- 1Department of Civil, Environmental, and Infrastructure Engineering, George Mason University, Fairfax, VA, United States
- 2Department of Geography and Geoinformation Science, George Mason University, Fairfax, VA, United States
- 3Department of Mathematics, George Mason University, Fairfax, VA, United States
As vegetation regulates water, carbon, and energy cycles from the local to the global scale, its accurate representation in land surface models is crucial. The assimilation of satellite-based vegetation observations in a land surface model has the potential to improve the estimation of global carbon and energy cycles, which in turn can enhance our ability to monitor and forecast extreme hydroclimatic events, ecosystem dynamics, and crop production. This work proposes the assimilation of a remotely sensed vegetation product (Leaf Area Index, LAI) within the Noah Multi-Parameterization land surface model using an Ensemble Kalman Filter technique. The impact of updating leaf mass along with LAI is also investigated. Results show that assimilating LAI data improves the estimation of transpiration and net ecosystem exchange, which is further enhanced by also updating the leaf mass. Specifically, transpiration anomaly correlation coefficients improve in about 77 and 66% of the global land area thanks to the assimilation of leaf area index with and without updating leaf mass, respectively. Random errors in transpiration are also reduced, with an improvement of the unbiased root mean square error in 70% (74%) of the total area without the update of leaf mass (with the update of leaf mass). Similarly, net ecosystem exchange anomaly correlation coefficients improve from 52 to 75% and random errors improve from 49 to 62% of the total pixels after the update of leaf mass. Better performances for both transpiration and net ecosystem exchange are observed across croplands, but the largest improvement is shown over forests and woodland. The global scope of this work makes it particularly important in data poor regions (e.g., Africa, South Asia), where ground observations are sparse or not available altogether but where an accurate estimation of carbon and energy variables can be critical to improve ecosystem and crop management.
Introduction
Vegetation regulates water, carbon, and energy cycles by supporting critical functions in the biosphere. Vegetation dynamics therefore play a crucial role in land surface modeling (Littell et al., 2011) and are commonly represented using dynamic vegetation models (DVMs; Dai et al., 2003; Clark et al., 2011; Peterson et al., 2014; Wullschleger et al., 2014). For instance, the Noah Multi-Parameterization LSM (hereinafter Noah-MP; Niu et al., 2011) uses multiple options for key land hydrologic processes, along with the Balls Berry scheme, a dynamic vegetation module that allocates carbon to various parts of vegetation and soil carbon pools. If input forcing data and parameters are available at the global scale, LSMs and DVMs can be run in combination to estimate water, carbon, and energy variables globally. Nevertheless, such model simulations are prone to errors because of inaccurate initialization, mis-specified forcing data and parameters, or inadequate model physics (Bonan et al., 2019).
Satellite observations can be a valid alternative to model estimates and can be adopted for monitoring vegetation globally. Satellite observations, based on spectral reflectance measurements acquired in the red and near-infrared regions, produce maps of vegetation indices such the Leaf Area Index (LAI), defined as the one-sided leaf surface area measured over unit ground, and the Normalized Difference Vegetation Index (NDVI). LAI is recognized as a useful indicator of the exchange of water vapor and CO2 between the vegetation canopy and atmosphere (Xiao et al., 2016; Albergel et al., 2017), whereas NDVI is an indicator of the density of green vegetation on a patch of land (Yang et al., 2012). Some examples include the Moderate Resolution Imaging Spectroradiometer (MODIS), which has been acquiring data in 36 spectral bands since 2000 (Rees and Danks, 2007) and the Advanced Very High-Resolution Radiometer (AVHRR; Tucker et al., 2005), which produces global maps of LAI at a resolution of 4 km every 10 days.
Nevertheless, satellite-based observations have gaps in their spatial and temporal coverage (often due to cloud coverage). To fill in such gaps and to guarantee continuous time series, observations are often merged with model simulations. A well-known technique for optimally combining the information from such observations and model estimates based on their respective uncertainties is known as Data Assimilation (DA) (Rodell et al., 2004; Dee, 2005). In the past decades, Land Data Assimilation Systems (LDASs) have been successfully used to merge satellite observations of soil moisture and surface temperature into LSMs (e.g., Reichle, 2008; Reichle et al., 2008; Maggioni and Houser, 2017) and, in the recent past, researchers have applied the same concept to vegetation observations and vegetation dynamics models.
For instance, Albergel et al. (2010) jointly assimilated observations of LAI and Surface Soil Moisture (SSM) within a LSM over western France and showed a positive impact on the estimation of carbon, water, and heat fluxes. Barbu et al. (2011) developed an LSM to jointly assimilate soil moisture and LAI data and to simulate photosynthesis processes, surface carbon fluxes, and vegetation biomass. Nearing et al. (2012) assimilated remote sensing observations of LAI and SSM for wheat yield estimates with an observing system simulation experiment using ensemble Bayesian state-updating filters for mitigating modeling uncertainty on end-of-season wheat yield estimates. A study by Huang et al. (2015) jointly assimilated MODIS LAI and evapotranspiration (ET) products into the soil water atmosphere plant (SWAP) model for winter wheat yield estimation. In another study by Albergel et al. (2017), a global land data assimilation system (LDAS-Monde) was applied over Europe and the Mediterranean basin to improve land surface variable estimation when SSM and LAI satellite-derived observations were assimilated using a Simplified Extended Kalman Filter. Although being more effective in estimating soil moisture in the top-soil layers LDAS-Monde had less sensitivity to SSM with depth and had almost no impact below 60 cm. Bonan et al. (2019) jointly assimilated SSM and LAI within LDAS-Monde over the Euro-Mediterranean region using an ensemble square root filter (EnSRF) DA technique. All these previous studies assimilated vegetation indices over local domain or small crop field to improve the estimation of crop yields.
Some recent work has successfully assimilated Global LAnd Surface Satellite (GLASS; Liang et al., 2013) LAI within a land surface model across larger domains. For instance, Kumar et al. (2019) merged GLASS LAI observations with Noah-MP estimates over CONUS and observed beneficial impacts on several water budget variables such as soil moisture, ET, snow depth, and streamflow. Furthermore, the assimilation of LAI improved the estimation of gross primary production and net ecosystem exchange. Another work by Ling et al. (2019) assimilated GLASS LAI into the Community Land Model with carbon and nitrogen components (CLM4CN) using an Ensemble Adjustment Kalman Filter. This experiment showed improvements in ET and gross primary production. Albergel et al. (2020) has jointly assimilated SSM and LAI using the Simplified Extended Kalman Filter data assimilation technique to predict the impact of extreme events like heatwaves and droughts on land surface conditions over the globe. They have used LDAS-Monde as the land surface model and assimilated ASCAT soil water index (SWI) and LAIGEOV1 LAI observation data within that model. Zhang et al. (2020) proposed a global synthetic experiment to assimilate LAI within Noah-MP using an EnKF. They showed that LAI assimilation can improve global water fluxes and reduce the impact of high precipitation biases in the estimation of water variables. Rahman et al. (2020) showed that even the simplest LAI data assimilation technique (e.g., direct insertion) can improve the estimation of water, energy, and carbon variables.
Building on previous literature, this work proposes to assimilate satellite GLASS LAI observations within the Noah-MP land surface model at the global scale using an EnKF. This work investigates the impact of updating an additional prognostic variable (leaf mass) along with LAI at every time step when an observation becomes available on a set of water, energy, and carbon variables.
Methodology
In this work the Noah-MP Land Surface Model is used to test the hypothesis that by combining satellite LAI observations within a land surface model using a data assimilation technique, the estimation of water and carbon cycle variables can be improved. The experiment is performed during 2011 over the global domain (Figure 1).
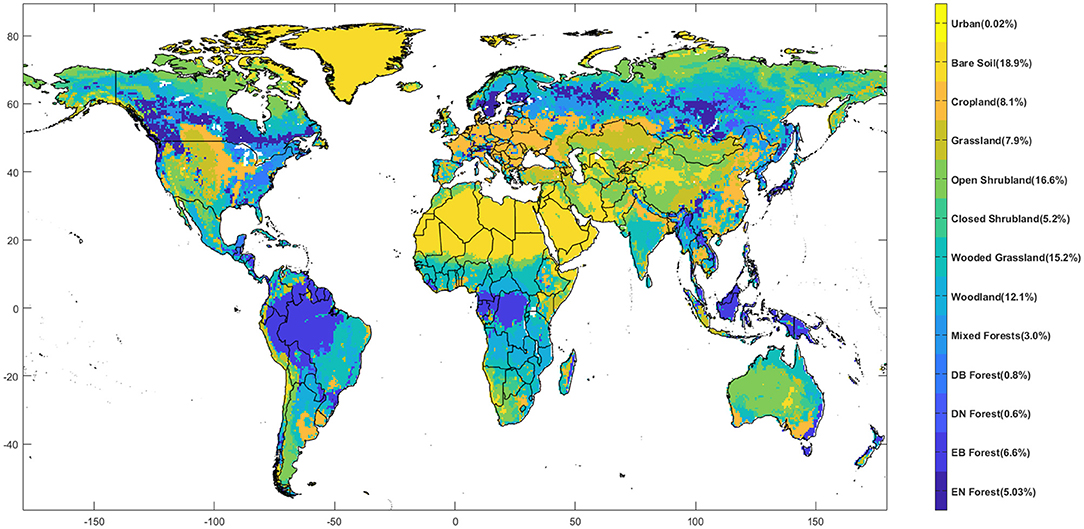
Figure 1. Land surface classification for the study region by UMD Land Cover Classification used in the Noah-MP model. The percentage area for each class is shown in the legend (EN, Evergreen Needleleaf; EB, Evergreen Broadleaf; DN, Deciduous Needleleaf; DB, Deciduous Broadleaf).
