Abstract
Recent experimental evidence in experience-based decision-making suggests that people are more risk seeking in the gains domain relative to the losses domain. This critical result is at odds with the standard reflection effect observed in description-based choice and explained by Prospect Theory. The so-called reversed-reflection effect has been predicated on the extreme-outcome rule, which suggests that memory biases affect risky choice from experience. To test the general plausibility of the rule, we conducted two experiments examining how the magnitude of prospective outcomes impacts risk preferences. We found that while the reversed-reflection effect was present with small-magnitude payoffs, using payoffs of larger magnitude brought participants’ behavior back in line with the standard reflection effect. Our results suggest that risk preferences in experience-based decision-making are not only affected by the relative extremeness but also by the absolute extremeness of past events.
Similar content being viewed by others
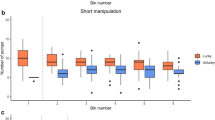
Introduction
Most studies to date on decision-making under risk have focused on one-shot decision paradigms, where participants are given explicit verbal descriptions about the outcomes and their associated probabilities for each choice alternative (e.g., Kahneman & Tversky, 1979; Tversky & Kahneman, 1992). The past 10 years or so have seen a surge of interest in experiential decision-making, where information about each alternative (i.e., outcomes and probabilities) is inferred/learned through repeated experience (see e.g., Barron & Erev, 2003). While these two paradigms present participants with identical information for each decision alternative, past research has highlighted robust contrasts regarding risk preferences and decision patterns between the two decision modalities, suggesting a description-experience “gap” in risky choice (Camilleri & Newell, 2011; Hertwig, Barron, Weber, & Erev, 2004; Hertwig & Erev, 2009).
The most well-studied aspect of the “gap” is the differential treatment of rare events (i.e., p < 0.20). For example, consider the choice between an option which returns $3 with certainty and a risky option which returns $4 with p = 0.8, and nothing otherwise. Here the rare event is the .20 chance of receiving nothing. In description-based choice, the majority of participants prefer the certain option because they treat rare events as if they had a higher probability of occurrence, suggesting an “overweighting” of those events. In contrast, experience-based studies have documented that rare events are “underweighted”, thus leading most people to choose the risky option in the above example (Hertwig et al., 2004).
Recently, Ludvig and Spetch (2011) showed that description and experience-based choice do not only diverge with regards to rare events, but also when the risky option offers equiprobable (50–50%) outcomes. In two experiments, they observed that in experience-based choice, participants are more risk-seeking (i.e., clear preference for the risky option) in the gain relative to the loss domain (see also Ludvig, Madan, & Spetch, 2014; Madan, Ludvig, & Spetch, 2014). This behavioral pattern is at odds with the well-documented reflection effect in description-based choice, which suggests a switch from risk-aversion in the gain domain to risk-seeking in the loss domain.Footnote 1 For example, most people prefer a certain win of $200 over a risky option offering $400 with 50% (gain domain; prospects have identical expected values). However, when these two prospects are framed as losses, people tend to prefer the risky option (hoping for a 50% chance of losing nothing) over the certain loss (see Kahneman & Tversky, 1979). This standard reflection effect is usually attributed to a non-linear utility function characterized by diminishing sensitivity. This function captures the idea that the subjective impact of change in the received payoffs diminishes with the distance from zero (as in the utility function of Prospect Theory, which is concave for gains and convex for losses; see Kahneman & Tversky, 1979). In contrast, the reversed-reflection effect, documented by Ludvig and colleagues, presents an inversion of the basic scheme of risk preferences observed in description-based choice. When participants learn the outcomes and probabilities of each choice option through repeated experience, they show higher selection rates from the risky option in the gain domain relative to the loss domain (where they prefer the certain medium loss, e.g., $200, over the risk of losing a higher amount, 50% of losing $400; see also Table 1).
The question then is what generates the reversed-reflection effect in experience-based choice? According to Madan et al. (2014), the effect is found because people tend to better recall salient past events and ascribe more importance to them. Particularly in the context of risky decision-making, this bias makes people more “sensitive to the biggest gains and losses they encounter” (Madan et al., 2014, p. 629). Considering the previous gamble, the prospect of winning $400 or losing the same amount (i.e., best and worst outcomes in the decision context, respectively) is overweighted in memory, thus leading to more selections from the risky option in the gain domain ($400, 0.5; 0 ≽ $200, 1), and at the same time, more selections from the certain option in the loss domain (-$400, 0.5; 0 ≺ -$200, 1).Footnote 2 The differential impact of extreme payouts is captured by what Madan, Ludvig, and Spetch (2017) describe as the extreme-outcome rule. In a nutshell, this rule states that people seek the option which returns the “best” outcome in a given context and avoid the option which returns the “worst” outcome.
