TSTE.2011.2179953
Teleke S, Baran ME, Huang AQ, Bhattacharya S, Anderson L (2009) Control strategies for battery energy storage for wind farm dispatching. IEEE Trans Energy Convers 24:725–732. https://doi.org/10.1109/TEC.2009.2016000
Teleke S, Baran ME, Bhattacharya S, Huang AQ (2010) Rule-based control of battery energy storage for dispatching intermittent renewable sources. IEEE Trans Sustain Energy 1:117–124. https://doi.org/10.1109/TSTE.2010.2061880
Arifujjaman M (2015) A comprehensive power loss, efficiency, reliability and cost calculation of a 1 MW/500 kWh battery based energy storage system for frequency regulation application. Renew Energy 74:158–169. https://doi.org/10.1016/j.renene.2014.07.046
Liu W, Liu Y (2020) Hierarchical model predictive control of wind farm with energy storage system for frequency regulation during black-start. Electr Power Energy Syst 119:105893. https://doi.org/10.1016/j.ijepes.2020.105893
Boyle J, Littler T, Foley A (2020) Battery energy storage system state-of-charge management to ensure availability of frequency regulating services from wind farms. Renew Energy 160:1119–1135. https://doi.org/10.1016/j.renene.2020.06.025
Engels J, Claessens B, Deconinck G (2020) Optimal combination of frequency control and peak shaving with battery storage systems. IEEE Trans Smart Grid 11:3270–3279. https://doi.org/10.1109/TSG.2019.2963098
Krata J, Saha TK (2019) Real-time coordinated voltage support with battery energy storage in a distribution grid equipped with medium-scale PV generation. IEEE Trans Smart Grid 10:3486–3497. https://doi.org/10.1109/TSG.2018.2828991
Xu Y, Hu J, Gu W, Su W, Liu W (2016) Real-time distributed control of battery energy storage systems for security constrained DC-OPF. IEEE Trans Smart Grid. https://doi.org/10.1109/TSG.2016.2593911
Zhu Y, Liu C, Sun K, Shi D, Wang Z (2019) Optimization of battery energy storage to improve power system oscillation damping. IEEE Trans Sustain Energy 10(2):1015–1024. https://doi.org/10.1109/TSTE.2018.2858262
Shayeghi H, Monfaredi F, Dejamkhooy A, Sha M, Catalão JPS (2021) Assessing hybrid supercapacitor-battery energy storage for active power management in a wind-diesel system. Electr Power Energy Syst. https://doi.org/10.1016/j.ijepes.2020.106391
Georgiou GS, Christodoulides P, Kalogirou SA (2020) Optimizing the energy storage schedule of a battery in a PV grid-connected nZEB using linear programming. Energy 208:118177. https://doi.org/10.1016/j.energy.2020.118177
Mégel O, Mathieu JL, Andersson G (2015) Scheduling distributed energy storage units to provide multiple services under forecast error. Int J Electr Power Energy Syst 72:48–57. https://doi.org/10.1016/j.ijepes.2015.02.010
Grillo S, Pievatolo A, Tironi E (2016) Optimal storage scheduling using Markov decision processes. IEEE Transactions on Sustainable Energy 7(2):755–764. https://doi.org/10.1109/TSTE.2015.2497718
Böcker B, Kippelt S, Weber C, Rehtanz C (2017) Storage valuation in congested grids. IEEE Trans Smart Grid. https://doi.org/10.1109/TSG.2017.2721982
Malysz P, Sirouspour S, Emadi A (2014) An optimal energy storage control strategy for grid-connected microgrids. IEEE Trans Smart Grid 5:1785–1796. https://doi.org/10.1109/TSG.2014.2302396
Moradi H, Esfahanian M, Abtahi A, Zilouchian A (2018) Optimization and energy management of a standalone hybrid microgrid in the presence of a battery storage system: Battery State of Charge Distributed Energy resources. Energy 147:226–238. https://doi.org/10.1016/j.energy.2018.01.016
Engels J, Claessens B, Deconinck G (2019) Combined stochastic optimization of frequency control and self-consumption with a battery. IEEE Trans Smart Grid 10:1971–1981. https://doi.org/10.1109/TSG.2017.2785040
Olivella-Rosell P, Rullan F, Lloret-Gallego P, Prieto-Araujo E, Ferrer-San-José R, Barja-Martinez S et al (2020) Centralized and distributed optimization for aggregated flexibility services provision. IEEE Trans Smart Grid 11:3257–3269. https://doi.org/10.1109/TSG.2019.2962269