The Noah-MP Land Surface Model
In the Noah-MP model the canopy layer is separated from the land surface, which is called a semi-tile sub grid scheme (Niu et al., 2011). The shortwave radiation transfer is computed over the entire grid cell, while longwave radiation, latent heat, sensible heat, and ground heat fluxes are computed separately over two tiles: the fractional vegetated area and the fractional bare ground area. Noah-MP avails multiple options for surface water infiltration, runoff, groundwater transfer and storage, dynamic vegetation, canopy resistance, and frozen soil physics (Niu and Yang, 2007). Furthermore, the Ball-Berry scheme (Ball et al., 1987) allocates carbon to vegetation leaves, stems, wood, and roots and to soil carbon fast and slow pools.
In this work, Noah-MP is forced with the Modern-Era Retrospective analysis for Research and Applications, version 2 (MERRA-2; Gelaro et al., 2017) dataset. MERRA-2 provides data since 1980 over the globe. The advancement in the assimilation system replaces the original MERRA dataset that enables the merging of modern hyperspectral radiance and microwave observations, along with GPS-Radio Occultation dataset. The spatial resolution of MERRA-2 is 0.5°/0.625° in the latitudinal/longitudinal and the temporal resolution is hourly. Atmospheric temperature, radiation, water vapor, precipitation, wind speed, topography, altitude are the forcing variables from the MERRA-2 dataset used to force Noah-MP in this study.
Slope type, vegetation effect on soil heat, soil evaporation, soil heat capacity, surface runoff parameterization, depth of lower boundary, and soil temperature are the general model parameters in Noah-MP 3.6. This version of the model also uses some soil parameters such as saturation soil moisture content, soil conductivity, soil diffusivity, wilting point of soil moisture and some vegetation parameters such as- green vegetation fraction, rooting depth, stomatal resistance, minimum and maximum leaf area index, minimum and maximum background emissivity, minimum and maximum background albedo, minimum and maximum background roughness, optimum transpiration in air temperature, canopy water capacity etc.
The NASA Land Information System (LIS; Kumar et al., 2006) is used in this work to run the Noah-MP model and implement the data assimilation scheme. LIS provides a portable, extensible, and flexible infrastructure for testing our hypothesis. The common framework provided by LIS is capable of ensemble land surface modeling on grid points across the global land. The high-resolution capability of LIS and its large flexibility makes it the perfect framework to perform the experiment proposed here.
The Global LAnd Surface Satellite LAI
The satellite-based LAI product merged within the land surface model is derived from the GLASS product. GLASS LAI observations are generated using a general regression neural network approach that produces a long-term, spatially and temporally consistent record of vegetation conditions (Xiao et al., 2016). This dataset includes LAI data from two sources (MODIS and AVHRR) with a spatial resolution of 0.05° and 500 m and a temporal resolution of 4–8 days. The product derived from MODIS is available for 2000–2018, whereas the AVHRR data are available for 1981–2018.
Because of the improved spatiotemporal coverage of the GLASS product, it is preferred to standard MODIS- or AVHRR-based LAI, which are affected by cloud obscuration gaps. Although limited over (Liang et al., 2013)complex terrain (Jin et al., 2017), the performance of GLASS LAI was found comparable to other satellite LAI products, e.g., MODIS, GEOV1, GLOBMAP, and JRC-TIP (Fang et al., 2013) and consistent with independent ground observations (Xiao et al., 2016). MODIS-based GLASS LAI V50 product is used in this work. The spatial and temporal resolution of this product is 0.05° and 8 days, respectively. In this work, a temporal interpolation is used to generate the data for everyday resolution.
Validation Dataset
A variety of ground observations is used for validating the proposed system, as an independent dataset for comparison with the land surface model outputs from different experiments. These observations are obtained from two main sources: the Global Land Evaporation Amsterdam Model (GLEAM) and the FLUXCOM datasets.
GLEAM is a set of algorithms that separately estimate the different components of land evaporation (or evapotranspiration): transpiration, bare-soil evaporation, interception loss, open-water evaporation, and sublimation (Miralles et al., 2011; Martens et al., 2017). The GLEAM dataset, which also includes other variables (like soil moisture), is freely available and continuously revised and updated since its development in 2011. GLEAM evapotranspiration data are derived based on the Priestley and Taylor's evaporation definition and use remote sensing observations of near surface temperature and surface net radiation (Miralles et al., 2011; Frappart, 2020). Because of their global and spatially continuous coverage, GLEAM data are preferred in this study to in-situ datasets (e.g., FLUXNET, AU-ASM), traditionally considered to be more reliable. Moreover, GLEAM products showed good agreement with several ground-based observations (Martens et al., 2017; Jung et al., 2019a). Specifically, GLEAM version 3.3b, adopted here for validating the proposed DA system, was introduced in 2017 and is available globally from 2003 to 2018, at a temporal/spatial resolution of 1 day/0.25°.
The FLUXCOM dataset is derived from a combination of FLUXNET site observations, satellite remote sensing, and meteorological data. A machine learning technique is used for estimating the Net Ecosystem Exchange (NEE), defined as the amount of carbon exchange between plant and atmosphere. FLUXCOM shows a large carbon sink in the tropics and lacks the effect of CO2 fertilization (Jung et al., 2020). Despite these limitations, current FLUXCOM estimates of seasonal NEE provide useful constraints for the global carbon cycle (Tramontana et al., 2016; Jung et al., 2019b). FLUXCOM was chosen here for its global coverage, multiple data sources, and tempo-spatial resolution of 1 day/0.5° during 1980–2013.
The Data Assimilation System
The Ensemble Kalman Filter (EnKF) is used as the DA technique in this experiment. EnKF is an approximated version of the traditional Kalman filter. It is based on Monte Carlo simulation. In EnKF, the state distribution is represented by a sample (or “ensemble”) of the distribution, which is propagated forward in time and updated when a new observation becomes available (Reichle et al., 2002, 2008; Katzfuss et al., 2016). The EnKF technique has flexibility in treating errors in model equations and parameters and is particularly suitable for non-linear problems, such as soil dynamics. These are the major advantages of using an EnKF in land surface modeling (Pan and Wood, 2006; Kumar et al., 2008).
In this work, twenty ensemble members are generated by perturbing the atmospheric forcing. Following previous work (Kumar et al., 2019; Zhang et al., 2020), selected MERRA-2 atmospheric variables, such as shortwave and longwave radiation and precipitation, are perturbed hourly. Multiplicative perturbations are applied to the shortwave radiation and precipitation with a mean of 1 and standard deviations of 0.3 and 0.5, respectively. The longwave radiation is perturbed with an additive perturbation method with a standard deviation of 50 W/m2. The perturbations of all the three meteorological variables are cross correlated: the cross correlation between shortwave radiation and precipitation is set to −0.8, the cross correlation between longwave radiation and precipitation to 0.5, and the cross correlation between shortwave and longwave radiations to −0.5. The GLASS LAI observations are perturbed via an additive model with a standard deviation of 0.1. In this experiment, the EnKF is used to update not only LAI, but also an additional model prognostic variable (i.e., leaf mass).
Thus, three main experiments are run: (i) an open-loop (OL) simulation, in which no assimilation is performed; (ii) the model LAI state alone is updated whenever GLASS LAI is available; and (iii) the model leaf mass state is also updated along with LAI, whenever GLASS LAI is available. Figure 2 presents a schematic framework of the experimental setup.
System Evaluation
Daily transpiration, NEE, and soil moisture from the OL and the two DA simulations are compared to the corresponding in-situ references, described in section Validation Dataset. Specifically, the ground-based measurements from the FLUXCOM and GLEAM datasets are used to validate modeled transpiration and NEE, respectively. To ease the validation procedure, the ground-based data are converted to the resolution of the model outputs (0.5° × 0.625°) by simply averaging all points falling within the model grid cell (Johnston, 2021).