One key characteristic of demonstrating the reversed-reflection effect in experience-based choice is the intermixing of gain and loss decision trials within the same experimental task: participants on each trial are presented with a choice pair (certain and risky options) framed as either gains or losses (the Method section and for more details see, Ludvig et al., 2014). This intermixing of gain and loss trials appears to be crucial because when participants are presented with decision trials in a block-wise manner (repeated play from the gain trials followed by the loss trials or vice-versa), then the standard reflection effect is observed (see Erev, Ert, & Yechiam, 2008; Ert & Yechiam, 2010; Ludvig et al., 2014).
However, even when the standard reflection effect is observed, it is moderated by the magnitude of the prospective outcomes, in a way that lower or higher nominal payoffs affect people’s risk preferences. For example, Erev et al. (2008) found in an experience-based choice experiment that when participants were presented with low nominal payoffs, they exhibited risk neutrality (i.e., no apparent preference for either the certain or the risky option, an effect that can be accounted for by a linear utility function), but when the payoffs were multiplied by a factor of 100, the diminishing sensitivity and the typical reflection effects were observed. The increase in risk aversion with high nominal payoffs is the basis of the “incentive” effect (Holt and Laury, 2002); according to this effect, people become relatively more risk averse when they are presented with higher payoff options. Similarly, the “peanuts” effect suggests that people prefer the risky option when the stakes are relatively low, thus becoming more risk averse with high-magnitude payoffs (e.g., Markowitz, 1952; Prelec & Loewenstein, 1991). The reflection of the “peanuts” effect in the loss domain indicates higher risk seeking with high nominal payoffs (Weber & Chapman, 2005).
The fact that the standard reflection effect is moderated by the magnitude of the prospective outcomes raises the question of how the reversed-reflection effect might be impacted by magnitude. Ludvig et al. (2014) observed the reversed-reflection effect using low-magnitude payoffs: a certain option which returned 20 points (or a loss of the same amount in the loss domain) and a risky option with a 50% chance of receiving 40 points (or a loss of the same points; and 0 otherwise). In fact, most of the experiments investigating the extreme-outcome rule used low-magnitude payoffs (see Ludvig, Madan, & Spetch, 2015; Ludvig & Spetch, 2011; Madan et al., 2014, 2017), showing risk neutrality/risk seeking in the gain domain and risk aversion in the loss domain. A strong interpretation of the extreme-outcome rule is that the magnitude of the prospective gains and losses in a given decision context should have no effect on people’s risk preferences; the most extreme outcomes should moderate decision-making behavior, regardless of how small or large they are. Based on the previous example, if the risky option offers 4,000 points instead of 40 points (or a loss of the same amount), it should not impact choice behavior, as people would seek the best outcome in the context (i.e., 4,000; hence showing risk seeking) and avoid the worst outcome (i.e., − 4,000; showing risk aversion, see Table 1).
Table 1 outlines the two competing hypotheses: based on diminishing sensitivity the magnitude of the nominal payoffs should moderate people’s risk preferences given the assumption that higher-magnitude payoffs should make participants more risk averse in the gain domain and more risk seeking in the losses domain. On the other hand, a strong interpretation of the extreme outcome rule predicts the same pattern of risk preferences regardless of outcome magnitude: more risk seeking in the gain domain relative to the loss domain. We set out to test these two contradictory accounts using the same methodology as in Ludvig et al.’s (2014) study; that is, presenting participants with intermixed gain and loss choices. Thus, the main question is whether the extreme outcome rule is truly insensitive to the magnitude of the offered payoffs.
Experiments 1 and 2
We present two experiments that investigate whether the extreme-outcome rule is invariant to changes in nominal payoff. The experiments described here are analogous to the design used by Ludvig et al. (2014).Footnote 3
Method
Participants
All participants were undergraduate psychology students and recruited via an online system (Experiment 1: N = 98, 71% Female, M a g e = 18.9 years, S D = 1.8; Experiment 2: N = 104, 65% Female, M a g e = 19.2 years, S D = 1.6). They were allocated course credit for participation. Participants in Experiment 2 could also earn a maximum of $16 based on their performance in the task.
Task and procedure
Participants completed a computerized experience-based choice task where the goal was to maximize the total number of points earned by clicking on colored squares. There were three types of trials: decision, catch, and single trials (see Fig. 1). In decision trials, participants were presented with two colored squares, which represented a choice between a certain and a risky option framed as either gains or losses. The certain option always returned the same payoff (+ / − 20,1) each time it was selected, whereas the risky option produced one of two equally likely payoffs (+ / − 40,0.5;0; both options have the same expected value = + / − 20). Hence, there were four possible color/option squares (i.e., gains-certain, gains-risky, losses-certain, losses-risky). The contingency between colors and options remained invariant throughout each experimental session but was randomized across participants. Participants were also presented with two colored squares that consisted of a choice between a gain option (certain or risky) and a loss option (certain or risky). These catch trials permitted an evaluation of whether participants had learned the correct color/option association. Following Ludvig et al. (2014), an exclusion criterion was set that removed any participants that failed to select the gain square on less than 60% of the catch trials.Footnote 4 Finally, participants were presented with only a single square (single trials). The square had to be clicked in order to continue and ensured that all squares had been experienced.
