Abstract
When a treatment cannot be enforced, but only encouraged, noncompliance naturally arises. In applied economics, the common empirical strategy for dealing with noncompliance is to rely on Instrumental Variables methods. When the effects are heterogeneous, these methods allow, under a set of assumptions, to identify the causal effect for Compliers, i.e., the subset of units whose treatment is affected by the encouragement. One of the identification assumptions is the Exclusion Restriction (ER), which essentially rules out the possibility of a causal effect for Never Takers, i.e., those whose treatment is not affected by the encouragement. In this paper, we show the consequences of violations of this assumption in the impact evaluation of an intervention implemented in Uganda, where targeted households were encouraged to join a community health financing (CHF) scheme through activities of sensitization. We conduct the analyses using Bayesian model-based principal stratification, first assuming and then relaxing the ER for Never Takers. This allows showing the positive impact of the intervention on the health costs of both Compliers and Never Takers. While the causal effects for the former could be due to the encouragement but also to the actual participation in the scheme, those for the latter are unequivocally attributable to the encouragement. This indicates that sensitization alone is extremely effective in reducing vulnerability against health costs. This finding is of paramount importance for policy-making, as it is much easier and more cost-effective to implement awareness-raising campaigns than CHF schemes.
Similar content being viewed by others
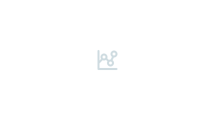
1 Introduction
In Low- and Middle-Income Countries (LMICs), direct payments often dominate health care financing and represent a serious obstacle to the achievement of Universal Health Coverage (UHC). Out-of-pocket expenditures tend to exacerbate the severity of poverty mainly among the poorest and rural populations who lack adequate financial protection (Kruk et al. 2009; Leive and Xu 2008; Wagstaff et al. 2011). Interventions of community health financing (CHF) involve the introduction of a prepayment financing mechanism aimed, on the one hand, at enhancing access to health services and, on the other, at protecting individuals from financial catastrophe due to health-related costs. Informal and rural sectors represent the main target of these interventions, where the community is actively involved in the design and management of the financing mechanism (Jütting 2004).
Several studies analyze the impact of CHF schemes on health expenditures, providing evidence that this model often presents the limitation of not including the most disadvantaged and vulnerable because they lack the financial capacity to pay the initial participation fee. Although the financial contribution provided by the scheme is thus not extended to these vulnerable individuals, the comprehension of principles of prepayment for health financing could make them rethink their health-seeking behaviors and savings practices even if they decide not to enroll. This means that there could be either beneficial or detrimental effects of CHF schemes even on those who opt out: investigating this possibility is paramount to advance the debate on effective policies for UHC in LMICs.
The analyses focus on a case study in rural Uganda, where an intervention of CHF was implemented. Households were offered to enroll in the CHF scheme upon being assigned to sensitization sessions. In this study, the participation in the CHF scheme is the treatment of interest, while the sensitization and offer to enroll are an encouragement to take the treatment. The fact that enrollment was on a voluntary basis gave rise to noncompliance, in the sense that there were households who, after being encouraged, accepted the offer to join the scheme, as well as households who declined despite the encouragement.
Nannini et al. (2021) has already conducted an impact evaluation of this intervention using an Instrumental Variables (IV) method to address the issue of noncompliance. On the one hand, this method allows, under a set of assumptions, to identify causal effects of the intervention for the subset of households whose enrollment is affected by the instrument (i.e., the sensitization and the offer to enroll in the scheme). These causal effects are attributed to the actual participation in the scheme for households that would join the scheme if encouraged to. On the other hand, it rules out the possibility of causal effects of the intervention for households whose enrollment is unaffected by the instrument, i.e., those households that would opt out even if encouraged to join the scheme. These causal effects would be attributable to the initial sensitization and offer to enroll, and could not derive from participating in the scheme.
As we previously argued, investigating the presence of effects that are not channeled by the actual participation in the scheme but instead derive from the sensitization sessions would provide important insights for policy making in the field of public health. It is here, therefore, that we make our contribution. To address the issue of noncompliance, instead of using IV methods, we use principal stratification (PS) (Frangakis and Rubin 2002). This approach allows us to define and estimate heterogeneous causal effects for specific latent subpopulations. The membership of the households in these subpopulations is determined by their joint potential enrollment in the CHF scheme under the alternative encouragement levels. More specifically, PS accounts for the presence of associative and dissociative causal effects. The former are the effects for those whose enrollment is affected by the encouragement and thus may derive from (though not only from) the participation in the scheme. The latter are the effects for those whose enrollment is unaffected by the encouragement and thus cannot derive from participating in the scheme (Frangakis and Rubin 2002). Therefore, the present paper aims to advance the analysis by identifying the distinctive characteristics of these groups and by acknowledging the possibility of causal effects of attending sensitization sessions and receiving the offer to join the CHF scheme, whether or not that offer is accepted. Bayesian inferential methods are used for inference, specifying flexible parametric models conditional on covariates and weakly informative prior distributions for the parameters.
The paper is organized as follows. Section 2 introduces the case study and the data. Section 3 describes the methodology adopted in relation to the common empirical strategy. Section 4 deepens the technical aspects of the analyses. Section 5 shows the results. Section 6 contains sensitivity analyses, and Sect. 7 concludes.
2 The intevention of community health financing
The intervention of CHF was run in Oyam by the international non-governmental organization ‘Doctors with Africa CUAMM’. The financial protection scheme has been implemented in two subcounties (Ngai and Myene), randomly selected out of a total of twelve in the Oyam district. Hereafter, we refer to these two subcounties as the intervention area.
In the intervention area, 359 community groups are present. A community group is a savings group or a mutual-help association whose purpose is to help people facing financial hardship. These groups include nearly 75–80% of the population (Biggeri et al. 2018). Since coverage of CHF can be strengthened by nesting the scheme into these existing informal groups within the community (Chemin 2018; Mladovsky et al. 2014; Sommerfeld et al. 2002), the intervention targeted 42 community groups,Footnote 1 randomly selected out of 359, including a total of 2137 households.
Targeted households first attended activities of sensitization aimed at raising awareness on the impoverishing effects of illness and on the importance of timely preparedness for unexpected health expenses; then, they received the offer to join the scheme and decided whether to enroll or not on a voluntary basis. Although the intervention is explained in detail elsewhere (Nannini et al. 2021), some important points are here reported. Those households who accepted to enroll into the scheme contributed a fixed amount (5000 UGX/year per member) to create and maintain a community fund. In case of health needs, each enrolled member could borrow money from that fund and, after receiving the necessary medical service (inpatient or outpatient care), had four months to repay with zero interest. The maximum amount that could be borrowed each time is 150,000 UGX, corresponding to the 85th percentile of the distribution of households’ health expenditures observed in a previous study (Biggeri et al. 2018). At the end of the first year of intervention, households could decide whether to renew their membership for the following year.
2.1 Study design
Data on the intervention were collected through a panel households survey conducted in the intervention area and in a third subcounty of the district (Iceme). The latter was included because interviewing households resident in a subcounty where the intervention was not implement limits the possibility of interference effects. Hereafter, we refer to this third subcounty as the control area. This area was chosen because it is similar to the two subcounties in the intervention area in terms of socioeconomic profile and distance from the main road and health facilities. To determine the sample of households that were to be interviewed, a two-stage sampling design was applied.
In the first stage, 63 villages were selected, including 62 in the intervention area and one in the control area. The 62 villages in the intervention area were chosen because they are homogeneous in terms of population density and distance from health facilities, and the community groups targeted by the intervention resided there.Footnote 2 Then, a village with the same demographic and geographical characteristics was selected in the control area.
In the second stage, among residents of these 63 villages, 282 households were randomly chosen to be survey participants, and were interviewed at the baseline (shortly before the roll-out of the intervention), in January 2019, and during the second round of the longitudinal survey, in January 2020. These households are distributed in the encouragement groups as shown in Table 1.