Satellite observations, model simulations, and the validation dataset are intrinsically different in nature and therefore systematic biases are inevitable. However, to avoid any manipulation of the data prior to the assimilation procedure, the system is only evaluated based on statistical metrics that are minimally affected by systematic errors: the daily Anomaly Correlation Coefficient (ACC) and the unbiased Root Mean Square Error (uRMSE). ACC and uRMSE are calculated for the OL and DA model outputs with respect to the validation datasets.
ACC is computed based on anomalies, defined as differences between the daily values and the yearly climatological average. Each of the anomaly time series is computed relative to the mean of its respective model run. ACC is defined as follows:
where represents the output from the model (OL or DA), Xobs is the corresponding reference variable, and N is the number of days. The ACC metric captures the correspondence in phase between model estimates and the reference data, regardless of potential mean biases or differences in dynamic range (Entekhabi et al., 2010).
The unbiased Root Mean Square Error (uRMSE) is adopted here as a measure of the random error between the model estimate and the reference. Model outputs are first multiplied by a factor to remove the bias between estimates and observations. Bias factors (bf) are calculated for each pixel within the study area as follows (Tesfagiorgis et al., 2011):
where, is the time averaged output from the model (OL or DA) at each pixel, and is the time averaged value of the corresponding reference variable (Xobs). The model outputs (OL or DA) are multiplied by the bias factor to generate an adjusted model output :
The uRMSE is then computed based on the two unbiased time series as follows:
where N is the number of days.
To investigate the improvement (or degradation) in ACC due to DA, the difference between ACC in DA run and OL is computed as follows:
Similarly, to determine the improvement (or degradation) in uRMSE due to DA, the difference between the uRMSE of OL run and the one from the DA run is assessed:
If the differences in ACC are positive (negative), the DA output has higher (lower) correlation; whereas, for uRMSE, positive (negative) difference values show lower (higher) errors in DA compared to OL.
Mean ACCs and uRMSEs are calculated over different vegetation covers based on UMD land cover classification (Figure 1). Four major vegetation covers are considered in this analysis: Forest and Woodland, Shrubland, Grassland, and Cropland. For both ACC and uRMSE, 95% Confidence Intervals (CIs) are also calculated over different vegetation covers:
where, n is the number of grids for a specific vegetation cover and ts is the T-score, computed here for the 95% confidence level.
Results
As a first step, the impact of globally assimilating GLASS LAI with respect to a regular run of the Noah-MP model (i.e., the OL simulation) is investigated and the two DA methods (with and without updating the leaf mass together with LAI) are compared. Next, ACC is calculated for both OL and DA runs with respect to the reference datasets, i.e., the GLEAM transpiration and the FLUXCOM NEE products. Then, the DA performance is investigated based on different vegetation covers.
The Impact of LAI DA
Percentage changes in carbon (LAI, Leaf mass, and NEE) and water variables (Transpiration, SSM, and RZSM) between the DA run (without updating leaf mass) and the OL simulation are shown in Figure 3. The red (blue) color shows where the estimate from the OL is higher (lower) than the one from the DA simulation of the variables. Darker color tones highlight locations where the impact of DA is larger and lighter tones show where the difference between the two simulations is smaller. The assimilation of GLASS LAI has larger impact on the vegetation related variables when compared to soil moisture variables.
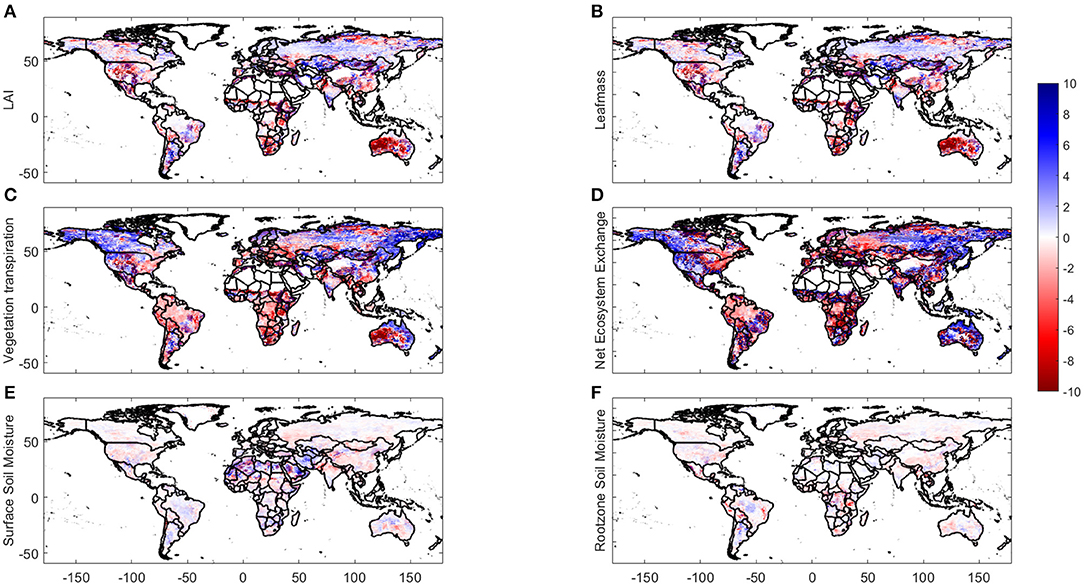
Figure 3. Percentage difference in the estimation of carbon variables between DA and OL without updating leaf mass: (A) LAI; (B) leaf mass; (C) transpiration; (D) NEE; (E) SSM; and (F) RZSM.
Similarly, to what presented above, Figure 4 shows percentage changes in carbon and water variables between the DA and OL runs, but in this case the DA also updates the leaf mass along with LAI. The global maps clearly show that this modification in the DA technique changes the estimation of all carbon variables noticeably. As the change in the two soil moisture variables is minimal, these two variables are no further analyzed in this work.
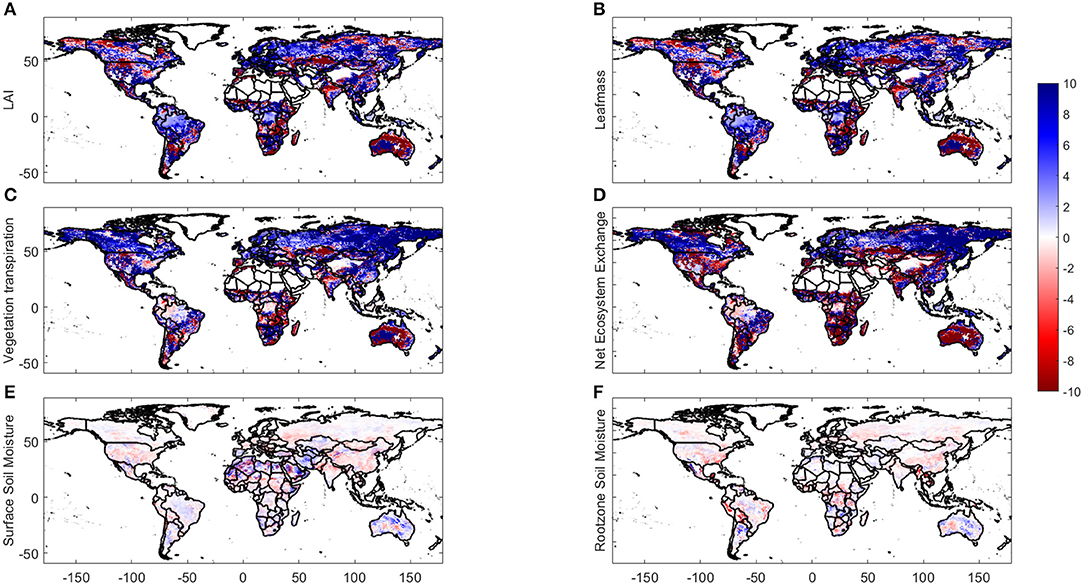
Figure 4. Same as in Figure 3 but for the DA technique that updates leaf mass along with LAI.
In both DA simulations, merging GLASS LAI brings the vegetation variables (transpiration and NEE) over forest and woodland areas to higher values compared to the OL model output. Such estimations get even higher when the leaf mass is updated along with LAI.