Schematic representation of the experimental design: a Decision trials (gain or loss domain) consisted of choices between a certain (+ / − 20,1) and a risky option (+ / − 40,0.5;0). b Catch trials consisted of a choice between a gain (certain or risky) and a loss option (certain or risky), and ensured that participants had learned the correct color/option association. c Single doors ensured that all options had been experienced
The main experimental manipulation was the magnitude of the prospective payoffs: Participants in both experiments were randomly assigned to one of four magnitude conditions where the original payoffs (M1 = 20; 40) were multiplied by a factor of 0.1 (M0.1 = 2; 4), 10 (M10 = 200; 400), or 100 (M100 = 2,000; 4,000).
Following Ludvig et al. (2014), participants completed five blocks of 48 trials. Each block randomly intermixed the three different trial types and consisted of 24 decision trials (12 gain; 12 loss), 16 catch trials, and eight single trials (each square presented twice) for a total of 240 trials. Squares were presented on each side of the screen equally often. Points were accrued or lost on each of the 240 trials, with the overall expected value on decision and single trials equal to 0. Only on catch trials could participants gain points. Once a square had been clicked, feedback was provided immediately, indicating how many points had been gained or lost, and stayed visible on the screen until participants clicked on a “Proceed” button to advance to the next trial of the task. No feedback about the unselected box was provided (partial feedback procedure). The points tally was updated and visible throughout the experiment.Footnote 5
During Experiment 1, participants accrued only points and were instructed to earn as many as possible throughout the session. Participants in Experiment 2 were similarly told to maximize the number of points earned, but that each point was also worth a nominal amount of money. The conversion rate in Experiment 2 was determined by the magnitude condition to which the participant was assigned. Specifically, participants in conditions M0.1, M1, and M10 received 0.1 cents per point whereas participants in the M100 condition received 0.01 cents per point.
These conversion rates, however, resulted in varying degrees of accumulated wealth across payoff conditions. To balance out the net earnings, each participant in Experiment 2 completed a second experimental session to top up their winnings. Participants were told that all accrued money from the first session was safe. For these “top up” sessions, the task was identical to that of the first session, only the color of the squares changed to avoid confusion with the previous set of colors. Each participant was assigned to a new magnitude condition. Specifically, participants in conditions M0.1 and M1 continued (randomly allocated) to either M10 or M100 (and vice-versa). The conversion rate remained unchanged between sessions.
Results
We are interested in two main aspects of the dataFootnote 6: first, is the reversed-reflection effect reliable under the conditions tested by Ludvig et al. (2014), and second, if so, does magnitude moderate participants’ risk preferences (see Table 1 for the pattern of results predicted by each account)? Fig. 2 presents the proportion of risky choices (PR) across the two domains (gain: PR G and loss: PR L ) and the four magnitude levels, separately for Experiments 1 and 2. An initial inspection of the figure suggests that magnitude appears to have an effect on people’s risk preferences (i.e., the relative heights of the green and red bars change as reward magnitude changes). We analyzed PR using a logit generalized mixed-effects modelFootnote 7 (Bates, Mächler, Bolker, & Walker, 2015), predicting selection of the risky option, with domain (gains, losses; within-subjects) and magnitude (four levels - polynomial contrasts; between-subjects) as fixed-effects and random intercepts for each participant. The main contrast of interest is the interaction (linear contrast) between domain and magnitude: according to the diminishing sensitivity hypothesis, we should find a pattern of results consistent with the extreme-outcome rule in the low-magnitude conditions (higher risk seeking in gains compared to losses), but we should see the standard reflection effect in the high-magnitude conditions.