The sample of 282 households includes: 243 households that are part of at least one community group and were therefore eligible for the intervention,Footnote 3 and 39 households who are not part of any community group and were therefore non-eligible. Given that households that are part of at least one community group are likely to differ from households that are not part of any community group with respect to observable but also unobservable characteristics, we do not include in the analyses the latter subgroup. Thus, our final sample is composed of 243 households, who are distributed in the encouragement groups as shown in Table 2. All the causal contrasts that will be the subject of our investigation are referred to this finite sample.
2.2 Data
The survey questionnaire includes questions on a wide range of household and individual characteristics (e.g., demographic and socioeconomic factors, occurrence of negative shocks, and health-related events and perceptions). Retrospective questions consider a recall period of either one month or one year, depending on the issue. For example, questions about the household’s expenses are referred to the previous month to prevent significant recall bias, whereas questions on whether the household had taken out a loan are referred to the previous year.
In Table 3, we present some summary statistics for the 243 households in the final sample (at the baseline survey). In particular, for each covariate, the table shows (i) the sample average values by encouragement group, that we denote by \({\overline{X}}_0\) for those unencouraged and by \({\overline{X}}_1\) for those encouraged; (ii) the sample variance by encouragement group, that we denote by \(s^2_0\) and \(s^2_1\); and (iii) the normalized difference in average covariate values, i.e., \(\Delta _X = ({\overline{X}}_1 - {\overline{X}}_0)/\sqrt{(s^2_1+s^2_0)/2}\). From this Table, we can observe that, as it is often the case in small-scale field experiments, there are considerable imbalances in the covariate distributions.
Notably, while the share of health costs out of total monthly expenditures is roughly the same, on average, for the two encouragement groups, the total monthly expenditures for households in the control group are significantly higher. These households tend also to be less satisfied with the health status of their members, but nevertheless they are much less willing to pay 10,000 UGX/year per member to have a health insurance (5000 UGX is the amount required to enroll into the CHF scheme). They also experienced a considerably higher number of shocks in the previous year. Moreover, they do not have food to eat in the household more frequently, probably due to a lack of economic resources. Putting all the pieces together, households that are not encouraged to join the CHF scheme seem to be slightly more disadvantaged.
2.2.1 Outcome
As extensively outlined in Sect. 1, the main purpose of introducing interest-free loans in the context of rural Uganda was to increase the utilization of health services by the households but without the financial hardship that this would have normally caused them. In addition, this utilization of health services is expected to be more timely given that the economic barrier that delays or prevents the seeking of treatment has been lowered through the implementation of the CHF scheme. This is especially important because the timely seeking of medical consultation or treatment prevents diseases from worsening and thus improves prognosis and reduces the occurrence of catastrophic health expenditures. However, we would like to point out that, although it is desirable that, in general, healthcare costs fall, it is not necessarily a success if a household spends nothing on medical products/services. Indeed, in that case it may be that the household did not actually need them, but it may also be that they could not afford them at all. For these reasons, we decided to analyze the share of health costs over the total monthly expenditures, and to define three causal estimands as contrasts of different features of the distribution of this outcome under the two encouragement levels. The first estimand aims to measure the causal effect on the share of health costs over the total monthly expenditures; the second one on the incidence of catastrophic health expenditures; the third one on zero health expenditures. The money borrowed from the common fund does not figure as part of these monthly medical expenses, but the money being paid back with zero-interest to the common fund does. Also, health expenditures are considered catastrophic in case they amount to at least 10% of households’ monthly expenditures (Wagstaff et al. 2018).
The histograms of the empirical distribution of the share of health costs over the total monthly expenditures by encouragement group are shown in Fig. 1.
From this plot, it is evident that the distribution of the outcome for households that received sensitization, regardless of whether they enrolled in CHF scheme or not, is more shrunk towards zero, which means that the share of health expenditures tend to be lower for those who attended the sensitization sessions. In fact, for the latter the mean and the median are, respectively, 0.12 and 0.09, while the same statistics for those who did not attend the sensitization sessions amount to 0.17 and 0.12. Regarding the incidence of catastrophic health expenditures, its observed average in the group that received sensitization is 0.12 (with a standard deviation of 0.11); instead, the same quantity in the group that did not receive sensitization amounts to 0.23 (with a standard deviation of 0.15), and is thereby almost double. Finally, regarding the zero health expenditures, 45 households reported zero monthly healthcare costs at the baseline, while this number reduces to 16 after the intervention, 12 among the encouraged and 4 among the unencouraged.
On the one hand, these descriptive statistics suggest that the intervention might have the positive effect of reducing the amount of healthcare costs, and also, more specifically, the incidence of catastrophic health costs. On the other hand, it might also increase the number of households that have some healthcare expenditures in a month; as we argued before, this effect would not necessarily be detrimental.
3 Methodology
The voluntary nature of enrollment in the CHF scheme results in treated units differing from untreated units not only in the treatment itself, but also in the observed and unobserved characteristics that led them to choose to take or not take the treatment. Therefore, treatment is potentially endogenous, and an appropriate methodology is necessary to give a causal interpretation to any comparison between treated and untreated units.
3.1 Notation
Our sample consists of \(N=243\) households, indexed by \(i=1,\hdots ,N\). For each household i, let us denote the observed encouragement assignment, i.e., the assignment to the sensitization and offer to enroll, by \(Z_i \in \{0,1\}\). \(Z_i=1\) indicates that the household was assigned to the encouragement, \(Z_i=0\) that it was not. Let us also denote the treatment by \(M_i \in \{0,1\}\), where \(M_i=1\) indicates that the household enrolled in the CHF scheme, and \(M_i=0\) that it did not. As for the outcome, we indicate with \(Y_i \in [0,1)\) the share of health costs out of the total monthly expenditures. Finally, for each household i, we observe a vector of K pre-treatment covariates, \(\varvec{X}_i\).
We denote with \(\varvec{Z}\), \(\varvec{M}\) and \(\varvec{Y}\) the N-dimensional vectors of, respectively, the observed encouragement, treatment and outcome for the whole sample, with i-th elements equal to \(Z_i\), \(M_i\) and \(Y_i\). Also, we denote with \(\varvec{X}\) the \(N \times K\) matrix with i-th row equal to \(\varvec{X}_i\).
Let us now introduce the potential outcomes for the relevant post-encouragement variables. Let \(\varvec{z}\) be a N-dimensional vector of encouragement assignments, with i-th element equal to \(z_i: \varvec{z} \in \{0,1\}^N\). Then, \(M_i(\varvec{z})\) is the potential enrollment in the CHF scheme given the encouragement vector \(\varvec{z}\), and \(Y_i(\varvec{z})\) is the share of health expenditures out of the total monthly expenditures given the same encouragement vector.
We assume that the Stable Unit Value Assumption (SUTVA, Rubin 1980) holds for the potential outcomes \(M_i(\varvec{z})\) and \(Y_i(\varvec{z})\):
Assumption 1
SUTVA for \(M_i(\varvec{z})\) and \(Y_i(\varvec{z})\).
No hidden variations of encouragement: For all \(\varvec{z}, \varvec{z}'\): \(\varvec{z}=\varvec{z}',\) \(M_i(\varvec{z})=M_i(\varvec{z}')\) and \(Y_i(\varvec{z})=Y_i(\varvec{z}').\) No-interference: For all \(\varvec{z}, \varvec{z}'\): \(z_i = z_i',\) \(M_i(\varvec{z})=M_i(\varvec{z}')\) and \(Y_i(\varvec{z})=Y_i(\varvec{z}').\)
This assumption implies that no different forms or versions of the encouragement exist and that the treatment and the outcome of a given household do not vary with the encouragement of the other households. Thus, the potential outcomes can be written as \(M_i(\varvec{z})=M_i(z_i)\) and \(Y_i(\varvec{z})=Y_i(z_i)\); to simplify the notation, in the sequel we use \(M_i(z) \equiv M_i(z_i)\) and \(Y_i(z) \equiv Y_i(z_i)\). Also, under Assumption 1, the following relations hold: \(M_i = M_i(Z_i)\) and \(Y_i = Y_i(Z_i)\).