Validation
In this section, ACC and uRMSE are determined following the definitions presented in section System Evaluation for the OL and the two DA runs with respect to the GLEAM transpiration dataset and the FLUXCOM NEE product. Figure 5 presents the difference between the ACC obtained from the DA (with and without updated leaf mass) run and the one from the OL run with respect to the GLEAM transpiration product, whereas Figure 6 shows the same analysis for uRMSE. For ACC, the blue (red) color indicates higher (lower) correlation in DA with respect to the reference data, which means that DA improves (degrades) the estimation of transpiration. For uRMSE, the blue (red) color indicates lower (higher) error in DA compared to OL, with respect to the reference data, which shows an improvement (degradation) due to DA. In general, both DA approaches bring the land surface model estimation of transpiration closer to the reference data from GLEAM (most pixels on the maps are blue). Moreover, the estimation of transpiration improves even further (darker blue) when leaf mass is also updated compared to the DA technique that only updates LAI. In terms of ACC with respect to GLEAM transpiration, 66% of the total area shows an improvement due to DA. When the DA technique also updates the leaf mass (besides LAI), such area reaches 77%, which clearly demonstrates the superiority of the more complex DA approach in estimating transpiration. In terms of uRMSE, 70% of total area shows an improvement due to DA without the update of leaf mass, which increases to 74% when leaf mass is also updated in the system. Figure 7 shows frequency plots of ACC and uRMSE differences (between the DA and the OL simulations) for transpiration. When the leaf mass is not updated, the histograms are mostly centered around zero, but when the leaf mass is updated, these distributions are skewed toward positive values (which corresponds to higher correlations and smaller random errors), demonstrating a larger improvement in both ACC and uRMSE when the leaf mass is updated along with LAI.
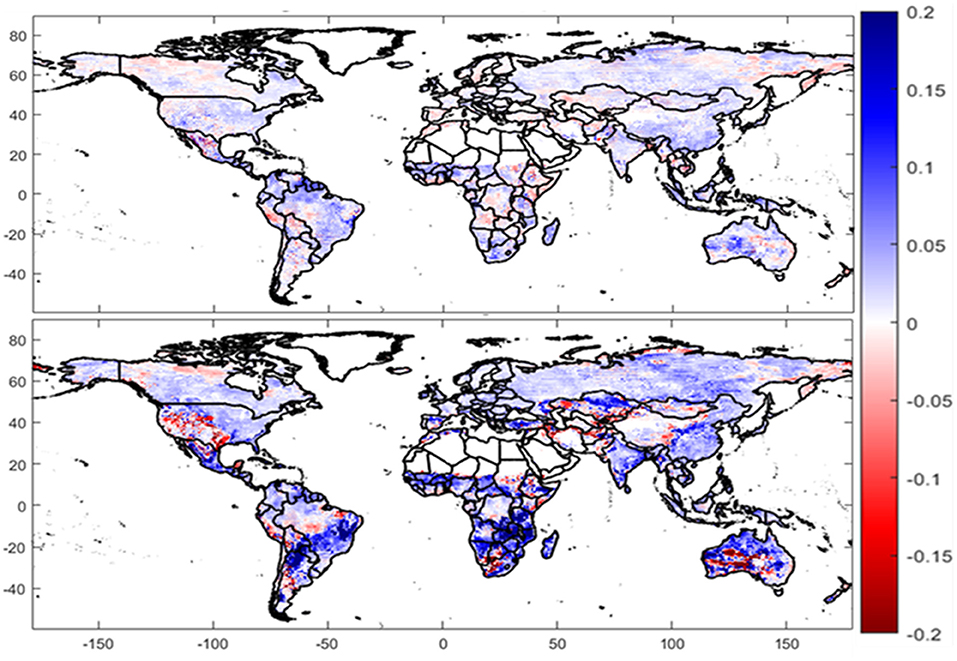
Figure 5. Difference in ACC between DA and OL with respect to GLEAM for transpiration during 2011. The upper panel shows results for GLASS LAI DA without updating the leaf mass and the lower panel shows results for GLASS LAI DA with updated leaf mass. Blue (red) color indicates improvement (degradation) after DA.
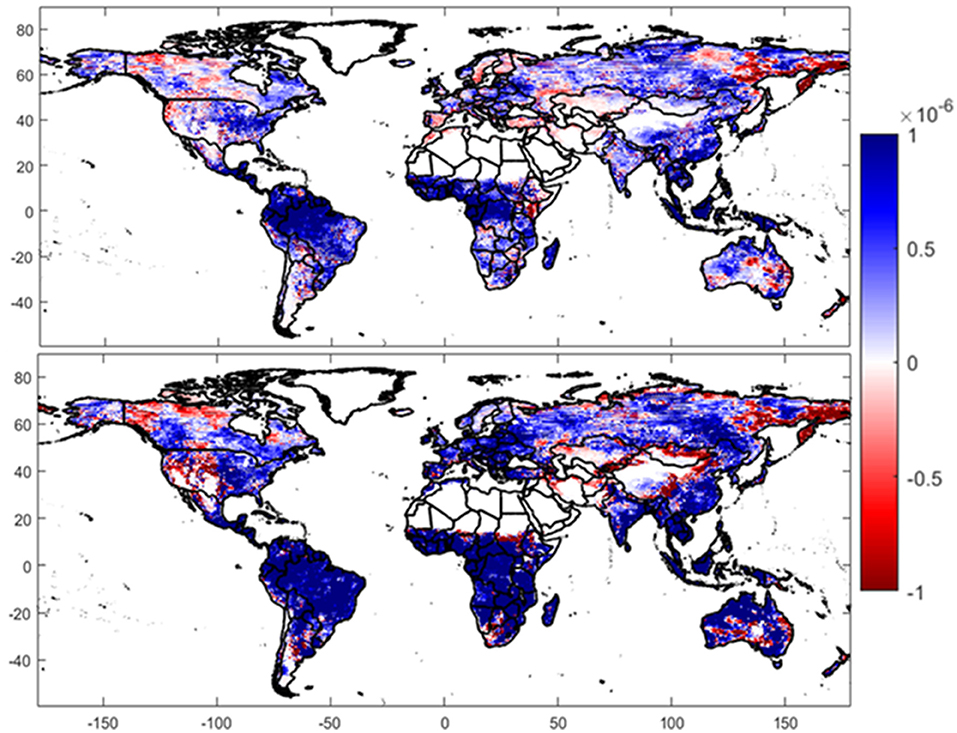
Figure 6. Difference in uRMSE between DA and OL with respect to GLEAM for transpiration during 2011. The upper panel shows results for GLASS LAI DA without updating the leaf mass and the lower panel shows results for GLASS LAI DA with updated leaf mass. Blue (red) color indicates improvement (degradation) after DA.
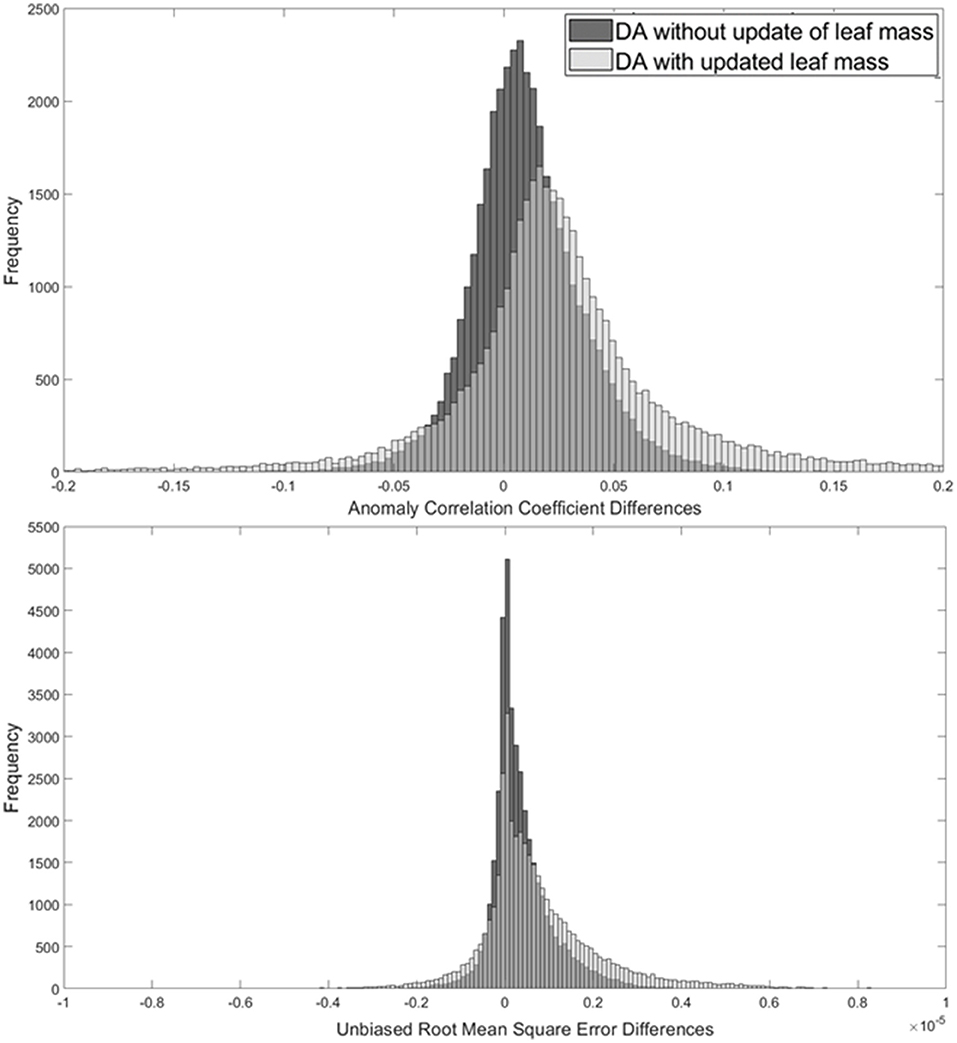
Figure 7. Frequency histograms of ACC (upper panel) and uRMSE (lower panel) differences between OL and DA for transpiration.