The analysis of the Experiment 1 data revealed a significant interaction, χ 2(3) = 23.99, p < .001, suggesting that relative risk taking between the gain and loss domains is moderated by payoff magnitude. More specifically, we found that the linear contrast was significant, b = − 0.39, z = − 4.81,p < .001,Profile Likelihood (PL) 95% CI [− 0.58,− 0.23], suggesting a steady decrease of the reversed-reflection effect across magnitude conditions. In other words, while the reversed-reflection effect is observed in the low-magnitude conditions, the relative difference between risk taking in gains and losses shrinks as we move to payoffs of higher magnitude, and even reverses (i.e., back in line with the standard reflection effect - albeit, non-significant) in the highest-magnitude condition. We followed up the significant interaction with pairwise mixed-effects logit regressions (four in total; adjusting p values for multiple comparisons using the Benjamini-Hochberg procedure), comparing PR between the gain and the loss domain in each magnitude level: we found that in the two low-magnitude conditions the results are consistent with the extreme-outcome rule (M0.1: PR G −PR L , the difference in the PR between gain and loss domain = 9.27%, b = 0.45,z = 5.34,p < .001, PL 95% CI [ 0.28,0.61]; M1: PR G −PR L = 6.46%, b = 0.29,z = 3.68,p < .001, PL 95% CI [ 0.14,0.45]), showing more risk seeking in gains than losses, but not in the two high-magnitude conditions (M10: PR G −PR L = 3.20%, b = 0.16,z = 1.98,p = .06, PL 95% CI [ 0.001,0.32]; M100: PR G −PR L = − 1.93%, b = − 0.08,z = − 1.12,p = .26, PL 95% CI [ − 0.24,0.06]).
In Experiment 2, conducting the same analysis as in Experiment 1, we observed a significant interaction between domain and magnitude, χ 2(3) = 111.12, p < .001, with reliable linear and quadratic effects (b l i n e a r = − 0.62,z = − 8.07,p < .001, PL 95% CI [ − 0.77,− 0.47], b q u a d r a t i c = − 0.25,z = − 3.23,p < .001, PL 95% CI [ − 0.40,− 0.10]). We followed up the significant interaction with pairwise mixed-effects logit regressions: the reversed-reflection effect is present in conditions M0.1 (PR G −PR L = 11.28%, b = 0.49,z = 6.58,p < .001, PL 95% CI [0.35, 0.64]) and M10 (PR G −PR L = 8.53%, b = 0.41,z = 5.18,p < .001, PL 95% CI [0.25, 0.56]), suggesting higher risk seeking in the gain domain compared to the loss domain. Even though the results in condition M1 are not seemingly consistent with the extreme-outcome rule (i.e., the contrast did not reveal reliable differences; PR G −PR L = 1.18%, b = 0.04,z = 0.65,p = .52, PL 95% CI [ − 0.10,0.20]), it is important to note that we observed the reversed-reflection effect in this condition (thus, replicating the results in Ludvig et al., 2014) when we analyzed the last two choice blocks (PR G −PR L = 10.07%, b = 0.47, z = 3.68,p < .001). In the highest-magnitude condition, there was a reliable difference in risk taking behavior, but this time in the opposite direction, with more risk seeking in the loss domain compared to the gain domain (i.e., the standard reflection effect, see Table 1; PR G −PR L = − 11.54%, b = − 0.55,z = − 6.99,p < .001, PL 95% CI [ − 0.71,− 0.40]).
Figure 3 investigates the relationship between magnitude and relative risk taking behavior on the individual level. The plot shows the difference in the proportion of risky choices (PR G −PR L ) between the gain and loss domains across magnitude conditions in Experiments 1 and 2. The figure also presents the difference in the last block (triangle markers). It is evident that the proportion of participants showing behavior consistent with the extreme-outcome rule decreases as the magnitude of the prospective wins and losses increases. It is of interest to note that more participants show higher risk seeking for gains compared to losses in the last stages of the task, but in the highest-magnitude conditions, the proportion does not exceed 0.5.
Individual difference scores (PR G −PR L ) across magnitude conditions and experiments. Each marker represents a single participant. Red markers indicate higher risk seeking in gains than losses and blue markers indicate higher risk seeking in losses than gains. Circles represent difference scores across all experimental trials whereas triangles refer to the last experimental block. Numbers on top of each line of points represent the proportion of participants who showed behavior consistent with the predictions of the extreme-outcome rule. Darker colored markers indicate overlapping difference scores of multiple participants
General discussion
The extreme-outcome rule (or slight modifications thereof) offers a psychologically plausible and parsimonious explanation for a range of observed effects in experiential choice, including the reversed-reflection effect. It has been proposed as a possible explanation for behavioral effects where salient events tend to get overweighted (see e.g., Rigoli, Rutledge, Dayan, & Dolan, 2016; Tsetsos, Chater, & Usher, 2012; Zeigenfuse, Pleskac, & Liu, 2014), and as a rational means to account for limited cognitive resources in decision-making under risk and uncertainty (Lieder, Griffiths, & Hsu, 2017). In two experiments (experiment-point and financially incentivized), we tested the idea that the magnitude of prospective outcomes plays a role in determining risk preferences. To date, the extreme outcome rule has only been observed in contexts where the relative payoffs are of low magnitude. Despite this, the rule appears to imply that risk preferences should remain unchanged regardless of the magnitude of the payoffs. Consistent with the diminishing sensitivity assumption in Prospect Theory and analogous to documented effects in the literature (i.e., “incentive” and “peanuts”; see also Erev et al., 2008), we predicted that the absolute “extremeness” of extreme outcomes should moderate risk preferences. Our results supported this prediction and offer a new avenue for extending the discussion and explanations surrounding overweighting of extreme/salient events/outcomes.
