Abstract
A wireless sensor networks (WSN’s) has stimulated significant research work among the researchers in monitoring and tracking tasks. It’s a quite challenging task that needs to cope up with various conflicting issues such as energy efficiency, network lifetime, connectivity, coverage, etc. in WSN’s for designing various applications. This paper explores the recent work and efforts done in addressing the various issues in WSN’s. This paper focused on basic concepts regarding the WSN’s and discusses meta-heuristics and heuristics algorithms for solving these issues with recent investigations. Various optimization algorithms in the context of WSN, routing algorithms, and clustering algorithms were discussed with details of earlier work done. This paper delivers various Multi-Objective Optimization approaches deeply for solving issues and summarizes the recent research work and studies. It provides researchers an understanding of the various issues, trade-offs between them, and meta-heuristics and heuristics approach for solving these issues. A glimpse of open research challenges has also been provided which will be helpful for researchers. This paper also gives an insight into various issues, open challenges that still exist in WSN’s with their heuristics and meta-heuristics solutions and also focuses on various conflicting issues as well.










Similar content being viewed by others
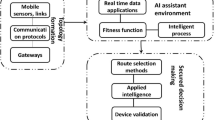
References
Fei, Z., Li, B., Yang, S., Xing, C., Chen, H., & Hanzo, L. (2016). A survey of multi-objective optimization in wireless sensor networks: Metrics, algorithms, and open problems. IEEE Communications Surveys and Tutorials, 19(1), 550–586.
Marler, R. T., & Arora, J. S. (2004). Survey of multi-objective optimization methods for engineering. Structural and Multidisciplinary Optimization, 26(6), 369–395.
Tharmarasa, R., Kirubarajan, T., & Peng, J. (2005, September). Dynamic sensor management for distributed tracking. In Signal and Data Processing of Small Targets 2005 (Vol. 5913, p. 59130Y). International Society for Optics and Photonics.
Wang, X. J., Zhang, C. Y., Gao, L., & Li, P. G. (2008). A survey and future trend of study on multi-objective scheduling. In 2008 Fourth International Conference on Natural Computation (Vol. 6, pp. 382–391). IEEE.
Marks, M. (2010). A survey of multi-objective deployment in wireless sensor networks. Journal of Telecommunications and Information Technology, 36–41.
Gao, T., Song, J. Y., Zou, J. Y., Ding, J. H., Wang, D. Q., & Jin, R. C. (2016). An overview of performance trade-off mechanisms in routing protocol for green wireless sensor networks. Wireless Networks, 22(1), 135–157.
Iqbal, M., Naeem, M., Anpalagan, A., Ahmed, A., & Azam, M. (2015). Wireless sensor network optimization: Multi-objective paradigm. Sensors, 15(7), 17572–17620.
Iqbal, M., Naeem, M., Anpalagan, A., Qadri, N. N., & Imran, M. (2016). Multi-objective optimization in sensor networks: Optimization classification, applications and solution approaches. Computer Networks, 99, 134–161.
Akyildiz, I. F., Su, W., Sankarasubramaniam, Y., & Cayirci, E. (2002). A survey on sensor networks. IEEE Communications magazine, 40(8), 102–114.
Rawat, P., Singh, K. D., Chaouchi, H., & Bonnin, J. M. (2014). Wireless sensor networks: A survey on recent developments and potential synergies. The Journal of Supercomputing, 68(1), 1–48.
Iannacci, J. (2019). Microsystem based Energy Harvesting (EH-MEMS): Powering pervasivity of the Internet of Things (IoT)–A review with focus on mechanical vibrations. Journal of King Saud University-Science, 31(1), 66–74.
Arampatzis, T., Lygeros, J., & Manesis, S. (2005). A survey of applications of wireless sensors and wireless sensor networks. In Proceedings of the 2005 IEEE International Symposium on, Mediterrean Conference on Control and Automation Intelligent Control, 2005. (pp. 719–724). IEEE.
Spachos, P., & Hatzinakos, D. (2015). Real-time indoor carbon dioxide monitoring through cognitive wireless sensor networks. IEEE Sensors Journal, 16(2), 506–514.
Milenković, A., Otto, C., & Jovanov, E. (2006). Wireless sensor networks for personal health monitoring: Issues and an implementation. Computer Communications, 29(13–14), 2521–2533.
Ketu, S., & Mishra, P. K. (2021). Cloud, Fog and Mist Computing in IoT: An Indication of Emerging Opportunities. IETE Technical Review. https://doi.org/10.1080/02564602.2021.1898482
Chinrungrueng, J., Sununtachaikul, U., & Triamlumlerd, S. (2006). A vehicular monitoring system with power-efficient wireless sensor networks. In 2006 6th International Conference on ITS Telecommunications (pp. 951–954). IEEE.
Butun, I., Morgera, S. D., & Sankar, R. (2013). A survey of intrusion detection systems in wireless sensor networks. IEEE Communications Surveys and Tutorials, 16(1), 266–282.
Wu, F. J., Kao, Y. F., & Tseng, Y. C. (2011). From wireless sensor networks towards cyber physical systems. Pervasive and Mobile Computing, 7(4), 397–413.
Anastasi, G., Conti, M., Di Francesco, M., & Passarella, A. (2009). Energy conservation in wireless sensor networks: A survey. Ad hoc Networks, 7(3), 537–568.
Wang, D., Xie, B., & Agrawal, D. P. (2008). Coverage and lifetime optimization of wireless sensor networks with gaussian distribution. IEEE Transactions on Mobile Computing, 7(12), 1444–1458.
Sohrabi, K., Gao, J., Ailawadhi, V., & Pottie, G. J. (2000). Protocols for self-organization of a wireless sensor network. IEEE Personal Communications, 7(5), 16–27.
Bai, X., Yun, Z., Xuan, D., Chen, B., & Zhao, W. (2011). Notice of Violation of IEEE Publication Principles: Optimal multiple-coverage of sensor networks. In 2011 Proceedings IEEE INFOCOM (pp. 2498–2506). IEEE.