3.2 The principal stratification approach
PS was proposed by Frangakis and Rubin (2002) as a general framework for assessing the causal effects of a treatment that takes into account complications that may arise after an encouragement or a treatment has been assigned. Indeed, it allows to identify latent subgroups of subjects, called “principal strata”, defined by the joint potential values of a post-assignment variable under alternative levels of the assignment.
In our study, we classify households into principal strata based on their participation in the CHF scheme under the alternative levels of the encouragement assignment. Note that, since the possibility of enrolling was given only to households who were encouraged to do so, non-compliance is one-sided: for each household \(i=1,\hdots ,N\), \(M_i(0)=0\), while \(M_i(1) \in \{0,1\}\). Formally, let \(G_i=(M_i(0), M_i(1))\), then the principal strata are given by the possible values of \(G_i\):
We relabel the possible values of \(G_i\) as \(0m_1\), so \(G_i \in \{00,01\}\). We name these two principal strata as shown in Table 4.
On the one hand, the stratum of Compliers is made of those who, if encouraged, would decide to enroll. On the other hand, the stratum of Never Takers is made of those who would not enroll in the program even if encouraged. Unfortunately, it is only possible to observe the potential outcomes associated with the actual encouragement assignment, while the potential outcomes associated with the alternative one are missing. This is what makes the principal strata latent, in the sense that the principal strata membership is generally unknown.
3.2.1 Definition of principal causal effects
The overall effect of the intervention within a principal stratum is named Principal Causal Effect (PCE) and, for a finite sample, it is given by the average difference in the potential outcomes of the units belonging to that stratum. In this study, we are interested in three different causal effects, namely, that on the share of health costs over the total monthly expenditures, that on the incidence of catastrophic health expenditures, and that on the incidence of zero health expenditures. Therefore, we have three different PCEs for each principal stratum g (\(g \in \{00,01\)}). We name the first Health Expenditures Effect (HEE), the second Catastrophic Health Expenditures Effect (CHEE) and the third Zero Health Expenditures Effect (ZHEE). We define these PCEs as follows:
where \(\mathbbm {1}(\cdot )\) is the indicator function, taking the value 1 if its argument is true and 0 otherwise, and \(N_g\) is the total number of households in the sample belonging to principal stratum g. \(HEE_g\) is the average difference between the health costs over the total monthly expenditures under the two encouragement levels for households belonging to the stratum g. \(CHEE_g\) is given by an analogous comparison between the proportions of catastrophic health expenditures, and \(ZHEE_g\) between the proportions of zero health expenditures.
3.2.2 Identification issues
In this study, we know that those who attended the sensitization sessions and then joined the program are necessarily Compliers, because a Never Taker would never join the program by definition. Conversely, those who attended the sensitization sessions and then refused to join the program are necessarily Never Takers. What is unknown is the principal stratum membership of those households that did not attend the sensitization sessions; in fact, we cannot know whether they would have joined the program if they had received sensitization and been offered to enroll. In Table 5 we summarize the possible principal strata for each observed group.
The latent nature of the principal strata makes it necessary to introduce some assumptions to identify and estimate PCEs. One of these assumptions is the monotonicity of compliance (Imbens and Angrist 1994), which rules out the presence of units that end up refusing to enroll in the CHF scheme if encouraged, but would have enrolled if not encouraged. Formally,
Assumption 2
Monotonicity of compliance: \(M_i(1) \ge M_i(0) \quad \forall i.\)
Since noncompliance is one-sided in this study, this assumption holds by design. Therefore, to identify the PCE for Compliers, also called Compliers Average Causal Effect (CACE), the following assumptions are sufficient: the unconfoundedness of the encouragement and the exclusion restriction for Never Takers (e.g., Mealli and Mattei 2012).
The unconfoundedness of the encouragement assumption is as follows:
Assumption 3
Unconfoundedness of the encouragement: .
It amounts to assuming that, within cells defined by the values of observed pre-treatment covariates, the encouragement is independent of the potential outcomes. This assumption implies that , and thus allows for a comparison between households who are in different encouragement groups, but belong to the same principal stratum and have same values of the observed pre-treatment covariates. Assumption 3 would hold by design in a (block) randomized experiment. In this study, it is plausible because the control area has been chosen because it was similar to the intervention area in terms of characteristics that may reasonably affect the outcome variables (more details were given in Sect. 2.1). As a result, we can reasonably rely on the validity of Assumption 3, and the conditioning on a rich set of covariates makes it more plausible. This set, in fact, contains many pieces of information about household and household head characteristics, the health status of household members, as well as proxies for the financial status of the household (we will provide the full list of covariates included in \(\varvec{X}_i\) in the Sect. 4.2).
The exclusion restriction, on the other hand, rules out the presence of any direct effect of the encouragement on the outcome. Under this assumption, only the presence of an indirect effect, that is, an effect that passes through the treatment, is allowed. In this study, this amounts to assuming that attending the sensitization sessions and receiving the offer to participate in the program do not have any direct influence on the outcome, but could only have an effect that passes through the actual participation. We make this assumption for Never Takers only. As we explain in detail later, we impose it by assuming prior equality of some models’ parameters.
Assumption 4
Exclusion Restriction (ER) for Never Takers: \(Pr(Y_i(1) \vert G_i=00, \varvec{X}_i) = Pr(Y_i(0) \vert G_i=00, \varvec{X}_i)\).
Given that, for Never Takers, an effect of actually participating in the scheme cannot exist, this restriction essentially rules out the presence of any causal effect of the intervention for this principal stratum. On the contrary, a causal effect of the intervention can exist for Compliers, and can be attributable to an effect of participating in the scheme as well as to a direct effect of the encouragement.
Often ERs are controversial, and their plausibility needs to be assessed at a case-by-case level. In the application considered here, on one hand, receiving sensitization does not provide the households with additional tools to improve health financing and outcomes of financial protection; on the other hand, however, participating in sensitization sessions may lead to change in health-seeking behavior after awareness-raising. In view of this, the ER for Never Takers might not hold. For this reason, we also obtain the results without assuming it, thus allowing for the possibility that an effect the encouragement may exist also for Never Takers.
3.3 Common empirical strategy
Applied economists typically analyze data from studies characterized by noncompliance using IV methods (for a review, see Angrist and Pischke 2008). These methods were developed in the 1920s to draw causal conclusions in contexts where the treatment cannot be deemed as randomly assigned, even conditional on covariates. If the effects are heterogeneous, Imbens and Angrist (1994) provided a set of assumptions that allow identifying the causal effect for Compliers. The first is the fact that the so-called instrumental variable (here, the encouragement assignment) is either randomized or at least as good as if it was randomized. In our application, the encouragement is assumed to be unconfounded (Assumption 3), therefore this first requirement would be fulfilled. The second is the fact that the instrumental variable influences the treatment but does not affect the outcome directly. Like Assumption 4, this is an exclusion restriction. However, it also applies to Compliers, not only to Never Takers. Therefore, in addition to stating that there are not effects of the intervention for Never Takers, this amounts attributing the effect for Compliers to the participation to the CHF scheme only, and not to the sensitization received. It is worth noting, however, that this assumption only concerns the interpretation of PCE for Compliers, while its identification or estimation does not change compared to when the ER is stated for Never Takers only (e.g., Mealli and Rubin 2002; Mealli and Mattei 2012). The third assumption on which the IV methods rely is monotonicity of compliance (Assumption 2). As argued in Sect. 3.2.2, in this study this assumption is automatically fulfilled. Finally, the last condition needed for using an IV method is that the instrument must be substantially correlated with the intermediate variable. This last condition can be verified from the data: here, it is satisfied because the correlation between the attendance to the sensitization sessions and the enrollment in the CHF scheme is 0.74.
When all the underlying assumptions are satisfied, the IV estimand is equivalent to the average causal effect for Compliers (Imbens and Angrist 1994; Angrist et al. 1996). However, when the ER does not hold, the IV estimand is equal to the average causal effect for Compliers plus a bias term. The size of the latter depends on the proportion of Never Takers: the more Never Takers, the greater the bias is (Angrist et al. 1996). In any case, even in the presence of a few Never Takers, it is important to know whether there are causal effects of the sensitization for them. This would, in fact, have paramount practical implications for policy-making. First, delivering sensitization sessions is much easier and less costly than implementing CHF schemes. Hence, hypothetical future awareness-raising campaigns would easily reach many more people. Moreover, even the poorest and most vulnerable, who would not have the financial capacity to pay the initial participation fee required to join a CHF scheme, could benefit from these campaigns. Therefore, it would be paramount to think carefully about how to design them to maximize their effectiveness.