We replicated Ludvig and Spetch’s (2011) original finding and observed the reversed-reflection effect using the same nominal payoffs. However, across both experiments risk preferences were not identical across all of the magnitude conditions. The primary effect, seen in both experiments, was a linearly decreasing proportion of risky choices in the gains domain as a function of payoff magnitude. Results from the Policy Task (which are presented in the Appendix) closely match the results and conclusions from the analyses of choice data in Experiments 1 and 2. This pattern was more pronounced in the second experiment when participants were incentivized with money rather than non-consequential points. Critically, in the highest-magnitude condition—where payoffs were multiplied by a factor of 100—there was evidence for a statistically significant reversal in risk preferences. Our primary finding, then, is that the evidence in favor of the extreme-outcome rule seems to diminish in contexts where the nominal payoffs are much larger, and that risk preference is not as simple as a tendency to favor the most extreme outcomes within a particular decision context. The absolute extremeness of payoffs seems to regulate risk preferences too, especially when incentivized payoffs are used.
Potential explanations and future directions
How should our results be interpreted in light of the competing accounts outlined in Table 1? One possibility is that rather than an “either /or”, the notion of a bias toward extreme outcomes and some form of diminishing sensitivity are required to explain the results. Assuming that choices in risky decisions from experience follow a (evidently) biased (for example, due to underweighting of rare events, see Hertwig & Erev, 2009) multiplicative integration (i.e., expected value calculation) of observed outcomes and learned probabilities (see Erev, Ert, Plonsky, Cohen, & Cohen, 2017; Gonzalez & Dutt, 2011), then a choice bias for the extreme events can be manifested either on the numerical value of the outcome (i.e., utility function), or the objective probabilities (i.e., probability weighting function), or it can affect both.
One explanation lies in the extreme events having an additive and constant effect on the utility of extreme outcomes across magnitude levels. Specifically, for each outcome we assume a power utility function with diminishing sensitivity, \(u(o_{i})=o_{i}^{\alpha }\) with 0 < α < 1, whereas for extreme outcomes, u(o E ) = (o E + κ)α, where κ is the constant bias added to the value of the extreme outcome. As diminishing sensitivity and concavity (convexity for losses) of the curve increases with the magnitude of the payoff, it can be shown that the absolute distance between (o E + κ)α and \(o_{E}^{\alpha }\) (that is, the “unbiased” utility of an extreme outcome) is closer to κ when payoff magnitude is low. The absolute size of κ determines the switch from relative extremity to absolute extremity driving choice and risk preferences. Alternatively, the value of κ may be determined by its own functional form, which could be a context-dependent utility transformation, u(o E ) = (o E + f(o E ))α.
The present results could also be explained via a single utility function with multiple inflection points (see Markowitz, 1952; Weber & Chapman, 2005). This is the idea that the utility function can be concave and convex within a domain (gains or losses) and magnitude defines the switch (i.e., inflection point) from one functional form to the other. For example, in the gain domain a convex utility function for small gains can account for increased risk seeking whereas for larger gains it becomes concave (accounting for risk aversion and showing diminishing sensitivity). Similarly, for small losses the utility function is concave (showing risk aversion) but it becomes convex with losses of higher magnitude. However, while this account can explain a magnitude effect, it leaves unaccounted evidence from previous research (e.g., Madan et al., 2014) which showed that extreme events are over-represented in measures of memory recall as compared to non-extreme events of equal expected value.
Alternatively, the overweighting of extreme events in memory might reflect or be the product of overestimation of objective probabilities of occurrence, in a way that the subjective decision weight for objective probability p = 0.50 is greater than .50, w(.50) > .50. This indicates that the extremity of these events makes them seem more probable than they are. The overestimation of p = 0.50 is inconsistent with Prospect Theory which assumes underweighting of medium probability events (see also Abdellaoui, Bleichrodt, & L’Haridon, 2008). However, previous research in decisions from experience has found that the relative frequency of extreme events (as compared to non-extremes) is overestimated (see Madan et al., 2014). If the effect of extreme outcomes on subjective probabilities across magnitude levels is constant (i.e., no interactions between utility and probability weighting), then in light of the present results, our magnitude manipulation appears to affect the shape of the utility function, with outcomes of higher magnitude leading to strong diminishing sensitivity. This is consistent with findings in decisions from experience (see Erev et al., 2008; Yechiam & Busemeyer, 2005; Yechiam & Ert, 2007), suggesting linear utility functions with low-magnitude outcomes (i.e., α = 1), but strong diminishing sensitivity with high-magnitude outcomes. The notion that the extremity of an outcome distorts and weights each outcome’s associated probability is a core component of Lieder et al.’s (2017) utility-weighted sampling model.
