Abstract
In this paper, based on in-depth analysis of remote multimedia images, the automatic annotation and classification of graphics are tested and analyzed by algorithms of deep learning. To reduce the time of remote multimedia image labeling and online classification, and improve efficiency, we study the use of deep learning methods to automate annotation and online classification of remote multimedia images. An image is re-labeling algorithm based on modeling the correlation of hidden feature dimensions is proposed to improve the effect of hidden feature models by modeling the correlation between hid feature dimensions. The algorithm constructs the correlation between each pair of dimensions in the hidden features through the outer product operation to form a two-dimensional interactive graph. The information in the interaction graph is refined layer by layer by using the ability of the convolutional neural network to model local features, and finally, a representation of the correlation of all dimensions in the hidden features is formed to realize the re-labeling of social images. The experimental results show that this method can utilize the hidden feature information more effectively and improve the image re-labeling results. The light-weight feature extraction network significantly reduces the number of model parameters at the expense of a small amount of detection accuracy, and the FPN pyramid structure enhances the feature characterization ability of the feature extraction network. The performance is close to that of the Yolo algorithm.









Similar content being viewed by others
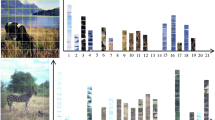
References
Al-Jarrah MA, Al-Dweik A, Kalil M et al (2018) Decision fusion in distributing cooperative wirelesses sensor networks[J]. IEEE Trans Veh Technol 68(1):797–811
Bihari A, Djenouri Y, Lin JCW et al (2020) Trajectory outlier detection: Algorithms, taxonomy, evaluation, and open challenges[J]. ACM Trans Manag Inf Syst (TMIS) 11(3):1–29
De S, Bruzzone L, Bhattacharya A et al (2017) A novel technique based on deep learning and a synthetic target database for classification of urban areas in PolSAR data[J]. IEEE J Sel Top Appl Earth Obs Remote Sens 11(1):154-170.7
Faraday A, Gross G, Nagi R et al (2016) Social network analysis with data fusion[J]. IEEE Trans Comput Soc Syst 3(2):88–99
Ghamisi P, Rasti B, Yokoya N et al (2019) Multisource and multicultural data fusion in remote sensing: A comprehensive review of the state of the art[J]. IEEE Geosci Remote Sens Mag 7(1):6–39
Haida AB, Benoit A, Lambert P et al (2018)3-D deep learning approach for remote sensing image classification[J]. IEEE Trans Geosci Remote Sens 56(8):4420–4434
Hülsmann J, Traub J, Markl V (2020)Demand-based sensor data gathering with multi-query optimization[J]. Proc VLDB Endow 13(12):2801-2804
Jalali A, Farsi H (2020) A new roentgenography algorithm based on video sparse representation[J]. Multimed Tools Appl 79(3):1821–1846
Jiang L, Yan L, Xia Y et al (2017) Asynchronous multiple multisensor data fusion over unreliable measurements with correlated noise[J]. IEEE Trans Aerosp Electron Syst 53(5):2427–2437
Li L, Ota K, Dong M (2018) Deep learning for smart industry: Efficient manufactures inspection system with fog computing[J]. IEEE Trans Industr Inform 14(10):4665–4673
Mittal N, Singh U, Salgotra R et al (2019) An energy efficient stable clustering approach using fuzzy extended grey wolfs optimization algorithm for WSNs[J]. Wirel Netw 25(8):5151–5172
Nada D, Bousbia-Salah M, Bettayeb M (2018)Multi-sensor data fusion for wheelchair position estimation with unaccented Kalman Filter[J]. Int J Autom Comput 15(2):207–217
Parcheesi M, Garcia M, Lavalle M et al (2018) A machine-learning approach to PolInSAR and LiDAR data fusion for improved tropical forest canopy height estimation using NASA AfriSAR Campaign data[J]. IEEE J Sel Top Appl Earth Obs Remote Sens 11(10):3453–3463
Pierson HA, Gashler MS (2017) Deep learning in robotics: a review of recent research[J]. Adv Robot 31(16):821–835
Rahu V, Tong C, Bhattacharya S et al (2018) Multimodal deep learning for activity and context recognition[J]. Proc ACM Interact Mob Wearable Ubiquitous Technol 1(4):1-27
Song H, Thiagarajan J, Sattigeri P et al (2018) Optimizing kernel machines using deep learning[J]. IEEE Trans Neural Netw Learn Syst 29(11):5528–5540
Wu H, Zhang Z, Jiao C et al (2019) Learn to sense: a meta-learning-based sensing and fusion framework for wireless sensor networks[J]. IEEE Internet Things J 6(5):8215–8227
Yuan X, Pu Y (2018) Parallel legless compressive imaging via deep convolutional neural networks[J]. Opt Express 26(2):1962–1977
Zappone A, Di Renzo M, Debbah M (2019) Wireless networks design in the era of deep learning: Model-based, AI-based, or both?[J]. IEEE Trans Commun 67(10):7331–7376
Zhang H, Zhou X, Wang Z et al (2018) Adaptive consensus-based distributed target tracking with dynamic cluster in sensor networks[J]. IEEE Trans Cybern 49(5):1580–1591
Zhao Z, Wang X, Wang T (2018) A novel measurement data classification algorithm based on SVM for tracking closely spaced targets[J]. IEEE Trans Instrum Meas 68(4):1089–1100
Zhou Q, Zheng Y (2020) Long link wireless sensor routing optimization based on improved adaptive ant colony algorithm[J]. Int J Wirel Inf Netw 27(2):241–252
Author information
Authors and Affiliations
Corresponding author
Additional information
Publisher’s Note
Springer Nature remains neutral with regard to jurisdictional claims in published maps and institutional affiliations.
Rights and permissions
About this article
Cite this article
Kang, S. Deep learning-based automatic annotation and online classification of remote multimedia images. Multimed Tools Appl 81, 36239–36255 (2022). https://doi.org/10.1007/s11042-021-11854-4
Received:
Revised:
Accepted:
Published:
Issue Date:
DOI: https://doi.org/10.1007/s11042-021-11854-4