Konstantinidis, A., Yang, K., Zhang, Q., & Zeinalipour-Yazti, D. (2010). A multi-objective evolutionary algorithm for the deployment and power assignment problem in wireless sensor networks. Computer Networks, 54(6), 960–976.
Krishnamachari, B., & Ordónez, F. (2003). Analysis of energy-efficient, fair routing in wireless sensor networks through non-linear optimization. In 2003 IEEE 58th Vehicular Technology Conference. VTC 2003-Fall (IEEE Cat. No. 03CH37484) (Vol. 5, pp. 2844–2848). IEEE.
Syarif, A., Benyahia, I., Abouaissa, A., Idoumghar, L., Sari, R. F., & Lorenz, P. (2014). Evolutionary multi-objective based approach for wireless sensor network deployment. In 2014 IEEE International Conference on Communications (ICC) (pp. 1831–1836). IEEE.
Wang, X., Xing, G., Zhang, Y., Lu, C., Pless, R., & Gill, C. (2003, November). Integrated coverage and connectivity configuration in wireless sensor networks. In Proceedings of the 1st international conference on Embedded networked sensor systems (pp. 28–39).
Xing, G., Wang, X., Zhang, Y., Lu, C., Pless, R., & Gill, C. (2005). Integrated coverage and connectivity configuration for energy conservation in sensor networks. ACM Transactions on Sensor Networks (TOSN), 1(1), 36–72.
Ammari, H. M., & Das, S. K. (2006). Coverage, connectivity, and fault tolerance measures of wireless sensor networks. In Symposium on Self-Stabilizing Systems (pp. 35–49). Springer, Berlin, Heidelberg.
Ammari, H. M., & Das, S. K. (2008). Integrated coverage and connectivity in wireless sensor networks: A two-dimensional percolation problem. IEEE Transactions on Computers, 57(10), 1423–1434.
Li, Y., Song, Y. Q., Zhu, Y. H., & Schott, R. (2010). Deploying wireless sensors for differentiated coverage and probabilistic connectivity. In 2010 IEEE Wireless Communication and Networking Conference (pp. 1–6). IEEE.
Chang, J. H., & Tassiulas, L. (2004). Maximum lifetime routing in wireless sensor networks. IEEE/ACM Transactions on Networking, 12(4), 609–619.
Rajagopalan, R., Mohan, C. K., Varshney, P., & Mehrotra, K. (2005). Multi-objective mobile agent routing in wireless sensor networks. In 2005 IEEE Congress on Evolutionary Computation (Vol. 2, pp. 1730–1737). IEEE.
Lanza-Gutierrez, J. M., & Gomez-Pulido, J. A. (2015). Assuming multiobjective metaheuristics to solve a three-objective optimisation problem for relay node deployment in wireless sensor networks. Applied Soft Computing, 30, 675–687.
Ammari, H. M., & Das, S. K. (2005). Trade-off between energy savings and source-to-sink delay in data dissemination for wireless sensor networks. In Proceedings of the 8th ACM international symposium on Modeling, analysis and simulation of wireless and mobile systems (pp. 126–133).
Ammari, H. M., & Das, S. K. (2008). A trade-off between energy and delay in data dissemination for wireless sensor networks using transmission range slicing. Computer Communications, 31(9), 1687–1704.
Zhang, J., Yan, T., & Son, S. H. (2006). Deployment strategies for differentiated detection in wireless sensor networks. In 2006 3rd Annual IEEE Communications Society on Sensor and Ad Hoc Communications and Networks (Vol. 1, pp. 316–325). IEEE.
Zou, Y., & Chakrabarty, K. (2004). Uncertainty-aware and coverage-oriented deployment for sensor networks. Journal of Parallel and Distributed Computing, 64(7), 788–798.
Zou, Y., & Chakrabarty, K. (2004). Sensor deployment and target localization in distributed sensor networks. ACM Transactions on Embedded Computing Systems (TECS), 3(1), 61–91.
Bhuiyan, M. Z. A., Wang, G., Cao, J., & Wu, J. (2013). Deploying wireless sensor networks with fault-tolerance for structural health monitoring. IEEE Transactions on Computers, 64(2), 382–395.
Patra, R. R., & Patra, P. K. (2011). Analysis of k-coverage in wireless sensor networks. International Journal of Advanced Computer Science and Applications, 2(9), 91–96.
Zhu, J., Hung, K. L., Bensaou, B., & Nait-Abdesselam, F. (2008). Rate-lifetime tradeoff for reliable communication in wireless sensor networks. Computer Networks, 52(1), 25–43.
Mo, J., & Walrand, J. (2000). Fair end-to-end window-based congestion control. IEEE/ACM Transactions on Networking, 8(5), 556–567.
Ammari, Y. M. (2009). Challenges and opportunities of connected k-covered wireless sensor networks. Studies in Computational Intelligence. Springer.
Ammari, H. M. (2013). On the energy-delay trade-off in geographic forwarding in always-on wireless sensor networks: A multi-objective optimization problem. Computer Networks, 57(9), 1913–1935.
Adnan, M., Razzaque, M. A., Ahmed, I., & Isnin, I. F. (2014). Bio-mimic optimization strategies in wireless sensor networks: A survey. Sensors, 14(1), 299–345.
Jabbar, S., Iram, R., Minhas, A. A., Shafi, I., Khalid, S., & Ahmad, M. (2013). Intelligent optimization of wireless sensor networks through bio-inspired computing: survey and future directions. International Journal of Distributed Sensor Networks, 9(2), 421084.
Saaty, T. L. (2008). Relative measurement and its generalization in decision making why pairwise comparisons are central in mathematics for the measurement of intangible factors the analytic hierarchy/network process. RACSAM-Revista de la Real Academia de Ciencias Exactas, Fisicas y Naturales Serie A Matematicas, 102(2), 251–318.
Saaty, T. L. (1990). Decision making for leaders: the analytic hierarchy process for decisions in a complex world. RWS Publications.
Ray, A., Sarkar, B., & Sanyal, S. (2009). The TOC-based algorithm for solving multiple constraint resources. IEEE Transactions on Engineering Management, 57(2), 301–309.