Future research can shed more light on the psychological and behavioral plausibility of the aforementioned explanations. One interesting avenue is to collect retrospective memory judgments on the occurrence of these events for each magnitude level (as in Madan et al., 2014, 2017). This will provide further evidence as to which account can capture the interaction between relative extreme events and absolute outcome magnitude. One important consideration pertinent to the aforementioned accounts is that they assume that participants have a complete and perfect representation of each option’s outcomes and associated probabilities (in other words, perfect learning and memory). However, this is hardly the case as previous research in decisions from experience has shown that choice behavior is moderated by recency effects (e.g., Hertwig et al., 2004), sequential dependencies (e.g., Plonsky, Teodorescu, & Erev, 2015), exploration versus exploitation (e.g., Mehlhorn et al., 2015), and the search for predictable outcome patterns (e.g., Ashby et al., 2017; Shanks, Tunney, & McCarthy, 2002). To that end, future work would benefit from the inclusion of computational modeling approaches which can provide insight into the interplay between memory biases, diminishing sensitivity, recency effects, and the possible differential weighting of extreme outcomes and extreme probabilities. Erev et al. (2008) incorporated a utility function in their model of experience based choice (see also Ahn, Busemeyer, Wagenmakers, & Stout, 2008; Speekenbrink & Konstantinidis, 2015; Yechiam & Busemeyer, 2005), and noted that “the addition of diminishing sensitivity assumption to models that assume oversensitivity to small samples can improve the value of these models” (p. 587). Our results corroborate this statement by reinforcing the necessity to include utility functions in any future attempt to model specific effects in decisions from experience.
Notes
This applies to situations where the probabilities are high. When probabilities are small, the opposite pattern is observed: risk seeking in the gain domain and risk aversion in the loss domain, leading to the well-documented four-fold pattern of risk preferences in description-based decision-making (see e.g., Tversky & Fox, 1995).
For the demonstration of two-outcome gambles we use the notation “ x,p; y” which indicates that outcome x occurs with probability p, and outcome y with probability 1 − p. For choice between two options and preference notation, we use the following symbols: A | B indicates a choice between A or B; \(\thicksim \) for indifference; ≽ or ≼ for weak preference; ≻ or ≺ for strong preference.
The only aesthetic differences between our experiments and Ludvig et al.’s (2014) experiments were that (a) instead of presenting pictures of colored doors, our participants were presented with colored squares, and (b) we did not utilize cartoon graphics (e.g., a pot of gold for a positive outcome and a thief for a negative outcome) for the presentation of the outcome feedback. This was presented in black font (regardless of whether it was a positive or a negative outcome) after participants had clicked a colored square. Experiment scripts, datasets, and analysis scripts are available online on the Open Science Framework (OSF) website: https://osf.io/z57tn/
At the end of the repeated-choice experiment, participants were given a policy setting task where they could distribute their choices across 100 imaginary future and non-consequential trials. This constitutes a preference elicitation method whereby exploration and/or payoff pattern exploitation are irrelevant (see Ashby, Konstantinidis, & Yechiam, 2017). Participants were shown two options (as in the decision trials setting) and told that they would have to make another 100 choices, but this time indicating what proportion of the 100 choices they would allocate to the certain or risky options. The policy task was presented twice, separately for gains and losses options. Each option was presented with a default value of 50 trials. Results from this assessment are presented in the Appendix.
We note here that while participants in Experiment 2 completed two experimental sessions, we consider only the data from the first session. The reason for this is that the context for the second session had changed dramatically and each participant began the second session with varying degrees of accumulated wealth. These changes make predictions about the nature of context and what now counts as an extreme outcome rather complex. We thus refrain from speculative and exploratory analyses of these data. The data are, however, available on OSF.
We did not use the traditional general linear model type of analyses (e.g., ANOVA and t test) because the dependent variable is binary/categorical.