The global vegetation map presented in Figure 1 shows how the areas in which transpiration degrades after applying DA (i.e., red pixels in Figures 5, 6) are covered by shrubland. This may be due to the fact that the transpiration process in short-rooted plant in dry regions is highly dependent on precipitation (Cavanaugh et al., 2011). This confirms what discuss by Ling et al. (2019), who also showed that LAI assimilation is not sensitive to extreme dry regions.
Similarly, to Figures 5, 6, 8, 9 show the differences in ACC and uRMSE for NEE with respect to the FLUXCOM data. In terms of ACC, the GLASS LAI DA improves the estimation of NEE over 52% of the global land area, value that increases to 75% after updating the leaf mass. For uRMSE this improvement increases from 49% of the total area to 62% of the total area.
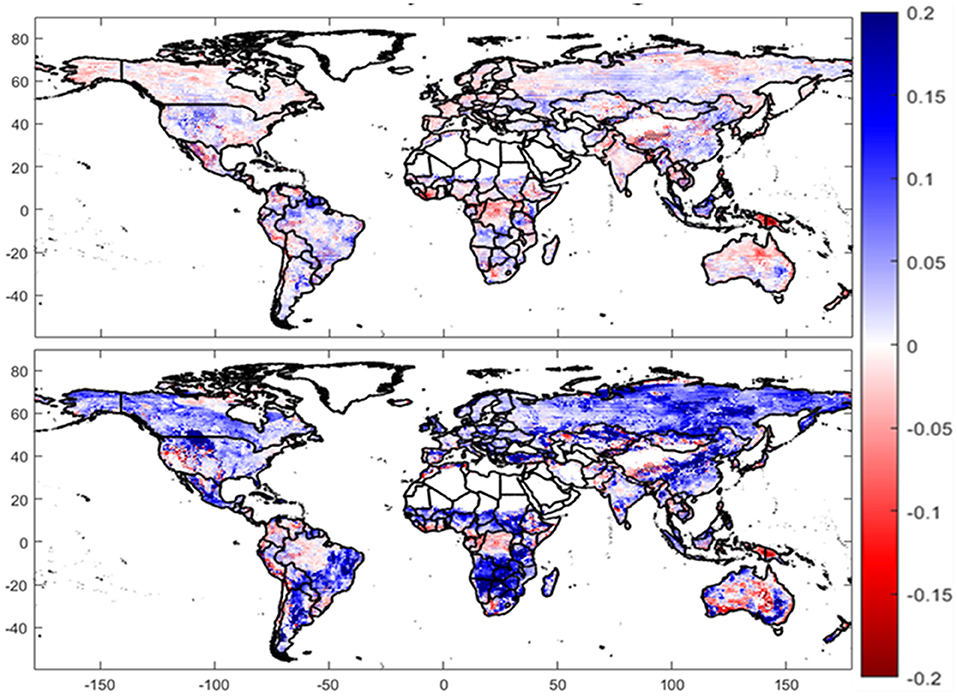
Figure 8. Same as Figure 5 but for NEE with respect to the FLUXCOM data.
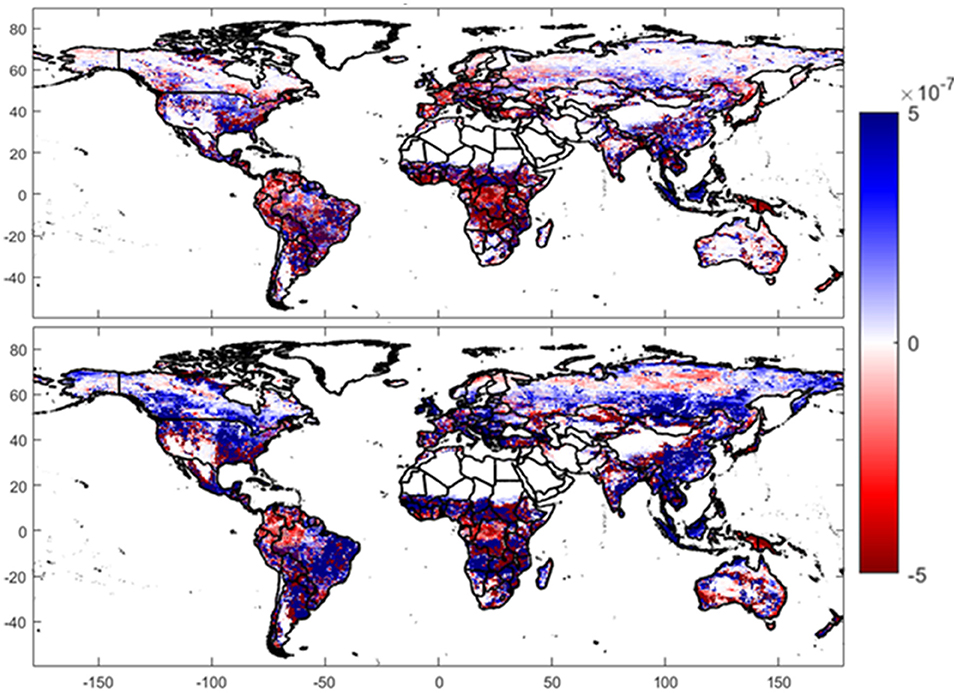
Figure 9. Same as Figure 6 but for NEE with respect to the FLUXCOM data.
This shows that the update of leaf mass along with LAI has a larger impact on NEE when compared to transpiration, as it improves the estimation of NEE over a larger area. Although limited, LAI DA without the leaf mass update improves the estimation of NEE compared to OL, especially over forest and woodland. However, the improvement becomes remarkable (and extends to more regions) when leaf mass is also updated (darker blue in the bottom panel of Figures 8, 9). Frequency plots of differences in NEE ACC and uRMSE (between the DA and the OL simulations) confirm what observed in the maps, presenting histograms skewed toward positive values when the leaf mass is updated together with LAI (Figure 10).
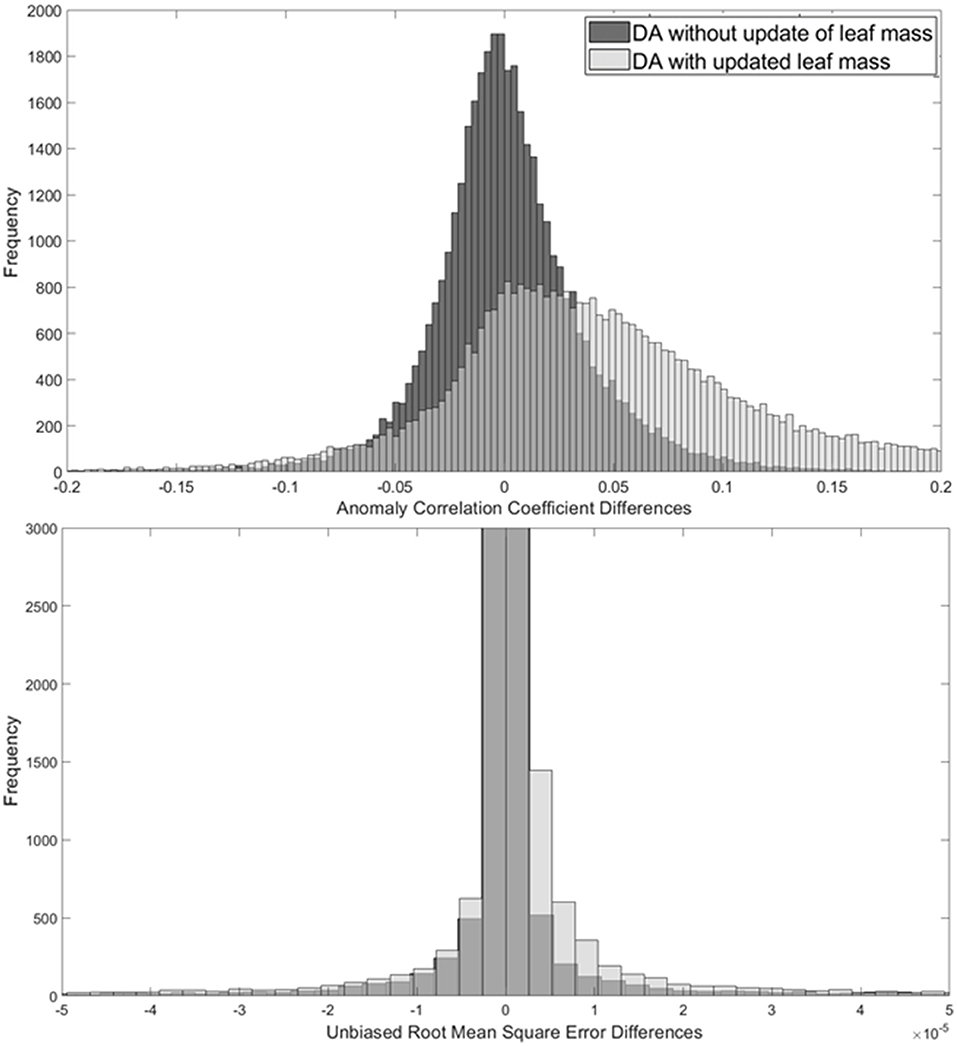
Figure 10. Same as Figure 7 but for NEE.
