Abstract
In this paper, we present a novel approach to achieve finite-time synchronization (FTS) in a certain class of fractional-order complex-valued neural networks (CVNNs) containing reaction-diffusion terms. The proposed method uses intermittent control and provides a theoretical analysis to establish criteria for achieving FTS. This is achieved through new Lyapunov functions based on the proposed system, deriving inequalities in the complex domain. To realize FTS, the study designs complex-valued intermittent controllers for the targeted CVNNs relying solely on the information obtained from the controlled nodes. Moreover, an adaptive controller is introduced to effectively regulate the control gain, and the FTS of CVNNs is analyzed. The effectiveness of the proposed control strategies and derived results is demonstrated by numerical examples.







Similar content being viewed by others
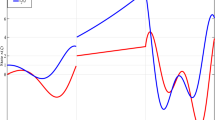
Data availability
No new data were created or analyzed in this study. Data sharing is not applicable to this article.
References
Song J, Niu Y, Zou Y (2016) Finite-time sliding mode control synthesis under explicit output constraint. Automatica 65:111–114
Hernandez-Gonzalez M, Hernandez-Vargas EA, Basin MV (2018) Discrete-time high order neural network identifier trained with cubature kalman filter. Neurocomputing 322:13–21
Ali MS, Saravanan S, Arik S (2016) Finite-time \({H}_\infty \) state estimation for switched neural networks with time-varying delays. Neurocomputing 207:580–589
Ali MS, Saravanan S, Palanisamy L (2019) Stochastic finite-time stability of reaction-diffusion cohen-grossberg neural networks with time-varying delays. Chinese J Phys 57:314–328
Kan Y, Lu J, Qiu J, Kurths J (2019) Exponential synchronization of time-varying delayed complex-valued neural networks under hybrid impulsive controllers. Neural Netw 114:157–163
Yan S, Gu Z, Park JH, Xie X. Synchronization of delayed fuzzy neural networks with probabilistic communication delay and its application to image encryption, IEEE Transactions on Fuzzy Systems (2022)
Long C, Zhang G, Zeng Z, Hu J (2022) Finite-time stabilization of complex-valued neural networks with proportional delays and inertial terms: a non-separation approach. Neural Netw 148:86–95
Arena P, Fortuna L, Porto D (2000) Chaotic behavior in noninteger-order cellular neural networks. Phys RevE 61(1):776
Bao H, Park JH, Cao J (2016) Synchronization of fractional-order complex-valued neural networks with time delay. Neural Netw 81:16–28
Wu A, Zeng Z (2016) Boundedness, mittag-leffler stability and asymptotical \(\omega \)-periodicity of fractional-order fuzzy neural networks. Neural Netw 74:73–84
Chen J, Zeng Z, Jiang P (2014) Global mittag-leffler stability and synchronization of memristor-based fractional-order neural networks. Neural Netw 51:1–8
Yuan Y, Song Q, Liu Y, Alsaadi FE (2019) Synchronization of complex-valued neural networks with mixed two additive time-varying delays. Neurocomputing 332:149–158
Yang S, Yu J, Hu C, Jiang H (2018) Quasi-projective synchronization of fractional-order complex-valued recurrent neural networks. Neural Netw 104:104–113
Song Q, Zhao Z (2016) Stability criterion of complex-valued neural networks with both leakage delay and time-varying delays on time scales. Neurocomputing 171:179–184
Song X, Li X, Song S, Zhang Y, Ning Z (2021) Quasi-synchronization of coupled neural networks with reaction-diffusion terms driven by fractional brownian motion. J Franklin Inst 358(4):2482–2499
Kondo S, Miura T (2010) Reaction-diffusion model as a framework for understanding biological pattern formation. Science 329(5999):1616–1620
Adamatzky A, Arena P, Basile A, Carmona-Galán R, Costello BDL, Fortuna L, Frasca M, Rodríguez-Vázquez A (2004) Reaction-diffusion navigation robot control: from chemical to vlsi analogic processors. IEEE Trans Circuits Syst I: Regular Papers 51(5):926–938
Wei T, Lin P, Zhu Q, Wang L, Wang Y (2018) Dynamical behavior of nonautonomous stochastic reaction-diffusion neural-network models. IEEE Trans Neural Netw Learn Syst 30(5):1575–1580
Sun L, Wang J, Chen X, Shi K, Shen H (2021) \(h_\infty \) fuzzy state estimation for delayed genetic regulatory networks with random gain fluctuations and reaction-diffusion. J Franklin Inst 358(16):8694–8714
Alqudah MA, Ashraf R, Rashid S, Singh J, Hammouch Z, Abdeljawad T (2021) Novel numerical investigations of fuzzy cauchy reaction-diffusion models via generalized fuzzy fractional derivative operators. Fractal Fract 5(4):151
Cao Y, Cao Y, Guo Z, Huang T, Wen S (2020) Global exponential synchronization of delayed memristive neural networks with reaction-diffusion terms. Neural Netw 123:70–81
Wei T, Li X, Stojanovic V (2021) Input-to-state stability of impulsive reaction-diffusion neural networks with infinite distributed delays. Nonlinear Dyn 103:1733–1755
Shabunin A, Astakhov V, Demidov V, Provata A, Baras F, Nicolis G, Anishchenko V (2003) Modeling chemical reactions by forced limit-cycle oscillator: synchronization phenomena and transition to chaos. Chaos, Solitons & Fractals 15(2):395–405