4 Analyses
4.1 Weakly identified causal effects
Without assuming the ER for Never Takers, the causal effects of interest can only be partially identified. This means that the observed data do not allow to infer their true value, but rather a set of possible values consistent with the observed data and strictly included in the parameter space. This set is called the “identified set”, and is, essentially, a region of identification of the target estimand.
Bayesian statistics is an inferential approach where any available background knowledge about the parameters in a model is updated in the light of the observed data. The background knowledge is expressed through a prior distribution while the information in the data is given by the likelihood function; the two are combined through the Bayes’ theorem to obtain the posterior distribution (for a review, see van de Schoot et al. 2021). In a Bayesian framework we have that, as the sample size goes to infinity, the marginal posterior distribution of the partially identified causal effects will converge to a non-degenerate distribution with support equal to the identified set (Gustafson 2010). Despite this issue, in finite samples if the prior distribution is proper the posterior distribution will also be proper.
To help achieve identification and sharpen the inference, various methods were proposed in the literature. Assuming the ER for one or more principal strata is probably the most widely used. An alternative is to introduce more plausible restrictions on covariates or secondary outcomes (Mattei et al. 2013; Mealli and Pacini 2013; Mealli et al. 2016). Moreover, flexible parametric models for the potential outcomes conditional on principal stratum and covariates can be specified in order to reduce the width of the large sample bounds of the causal effects (e.g. Forastiere et al. 2021). The latter is the approach that we follow here, using a Bayesian approach to inference. This usually leads to weakly identified causal effects; “identified” in the sense of having a proper posterior distribution, but “weak” in the sense that this distribution will have regions of flatness around its maximum—in frequentist terms, there are not unique maximum likelihood estimates (Hirano et al. 2000).
4.2 Models specification
A Bayesian model-based PS analysis requires the specification of two sets of models: one for the principal strata membership given the covariates and one for the potential outcomes conditional on the covariates and the principal strata.
We model the principal strata membership using a logit model:
where \(\varvec{W}_i\) is a vector of pre-treatment covariates, partially different from \(\varvec{X}_i\), entering the principal stratum model. Specifically, \(\varvec{W}_i\) includes: the share of health expenditures out of the total monthly expenditures and the total monthly expenditures prior to the intervention; whether the household head is female, her/his age and her/his literacy level; whether in the household there are members aged 5 or less; whether the main source of income is subsistence farming; whether a serious illness occurred in the household in the previous year and whether the respondent is scared about the cost of health expenditures; the number of shocks that the household experienced in the previous year; and, finally, its willingness to pay 10,000 UGX/year per household member for a health insurance. A different model could have been chosen, for instance, a probit model. However, since the model is specified as a flexible function of the covariates, we expect to obtain the same results.
As for the outcome, a common model choice for outcomes that are continuous but restricted to the interval (0,1), such as rates or proportions, is the beta distribution (e.g., Ferrari and Cribari-Neto 2004; Kieschnick and McCullough 2003). The latter has the advantage to be very flexible since its density can have quite different shapes depending on the values of the two parameters that index the distribution. However, it includes neither zero nor one in its support. In our study, some households reported that they had not incurred any health expenditures in the previous month; thus, \(Y_i \in [0,1) \, \forall i\). Therefore, the beta distribution is not suitable to model our outcome. In similar cases, in which rates or proportions data include a non-negligible number of zeros and/or ones, a more appropriate choice is the zero and/or one inflated beta distribution (Ospina and Ferrari 2010, 2012). Indeed, the latter is a mixed continuous-discrete distribution defined on the unit interval with probability mass at zero and/or one. For this reason, for the potential outcomes we assume a zero inflated beta distribution composed of two parts: a binary part, responsible for the zeros, and a continuous part, that is responsible for the outcome values that are greater than zero.
Here, \(\omega ^{(z,g)}_i\) is the probability of household i of having zero health expenditures under the encouragement level z and given its membership in the principal stratum g. \(Beta(y; \mu _i^{(z,g)}, \phi _i^{(z,g)})\) is instead the density of a beta distribution with parameters \(\mu ^{(z,g)}_i \in (0,1)\) and \(\phi _i^{(z,g)} > 0\), that has the form
for \(z \in \{0,1\}\) and \(g \in \{00,01\}\). \(\mu _i^{(z,g)}\) is the mean of the latter distribution, and \(\phi _i^{(z,g)}\) is responsible for its precision: for fixed \(\mu _i^{(z,g)}\), the larger the value of \(\phi _i^{(z,g)}\), the smaller the variance of \(Y_i(z)\). This parameterization of the beta distribution is not the usual one, but it is convenient here because it allows modeling its mean, i.e., the mean of the continuous part of the zero inflated beta distribution, and its precision parameter as a function of the covariates directly (Ospina and Ferrari 2012). In order to have a more parsimonious model, we assume that \(\phi _i^{(z,g)}\) depends only on the encouragement level and the principal stratum membership, and not on covariates: thus, \(\phi _i^{(z,g)} = \phi ^{(z,g)} \, \forall i\). On the contrary, we assume that \(\omega ^{(z,g)}_i\) and \(\mu ^{(z,g)}_i\) are defined as:
where \(\varvec{\rho }^{(z,g)} = (\rho ^{(z,g)},\varvec{\rho }_{X}^{(z,g)})^{'}\) and \(\varvec{\beta }^{(z,g)} = (\beta ^{(z,g)}, \varvec{\beta }^{(z,g)}_X)^{'}\) are vectors of unknown regression parameters. Assuming that the covariates are linear functions of the parameters and specifying a logit link function we have:
We assume, for the sake of parsimony, that the effect of the covariates on the outcome is the same for all the households: \(\varvec{\rho }^{(z,g)}_X \equiv \varvec{\rho }_X\) and \(\varvec{\beta }^{(z,g)}_X \equiv \varvec{\beta }_X\).
Furthermore, it would be possible to include different covariates in the binary and in the continuous part of the zero inflated beta model. Yet, we choose the same set of covariates, \(\varvec{X}_i\), for both parts, since we believe that the predictors of zero medical expenses and the amount of non-zero medical expenses are plausibly the same. Specifically, \(\varvec{X}_i\) includes: the share of health expenditures out of the total monthly expenditures prior to the intervention; the total monthly expenditures and the amount of monthly savings; whether the household head is female; whether in the household there are members aged 5 or less and, more generally, the size of the household; whether the main source of income is subsistence farming; whether it happened the previous month that there was not food to eat in the house because of the lack of resources to get the food; and, finally, the level of satisfaction with the health status of household members. This set of covariates is partially different from the one that enters the principal strata model, \(\varvec{W}_i\). In fact, we have observed variables that we reasonably believe are predictors of compliance and also of the outcome, as well as variables that are related to only one of them. For example, the willingness to pay for a health financing scheme is considered in the literature as a specific proxy of the ability to pay for it (Adebayo et al. 2015), thus affecting the enrolment in CHF.
We specify weakly informative prior distributions for the parameters. Specifically, for the parameters of the principal strata model, \(\alpha\) and \(\varvec{\alpha }_{W}\), we assume Normal prior distributions with mean 0 and standard deviation equal to 5. Also for the parameters in the potential outcomes model we assume Normal prior distributions centered at zero. However, for the intercepts of the binary and the continuous part of the model, \(\rho ^{(z,g)}\) and \(\beta ^{(z,g)}\), we set the prior standard deviation to 2, while for the regression coefficients of the model, \(\varvec{\rho }_X\) and \(\varvec{\beta }_X\) we set it to 5. This diversification of the standard deviations is motivated by stability reasons. Finally, \(\phi ^{(z,g)} \sim Gamma(0.25,0.25)\), where the hyperparameters are chosen to make the prior variance for the potential outcomes similar to the observed outcome variance.