System Performance as a Function of Vegetation Cover
Figures 11, 13 show ACC of OL, DA without updated leaf mass, and DA with updated leaf mass for transpiration and NEE with respect to GLEAM and FLUXCOM reference datasets, respectively, as a function of vegetation type. On the other hand, Figures 12, 14 show similar analyses but for uRMSE. The black error bars in the bar plots show the 95% CIs to evaluate the statistical significance of performance differences among the three model runs.
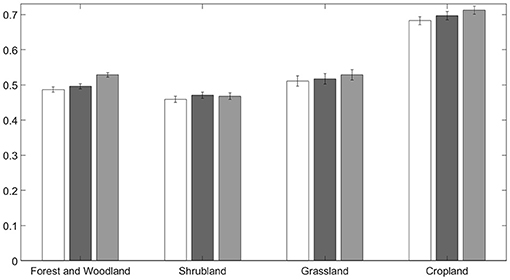
Figure 11. ACCs of transpiration for the OL and DA runs with respect to GLEAM transpiration over four main vegetation types. 95% CIs are included in the bar plots.
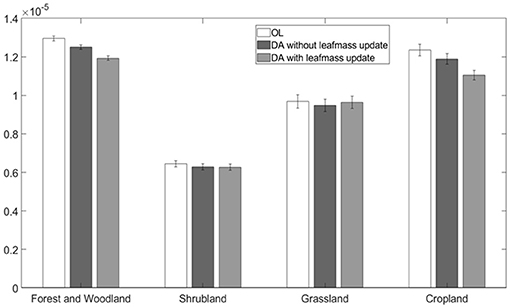
Figure 12. uRMSEs of transpiration for the OL and DA runs with respect to GLEAM transpiration over four main vegetation types. 95% CIs are included in the bar plots.
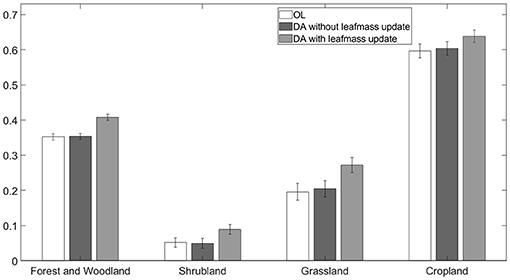
Figure 13. Same as in Figure 11 but for net ecosystem exchange. ACCs are calculated with respect to FLUXCOM NEE.
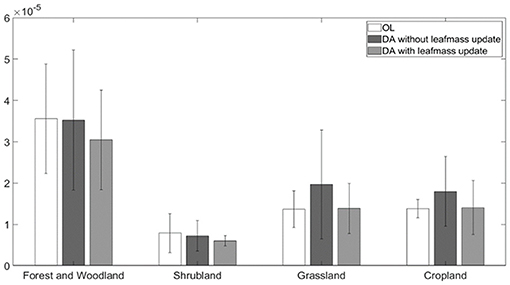
Figure 14. Same as in Figure 12 but for net ecosystem exchange. uRMSEs are calculated with respect to FLUXCOM NEE.
The highest ACCs in transpiration are obtained for cropland areas, with average values above 0.65, whereas the other three main vegetation types show similar ACC values (Figure 11). The largest ACC improvement due to the assimilation of GLASS LAI with updated leaf mass is observed in forest and woodland regions (and cropland next). The smallest DA impact is observed in shrubland regions, as anticipated above in the discussion of Figure 5. These are commonly drier regions, that would possible benefit more from the assimilation of soil moisture observations rather than vegetation information. Although the improvement in transpiration due to LAI DA is not significant at the 95% confidence level, the improvement due to the update of leaf mass with respect to the benchmark OL run is significant across forests, woodlands, and croplands. Similarly, the smallest changes in transpiration uRMSE between the OL and DA runs are observed in shrublands and grasslands (Figure 12). For cropland and forested areas, uRMSE is reduced after the application of DA and is significantly reduced at the 95% CI with the update of leaf mass when compared to the OL. This again shows that the DA has the potential to improve the estimation of transpiration over these two vegetation covers.
Figure 13 shows overall increases in the correlation coefficients of NEE (with respect to the FLUXCOM reference data) after DA is applied over all vegetation types and significant improvements (at the 95% confidence level) are observed when leaf mass is updated. As for transpiration, the highest ACCs in NEE are obtained for cropland regions, where the agreement between simulated NEE and the FLUXCOM data is at its maximum. It is important to note how the ACC differences between DA with updated leaf mass and OL are significant at the 95% confidence level across all vegetation covers, whereas the LAI update alone does not show any significant improvement with respect to the benchmark run. The smallest ACCs for NEE are observed in shrubland regions. Non-woody semiarid ecosystems strongly respond to the availability of soil water (Walther et al., 2019), but the NEE in the land surface model not only depends on soil water but also on the growth respiration of root, leaf, and wood. As LAI only provides an indicator of the greenness of the pixel, its assimilation in the model may not be enough to improve the estimation of NEE for small vegetation in semi-arid regions. FLUXCOM uses greenness observation measures along with the measure of light that is emitted by pigments in the plants that are photosynthetically active (chlorophyll fluorescence), and then uses machine learning to merge the two datasets to estimate net ecosystem exchange (Jung et al., 2019a; Walther et al., 2019). This is possibly a reason for the low correlations between NEE observations and model estimates over shrublands.
The NEE random error decreases thanks to DA with the update of leaf mass for all vegetation covers (Figure 14). Forested and cropland regions show a larger reduction in mean uRMSE when the DA updates the leaf mass compared to the OL. However, none of the uRMSE differences between DA and OL are significant at the 95% confidence level, due to the large variability around the mean (quantified by the black bars in the plot). Once again, this could be due to the different nature of the FLUXCOM observations with respect to the NEE model estimates.
Conclusions
This work showed that assimilating GLASS LAI within a land surface model using an EnKF technique had an impact on the estimation of several variables related to vegetation (e.g., LAI, leaf mass, transpiration, NEE) at the global scale. However, minimal changes were observed in water variables such as surface and rootzone soil moisture. Furthermore, updating the leaf mass along with LAI was shown to modify the estimation of carbon variables when compared to only updates LAI. As soil moisture variables has not shown noticeable changes with the application of only LAI DA even with the update of leaf mass, the future work should include the assimilation of soil moisture along with LAI.
The DA simulations have been evaluated in terms of transpiration and NEE, using two independent datasets: the GLEAM transpiration dataset and the FLUXCOM NEE product. Overall, the assimilation of GLASS LAI in Noah-MP showed potential for improving the estimation of vegetation globally. Such improvement was particularly evident in forest, woodland, and cropland regions and especially when the more complex DA technique that updated both LAI and leaf mass was adopted. Specifically, this DA approach was able to improve the estimation of transpiration and NEE with respect to the open loop run over 77 and 75% as per ACC of the global land, respectively, by bringing them closer to the reference datasets (from the GLEAM and FLUXCOM products). Nevertheless, the impact of DA on these two variables was smaller in semi-arid regions characterized by small-rooted plants (e.g., shrubs), which confirms what shown by previous regional studies that demonstrated low sensitivity of LAI assimilation to extremely dry regions. A reduction in the random error was observed in 74 and 62% of the total global area when estimating transpiration and NEE, respectively. Such improvement was particularly prominent over areas covered by forests and croplands.