Lestienne R (2001) Spike timing, synchronization and information processing on the sensory side of the central nervous system. Prog Neurobiol 65(6):545–591
Yang T, Chua LO (1997) Impulsive stabilization for control and synchronization of chaotic systems: theory and application to secure communication. IEEE Trans Circuits Syst I: Fundam Theory Appl 44(10):976–988
Yang X, Cao J (2013) Exponential synchronization of delayed neural networks with discontinuous activations. IEEE Trans Circuits Syst I: Regular Papers 60(9):2431–2439
Sun W, Wang S, Wang G, Wu Y (2015) Lag synchronization via pinning control between two coupled networks. Nonlinear Dyn 79:2659–2666
Pecora LM, Sorrentino F, Hagerstrom AM, Murphy TE, Roy R (2014) Cluster synchronization and isolated desynchronization in complex networks with symmetries. Nat Commun 5(1):4079
Palva S, Palva JM (2011) Functional roles of alpha-band phase synchronization in local and large-scale cortical networks. Front Psychol 2:204
Li H-L, Cao J, Jiang H, Alsaedi A (2018) Graph theory-based finite-time synchronization of fractional-order complex dynamical networks. J Franklin Inst 355(13):5771–5789
Luo T, Wang Q, Jia Q, Xu Y (2022) Asymptotic and finite-time synchronization of fractional-order multiplex networks with time delays by adaptive and impulsive control. Neurocomputing 493:445–461
Stamova I, Stamov G (2017) Mittag-leffler synchronization of fractional neural networks with time-varying delays and reaction-diffusion terms using impulsive and linear controllers. Neural Netw 96:22–32
Zhang L, Zhong J, Lu J (2021) Intermittent control for finite-time synchronization of fractional-order complex networks. Neural Netw 144:11–20
Cai S, Hou M (2021) Quasi-synchronization of fractional-order heterogeneous dynamical networks via aperiodic intermittent pinning control. Chaos, Solitons & Fractals 146:110901
Zhao L (2021) A note on cluster synchronization of fractional-order directed networks via intermittent pinning control. Physica A: Stat Mech Appl 561:125150
Wang Y, Wu Z (2021) Cluster synchronization in variable-order fractional community network via intermittent control. Mathematics 9(20):2596
Lu X, Zhang X, Liu Q (2018) Finite-time synchronization of nonlinear complex dynamical networks on time scales via pinning impulsive control. Neurocomputing 275:2104–2110
Song X, Man J, Song S, Zhang Y, Ning Z (2020) Finite/fixed-time synchronization for markovian complex-valued memristive neural networks with reaction-diffusion terms and its application. Neurocomputing 414:131–142
Li X, Fang J-A, Zhang W, Li H (2018) Finite-time synchronization of fractional-order memristive recurrent neural networks with discontinuous activation functions. Neurocomputing 316:284–293
Narayanan G, Muhiuddin G, Ali MS, Diab AAZ, Al-Amri JF, Abdul-Ghaffar H (2022) Impulsive synchronization control mechanism for fractional-order complex-valued reaction-diffusion systems with sampled-data control: Its application to image encryption. IEEE Access 10:83620–83635
Podlubny I (1998) Fractional differential equations: an introduction to fractional derivatives, fractional differential equations, to methods of their solution and some of their applications, Elsevier
Gan Q (2013) Exponential synchronization of stochastic fuzzy cellular neural networks with reaction-diffusion terms via periodically intermittent control. Neural Process Lett 37(3):393–410
Palanivel J, Suresh K, Premraj D, Thamilmaran K (2018) Effect of fractional-order, time-delay and noisy parameter on slow-passage phenomenon in a nonlinear oscillator. Chaos, Solitons & Fractals 106:35–43
Acknowledgements
We gratefully acknowledge this work is funded by the Centre for Nonlinear Systems, Chennai Institute of Technology (CIT), India, vide funding number CIT/CNS/2024/RP-005.
Author information
Authors and Affiliations
Contributions
SS and GN contributed to conceptualization, methodology, and writing-original draft; KR helped in formal analysis; KR and MSA done investigation and writing-review & editing; GN done software; SS and MSA done validation; SS contributed to visualization. All authors have read and agreed to the published version of the manuscript
Corresponding author
Ethics declarations
Conflict of interest
The author declares that there is no conflict of interest regarding the publication of this paper.
Additional information
Publisher's Note
Springer Nature remains neutral with regard to jurisdictional claims in published maps and institutional affiliations.
Rights and permissions
Springer Nature or its licensor (e.g. a society or other partner) holds exclusive rights to this article under a publishing agreement with the author(s) or other rightsholder(s); author self-archiving of the accepted manuscript version of this article is solely governed by the terms of such publishing agreement and applicable law.
About this article
Cite this article
Shanmugam, S., Narayanan, G., Rajagopal, K. et al. Finite-time synchronization of complex-valued neural networks with reaction-diffusion terms: an adaptive intermittent control approach. Neural Comput & Applic 36, 7389–7404 (2024). https://doi.org/10.1007/s00521-024-09467-7
Received:
Accepted:
Published:
Issue Date:
DOI: https://doi.org/10.1007/s00521-024-09467-7