Wang, J. Q., Zhang, Z. T., Chen, J., Guo, Y. Z., Wang, S., Sun, S. D., & Huang, G. Q. (2013). The TOC-based algorithm for solving multiple constraint resources: A re-examination. IEEE Transactions on Engineering Management, 61(1), 138–146.
Li, C., Anavatti, S. G., & Ray, T. (2013). Analytical hierarchy process using fuzzy inference technique for real-time route guidance system. IEEE Transactions on Intelligent Transportation Systems, 15(1), 84–93.
Min, W., & Shining, L. (2010). An energy-efficient load-balanceable multipath routing algorithm based on AHP for wireless sensor networks. In 2010 IEEE International Conference on Intelligent Computing and Intelligent Systems (Vol. 2, pp. 251–256). IEEE.
Gao, T., Jin, R., Qin, J., & Wang, L. (2010). A novel node-disjoint multipath routing protocol for wireless multimedia sensor networks. In 2010 2nd International Conference on Signal Processing Systems (Vol. 1, pp. V1–790). IEEE.
Eichfelder, G. (2009). Scalarizations for adaptively solving multi-objective optimization problems. Computational Optimization and Applications, 44(2), 249.
Özcan, U., & Toklu, B. (2009). Multiple-criteria decision-making in two-sided assembly line balancing: A goal programming and a fuzzy goal programming models. Computers and Operations Research, 36(6), 1955–1965.
Tang, Y. C., & Chang, C. T. (2012). Multicriteria decision-making based on goal programming and fuzzy analytic hierarchy process: An application to capital budgeting problem. Knowledge-Based Systems, 26, 288–293.
Romero, C. (2004). A general structure of achievement function for a goal programming model. European Journal of Operational Research, 153(3), 675–686.
Wierzbicki, A. P. (1980). The use of reference objectives in multiobjective optimization. In Multiple criteria decision making theory and application (pp. 468–486). Springer.
Garcia-Sanchez, A. J., Garcia-Sanchez, F., Rodenas-Herraiz, D., & Garcia-Haro, J. (2012). On the optimization of wireless multimedia sensor networks: A goal programming approach. Sensors, 12(9), 12634–12660.
Ustun, O. (2012). Multi-choice goal programming formulation based on the conic scalarizing function. Applied Mathematical Modelling, 36(3), 974–988.
Zitzler, E., & Thiele, L. (1999). Multiobjective evolutionary algorithms: A comparative case study and the strength Pareto approach. IEEE Transactions on Evolutionary Computation, 3(4), 257–271.
Ripon, K. S. N., Tsang, C. H., & Kwong, S. (2006). Multi-objective evolutionary job-shop scheduling using jumping genes genetic algorithm. In The 2006 IEEE International Joint Conference on Neural Network Proceedings (pp. 3100–3107). IEEE.
Zhang, Z., Long, K., Wang, J., & Dressler, F. (2013). On swarm intelligence inspired self-organized networking: Its bionic mechanisms, designing principles and optimization approaches. IEEE Communications Surveys and Tutorials, 16(1), 513–537.
Pal, S. K., Bandyopadhyay, S., & Murthy, C. A. (1998). Genetic algorithms for generation of class boundaries. IEEE Transactions on Systems Man and Cybernetics Part B (Cybernetics), 28(6), 816–828.
Jourdan, D. B., & de Weck, O. L. (2004). Layout optimization for a wireless sensor network using a multi-objective genetic algorithm. In 2004 IEEE 59th Vehicular Technology Conference. VTC 2004-Spring (IEEE Cat. No. 04CH37514) (Vol. 5, pp. 2466–2470). IEEE.
Fonseca, C. M., & Fleming, P. J. (1993) Genetic Algorithms for Multiobjective Optimization: FormulationDiscussion and Generalization. In Icga (Vol. 93, No. July, pp. 416–423).
Jourdan, D. B., & de Weck, O. L. (2004). Multi-objective genetic algorithm for the automated planning of a wireless sensor network to monitor a critical facility. In Sensors, and Command, Control, Communications, and Intelligence (C3I) Technologies for Homeland Security and Homeland Defense III (Vol. 5403, pp. 565–575). International Society for Optics and Photonics.
Srinivas, N., & Deb, K. (1994). Muiltiobjective optimization using nondominated sorting in genetic algorithms. Evolutionary Computation, 2(3), 221–248.
Horn, J., Nafpliotis, N., & Goldberg, D. E. (1994). A niched Pareto genetic algorithm for multiobjective optimization. In Proceedings of the first IEEE conference on evolutionary computation. IEEE world congress on computational intelligence (pp. 82–87). Ieee.
Storn, R., & Price, K. (1997). Differential evolution–a simple and efficient heuristic for global optimization over continuous spaces. Journal of Global Optimization, 11(4), 341–359.
Das, S., & Suganthan, P. N. (2010). Differential evolution: A survey of the state-of-the-art. IEEE Transactions on Evolutionary Computation, 15(1), 4–31.
Yousefi, M., Yousefi, M., & Darus, A. N. (2012). A modified imperialist competitive algorithm for constrained optimization of plate-fin heat exchangers. Proceedings of the Institution of Mechanical Engineers, Part A: Journal of Power and Energy, 226(8), 1050–1059.
Mohammadi-Ivatloo, B., Rabiee, A., Soroudi, A., & Ehsan, M. (2012). Imperialist competitive algorithm for solving non-convex dynamic economic power dispatch. Energy, 44(1), 228–240.
Enayatifar, R., Yousefi, M., Abdullah, A. H., & Darus, A. N. (2014). A novel sensor deployment approach using multi-objective imperialist competitive algorithm in wireless sensor networks. Arabian Journal for Science and Engineering, 39(6), 4637–4650.
Wei, X., & Zhi, L. (2010). The multi-objective routing optimization of WSNs based on an improved ant colony algorithm. In 2010 6th International Conference on Wireless Communications Networking and Mobile Computing (WiCOM) (pp. 1–4). IEEE.
Karaboga, D., & Basturk, B. (2007). A powerful and efficient algorithm for numerical function optimization: Artificial bee colony (ABC) algorithm. Journal of Global Optimization, 39(3), 459–471.