References
Abdellaoui, M, Bleichrodt, H, & L’Haridon, O (2008). A tractable method to measure utility and loss aversion under prospect theory. Journal of Risk and Uncertainty, 36, 245–266. https://doi.org/https://doi.org/10.1007/s11166-008-9039-8
Ahn, W.-Y., Busemeyer, J R, Wagenmakers, E. - J., & Stout, J C (2008). Comparison of decision learning models using the generalization criterion method. Cognitive Science, 32, 1376–1402. https://doi.org/10.1080/03640210802352992
Ashby, N J S, Konstantinidis, E, & Yechiam, E (2017). Choice in experiential learning: True preferences or experimental artifacts? Acta Psychologica, 174, 59–67. https://doi.org/10.1016/j.actpsy.2017.01.010
Barron, G, & Erev, I (2003). Small feedback-based decisions and their limited correspondence to description-based decisions. Journal of Behavioral Decision Making, 16, 215–233. https://doi.org/10.1002/bdm.443
Bates, D, Mächler, M., Bolker, B, & Walker, S (2015). Fitting linear mixed-effects models using lme4. Journal of Statistical Software, 67, 1–48. https://doi.org/10.18637/jss.v067.i01
Camilleri, A R, & Newell, B R (2011). When and why rare events are underweighted: A direct comparison of the sampling, partial feedback, full feedback and description choice paradigms. Psychonomic Bulletin & Review, 18, 377–384. https://doi.org/10.3758/s13423-010-0040-2.
Erev, I, Ert, E, Plonsky, O, Cohen, D, & Cohen, O (2017). From anomalies to forecasts: Toward a descriptive model of decisions under risk, under ambiguity, and from experience. Psychological Review, 124, 369–409. https://doi.org/10.1037/rev0000062
Erev, I, Ert, E, & Yechiam, E (2008). Loss aversion, diminishing sensitivity, and the effect of experience on repeated decisions. Journal of Behavioral Decision Making, 21, 575–597. https://doi.org/10.1002/bdm.602
Ert, E, & Yechiam, E (2010). Consistent constructs in individuals? risk taking in decisions from experience. Acta Psychologica, 134, 225–232. https://doi.org/10.1016/j.actpsy.2010.02.003
Gonzalez, C, & Dutt, V (2011). Instance-based learning: Integrating sampling and repeated decisions from experience. Psychological Review, 118, 523–551. https://doi.org/10.1037/a0024558
Hertwig, R, Barron, G, Weber, E U, & Erev, I (2004). Decisions from experience and the effect of rare events in risky choice. Psychological Science, 15, 534–539. https://doi.org/10.1111/j.09567976.2004.00715.x
Hertwig, R, & Erev, I (2009). The description-experience gap in risky choice. Trends in Cognitive Sciences, 13, 517–523. https://doi.org/10.1016/j.tics.2009.09.004
Holt, C A, & Laury, S K (2002). Risk aversion and incentive effects. American Economic Review, 92, 1644–1655. https://doi.org/10.1257/000282802762024700
Kahneman, D, & Tversky, A (1979). Prospect theory: An analysis of decision under risk. Econometrica, 47, 263–291. https://doi.org/10.2307/1914185
Lieder, F, Griffiths, T L, & Hsu, M (2017). Overrepresentation of extreme events in decisions making reflects rational use of cognitive resources. Psychological Review. Advance online publication. https://doi.org/10.1037/rev0000074.
Ludvig, E A, Madan, C R, & Spetch, M L (2014). Extreme outcomes sway risky decisions from experience. Journal of Behavioral Decision Making, 27, 146–156. https://doi.org/10.1002/bdm.1792.
Ludvig, E A, Madan, C R, & Spetch, M L (2015). Priming memories of past wins induces risk seeking. Journal of Experimental Psychology: General, 144, 24–29. https://doi.org/10.1037/xge0000046
Ludvig, E A, & Spetch, M L (2011). Of black swans and tossed coins: Is the description-experience gap in risky choice limited to rare events?. PLoS ONE, 6, e20262. https://doi.org/10.1371/journal.pone.0020262
Madan, C R, Ludvig, E A, & Spetch, M L (2014). Remembering the best and worst of times: Memories for extreme outcomes bias risky decisions. Psychonomic Bulletin & Review, 21, 629–636. https://doi.org/10.3758/s13423-013-0542-9
Madan, C R, Ludvig, E A, & Spetch, M L (2017). The role of memory in distinguishing risky decisions from experience and description. The Quarterly Journal of Experimental Psychology, 70, 2048–2059.
Markowitz, H (1952). The utility of wealth. Journal of Political Economy, 60, 151–158. https://doi.org/10.1086/257177
Mehlhorn, K., Newell, B. R., Todd, P. M., Lee, M. D., Morgan, K., Braithwaite., V. A, ... Gonzalez, C (2015). Unpacking the exploration-exploitation tradeoff: A synthesis of human and animal literatures. Decision, 2, 191–215. https://doi.org/10.1037/dec0000033
Plonsky, O, Teodorescu, K, & Erev, I (2015). Reliance on small samples, the wavy recency effect, and similarity-based learning. Psychological Review, 122, 621–647. https://doi.org/10.1037/a0039413
Prelec, D, & Loewenstein, G (1991). Decision making over time and under uncertainty: A common approach. Management Science, 37, 770–786. https://doi.org/10.1287/mnsc.37.7.770
Rigoli, F, Rutledge, R B, Dayan, P, & Dolan, R J (2016). The influence of contextual reward statistics on risk preference. NeuroImage, 128, 74–84. https://doi.org/10.1016/j.neuroimage.2015.12.016.