4.2.1 Assuming and relaxing exclusion restriction
As anticipated in Sect. 3.2.2, we first obtain the results under the ER for Never Takers (Assumption 4). We assume it by imposing the following constraints on the models’ parameters:
A sensitivity analysis for this assumption can be performed by relaxing it and then comparing the posterior distributions of the estimated potential outcomes for Never Takers. The most straightforward way to do this is not to impose the constraints in Eqs. (7), (8) and (9) and examine how the posterior distributions of estimated finite-sample PCEs change (e.g., Imbens and Rubin 1997; Mattei et al. 2013). In fact, when assuming the ER for Never Takers, PCEs for this stratum are forced to be equal to zero. When relaxing it, they have a nondegenerate posterior distribution, which could be centered around zero, thus lending credibility to the ER, or not. This is the sensitivity analysis we perform later in the paper. In Appendix A.1, we describe an equivalent way of performing this sensitivity analysis.
4.3 Bayesian inference
Let \(\varvec{\theta } \in \{ \alpha , \varvec{\alpha }_W, \rho ^{(0,00)}, \rho ^{(1,00)}, \rho ^{(0,01)}, \rho ^{(1,01)}, \varvec{\rho }_X, \beta ^{(0,00)}, \beta ^{(1,00)}, \beta ^{(0,01)}, \beta ^{(1,01)}, \varvec{\beta }_X, \phi ^{(0,00)}, \phi ^{(1,00)}, \phi ^{(0,01)}, \phi ^{(1,01)} \}\) be the parameter vector, including the parameters of the distribution of the principal strata membership given the covariates in \(\varvec{W}_i\), and of the distribution of the potential outcomes given the covariates in \(\varvec{X}_i\) and the principal stratum. Assume a prior distribution \(p(\varvec{\theta })\). Denote also \(\pi _i^{(g)} \equiv Pr\big (G_i=g \mid \varvec{W}_i \big )\) and \(f_i(y)^{(z,g)} \equiv Pr\big (Y_i(z)=y \mid G_i=g,\varvec{X}_i \big )\). Given the latent nature of the principal strata, the observed data likelihood results in the following finite mixture model likelihood:
Consequently, the posterior \(p(\varvec{\theta } \mid \varvec{Z},\varvec{M},\varvec{Y},\varvec{W},\varvec{X}) \propto p(\varvec{\theta }) \times {\mathcal {L}}(\varvec{\theta })\) is analytically intractable. To solve this issue, we exploited Stan (Stan Development Team 2014), a free and open-source C++ software that performs Hamiltonian Monte Carlo using an adaptive variant of the algorithm (Hoffman and Gelman 2014).
5 Results
In this Section, we show and compare the results obtained first assuming the ER for Never Takers and then relaxing it.
5.1 With exclusion restriction for Never Takers
5.1.1 Principal strata
First of all, let us focus on the size and the characteristics of the principal strata. Regarding their size, the estimated posterior probabilities of principal strata membership are reported in Table 6.
The vast majority of the sample is made of Compliers (posterior mean 76.8%, 95% credible interval 72.4–80.7%). This means that the vast majority of the households would accept the offer to enroll in the CHF scheme after attending sensitization sessions. Examining the characteristics of the households that, instead, would opt out can provide us with insight into the reasons behind their lack of compliance.
We summarize the distributions of the characteristics of the principal strata in Fig. 2.
From this figure, it is evident that Compliers and Never Takers are somewhat different with regards to almost every aspect considered. First and foremost, Never Takers have a lower share of health expenditures but, at the same time, higher total expenditures. Therefore, one could suppose that these households would not accept to enroll in the CHF scheme because they do not need it. However, to a closer look, it is evident that this is not the case. Indeed, Never Takers more often have children aged 5 or less, which usually implies more healthcare costs, and also a higher incidence of serious illness among household’s members. It is noteworthy that Never Takers faced more difficulties than Compliers in the year prior to the intervention, since they experienced a remarkably higher number of shocks. These shocks include not only the occurrence of serious illness but also death of household’s members, as well as the occurrence of conflicts, thefts, fires, droughts, floods and other episodes that may partially explain the higher total expenditures and that are likely to have a major impact on the financial well-being of the family. Moreover, Never Takers declared to have concerns about the affordability of healthcare services more often than Compliers. In addition, they are less willing to pay 10,000 UGX/year per household member in order to have a health insurance; in the light of what we have said so far, this is reasonably due to the amount of money required, rather than to a lack of willingness to have a health insurance. Given the complete picture, it does not seem plausible that Never Takers did not need or did not want a health insurance, but rather that they could not afford it. Finally, we found further differences between Never Takers and Compliers with respect to the literacy level and gender of the household head. In particular, the former confirms the lower socio-economic status of Never Takers, while the latter indicates that female-headed households are more likely to be Compliers.
5.1.2 Estimated principal causal effects
Since we have assumed the ER for Never Takers, which states the absence of any treatment effect for this subgroup, we now focus on the estimated treatment effects for Compliers. The estimated finite-sample mean potential outcomes and PCEs for the latter principal stratum are reported in Tables 7 and 8, respectively.
The estimated share of health costs over the total monthly expenditures is fairly high for Compliers in the absence of intervention (posterior mean 16.7%, 95% credible interval 12.8–22.4%); however, it is significantly reduced by attending the sensitization sessions and enrolling into the CHF scheme (posterior mean 12.2%, 95% credible interval 11.3–13.2%). The intervention is shown to have a considerable positive impact on catastrophic health expenditures as well; indeed, their average incidence is 62.9% (95% credible interval 50.3–74.6%) in the absence of intervention, but it reduces to 48.6% (95% credible interval 44.4–52.9%) when the intervention is offered and undertaken. Consistently, in the latter case there is also a noteworthy increase in the incidence of zero monthly healthcare expenditures, which reaches 8.6%, on average (95% credible interval 6.0–11.9%); indeed, in the absence of the intervention, this incidence is, on average, 3.3% (95% credible interval 0.0–9.8%), that is, it amounts to less than half.
In light of these findings, the intervention has accomplished its goal of reducing health care costs for Compliers. The estimated effects are the result of attending the sensitization sessions and participating in the CHF scheme as a whole. In fact, on the one hand, sensitization was aimed at raising awareness on the importance of using health care services promptly when needed; on the other hand, the prompt access to health care services was facilitated by the possibility of using the common fund to cover the costs at the immediate time of need. Avoiding waiting for worsening health conditions before seeking medical consultation or treatment avoids having to pay for expensive emergency services. Moreover, the greater incidence of zero monthly health costs resulting from the intervention indicates that households could have further changed their health-seeking behaviors realizing the importance of using free prevention services instead of waiting for health problems to occur.
5.2 Without exclusion restriction for Never Takers
5.2.1 Principal strata
Let us now evaluate the results relative to the principal strata size and characteristics when ER for Never Takers is not assumed. The posterior distributions of the proportion of Compliers and Never Takers almost entirely overlap with those obtained when assuming it. We report some summary statistics in Table 9.
Not only the size of the principal strata, but also their characterization remains essentially the same. We report the boxplots summarizing the distributions of the covariates by principal stratum in Appendix A.2 (Fig. 4).
5.2.2 Estimated principal causal effects
Since we do not assume, at this stage, the ER for Never Takers, we allow for the presence of encouragement effects for this subgroup as well as for that of Compliers. For both principal strata, we report some summary statistics of the estimated finite-sample mean potential outcomes in Table 10.
From this Table it is evident that the share of health costs over the total monthly expenditures is extremely high for Never Takers when they do not attend sensitization sessions; specifically, it amounts to 24.2% of the total monthly expenditures, on average (95% posterior credible interval 13.5–37.9%). This mean is much higher than the threshold above which monthly health expenditures are considered “catastrophic”, (i.e., 10% of the total monthly expenditures). It follows that also the incidence of catastrophic health expenditures is extremely high for this principal stratum in absence of sensitization: in fact, in that case, the estimated percentage of Never Takers that have catastrophic health expenditures is, on average, 60.9% (95% posterior credible interval 33.3–83.6%). At the same time, however, the estimated incidence of zero health expenditures among these households is high as well: specifically, on average, 18.7% of Never Takers is estimated to have zero monthly health expenditures when not receiving sensitization (95% posterior credible interval 3.5–43.2%). Given the overall characteristics of this stratum discussed in Sect. 5.1.1, it seems plausible that most of these households could not afford any health expenditures.