Results in this work are limited by several factors. First off, the EnKF estimates the forecast error matrix as the sampling results of ensemble forecasts, which may lead to false correlations of the error matrix. Future work should evaluate the efficiency of a localization or inflation technique for initializing the forecast error matrix. Furthermore, future studies should implement bias correction techniques prior to the assimilation procedure to eliminate any bias in the observations and evaluate the impact of LAI DA (with and without the update of leaf mass) when the EnKF works under optimal condition (i.e., when there exists no bias between observations and model estimates).
Although all the data (atmospheric forcing, observations, and validation datasets) are available for multiple years, this work focused only on 1 year of data due to the large computational costs of the global DA simulations. Future studies should verify these findings using longer time series and different satellite vegetation products and validating them with a larger set of output variables. Future studies could also investigate if a changing climate has an impact on the estimation of different variables and how such estimation can be improved in future extreme climatic conditions.
Data Availability Statement
The original contributions presented in the study are included in the article/supplementary material, further inquiries can be directed to the corresponding author/s.
Author Contributions
AR performed all analyses and wrote the manuscript. XZ developed the code for the LAI data assimilation within the LIS framework. VM, PH, and TS conceptualized the experiment. AR and XZ contributed to the interpretation of results. All authors contributed to the article and approved the submitted version.
Funding
The work was funded by NASA Modeling, Analysis, and Prediction Program (MAP) program, award number is 80NSSC17K0109.
Conflict of Interest
The authors declare that the research was conducted in the absence of any commercial or financial relationships that could be construed as a potential conflict of interest.
Publisher's Note
All claims expressed in this article are solely those of the authors and do not necessarily represent those of their affiliated organizations, or those of the publisher, the editors and the reviewers. Any product that may be evaluated in this article, or claim that may be made by its manufacturer, is not guaranteed or endorsed by the publisher.
References
Albergel, C., Calvet, J.-C., Mahfouf, J.-F., Rüdiger, C., Barbu, A. L., Lafont, S., et al. (2010). Monitoring of water and carbon fluxes using a land data assimilation system: A case study for southwestern France. Hydrol. Earth Syst. Sci. Discuss. 14, 1109–1124. doi: 10.5194/hess-14-1109-2010
Albergel, C., Munier, S., Leroux, D. J., Dewaele, H., Fairbairn, D., Barbu, A. L., et al. (2017). Sequential assimilation of satellite-derived vegetation and soil moisture products using SURFEX_v8.0: LDAS-Monde assessment over the Euro-Mediterranean area. Geosci. Model Dev. Discuss. 2017, 1–53. doi: 10.5194/gmd-2017-121
Albergel, C., Zheng, Y., Bonan, B., Dutra, E., Rodríguez-Fernández, N., Munier, S., et al. (2020). Data assimilation for continuous global assessment of severe conditions over terrestrial surfaces. Hydrol. Earth Syst. Sci. 24, 4291–4316. doi: 10.5194/hess-24-4291-2020
Ball, J. T., Woodrow, I. E., and Berry, J. A. (1987). “A model predicting stomatal conductance and its contribution to the control of photosynthesis under different environmental conditions,” in Progress in Photosynthesis Research, ed J. Biggins (Dordrecht: Springer), 221–224. doi: 10.1007/978-94-017-0519-6_48
Barbu, A. L., Calvet, J.-C., Mahfouf, J.-F., Albergel, C., and Lafont, S. (2011). Assimilation of Soil Wetness Index and Leaf Area Index into the ISBA-A-gs land surface model: Grassland case study. Biogeosciences 8, 1971–1986. doi: 10.5194/bg-8-1971-2011
Bonan, B., Albergel, C., Zheng, Y., Barbu, A. L., Fairbairn, D., Munier, S., et al. (2019). An Ensemble Square Root Filter for the joint assimilation of surface soil moiture and leaf area index within LDAS-Monde: Application over the Euro-Mediterranean region [Preprint]. Hydrometeorol. Model. Approach. 2019:391. doi: 10.5194/hess-2019-391
Cavanaugh, M. L., Kurc, S. A., and Scott, R. L. (2011). Evapotranspiration partitioning in semiarid shrubland ecosystems: A two-site evaluation of soil moisture control on transpiration. Ecohydrology 4, 671–681. doi: 10.1002/eco.157
Clark, D. B., Mercado, L. M., Sitch, S., Jones, C. D., Gedney, N., Best, M. J., et al. (2011). The Joint UK Land Environment Simulator (JULES), model description - Part 2: Carbon fluxes and vegetation dynamics. Geosci. Model Dev. 4, 701–722. doi: 10.5194/gmd-4-701-2011
Dai, Y., Zeng, X., Dickinson, R. E., Baker, I., Bonan, G. B., Bosilovich, M. G., et al. (2003). The common land model. Bull. Am. Meteorol. Soc. 84, 1013–1024. doi: 10.1175/BAMS-84-8-1013
Dee, D. P. (2005). Bias and data assimilation. Q. J. R. Meteorol. Soc. 131, 3323–3343. doi: 10.1256/qj.05.137
Entekhabi, D., Reichle, R. H., Koster, R. D., and Crow, W. T. (2010). Performance metrics for soil moisture retrievals and application requirements. J. Hydrometeorol. 11, 832–840. doi: 10.1175/2010JHM1223.1
Fang, H., Jiang, C., Li, W., Wei, S., Baret, F., Chen, J. M., et al. (2013). Characterization and intercomparison of global moderate resolution leaf area index (LAI) products: Analysis of climatologies and theoretical uncertainties: INTERCOMPARISON OF GLOBAL LAI PRODUCTS. J. Geophys. Res. Biogeosci. 118, 529–548. doi: 10.1002/jgrg.20051
Frappart, F. (2020). Groundwater storage changes in the major North African transboundary aquifer systems during the GRACE Era (2003-2016). Water 12:2669. doi: 10.3390/w12102669
Gelaro, R., McCarty, W., Suárez, M. J., Todling, R., Molod, A., Takacs, L., et al. (2017). The Modern-Era retrospective analysis for research and applications, Version 2 (MERRA-2). J. Clim. 30, 5419–5454. doi: 10.1175/JCLI-D-16-0758.1
Huang, J., Ma, H., Su, W., Zhang, X., Huang, Y., Fan, J., et al. (2015). Jointly assimilating MODIS LAI and ET products into the SWAP Model for winter wheat yield estimation. IEEE J. Select. Topics Appl. Earth Observ. Remote Sensing 8, 4060–4071. doi: 10.1109/JSTARS.2015.2403135
Jin, H., Li, A., Bian, J., Nan, X., Zhao, W., Zhang, Z., et al. (2017). Intercomparison and validation of MODIS and GLASS leaf area index (LAI) products over mountain areas: A case study in southwestern China. Int. J. Appl. Earth Observ. Geoinform. 55, 52–67. doi: 10.1016/j.jag.2016.10.008
Johnston, J. (2021). Grid Value Search: GitHub. Available online at: https://github.com/jjohns60/ISMN_SoilMoisture/blob/main/gridvaluesearch.m
Jung, H. C., Getirana, A., Arsenault, K. R., Holmes, T. R. H., and McNally, A. (2019a). Uncertainties in Evapotranspiration estimates over West Africa. Remote Sensing 11:892. doi: 10.3390/rs11080892
Jung, M., Koirala, S., Weber, U., Ichii, K., Gans, F., Camps-Valls, G., et al. (2019b). The FLUXCOM ensemble of global land-atmosphere energy fluxes. Sci. Data 6:74. doi: 10.1038/s41597-019-0076-8
Jung, M., Schwalm, C., Migliavacca, M., Walther, S., Camps-Valls, G., Koirala, S., et al. (2020). Scaling carbon fluxes from eddy covariance sites to globe: Synthesis and evaluation of the FLUXCOM approach. Biogeosciences 17, 1343–1365. doi: 10.5194/bg-17-1343-2020
Katzfuss, M., Stroud, J. R., and Wikle, C. K. (2016). Understanding the ensemble kalman filter. Am. Stat. 70, 350–357. doi: 10.1080/00031305.2016.1141709
Kumar, S., Peterslidard, C., Tian, Y., Houser, P., Geiger, J., Olden, S., et al. (2006). Land information system: An interoperable framework for high resolution land surface modeling. Environ. Modell. Softw. 21, 1402–1415. doi: 10.1016/j.envsoft.2005.07.004