Parsopoulos, K. E., & Vrahatis, M. N. (2002, March). Particle swarm optimization method in multiobjective problems. In Proceedings of the 2002 ACM Symposium on Applied Computing (pp. 603–607).
Dorigo, M., & Di Caro, G. (1999). Ant colony optimization: a new meta-heuristic. In Proceedings of the 1999 congress on evolutionary computation-CEC99 (Cat. No. 99TH8406) (Vol. 2, pp. 1470–1477). IEEE.
Dorigo, M., Birattari, M., & Stutzle, T. (2006). Ant colony optimization. IEEE Computational Intelligence Magazine, 1(4), 28–39.
Del Valle, Y., Venayagamoorthy, G. K., Mohagheghi, S., Hernandez, J. C., & Harley, R. G. (2008). Particle swarm optimization: Basic concepts, variants and applications in power systems. IEEE Transactions on Evolutionary Computation, 12(2), 171–195.
Rani, K. S. S., & Devarajan, N. (2012). Multiobjective sensor node deployment in wireless sensor networks. International Journal of Engineering Science and Technology, 4(4), 1262–1266.
Barbancho, J., León, C., Molina, F. J., & Barbancho, A. (2007). Using artificial intelligence in routing schemes for wireless networks. Computer Communications, 30(14–15), 2802–2811.
Oldewurtel, F., & Mahonen, P. (2006). Neural wireless sensor networks. In 2006 International Conference on Systems and Networks Communications (ICSNC'06) (pp. 28–28). IEEE.
Dong, S., Agrawal, P., & Sivalingam, K. (2007). Reinforcement learning based geographic routing protocol for UWB wireless sensor network. In IEEE GLOBECOM 2007-IEEE Global Telecommunications Conference (pp. 652–656). IEEE.
Rovcanin, M., De Poorter, E., Moerman, I., & Demeester, P. (2014). A reinforcement learning based solution for cognitive network cooperation between co-located, heterogeneous wireless sensor networks. Ad Hoc Networks, 17, 98–113.
Suryadevara, N. K., Mukhopadhyay, S. C., Kelly, S. D. T., & Gill, S. P. S. (2014). WSN-based smart sensors and actuator for power management in intelligent buildings. IEEE/ASME Transactions on Mechatronics, 20(2), 564–571.
Wang, K., Wang, Y., Sun, Y., Guo, S., & Wu, J. (2016). Green industrial Internet of Things architecture: An energy-efficient perspective. IEEE Communications Magazine, 54(12), 48–54.
Wang, K., Shao, Y., Shu, L., Zhu, C., & Zhang, Y. (2016). Mobile big data fault-tolerant processing for ehealth networks. IEEE Network, 30(1), 36–42.
Nayak, P., & Devulapalli, A. (2015). A fuzzy logic-based clustering algorithm for WSN to extend the network lifetime. IEEE Sensors Journal, 16(1), 137–144.
Hedetniemi, S. M., Hedetniemi, S. T., & Liestman, A. L. (1988). A survey of gossiping and broadcasting in communication networks. Networks, 18(4), 319–349.
Kulik, J., Heinzelman, W., & Balakrishnan, H. (2002). Negotiation-based protocols for disseminating information in wireless sensor networks. Wireless Networks, 8(2), 169–185.
Khanna, G., Bagchi, S., & Wu, Y. S. (2004, June). Fault tolerant energy aware data dissemination protocol in sensor networks. In International Conference on Dependable Systems and Networks, 2004 (pp. 795–804). IEEE.
Singh, S. K., Kumar, P., & Singh, J. P. (2017). A survey on successors of LEACH protocol. IEEE Access, 5, 4298–4328.
Wang, A., Yang, D., & Sun, D. (2012). A clustering algorithm based on energy information and cluster heads expectation for wireless sensor networks. Computers and Electrical Engineering, 38(3), 662–671.
Manzoor, B., Javaid, N., Rehman, O., Akbar, M., Nadeem, Q., Iqbal, A., & Ishfaq, M. (2013). Q-LEACH: A new routing protocol for WSNs. Procedia Computer Science, 19, 926–931.
Bara’a, A. A., & Khalil, E. A. (2012). A new evolutionary based routing protocol for clustered heterogeneous wireless sensor networks. Applied Soft Computing, 12(7), 1950–1957.
Barcelo, M., Correa, A., Vicario, J. L., & Morell, A. (2016). Cooperative interaction among multiple RPL instances in wireless sensor networks. Computer Communications, 81, 61–71.
Srivastava, J. R., & Sudarshan, T. S. B. (2015). A genetic fuzzy system based optimized zone based energy efficient routing protocol for mobile sensor networks (OZEEP). Applied Soft Computing, 37, 863–886.
Chen, C. W., & Weng, C. C. (2012). A power efficiency routing and maintenance protocol in wireless multi-hop networks. Journal of Systems and Software, 85(1), 62–76.
Zhang, D. G., Song, X. D., Wang, X., & Ma, Y. Y. (2015). Extended AODV routing method based on distributed minimum transmission (DMT) for WSN. AEU-International Journal of Electronics and Communications, 69(1), 371–381.
Hayes, T., & Ali, F. H. (2015). Proactive Highly Ambulatory Sensor Routing (PHASeR) protocol for mobile wireless sensor networks. Pervasive and Mobile Computing, 21, 47–61.
Singh, S., Chand, S., & Kumar, B. (2016). Energy efficient clustering protocol using fuzzy logic for heterogeneous WSNs. Wireless Personal Communications, 86(2), 451–475.
Kumar, H., Arora, H., & Singla, R. K. (2013). Energy-Aware Fisheye Routing (EA-FSR) algorithm for wireless mobile sensor networks. Egyptian Informatics Journal, 14(3), 235–238.
Wang, K., Gao, H., Xu, X., Jiang, J., & Yue, D. (2015). An energy-efficient reliable data transmission scheme for complex environmental monitoring in underwater acoustic sensor networks. IEEE Sensors Journal, 16(11), 4051–4062.
Kuila, P., & Jana, P. K. (2014). Energy efficient clustering and routing algorithms for wireless sensor networks: Particle swarm optimization approach. Engineering Applications of Artificial Intelligence, 33, 127–140.