On the other hand, the estimates reported in Table 10 for Compliers when they do not attend sensitization sessions and thereby do not participate in the scheme are similar to those obtained under the ER for Never Takers. Hence, the disadvantage we found, based on pre-intervention characteristics, of Never Takers compared to Compliers persists or is even worsened at follow-up if no appropriate intervention is implemented.
The posterior distributions of the finite-sample PCEs are approximated by the histograms reported in Appendix A.2 (Fig. 5). These distributions are all well-shaped. Some summary statistics are shown in Table 11.
From this Table it is evident that beneficial effects of the intervention exist for both the principal strata, and that they are even bigger for Never Takers than for Compliers. In fact, we estimate a significant reduction of the share of health costs over the total monthly expenditures that averages \(-\)3.3% (95% credible interval \(-\)6.9 to 0.2%) for Compliers, while it reaches an average of \(-\)12.9% (95% credible interval \(-\)26.9 to \(-\)1.8%) for Never Takers. This effect might seem counter-intuitive, because Never Takers do not actually participate in the program, while Compliers do. However, the different characteristics of Never Takers and Compliers may help explain the finding. First, Never Takers have much higher health care costs in the absence of intervention (see Table 10), and thus significantly more room for improvement than Compliers. Moreover, the disadvantages Never Takers face, based on their observed characteristics, probably make them more sensitive and responsive to the principles taught during the sensitization sessions. Of course, this does not mean that participating in the program is detrimental and that it would be better to attend only the sensitization sessions; in fact, Compliers seem to benefit from the program. The results suggest that, for some individuals, a well designed sensitization may already produce some beneficial results.
This is reflected in the incidence of catastrophic health expenditures; in particular, we estimate that, on average, 12.2% fewer Compliers and 18.0% fewer Never Takers have catastrophic health expenditures when assigned to the encouragement. About the former effect, \(CHEE_{01}\), the 95% credible interval covers zero, although the posterior probability of this effect being negative is very high (0.955). The same is true for the latter effect, \(CHEE_{00}\), which has a posterior probability of being negative of 0.882.
Finally, the intervention also has an impact on the incidence of zero monthly health expenditures. On the one hand, we estimate that 6.4% more Compliers have zero monthly health expenditures. As previously argued in Sect. 5.1.2, this effect could be due to a more widespread use of free preventive services. On the other hand, we estimate that 12.1% fewer Never Takers have zero health expenditures, that is equivalent to say that 12.1% more Never Takers spend some money over the course of a month for medical or health services. Given the observed characteristics of Never Takers, we believe that most of them were facing financial hardship and therefore would not have spent any money for apparently postponable health problems if not encouraged to join the program; however, if encouraged, they are persuaded to seek for a medical consultation or treatment. In fact, during the sensitization sessions, these households were instructed on the importance of using the necessary health care services without stalling because this would have improved the health status of household members and that this, in turn, would have prevented them from facing additional health costs in the future. The 95% credible interval for the former effect, i.e. \(ZHEE_{01}\), does not include the zero, while that for the latter effect, i.e. \(ZHEE_{00}\), includes the zero but it is almost negative.
6 Additional sensitivity analyses
We evaluated the goodness-of-fit of the models, when we did not assume the ER for Never Takers, by conducting posterior predictive checks (Rubin 1984). This practice is well-established in Bayesian model-based PS analyses (e.g., Barnard et al. 2003; Mattei et al. 2013; Forastiere et al. 2021). A posterior predictive check involves a comparison between the observed data and replications of them generated from their posterior predictive distribution. Intuitively, if the models are specified appropriately, the latter should generate data similar to the observed ones. In addition to making a visual inspection, we can formally test the null hypothesis that a chosen observed statistic comes from the model we actually specified. A formal posterior predictive check involves first the choice of a discrepancy measure, which is a function of the observed data and the parameter vector \(\varvec{\theta }\), and second the computation of a Bayesian p-value. A widely used Bayesian p-value is the so-called posterior predictive p-value (PPPV) (Meng 1994; Gelman et al. 1996), which is, in this context, the probability over the posterior predictive distribution of the principal strata and the parameter vector \(\varvec{\theta }\) that a given discrepancy measure in a new study data, drawn with the same \(\varvec{\theta }\) as in the observed study, would be as or more extreme than its realized value in the observed study. An estimate of the PPPV can be derived by first obtaining the frequency distribution of the discrepancy measure in R replications of the data, i.e., the frequency distribution of \(\Delta ^{rep}\), and then calculating the percentage of draws in which \(\Delta ^{rep}\) exceeds the realized value of the discrepancy measure in the observed study, \(\Delta ^{obs}\). Formally, the PPPV of a generic discrepancy measure is calculated as \(\frac{1}{R} \sum _{r=1}^{R} \mathbbm {1} \{ \Delta ^{rep} \ge \Delta ^{obs}\}\). Looking at the p-value thus estimated, we assess whether the model can preserve features of the data relevant to the calculation of the discrepancy measure. If its value is extreme (close to 0 or 1), it means that the prior distribution and the likelihood cannot adequately replicate the discrepancy measure to which the p-value refers.
Let \(\varvec{\theta }^{r}\, (r=1, \ldots ,R)\) denote the draw of the parameters vector from its posterior distribution at iteration r. We simulate \(R=3000\) replicated data sets as follows. First, we generate the principal stratum membership of every subject in the sample from the hypothesized principal strata model given \(\varvec{\theta }^r\), i.e., \(G^{rep,r}_i\). Thus, it is possible to simulate the outcome \(Y^{rep,r}_i\) from its posterior predictive distribution, keeping the encouragement \(Z_i\) fixed at its observed value. We repeat the whole procedure R times and thus we obtain R replicated data sets, \(\left( G^{rep,r}_i, Y^{rep,r}_i, Z_i \right)\). Once we have these replicated data sets, we can use them to compute all the discrepancy measures of interest.
Let us indicate with \({\mathcal {D}}_{z,g}^{study} = \{i: Z_i=z\text { and }G_i^{study}=g \}\) the group of units belonging to \(G_i^{study}=g\) assigned to \(Z_i=z\) in the study data, where \(study=obs\) denotes the observed data and \(study=rep\) a replication of them obtained from their posterior predictive distribution as described above. Let \(\vert {\mathcal {D}}^{study}_{z,g} \vert\) indicate the cardinality of \({\mathcal {D}}_{z,g}^{study}\), i.e., the number of units in this group. \(Y_i^{study}\) is the realized value of the outcome variable in the study data. We adopt the following discrepancy measures:
where \(s^{2,study}_{z,g}\) denotes the variance of \(Y^{study}\) for the \({\mathcal {D}}^{study}_{z,g}\) group. The measures of signal, \(SI^{study}_{z,z',g}\), noise, \(NO^{study}_{z,z',g}\), and signal to noise, \(SN^{study}_{z,z',g}\), were proposed by Barnard et al. (2003), and were adopted by Mattei et al. (2013) in a Bayesian model-based PS analysis.
The estimated PPPVs for these discrepancy measures are reported in Table 12.
They are all far from zero and one for both Never Takers and Compliers, suggesting a good model fit.