Liang Z, Chen H, Wang X, Chen S, Zhang C (2020) Risk-based uncertainty set optimization method for energy management of hybrid AC/DC microgrids with uncertain renewable generation. IEEE Trans Smart Grid 11:1526–1542. https://doi.org/10.1109/TSG.2019.2939817
Kusakana K (2020) Optimal peer-to-peer energy management between grid-connected prosumers with battery storage and photovoltaic systems. J Energy Storage 32:101717. https://doi.org/10.1016/j.est.2020.101717
Xu X, Hu W, Cao D, Huang Q, Liu Z, Liu W et al (2020) Scheduling of wind-battery hybrid system in the electricity market using distributionally robust optimization. Renew Energy 156:47–56. https://doi.org/10.1016/j.renene.2020.04.057
Duong T, Khambadkone AM (2013) Energy management for lifetime extension of energy storage system in micro-grid applications. IEEE Trans Smart Grid 4:1289–1296. https://doi.org/10.1109/TSG.2013.2272835
Zou J, Peng C, Shi J, Xin X, Zhang Z (2015) State-of-charge optimising control approach of battery energy storage system for wind farm. IET Renew Power Gener 9:647–652. https://doi.org/10.1049/iet-rpg.2014.0202
Saxena V, Kumar N, Nangia U (2023) An extensive data-based assessment of optimization techniques for distributed generation allocation: conventional to modern. Arch Comput Methods Eng 30:675–701. https://doi.org/10.1007/s11831-022-09812-w
Bo Z, Xuesong Z, Jian C, Caisheng W, Li G (2013) Operation optimization of standalone microgrids considering lifetime characteristics of battery energy storage system. IEEE Trans Sustain Energy 4:934–943. https://doi.org/10.1109/TSTE.2013.2248400
Tan X, Wu Y, Tsang DHK (2016) Pareto optimal operation of distributed battery energy storage systems for energy arbitrage under dynamic pricing. IEEE Trans Parallel Distrib Syst 27:2103–2115. https://doi.org/10.1109/TPDS.2015.2478785
Zhang F, Wang G, Meng K, Zhao J, Xu Z, Dong ZY et al (2016) Improved cycle control and sizing scheme for wind energy storage system based on multi-objective optimization. IEEE Trans Sustain Energy. https://doi.org/10.1109/TSTE.2016.2636878
Ju C, Wang P, Goel L, Xu Y (2017) A two-layer energy management system for microgrids with hybrid energy storage considering degradation costs. IEEE Trans Smart Grid. https://doi.org/10.1109/TSG.2017.2703126
Purvins A, Sumner M (2013) Optimal management of stationary lithium-ion battery system in electricity distribution grids. J Power Sources 242:742–755. https://doi.org/10.1016/j.jpowsour.2013.05.097
Wächter A, Biegler LT (2006) On the implementation of an interior-point filter line-search algorithm for large-scale nonlinear programming. Math Program 106:25–57. https://doi.org/10.1007/s10107-004-0559-y
Gurobi optimizer reference manual (n.d.) http://www.gurobi.com/. Accessed 23 Mar 2018
Bellman R (1952) The theory of dynamic programming. Proc Natl Acad Sci USA 60:503–515
Firoozian R (2009) State variable feedback control theory. In: Firoozian R (ed) Servo motors and industrial control theory. Springer, Boston, pp 43–58. https://doi.org/10.1007/978-0-387-85460-1_3
Sadeghi J, Sadeghi S, Niaki STA (2014) Optimizing a hybrid vendor-managed inventory and transportation problem with fuzzy demand: an improved particle swarm optimization algorithm. Inf Sci 272:126–144. https://doi.org/10.1016/j.ins.2014.02.075
Bonyadi MR, Michalewicz Z (2017) Particle swarm optimization for single objective continuous space problems: a review. Evol Comput 25:1–54. https://doi.org/10.1162/EVCO_r_00180
Athari MH, Ardehali MM (2016) Operational performance of energy storage as function of electricity prices for on-grid hybrid renewable energy system by optimized fuzzy logic controller. Renew Energy 85:890–902. https://doi.org/10.1016/j.renene.2015.07.055
Afzali P, Anjom N, Rashidinejad M, Bakhshai A (2020) Techno-economic study driven based on available efficiency index for optimal operation of a smart grid in the presence of energy storage system. J Energy Storage 32:101853. https://doi.org/10.1016/j.est.2020.101853