Liu, M., Xu, S., & Sun, S. (2012). An agent-assisted QoS-based routing algorithm for wireless sensor networks. Journal of Network and Computer Applications, 35(1), 29–36.
Rao, P. S., Jana, P. K., & Banka, H. (2017). A particle swarm optimization based energy efficient cluster head selection algorithm for wireless sensor networks. Wireless Networks, 23(7), 2005–2020.
Hou, T. C., & Li, V. (1986). Transmission range control in multihop packet radio networks. IEEE Transactions on Communications, 34(1), 38–44.
Takagi, H., & Kleinrock, L. (1984). Optimal transmission ranges for randomly distributed packet radio terminals. IEEE Transactions on Communications, 32(3), 246–257.
Kranakis, E., Singh, H., & Urrutia, J. (1999). Compass routing on geometric networks. In in Proc. 11 th Canadian Conference on Computational Geometry.
Karp, B., & Kung, H. T. (2000). GPSR: Greedy perimeter stateless routing for wireless networks. In Proceedings of the 6th annual international conference on Mobile computing and networking (pp. 243–254).
Xu, Y., Heidemann, J., & Estrin, D. (2001). Geography-informed energy conservation for ad hoc routing. In Proceedings of the 7th annual international conference on Mobile computing and networking (pp. 70–84).
Yu, Y., Govindan, R., & Estrin, D. (2001). Geographical and energy aware routing: A recursive data dissemination protocol for wireless sensor networks.
He, T., Stankovic, J. A., Lu, C., & Abdelzaher, T. (2003). SPEED: A stateless protocol for real-time communication in sensor networks. In 23rd International Conference on Distributed Computing Systems, 2003. Proceedings. (pp. 46–55). IEEE.
Mann, P. S., & Singh, S. (2017). Energy efficient clustering protocol based on improved metaheuristic in wireless sensor networks. Journal of Network and Computer Applications, 83, 40–52.
Akyildiz, I. F., Vuran, M. C., & Akan, O. B. (2004, March). On exploiting spatial and temporal correlation in wireless sensor networks. In Proceedings of WiOpt (Vol. 4, pp. 71–80).
Rostami, A. S., Badkoobe, M., Mohanna, F., Hosseinabadi, A. A. R., & Sangaiah, A. K. (2018). Survey on clustering in heterogeneous and homogeneous wireless sensor networks. The Journal of Supercomputing, 74(1), 277–323.
Misra, S., Woungang, I., & Misra, S. C. (Eds.). (2009). Guide to wireless sensor networks. Springer Science & Business Media.
Rajpoot, P., & Dwivedi, P. (2021). MADM based Optimal Nodes Deployment for WSN with Optimal Coverage and Connectivity. In IOP Conference Series: Materials Science and Engineering (Vol. 1020, No. 1, p. 012003). IOP Publishing.
Rajpoot, P., & Dwivedi, P. (2020). Optimized and load balanced clustering for wireless sensor networks to increase the lifetime of WSN using MADM approaches. Wireless Networks, 26(1), 215–251.
Rajpoot, P., & Dwivedi, P. (2019). Multiple parameter based energy balanced and optimized clustering for WSN to enhance the Lifetime using MADM Approaches. Wireless Personal Communications, 106(2), 829–877.
Zhang, Y., Yang, L. T., & Chen, J. (2010). RFID and Sensor Networks, auerbach publication. International Standard Book, 978–1, 4200–7777.
Loscri, V., Morabito, G., & Marano, S. (2005). A twFo-levels hierarchy for low-energy adaptive clustering hierarchy (TL-LEACH). In IEEE vehicular technology conference (Vol. 62, No. 3, p. 1809). IEEE; 1999.
Rajpoot, P., Singh, S. H., Verma, R., Dubey, K., Pandey, S. K., & Verma, S. (2020). Multi-factor-based energy-efficient clustering and routing algorithm for WSN. Soft Computing: Theories and Applications (pp. 571–581). Springer.
Kumar, D., Aseri, T. C., & Patel, R. (2009). EEHC: Energy efficient heterogeneous clustered scheme for wireless sensor networks. Computer Communications, 32(4), 662–667.
Heinzelman, W. B. (2000). Application-specific protocol architectures for wireless networks (Doctoral dissertation, Massachusetts Institute of Technology).
Wang, W., Wang, Q., Luo, W., Sheng, M., Wu, W., & Hao, L. (2009). Leach-H: An improved routing protocol for collaborative sensing networks. In 2009 International Conference on Wireless Communications and Signal Processing (pp. 1–5). IEEE.
Ren, P., Qian, J., Li, L., Zhao, Z., & Li, X. (2010). Unequal clustering scheme based leach for wireless sensor networks. In 2010 Fourth International Conference on Genetic and Evolutionary Computing (pp. 90–93). IEEE.
Mahmood, D., Javaid, N., Mahmood, S., Qureshi, S., Memon, A. M., & Zaman, T. (2013). MODLEACH: a variant of LEACH for WSNs. In 2013 Eighth international conference on broadband and wireless computing, communication and applications (pp. 158–163). IEEE.
Singh, D., & Nayak, S. K. (2015, September). Enhanced modified LEACH (EMODLEACH) protocol for WSN. In 2015 International symposium on advanced computing and communication (ISACC) (pp. 328–333). IEEE.
Liu, J. L., & Ravishankar, C. V. (2011). LEACH-GA: Genetic algorithm-based energy-efficient adaptive clustering protocol for wireless sensor networks. International Journal of Machine Learning and Computing, 1(1), 79.
Younis, O., & Fahmy, S. (2004). HEED: A hybrid, energy-efficient, distributed clustering approach for ad hoc sensor networks. IEEE Transactions on Mobile Computing, 3(4), 366–379.
Ci, S., Guizani, M., & Sharif, H. (2007). Adaptive clustering in wireless sensor networks by mining sensor energy data. Computer Communications, 30(14–15), 2968–2975.
Gou, H., Yoo, Y., & Zeng, H. (2009). A partition-based LEACH algorithm for wireless sensor networks. In 2009 Ninth IEEE International Conference on Computer and Information Technology (Vol. 2, pp. 40–45). IEEE.