7 Concluding remarks
Although the statistical literature is abundant of studies in which principal stratification is used to analyze experimental data characterized by noncompliance, in the economics literature the use of the Instrumental Variables approach is still well-established. This article shows the consequences of violations of the Exclusion Restriction on which the Instrumental Variables method relies in the impact evaluation of a intervention of CHF implemented in rural Uganda. This sensitivity analysis is conducted by using a Bayesian model-based principal stratification first assuming an Exclusion Restriction and then relaxing it. Results show that this intervention has positive effects not only on Compliers, i.e. households that would join the scheme upon receiving an initial sensitization and offer to enroll, but also on Never Takers, i.e. households that would refuse to join the scheme despite the initial sensitization and offer to enroll. The effects for Compliers are associative, meaning that they may derive from the initial sensitization and offer to enroll but also from participating in the scheme, while those for Never Takers are dissociative, meaning that they can only derive from the initial sensitization and offer to enroll. The presence of the latter is ruled out when the Exclusion Restriction is assumed; however, our analysis provides evidence that they do exist. Therefore, principal stratification allows deepening the analysis by taking into account the possibility of causal effects of attending sensitization sessions and receiving the offer to enroll in the CHF scheme.
The analysis yields the following results. For Compliers, the intervention is shown to be strongly effective in reducing the share of health costs over the total monthly expenditures and the incidence of catastrophic health expenditures as well. Thus, the scheme improves financial protection for Compliers allowing them to promptly use health care services by delaying the economic burden of health expenses. Moreover, it increases the incidence of zero health expenditures, i.e., it increases the number of Compliers having zero monthly health costs. This might indicate a change in health-seeking behaviors leading to an increase in the utilization of preventive services free of charge and a decrease in that of expensive emergency services.
For Never Takers, the strongest causal effect of the intervention is in reducing the share of health costs over the total monthly expenditures and, to a weaker extent, in decreasing both the incidence of catastrophic and zero health expenditures. Hence, the intervention reduces the often excessively high health costs for some households while inducing others to spend something for health that they would not have spent otherwise. Therefore, we believe that Never Takers are of two different types. Some do not join the scheme because they cannot afford the initial amount required to participate (5000 UGX/year per member). However, by attending the sensitization sessions, they learned the importance of trying to save a little money to be able to deal with health problems that might occur, but also to spend on health today to avoid worse health problems and higher expenses in the future. Others are already facing financial hardship due to health problems that occurred in the previous year and thus do not join the scheme to save the initial money required to participate. They do, however, have many health-related expenses with and without the intervention but spend, in general, less because the sensitization makes them understand how to manage their finances more effectively.
Appendix A reports some additional results related to the analyses on health expenses. Appendix B reports a supplementary analysis aimed at assessing if this effect on health expenses was accompanied by an effect on the amount of monthly savings. The results indicate some positive effects for Compliers, but not for Never Takers. The lack of a clear effect for the latter group might be attributable to the fact that sensitization makes some households have fewer health costs and thereby more savings, but also makes others spend some money on health that they would not have spent otherwise, thereby preventing their savings from increasing substantially.
These analyses have two important implications in terms of policy making. The first is the fact that those that would benefit most from a health insurance do not seem to have the resources needed to take it out. The second is the fact that activities of sensitization alone can be extremely effective to reduce vulnerability against health costs for all population groups (not only enrolled members). The implementation of awareness-raising campaigns has thus potential to significantly contribute to enlarge health coverage and to reduce impoverishment for poor and vulnerable population groups who receive information and change their behaviors for health financing.
Notes
42 community groups were chosen in order to reach 10,304 individuals, accounting for nearly 20% of the population in the intervention area.
The vast majority of residents in the 62 selected villages in the intervention area were targeted by the intervention.
As explained in Sect. 2, the intervention was assigned to community groups.
References
Adebayo EF, Uthman OA, Wiysonge CS, Stern EA, Lamont KT, Ataguba JE (2015) A systematic review of factors that affect uptake of community-based health insurance in low-income and middle-income countries. BMC Health Serv Res 15(1):1–13
Angrist JD, Pischke J-S (2008) Mostly harmless econometrics. Princeton University Press
Angrist JD, Imbens GW, Rubin DB (1996) Identification of causal effects using instrumental variables. J Am Stat Assoc 91(434):444–455
Barnard J, Frangakis CE, Hill JL, Rubin DB (2003) Principal stratification approach to broken randomized experiments: a case study of school choice vouchers in New York city. J Am Stat Assoc 98(462):299–323
Biggeri M, Nannini M, Putoto G (2018) Assessing the feasibility of community health insurance in Uganda: a mixed-methods exploratory analysis. Soc Sci Med 200:145–155
Chemin M (2018) Informal groups and health insurance take-up evidence from a field experiment. World Dev 101:54–72
Ferrari S, Cribari-Neto F (2004) Beta regression for modelling rates and proportions. J Appl Stat 31(7):799–815
Forastiere L, Lattarulo P, Mariani M, Mealli F, Razzolini L (2021) Exploring encouragement, treatment, and spillover effects using principal stratification, with application to a field experiment on teens’ museum attendance. J Bus Econ Stat 39(1):244–258
Frangakis CE, Rubin DB (2002) Principal stratification in causal inference. Biometrics 58(1):21–29
Gelman A, Meng X-L, Stern H (1996) Posterior predictive assessment of model fitness via realized discrepancies. Stat Sin 6:733–760
Gustafson P (2010) Bayesian inference for partially identified models. Int J Biostat 6(2):17. https://doi.org/10.2202/1557-4679.1206
Hirano K, Imbens GW, Rubin DB, Zhou X-H (2000) Assessing the effect of an influenza vaccine in an encouragement design. Biostatistics 1(1):69–88
Hoffman MD, Gelman A (2014) The no-u-turn sampler: adaptively setting path lengths in Hamiltonian Monte Carlo. J Mach Learn Res 15(1):1593–1623
Imbens GW, Angrist JD (1994) Identification and estimation of local average treatment effects. Econometrica 62(2):467–475
Imbens GW, Rubin DB (1997) Bayesian inference for causal effects in randomized experiments with noncompliance. Ann Stat 25:305–327
Jütting JP (2004) Do community-based health insurance schemes improve poor people’s access to health care? Evidence from rural Senegal. World Dev 32(2):273–288
Kieschnick R, McCullough BD (2003) Regression analysis of variates observed on (0, 1): percentages, proportions and fractions. Stat Model 3(3):193–213
Kruk ME, Goldmann E, Galea S (2009) Borrowing and selling to pay for health care in low-and middle-income countries. Health Affairs 28(4):1056–1066
Leive A, Xu K (2008) Coping with out-of-pocket health payments: empirical evidence from 15 African countries. Bull World Health Organ 86:849-856C
Mattei A, Li F, Mealli F (2013) Exploiting multiple outcomes in Bayesian principal stratification analysis with application to the evaluation of a job training program. Ann Appl Stat 7(4):2336–2360
Mealli F, Mattei A (2012) A refreshing account of principal stratification. Int J Biostat 8(1):8. https://doi.org/10.1515/1557-4679.1380
Mealli F, Pacini B (2013) Using secondary outcomes to sharpen inference in randomized experiments with noncompliance. J Am Stat Assoc 108(503):1120–1131
Mealli F, Rubin DB (2002) Assumptions when analyzing randomized experiments with noncompliance and missing outcomes. Health Serv Outcomes Res Methodol 3(3):225–232
Mealli F, Pacini B, Stanghellini E (2016) Identification of principal causal effects using additional outcomes in concentration graphs. J Educ Behav Stat 41(5):463–480
Meng X-L (1994) Posterior predictive \(p\)-values. Ann Stat 22(3):1142–1160
Mladovsky P, Soors W, Ndiaye P, Ndiaye A, Criel B (2014) Can social capital help explain enrolment (or lack thereof) in community-based health insurance? Results of an exploratory mixed methods study from Senegal. Soc Sci Med 101:18–27
Nannini M, Biggeri M, Putoto G (2021) Financial protection and coping strategies in rural Uganda: an impact evaluation of community-based zero-interest healthcare loans. Health Policy Plan 36(7):1090
Ospina R, Ferrari SL (2010) Inflated beta distributions. Stat Pap 51(1):111–126
Ospina R, Ferrari SL (2012) A general class of zero-or-one inflated beta regression models. Comput Stat Data Anal 56(6):1609–1623
Rubin DB (1980) Randomization analysis of experimental data: the fisher randomization test comment. J Am Stat Assoc 75(371):591–593
Rubin DB (1984) Bayesianly justifiable and relevant frequency calculations for the applied statistician. Ann Stat 12:1151–1172
Sommerfeld J, Sanon M, Kouyate BA, Sauerborn R (2002) Informal risk-sharing arrangements (IRSAS) in rural Burkina Faso: lessons for the development of community-based insurance (CBI). Int J Health Plan Manag 17(2):147–163
Stan Development Team (2014) Stan: a c++ library for probability and sampling. http://mc-stan.org
van de Schoot R, Depaoli S, King R, Kramer B, Märtens K, Tadesse MG, Vannucci M, Gelman A, Veen D, Willemsen J et al (2021) Bayesian statistics and modelling. Nat Rev Methods Primers 1(1):1–26
Wagstaff A, Bilger M, Sajaia Z, Lokshin M (2011) Health equity and financial protection: streamlined analysis with ADePT software. World Bank Publications
Wagstaff A, Flores G, Hsu J, Smitz M-F, Chepynoga K, Buisman LR, van Wilgenburg K, Eozenou P (2018) Progress on catastrophic health spending in 133 countries: a retrospective observational study. Lancet Glob Health 6(2):e169–e179
Acknowledgements
We are grateful for the logistical support provided by CUAMM. We would like to thank the Oyam district authorities for their support during the fieldwork and the members of the research team. We would also like to thank the people of Oyam who participated in the survey with patience and courtesy.