Kumar, S. V., Mocko, D. M., Wang, S., Peters-Lidard, C. D., and Borak, J. (2019). Assimilation of remotely sensed leaf area index into the Noah-MP land surface model: Impacts on water and carbon fluxes and states over the Continental U.S. J. Hydrometeorol. 20:7. doi: 10.1175/JHM-D-18-0237.1
Kumar, S. V., Reichle, R. H., Peters-Lidard, C. D., Koster, R. D., Zhan, X., Crow, W. T., et al. (2008). A land surface data assimilation framework using the land information system: Description and applications. Adv. Water Resour. 31, 1419–1432. doi: 10.1016/j.advwatres.2008.01.013
Liang, S., Zhao, X., Liu, S., Yuan, W., Cheng, X., Xiao, Z., et al. (2013). A long-term Global LAnd Surface Satellite (GLASS) data-set for environmental studies. Int. J. Digital Earth 6, 5–33. doi: 10.1080/17538947.2013.805262
Ling, X. L., Fu, C. B., Guo, W. D., and Yang, Z. -L. (2019). Assimilation of remotely sensed LAI into CLM4CN using DART. J. Adv. Modeling Earth Systems. 11, 2768–2786. doi: 10.1029/2019MS001634
Littell, J. S., McKenzie, D., Kerns, B. K., Cushman, S., and Shaw, C. G. (2011). Managing uncertainty in climate-driven ecological models to inform adaptation to climate change. Ecosphere 2:art102. doi: 10.1890/ES11-00114.1
Maggioni, V., and Houser, P. R. (2017). “Soil Moisture Data Assimilation,” in Data Assimilation for Atmospheric, Oceanic and Hydrologic Applications (Vol. III), eds S. Park and L. Xu (Cham: Springer). doi: 10.1007/978-3-319-43415-5_9
Martens, B., Miralles, D. G., Lievens, H., van der Schalie, R., de Jeu, R. A. M., Fernández-Prieto, D., et al. (2017). GLEAM v3: Satellite-based land evaporation and root-zone soil moisture. Geosci. Model Dev. 10, 1903–1925. doi: 10.5194/gmd-10-1903-2017
Miralles, D. G., Holmes, T. R. H., De Jeu, R. A. M., Gash, J. H., Meesters, A. G. C. A., and Dolman, A. J. (2011). Global land-surface evaporation estimated from satellite-based observations. Hydrol. Earth Syst. Sci. 15, 453–469. doi: 10.5194/hess-15-453-2011
Nearing, G. S., Crow, W. T., Thorp, K. R., Moran, M. S., Reichle, R. H., and Gupta, H. V. (2012). Assimilating remote sensing observations of leaf area index and soil moisture for wheat yield estimates: An observing system simulation experiment: ENKF AND SIRF OSSES FOR WHEAT YIELD. Water Resour. Res. 48:11420. doi: 10.1029/2011WR011420
Niu, G.-Y., and Yang, Z.-L. (2007). An observation-based formulation of snow cover fraction and its evaluation over large North American river basins. J. Geophys. Res. 112:D21. doi: 10.1029/2007JD008674
Niu, G.-Y., Yang, Z.-L., Mitchell, K. E., Chen, F., Ek, M. B., Barlage, M., et al. (2011). The community Noah land surface model with multiparameterization options (Noah-MP): 1. Model description and evaluation with local-scale measurements. J. Geophys. Res. 116:D12. doi: 10.1029/2010JD015139
Pan, M., and Wood, E. F. (2006). Data assimilation for estimating the terrestrial water budget using a constrained ensemble kalman filter. J. Hydrometeorol. 7, 534–547. doi: 10.1175/JHM495.1
Peterson, D. W., Kerns, B. K., and Dodson, E. K. (2014). Climate Change Effects on Vegetation in the Pacific Northwest: A Review and Synthesis of the Scientific Literature and Simulation Model Projections. Portland, OR: U.S. Department of Agriculture, Forest Service, Pacific Northwest Research Station. doi: 10.2737/PNW-GTR-900
Rahman, A., Zhang, X., Xue, Y., Houser, P., Sauer, T., Kumar, S., et al. (2020). A synthetic experiment to investigate the potential of assimilating LAI through direct insertion in a land surface model. J. Hydrol. X 9:100063. doi: 10.1016/j.hydroa.2020.100063
Rees, W. G., and Danks, F. S. (2007). Derivation and assessment of vegetation maps for reindeer pasture analysis in Arctic European Russia. Polar Record 43:6420. doi: 10.1017/S0032247407006420
Reichle, R. H. (2008). Data assimilation methods in the Earth sciences. Adv. Water Resour. 31, 1411–1418. doi: 10.1016/j.advwatres.2008.01.001
Reichle, R. H., Crow, W. T., and Keppenne, C. L. (2008). An adaptive ensemble Kalman filter for soil moisture data assimilation: ADAPTIVE ENSEMBLE FILTER FOR SOIL MOISTURE ASSIMILATION. Water Resour. Res. 44:6357. doi: 10.1029/2007WR006357
Reichle, R. H., Walker, J. P., Koster, R. D., and Houser, P. R. (2002). Extended versus ensemble kalman filtering for land data assimilation. J. Hydrometeorol. 3, 728–740. doi: 10.1175/1525-7541(2002)003<0728:EVEKFF>2.0.CO;2
Rodell, M., Houser, P. R., Jambor, U., Gottschalck, J., Mitchell, K., Meng, C.-J., et al. (2004). The global land data assimilation system. Bull. Am. Meteorol. Soc. 85, 381–394. doi: 10.1175/BAMS-85-3-381
Tesfagiorgis, K., Mahani, S. E., Krakauer, N. Y., and Khanbilvardi, R. (2011). Bias correction of satellite rainfall estimates using a radar-gauge product - a case study in Oklahoma (USA). Hydrol. Earth Syst. Sci. 15, 2631–2647. doi: 10.5194/hess-15-2631-2011
Tramontana, G., Jung, M., Schwalm, C. R., Ichii, K., Camps-Valls, G., Ráduly, B., et al. (2016). Predicting carbon dioxide and energy fluxes across global FLUXNET sites with regression algorithms. Bio. Geosciences. 13, 4291–4313. doi: 10.5194/bg-13-4291-2016
Tucker, C. J., Pinzon, J. E., Brown, M. E., Slayback, D. A., Pak, E. W., Mahoney, R., et al. (2005). An extended AVHRR 8-km NDVI dataset compatible with MODIS and SPOT vegetation NDVI data. Int. J. Remote Sens. 26, 4485–4498. doi: 10.1080/01431160500168686
Walther, S., Duveiller, G., Jung, M., Guanter, L., Cescatti, A., and Camps-Valls, G. (2019). Satellite observations of the contrasting response of trees and grasses to variations in water availability. Geophys. Res. Lett. 46, 1429–1440. doi: 10.1029/2018GL080535
Wullschleger, S. D., Epstein, H. E., Box, E. O., Euskirchen, E. S., Goswami, S., Iversen, C. M., et al. (2014). Plant functional types in Earth system models: Past experiences and future directions for application of dynamic vegetation models in high-latitude ecosystems. Ann. Bot. 114, 1–16. doi: 10.1093/aob/mcu077
Xiao, Z., Liang, S., Wang, J., Xiang, Y., Zhao, X., and Song, J. (2016). Long-time-series global land surface satellite leaf area index product derived from MODIS and AVHRR surface reflectance. IEEE Transact. Geosci. Remote Sensing 54, 5301–5318. doi: 10.1109/TGRS.2016.2560522
Yang, M.-S., Wu, M.-C., and Liu, J.-K. (2012). “Analysis of spatial characteristics for landslides vegetation restoration monitoring by LiDAR surface roughness data and multispectrum imagery,” in 2012 IEEE International Geoscience and Remote Sensing Symposium (Munich: IEEE), 7561–7564. doi: 10.1109/IGARSS.2012.6351912
Keywords: land surface model, dynamic vegetation model, data assimilation, leaf area index, leaf mass, Ensemble Kalman Filter
Citation: Rahman A, Zhang X, Houser P, Sauer T and Maggioni V (2022) Global Assimilation of Remotely Sensed Leaf Area Index: The Impact of Updating More State Variables Within a Land Surface Model. Front. Water 3:789352. doi: 10.3389/frwa.2021.789352
Received: 04 October 2021; Accepted: 09 December 2021;
Published: 04 January 2022.
Edited by:
Yanjun Gan, University of Texas at Arlington, United StatesReviewed by:
Tugrul Yilmaz, Middle East Technical University, TurkeyXianhong Xie, Beijing Normal University, China
Guocan Wu, Beijing Normal University, China
Copyright © 2022 Rahman, Zhang, Houser, Sauer and Maggioni. This is an open-access article distributed under the terms of the Creative Commons Attribution License (CC BY). The use, distribution or reproduction in other forums is permitted, provided the original author(s) and the copyright owner(s) are credited and that the original publication in this journal is cited, in accordance with accepted academic practice. No use, distribution or reproduction is permitted which does not comply with these terms.
*Correspondence: Azbina Rahman, arahma19@gmu.edu