Thiagarajan, R. (2020). Energy consumption and network connectivity based on Novel-LEACH-POS protocol networks. Computer Communications, 149, 90–98.
Nguyen, N. T., Le, T. T., Nguyen, H. H., & Voznak, M. (2021). Energy-efficient clustering multi-hop routing protocol in a UWSN. Sensors, 21(2), 627.
Gupta, D., Rani, S., Ahmed, S. H., Garg, S., Piran, M. J., & Alrashoud, M. (2021). ICN-Based Enhanced Cooperative Caching for Multimedia Streaming in Resource Constrained Vehicular Environment. IEEE Transactions on Intelligent Transportation Systems.
Mishra, P. K., Verma, S. K., & Kumar, A. (2021). NSMTSEP: Neighbor Supported Modified Threshold Sensitive Stable Election Protocol for WSN. Wireless Personal Communications, 1–16.
Moussa, N., Hamidi-Alaoui, Z., & El Alaoui, A. E. B. (2020). ECRP: an energy-aware cluster-based routing protocol for wireless sensor networks. Wireless Networks, 1–14.
Vinodhini, R., & Gomathy, C. (2021). Fuzzy Based Unequal Clustering and Context-Aware Routing Based on Glow-Worm Swarm Optimization in Wireless Sensor Networks: Forest Fire Detection. Wireless Personal Communications, 1–22.
Liu, C., Wu, K., Xiao, Y., & Sun, B. (2006). Random coverage with guaranteed connectivity: Joint scheduling for wireless sensor networks. IEEE Transactions on Parallel and Distributed Systems, 17(6), 562–575.
Ok, C. S., Lee, S., Mitra, P., & Kumara, S. (2009). Distributed energy balanced routing for wireless sensor networks. Computers and Industrial Engineering, 57(1), 125–135.
Zhou, H., Wu, Y., Hu, Y., & Xie, G. (2010). A novel stable selection and reliable transmission protocol for clustered heterogeneous wireless sensor networks. Computer Communications, 33(15), 1843–1849.
Dutta, A. K., Elhoseny, M., Dahiya, V., & Shankar, K. (2020). An efficient hierarchical clustering protocol for multihop Internet of vehicles communication. Transactions on Emerging Telecommunications Technologies, 31(5), e3690.
Xu, L., Collier, R., & O’Hare, G. M. (2017). A survey of clustering techniques in WSNs and consideration of the challenges of applying such to 5G IoT scenarios. IEEE Internet of Things Journal, 4(5), 1229–1249.
Mishra, S. (2018). Financial management and forecasting using business intelligence and big data analytic tools. International Journal of Financial Engineering, 5(02), 1850011.
Mishra, S., & Triptahi, A. R. (2019). Platforms oriented business and data analytics in digital ecosystem. International Journal of Financial Engineering, 6(04), 1950036.
Mishra, S., & Tripathi, A. R. (2020). IoT platform business model for innovative management systems. International Journal of Financial Engineering (IJFE), 7(03), 1–31.
Srivastava, A., & Mishra, P. K. (2019). State-of-the-art prototypes and future propensity stem on internet of things. International Journal of Recent Technology and Engineering (IJRTE), 8(4), 2672–2683. https://doi.org/10.35940/ijrte.D7291.118419
Ketu, S., & Mishra, P. K. (2021). Enhanced Gaussian process regression-based forecasting model for COVID-19 outbreak and significance of IoT for its detection. Applied Intelligence, 51(3), 1492–1512.
Ketu, S., & Mishra, P. K. (2021). Hybrid classification model for eye state detection using electroencephalogram signals. Cognitive Neurodynamics. https://doi.org/10.1007/s11571-021-09678-x
Ketu, S., & Mishra, P. K. (2020). Performance Analysis of Machine Learning Algorithms for IoT-Based Human Activity Recognition. In Advances in Electrical and Computer Technologies (pp. 579–591). Springer.
Nguyen, L., & Nguyen, H. T. (2020). Mobility based network lifetime in wireless sensor networks: A review. Computer Networks, 174, 107236.
Sheng, J., Tang, Z., Wu, C., Ai, B., & Wang, Y. (2020). Game theory-based multi-objective optimization interference alignment algorithm for HSR 5G heterogeneous ultra-dense network. IEEE Transactions on Vehicular Technology, 69(11), 13371–13382.
Daanoune, I., Abdennaceur, B., & Ballouk, A. (2021). A comprehensive survey on LEACH-based clustering routing protocols in Wireless Sensor Networks. Ad Hoc Networks, 102409.
Selvi, M., Kumar, S. S., Ganapathy, S., Ayyanar, A., Nehemiah, H. K., & Kannan, A. (2020). An energy efficient clustered gravitational and fuzzy based routing algorithm in WSNs. Wireless Personal Communications, 1–30.
Shyjith, M. B., Maheswaran, C. P., & Reshma, V. K. (2020). Optimized and dynamic selection of cluster head using energy efficient routing protocol in WSN. Wireless Personal Communications, 1–23.
Mazinani, A., Mazinani, S. M., & Hasanabadi, S. FSCVG: A Fuzzy Semi-Distributed Clustering Using Virtual Grids in WSN. Wireless Personal Communications, 1–22.
Reddy, D. L., Puttamadappa, C., & Suresh, H. N. (2021). Merged glowworm swarm with ant colony optimization for energy efficient clustering and routing in Wireless Sensor Network. Pervasive and Mobile Computing, 71, 101338.
Pakdel, H., & Fotohi, R. (2021). A firefly algorithm for power management in wireless sensor networks (WSNs). The Journal of Supercomputing, 1–22.
Liu, X., Yu, J., Zhang, W., & Tian, H. (2021). Low-energy dynamic clustering scheme for multi-layer wireless sensor networks. Computers and Electrical Engineering, 91, 107093.
Singh, H., & Singh, D. (2021). Hierarchical clustering and routing protocol to ensure scalability and reliability in large-scale wireless sensor networks. The Journal of Supercomputing, 1–19.
Coello, C. C. (2006). Evolutionary multi-objective optimization: A historical view of the field. IEEE Computational Intelligence Magazine, 1(1), 28–36.