Shanks, D R, Tunney, R J, & McCarthy, J D (2002). A re-examination of probability matching and rational choice. Journal of Behavioral Decision Making, 15, 233–250. https://doi.org/10.1002/bdm.413
Speekenbrink, M, & Konstantinidis, E (2015). Uncertainty and exploration in a restless bandit problem. Topics in Cognitive Science, 7, 351–367. https://doi.org/10.1111/tops.12145
Tsetsos, K, Chater, N, & Usher, M (2012). Salience driven value integration explains decision biases and preference reversal. Proceedings of the National Academy of Sciences, 109, 9659–9664. https://doi.org/10.1073/pnas.1119569109
Tversky, A, & Fox, C R (1995). Weighing risk and uncertainty. Psychological Review, 102, 269–283. https://doi.org/10.1037/0033-295X.102.2.269
Tversky, A, & Kahneman, D (1992). Advances in prospect theory: Cumulative representation of uncertainty. Journal of Risk and Uncertainty, 5, 297–323. https://doi.org/10.1007/BF00122574
Weber, B J, & Chapman, G B (2005). Playing for peanuts: Why is risk seeking more common for low-stakes gambles? Organizational Behavior and Human Decision Processes, 97, 31–46. https://doi.org/10.1016/j.obhdp.2005.03.001
Yechiam, E, & Busemeyer, J R (2005). Comparison of basic assumptions embedded in learning models for experience-based decision making. Psychonomic Bulletin & Review, 12, 387–402. https://doi.org/10.3758/BF03193783
Yechiam, E, & Ert, E (2007). Evaluating the reliance on past choices in adaptive learning models. Journal of Mathematical Psychology, 51, 75–84. https://doi.org/10.1016/j.jmp.2006.11.002
Zeigenfuse, M D, Pleskac, T J, & Liu, T (2014). Rapid decisions from experience. Cognition, 131, 181–194. https://doi.org/10.1016/j.cognition.2013.12.012
Acknowledgements
We thank Alexis Porter, Adeline Siva, and Emma Williams for their help in collecting the data. We also thank two anonymous reviewers for helpful comments and constructive suggestions that improved the paper.
Author information
Authors and Affiliations
Corresponding author
Additional information
Open Access
This article is distributed under the terms of the Creative Commons Attribution 4.0 International License (http://creativecommons.org/licenses/by/4.0/), which permits unrestricted use, distribution, and reproduction in any medium, provided you give appropriate credit to the original author(s) and the source, provide a link to the Creative Commons license, and indicate if changes were made.
This research was supported by an Australian Research Council Discovery Project Grant (DP140101145).
Appendix: Policy Task
Appendix: Policy Task
Inspection of the data from the policy task largely confirms the patterns observed in the choice task (see Fig. 4). In Experiment 1, the analysis (logit generalized mixed-effects model with domain and magnitude as fixed effects and random intercepts for each participant) revealed a significant magnitude × domain interaction, χ 2(3) = 160.51,p < .001, indicating that participants’ policies were consistent with the reversed-reflection effect in the small-magnitude conditions (M 0.1 and M 1), but they were in line with the typical reflection effect in the large-magnitude conditions (M 10 and M 100; all mixed-effects t tests were significant, p < .05).
In Experiment 2, the interaction between magnitude and domain was significant, χ 2(3) = 142.50,p < .001, which was mainly driven by the differences in the smallest and largest-magnitude conditions (M 0.1 and M 100,p < .001). This pattern is consistent with the idea that the magnitude moderates risk preferences. The middle conditions, M 1 and M 10, did not reveal any difference between policies in the gain and loss domains.
Rights and permissions
Open Access This article is distributed under the terms of the Creative Commons Attribution 4.0 International License (http://creativecommons.org/licenses/by/4.0/), which permits unrestricted use, distribution, and reproduction in any medium, provided you give appropriate credit to the original author(s) and the source, provide a link to the Creative Commons license, and indicate if changes were made.
About this article
Cite this article
Konstantinidis, E., Taylor, R.T. & Newell, B.R. Magnitude and incentives: revisiting the overweighting of extreme events in risky decisions from experience. Psychon Bull Rev 25, 1925–1933 (2018). https://doi.org/10.3758/s13423-017-1383-8
Published:
Issue Date:
DOI: https://doi.org/10.3758/s13423-017-1383-8