Zheng QP, Wang J, Liu AL (2015) Stochastic optimization for unit commitment: a review. IEEE Trans Power Syst 30:1913–1924. https://doi.org/10.1109/TPWRS.2014.2355204
Bhardwaj A, Vikram Kumar K, Vijay Kumar S, Singh B, Khurana P (2012) Unit commitment in electrical power system-a literature review. In: 2012 IEEE international power engineering and optimization conference, Malaysia, pp 275–280. https://doi.org/10.1109/PEOCO.2012.6230874
Tran D, Zhou H, Khambadkone AM (2010) Energy management and dynamic control in Composite Energy Storage System for micro-grid applications. In: IECON 2010—36th annual conference of the IEEE Industrial Electronics Society, pp 1818–1824. https://doi.org/10.1109/IECON.2010.5675399
Saxena V, Kumar N, Nangia U (2022) An impact assessment of distributed generation in distribution network. In: Pandit M, Gaur MK, Rana PS, Tiwari A (eds) Artificial Intelligence and Sustainable Computing. Algorithms for Intelligent Systems. Springer, Singapore. https://doi.org/10.1007/978-981-19-1653-3_26
Saxena V, Kumar N, Nangia U (2021) Analysis of smart electricity grid framework unified with renewably distributed generation. In: Agrawal R, Kishore Singh C, Goyal A (eds) Advances in Smart Communication and Imaging Systems, vol 721. Lecture Notes in Electrical Engineering. Springer, Singapore. https://doi.org/10.1007/978-981-15-9938-5_68
Gabash A, Pu L (2013) Flexible optimal operation of battery storage systems for energy supply networks. IEEE Trans Power Syst 28:2788–2797. https://doi.org/10.1109/TPWRS.2012.2230277
Avril S, Arnaud G, Florentin A, Vinard M (2010) Multi-objective optimization of batteries and hydrogen storage technologies for remote photovoltaic systems. Energy 35:5300–5308. https://doi.org/10.1016/j.energy.2010.07.033
Kumar M, Diwania S, Sen S et al (2023) Emission-averse techno-economical study for an isolated microgrid system with solar energy and battery storage. Electr Eng 105:1883–1896. https://doi.org/10.1007/s00202-023-01785-8
Kumar M, Sen S, Jain H, Diwania S (2022) Optimal planning for building integrated microgrid system (BIMGS) for economic feasibility with renewable energy support. In: 2022 IEEE 10th Power India international conference (PIICON), New Delhi, India, pp 1–6. https://doi.org/10.1109/PIICON56320.2022.10045284
Kumbhar A, Dhawale PG, Kumbhar S, Patil U, Magdum P (2021) A comprehensive review: machine learning and its application in integrated power system. Energy Rep 7:5467–5474. https://doi.org/10.1016/j.egyr.2021.08.133
Saxena V, Kumar N, Nangia U (2022) An impact assessment of distributed generation in distribution network. In: Artificial intelligence and sustainable computing. algorithms for intelligent systems. Springer, Singapore. https://doi.org/10.1007/978-981-19-1653-3_26
Al-Saffar M, Musilek P (2020) Reinforcement learning-based distributed BESS management for mitigating overvoltage issues in systems with high PV penetration. IEEE Trans Smart Grid 11:2980–2994. https://doi.org/10.1109/TSG.2020.2972208
Funding
This research received no external funding.
Author information
Authors and Affiliations
Corresponding author
Ethics declarations
Conflict of interest
The author declares no conflict of interest.
Additional information
Publisher's Note
Springer Nature remains neutral with regard to jurisdictional claims in published maps and institutional affiliations.
Rights and permissions
Springer Nature or its licensor (e.g. a society or other partner) holds exclusive rights to this article under a publishing agreement with the author(s) or other rightsholder(s); author self-archiving of the accepted manuscript version of this article is solely governed by the terms of such publishing agreement and applicable law.
About this article
Cite this article
Saxena, V., Kumar, N. & Nangia, U. Computation and Optimization of BESS in the Modeling of Renewable Energy Based Framework. Arch Computat Methods Eng (2024). https://doi.org/10.1007/s11831-023-10046-7
Received:
Accepted:
Published:
DOI: https://doi.org/10.1007/s11831-023-10046-7