Konak, A., Coit, D. W., & Smith, A. E. (2006). Multi-objective optimization using genetic algorithms: A tutorial. Reliability Engineering and System Safety, 91(9), 992–1007.
Kulkarni, R. V., & Venayagamoorthy, G. K. (2010). Particle swarm optimization in wireless-sensor networks: A brief survey. IEEE Transactions on Systems, Man, and Cybernetics, Part C (Applications and Reviews), 41(2), 262–267.
Kulkarni, R. V., Förster, A., & Venayagamoorthy, G. K. (2010). Computational intelligence in wireless sensor networks: A survey. IEEE Communications Surveys and Tutorials, 13(1), 68–96.
Deif, D. S., & Gadallah, Y. (2013). Classification of wireless sensor networks deployment techniques. IEEE Communications Surveys and Tutorials, 16(2), 834–855.
Choi, W., & Das, S. K. (2005). A novel framework for energy-conserving data gathering in wireless sensor networks. In Proceedings IEEE 24th Annual Joint Conference of the IEEE Computer and Communications Societies. (Vol. 3, pp. 1985–1996). IEEE.
Suto, K., Nishiyama, H., Kato, N., & Huang, C. W. (2015). An energy-efficient and delay-aware wireless computing system for industrial wireless sensor networks. IEEE Access, 3, 1026–1035.
Abo-Zahhad, M., Sabor, N., Sasaki, S., & Ahmed, S. M. (2016). A centralized immune-Voronoi deployment algorithm for coverage maximization and energy conservation in mobile wireless sensor networks. Information Fusion, 30, 36–51.
Konstantinidis, A., & Yang, K. (2011). Multi-objective k-connected deployment and power assignment in wsns using a problem-specific constrained evolutionary algorithm based on decomposition. Computer Communications, 34(1), 83–98.
Schurgers, C., Tsiatsis, V., Ganeriwal, S., & Srivastava, M. (2002). Optimizing sensor networks in the energy-latency-density design space. IEEE Transactions on Mobile Computing, 1(1), 70–80.
Zorzi, M., & Rao, R. R. (2003). Geographic random forwarding (GeRaF) for ad hoc and sensor networks: Energy and latency performance. IEEE Transactions on Mobile Computing, 2(4), 349–365.
Yang, X., & Vaidya, N. H. (2004). A wakeup scheme for sensor networks: Achieving balance between energy saving and end-to-end delay. In Proceedings. RTAS 2004. 10th IEEE Real-Time and Embedded Technology and Applications Symposium, 2004. (pp. 19–26). IEEE.
Yu, Y., Krishnamachari, B., & Prasanna, V. K. (2004). Energy-latency tradeoffs for data gathering in wireless sensor networks. In IEEE INFOCOM 2004 (Vol. 1). IEEE.
Yu, Y., & Prasanna, V. K. (2005). Energy-balanced task allocation for collaborative processing in wireless sensor networks. Mobile Networks and Applications, 10(1), 115–131.
Yao, Y., Cao, Q., & Vasilakos, A. V. (2014). EDAL: An energy-efficient, delay-aware, and lifetime-balancing data collection protocol for heterogeneous wireless sensor networks. IEEE/ACM Transactions on Networking, 23(3), 810–823.
Borghini, M., Cuomo, F., Melodia, T., Monaco, U., & Ricciato, F. (2005). Optimal data delivery in wireless sensor networks in the energy and latency domains. In First International Conference on Wireless Internet (WICON'05) (pp. 138–145). IEEE.
Huynh, T. T., & Hong, C. S. (2006). An energy* delay efficient multi-hop routing scheme for wireless sensor networks. IEICE Transactions on Information and Systems, 89(5), 1654–1661.
Moscibroda, T., Von Rickenbach, P., & Wattenhofer, R. (2006). Analyzing the energy-latency trade-off during the deployment of sensor networks. In Infocom.
Leow, W. L., Pishro-Nik, H., & Ni, D. (2007). Delay and energy tradeoff in multi-state wireless sensor networks. In IEEE GLOBECOM 2007-IEEE Global Telecommunications Conference (pp. 1028–1032). IEEE.
Mao, J., Wu, Z., & Wu, X. (2007). A TDMA scheduling scheme for many-to-one communications in wireless sensor networks. Computer Communications, 30(4), 863–872.
Minhas, M. R., Gopalakrishnan, S., & Leung, V. C. (2009, June). Multiobjective routing for simultaneously optimizing system lifetime and source-to-sink delay in wireless sensor networks. In 2009 29th IEEE international conference on distributed computing systems workshops (pp. 123–129). IEEE.
Shahraki, A., Rafsanjani, M. K., & Saeid, A. B. (2011). A new approach for energy and delay trade-off intra-clustering routing in WSNs. Computers and Mathematics with Applications, 62(4), 1670–1676.
Dong, M., Ota, K., Liu, A., & Guo, M. (2015). Joint optimization of lifetime and transport delay under reliability constraint wireless sensor networks. IEEE Transactions on Parallel and Distributed Systems, 27(1), 225–236.
Nama, H., Chiang, M., & Mandayam, N. (2006, June). Utility-lifetime trade-off in self-regulating wireless sensor networks: A cross-layer design approach. In 2006 IEEE International Conference on Communications (Vol. 8, pp. 3511–3516). IEEE.
Zhu, J., Chen, S., Bensaou, B., & Hung, K. L. (2007). Tradeoff between lifetime and rate allocation in wireless sensor networks: A cross layer approach. In IEEE INFOCOM 2007–26th IEEE International Conference on Computer Communications (pp. 267–275). IEEE.
Chen, J., He, S., Sun, Y., Thulasiraman, P., & Shen, X. S. (2009). Optimal flow control for utility-lifetime tradeoff in wireless sensor networks. Computer Networks, 53(18), 3031–3041.
He, S., Chen, J., Xu, W., Sun, Y., Thulasiraman, P., & Shen, X. (2010). A stochastic multiobjective optimization framework for wireless sensor networks. EURASIP Journal on Wireless Communications and Networking, 2010, 1–10.