Funding
Open access funding provided by Università degli Studi di Firenze within the CRUI-CARE Agreement.
Author information
Authors and Affiliations
Corresponding author
Ethics declarations
Conflict of interest
The authors declare that they have no conflict of interest.
Ethics approval
Ethical approval for the study was obtained by the Uganda National Council for Science and Technology (ref: SS 5122).
Consent to participate
Informed written consents were collected from all research participants.
Additional information
Publisher's Note
Springer Nature remains neutral with regard to jurisdictional claims in published maps and institutional affiliations.
Appendices
Appendix A Additional results
1.1 A.1 Assessing the validity of the Exclusion Restriction
To conduct a sensitivity analysis for the ER for Never Takers, it is possible to contrast different features of the posterior distributions of the estimated potential outcomes for Never Takers, including the parameters of these distributions. Specifically, by assessing the posterior distributions of \({\widehat{\rho }}^{(1,00)}-{\widehat{\rho }}^{(0,00)}\), \({\widehat{\beta }}^{(1,00)}-{\widehat{\beta }}^{(0,00)}\) and \({\widehat{\phi }}^{(1,00)}-{\widehat{\phi }}^{(0,00)}\), it is possible to understand whether the assumption would hold if it were imposed. In fact, these distributions could be centered around zero, thus lending credibility to the ER, or not.
We show the result of this sensitivity analysis in Fig. 3.
The posterior distributions in the figure are not centered around zero and, while the 95% credible intervals for \(\rho ^{(1,00)}-\rho ^{(0,00)}\) and \(\phi ^{(1,00)}-\phi ^{(0,00)}\) still cover zero, the one for \(\beta ^{(1,00)}-\beta ^{(0,00)}\) does not. Therefore, this examination shows that the ER for Never Takers is not valid in this study.
1.2 A.2 Additional figures
Appendix B Supplementary analysis
We now want to assess whether the impact of the intervention in decreasing the monthly health costs of most of the households is also reflected in an increase in their monthly savings.
1.1 B.1 The outcome
The histograms of the empirical distribution of monthly savings by encouragement group are shown in Fig. 6.
From this plot it is evident that the distribution of this outcome for households that did not receive sensitization is more shrunk towards zero than that of households that receive sensitization and were offered CHF. Moreover, we can notice the presence of several outliers in the latter distribution, i.e., several households with an unusually large amount of monthly savings among those which received sensitization. The complication due the presence of these outliers can be addressed in several ways. A simple option could be to remove them and conduct the analysis on a subset of households only. However, we want to have here the same sample we had in our primary analysis so we can interpret the results of both analyses as a whole. Thus, we decided to categorize monthly savings according to the tertiles of its empirical distribution. We denote with \(\varvec{Y}^*\) the N-dimensional vector containing the resulting categorical outcome for the whole sample: \(Y_i^*=t\) if the monthly savings of i-th household are in the t tertile (\(t \in \{1,2,3\}\)). The sample size at hand does not allow a finer categorization. Relying on SUTVA (Assumption 1), we again index the individual potential outcomes by the individual encouragement only; so, we indicate with \(Y^{*}_i(z)\) the monthly savings tertile of i-th household under the encouragement level z (with \(z \in \{0,1\}\)). The rest of the notation remains the same as in the previous analyses.
1.2 B.2 Methodology
We define the same principal strata and we again estimate PCEs, i.e., stratum-specific effects of the intervention. We refer to the PCEs on savings as Savings Effect (SE), and we define them as follow:
Therefore, we have a PCE on each tertile t of the empirical distribution of savings for each principal stratum g. We do not assume, here, the ER for Never Takers given that the previous analyses revealed the existence of some causal effects even for this subgroup.
We again use Bayesian inference methods. We specify the same model for the principal strata membership given the covariates, but a different model for the potential outcomes conditional on the covariates and the principal strata. Specifically, given the categorical nature of \(\varvec{Y}^*\), we model it using a multinomial logistic model of the form:
with \(z \in \{0,1\}\) and \(t=1,2\) (the last category of \(Y^*\) is taken as the reference). \(\varvec{\gamma }_{S,t} = (\gamma _{1,t},...,\gamma _{K,t})^{'}\) are vectors of unknown regression parameters and \(\varvec{S}_i\) is a vector of K pre-treatment covariates. Specifically, \(\varvec{S}_i\) includes: the monthly savings prior to the intervention; the total monthly expenditures and the amount of monthly savings; whether the household is single, widow or divorced; whether in the household there are members aged 5 or less and, more generally, the size of the household; whether the main source of income is subsistence farming; and, finally, the level of satisfaction with the health status of household members.
We specify weakly informative prior distributions for the parameters. Specifically, for the parameters of the principal strata model we specify the same prior distributions that we specified in the analysis on health costs; for the parameters of the outcome model, we assume Normal prior distributions with mean 0 and standard deviation equal to 2.
1.3 B.3 Results
Regarding the principal strata, the estimated proportion of Compliers and Never Takers are more or less the same we found in the previous analyses (Table 13).
Likewise, also the observed characteristics of these principal strata are very similar to those we estimated before; we report the resulting boxplots in Fig. 7.
Regarding the estimated PCEs, reported in Table 14, we found some interesting results for Compliers.
On the one hand, we estimate a higher incidence of savings in the third tertile following the intervention: 16.2% more Compliers have savings in the third tertile, on average (95% credible interval \(-\)3.8% – 35.1%). On the other hand, we cannot conclude that the intervention clearly decreases the incidence of savings in the first tertile for this principal stratum, although the posterior distribution of the estimated effect is mostly negative. Finally, for Never Takers, the 95% credible intervals for the estimated PCEs are wider – because these households are fewer in number than Compliers – and include zero, so we cannot conclude that there is an effect of the sensitization received on saving.
Rights and permissions
Open Access This article is licensed under a Creative Commons Attribution 4.0 International License, which permits use, sharing, adaptation, distribution and reproduction in any medium or format, as long as you give appropriate credit to the original author(s) and the source, provide a link to the Creative Commons licence, and indicate if changes were made. The images or other third party material in this article are included in the article's Creative Commons licence, unless indicated otherwise in a credit line to the material. If material is not included in the article's Creative Commons licence and your intended use is not permitted by statutory regulation or exceeds the permitted use, you will need to obtain permission directly from the copyright holder. To view a copy of this licence, visit http://creativecommons.org/licenses/by/4.0/.
About this article
Cite this article
Noirjean, S., Biggeri, M., Forastiere, L. et al. Estimating causal effects of community health financing via principal stratification. Stat Methods Appl 32, 1317–1350 (2023). https://doi.org/10.1007/s10260-023-00706-0
Accepted:
Published:
Issue Date:
DOI: https://doi.org/10.1007/s10260-023-00706-0