Luo, J., Iyer, A., & Rosenberg, C. (2010). Throughput-lifetime trade-offs in multihop wireless networks under an SINR-based interference model. IEEE Transactions on Mobile Computing, 10(3), 419–433.
Xie, D., Wei, W., Wang, Y., & Zhu, H. (2013). Tradeoff between throughput and energy consumption in multirate wireless sensor networks. IEEE Sensors Journal, 13(10), 3667–3676.
Liao, S., & Zhang, Q. (2014). A multiutility framework with application for studying tradeoff between utility and lifetime in wireless sensor networks. IEEE Transactions on Vehicular Technology, 64(10), 4701–4711.
Molina, G., Alba, E., & Talbi, E. G. (2008). Optimal sensor network layout using multi-objective metaheuristics. Journal of Universal Computer Science, 14(15), 2549–2565.
Jia, J., Chen, J., Chang, G., Wen, Y., & Song, J. (2009). Multi-objective optimization for coverage control in wireless sensor network with adjustable sensing radius. Computers and Mathematics with Applications, 57(11–12), 1767–1775.
Woehrle, M., Brockhoff, D., Hohm, T., & Bleuler, S. (2010). Investigating coverage and connectivity trade-offs in wireless sensor networks: The benefits of MOEAs. In Multiple criteria decision making for sustainable energy and transportation systems (pp. 211–221). Springer.
Cheng, C. T., & Chi, K. T. (2012). An analysis on the delay-aware data collection network structure using Pareto optimality. In 2012 International Conference on Cyber-Enabled Distributed Computing and Knowledge Discovery (pp. 348–352). IEEE.
Miller, M. J., Sengul, C., & Gupta, I. (2005). Exploring the energy-latency trade-off for broadcasts in energy-saving sensor networks. In 25th IEEE International Conference on Distributed Computing Systems (ICDCS'05) (pp. 17–26). IEEE.
EkbataniFard, G. H., Monsefi, R., Akbarzadeh-T, M. R., & Yaghmaee, M. H. (2010, May). A multi-objective genetic algorithm based approach for energy efficient QoS-routing in two-tiered wireless sensor networks. In IEEE 5th International Symposium on Wireless Pervasive Computing 2010 (pp. 80–85). IEEE.
Xu, W., Shi, Q., Wei, X., Ma, Z., Zhu, X., & Wang, Y. (2014). Distributed optimal rate–reliability–lifetime tradeoff in time-varying wireless sensor networks. IEEE Transactions on Wireless Communications, 13(9), 4836–4847.
Razzaque, M., Hong, C. S., & Lee, S. (2011). Data-centric multiobjective QoS-aware routing protocol for body sensor networks. Sensors, 11(1), 917–937.
Ansari, M. S., Mahani, A., & Kavian, Y. S. (2013). Energy-efficient network design via modelling: Optimal designing point for energy, reliability, coverage and end-to-end delay. IET Networks, 2(1), 11–18.
Liu, W., Qin, G., Li, S., He, J., & Zhang, X. (2015). A multiobjective evolutionary algorithm for energy-efficient cooperative spectrum sensing in cognitive radio sensor network. International Journal of Distributed Sensor Networks, 11(5), 581589.
Armenia, S., Morabito, G., & Palazzo, S. (2007, May). Analysis of location privacy/energy efficiency tradeoffs in wireless sensor networks. In International Conference on Research in Networking (pp. 215–226). Springer.
Liu, A., Zheng, Z., Zhang, C., Chen, Z., & Shen, X. (2012). Secure and energy-efficient disjoint multipath routing for WSNs. IEEE Transactions on Vehicular Technology, 61(7), 3255–3265.
Adlakha, S., Ganeriwal, S., Schurgers, C., & Srivastava, M. B. (2003). density, accuracy, delay and lifetime tradeoffs in wireless sensor networks—a multidimensional design perspective. In Proceedings of the 1st international conference on Embedded networked sensor systems (pp. 296–297).
Tynan, R., O'Hare, G. M., O'Grady, M. J., & Muldoon, C. (2009, June). EDLA tradeoffs for wireless sensor network target tracking. In 2009 29th IEEE International Conference on Distributed Computing Systems Workshops (pp. 440–446). IEEE.
Lozano-Garzon, C., & Donoso, Y. (2011, September). A multi-objective routing protocol for a wireless sensor network using a SPEA2 approach. In Proc. International Conference on Applied, Numerical and Computational Mathematics (ICANCM’11)/International Conference on Computers, Digital Communications and Computing (ICDCCC’11) (pp. 39–44).
Bandyopadhyay, S., Tian, Q., & Coyle, E. J. (2005). Spatio-temporal sampling rates and energy efficiency in wireless sensor networks. IEEE/ACM Transactions on Networking, 13(6), 1339–1352.
Tang, D., Li, T., Ren, J., & Wu, J. (2014). Cost-aware secure routing (CASER) protocol design for wireless sensor networks. IEEE Transactions on Parallel and Distributed Systems, 26(4), 960–973.
Bara’a, A. A., Khalil, E. A., Özdemir, S., & Yıldız, O. (2015). A multi-objective disjoint set covers for reliable lifetime maximization of wireless sensor networks. Wireless Personal Communications, 81(2), 819–838.
Sengupta, S., Das, S., Nasir, M. D., & Panigrahi, B. K. (2013). Multi-objective node deployment in WSNs: In search of an optimal trade-off among coverage, lifetime, energy consumption, and connectivity. Engineering Applications of Artificial Intelligence, 26(1), 405–416.
Acknowledgements
The authors are extremely grateful to the executive editor Prof Ramjee Prasad and anonymous reviewers for their critical comments and kind commendations for improvement of the manuscript.
Author information
Authors and Affiliations
Corresponding author
Additional information
Publisher's Note
Springer Nature remains neutral with regard to jurisdictional claims in published maps and institutional affiliations.
Rights and permissions
About this article
Cite this article
Srivastava, A., Mishra, P.K. A Survey on WSN Issues with its Heuristics and Meta-Heuristics Solutions. Wireless Pers Commun 121, 745–814 (2021). https://doi.org/10.1007/s11277-021-08659-x
Accepted:
Published:
Issue Date:
DOI: https://doi.org/10.1007/s11277-021-08659-x